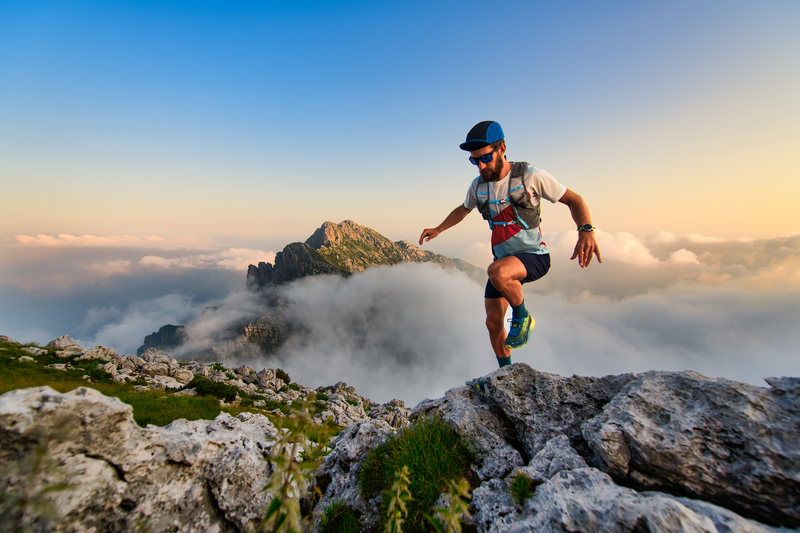
94% of researchers rate our articles as excellent or good
Learn more about the work of our research integrity team to safeguard the quality of each article we publish.
Find out more
REVIEW article
Front. Mol. Neurosci. , 07 April 2022
Sec. Methods and Model Organisms
Volume 15 - 2022 | https://doi.org/10.3389/fnmol.2022.873520
This article is part of the Research Topic Zebrafish as a Tool for Neurosciences: Evolutionary Conservation and Translational Relevance View all 7 articles
Sleep disorders and chronic sleep disturbances are common and are associated with cardio-metabolic diseases and neuropsychiatric disorders. Several genetic pathways and neuronal mechanisms that regulate sleep have been described in animal models, but the genes underlying human sleep variation and sleep disorders are largely unknown. Identifying these genes is essential in order to develop effective therapies for sleep disorders and their associated comorbidities. To address this unmet health problem, genome-wide association studies (GWAS) have identified numerous genetic variants associated with human sleep traits and sleep disorders. However, in most cases, it is unclear which gene is responsible for a sleep phenotype that is associated with a genetic variant. As a result, it is necessary to experimentally validate candidate genes identified by GWAS using an animal model. Rodents are ill-suited for this endeavor due to their poor amenability to high-throughput sleep assays and the high costs associated with generating, maintaining, and testing large numbers of mutant lines. Zebrafish (Danio rerio), an alternative vertebrate model for studying sleep, allows for the rapid and cost-effective generation of mutant lines using the CRISPR/Cas9 system. Numerous zebrafish mutant lines can then be tested in parallel using high-throughput behavioral assays to identify genes whose loss affects sleep. This process identifies a gene associated with each GWAS hit that is likely responsible for the human sleep phenotype. This strategy is a powerful complement to GWAS approaches and holds great promise to identify the genetic basis for common human sleep disorders.
Over 10% of Americans suffer from a clinically significant sleep disorder (Ram et al., 2010) and 25%–30% of adults worldwide report chronic sleep disturbances (Stranges et al., 2012), which can be associated with cardio-metabolic and neuropsychiatric disorders (Luyster et al., 2012; Fernandez-Mendoza and Vgontzas, 2013). In addition, up to 90% of individuals with a mental illness report sleep disturbances (Krystal, 2012), which may exacerbate underlying neurological symptoms of anxiety and related disorders (Cox and Olatunji, 2016). Despite the impact of poor sleep quality on human health, current options for treating sleep disorders are limited, and sleep disorders thus represent an unmet public health problem (Colten and Altevogt, 2006). This limitation is partly due to our poor understanding of genetic and neuronal mechanisms that regulate sleep, resulting in few known therapeutic targets. Thus, identifying genes that underlie human sleep disorders is a critical first step in order to develop novel therapies. Genes that underlie narcolepsy (Mahoney et al., 2019), some circadian sleep disorders (Gentry et al., 2021), and a small number of other sleep disorders (Gentry et al., 2021) have been identified based on studies using humans and animal models, but these disorders represent only a small fraction of commonly reported sleep disturbances. Nevertheless, these discoveries have already led to novel therapies. For example, the discoveries that narcolepsy results from loss of signaling by the neuropeptide hypocretin/orexin (Chemelli et al., 1999; Lin et al., 1999), and that hypocretin/orexin signaling is arousing (Adamantidis et al., 2007), led to the development of hypocretin/orexin receptor antagonists as an effective treatment for insomnia (Sun et al., 2021). Conversely, hypocretin/orexin receptor agonists are currently under development as therapies for narcolepsy (Sun et al., 2021). Most experimental sleep studies use model organisms such as rodents, zebrafish, fruit flies, and nematodes, and these studies have identified several genetic pathways that regulate sleep (Oikonomou and Prober, 2017; Saper and Fuller, 2017; Scammell et al., 2017; Liu and Dan, 2019). However, the relevance of these pathways to human sleep disorders is unclear. An alternative approach is to use human genetic studies to identify candidate genes that may underlie human sleep disorders, and then use animal models to validate these candidate genes, determine how they affect sleep, and develop therapeutics.
GWAS aim to identify associations between genetic variants, such as single-nucleotide polymorphisms (SNPs), and human traits or diseases from large population samples (see Uffelmann et al., 2021 for review). By correlating the frequency at which genetic variants are associated with a specific trait, this approach can identify genomic regions associated with that trait, also known as genomic risk loci. Since the introduction of GWAS, numerous genomic risk loci associated with various traits and diseases have been identified, including obesity (Frayling et al., 2007), autoimmune disorders (Siminovitch, 2004), and neuropsychiatric disorders (Schizophrenia Working Group of the Psychiatric Genomics, 2014; Grove et al., 2019; Levey et al., 2021). Genetic insights from some of these studies have led to the development of novel drugs to treat specific diseases (Moschen et al., 2019). Several GWAS that include hundreds of thousands to over a million human subjects have recently been described that analyze both subjective and objective sleep data (Gottlieb et al., 2015; Hu et al., 2016; Jones et al., 2016, 2019; Lane et al., 2016, 2017, 2019; Hammerschlag et al., 2017; Doherty et al., 2018; Dashti et al., 2019, 2021; Jansen et al., 2019; Wang et al., 2019; Garfield, 2021). Most of these studies utilized datasets from large biomedical databases such as the UK Biobank (Bycroft et al., 2018) and 23andMe (Eriksson et al., 2010), which primarily include individuals of European descent. Self-reported sleep traits assayed in these studies include sleep duration, difficulty initiating sleep, difficulty maintaining sleep, and excessive daytime sleepiness. Although there are limitations to self-reported sleep data (Lauderdale et al., 2008; Matthews et al., 2018), the validity of self-reported sleep duration data is supported by studies that use wrist-worn accelerometers (Doherty et al., 2018; Dashti et al., 2019; Jones et al., 2019). These studies have identified a large number of genomic loci associated with human sleep traits and sleep disorders, but in most cases it is unclear which gene within a particular genomic locus is associated with the sleep phenotype. This can be due to the presence of multiple genetic variants that are associated with the same phenotype but overlap with multiple neighboring genes (Figure 1A). In addition, even when only a single gene is located near a genetic variant, the gene responsible for the phenotype can be located in a more distant genomic region (Porcu et al., 2019). For example, SNPs associated with obesity and type-2 diabetes that are located in noncoding regions of the FTO gene locus interact with the promoter of the IRX3 gene at megabase distances, thus affecting the expression of IRX3, which regulates body weight in mice (Smemo et al., 2014; Claussnitzer et al., 2015). Thus, while it is often assumed that the gene nearest to a genetic variant is responsible for a phenotype, this is often not the case. Strategies such as heuristic linkage disequilibrium, penalized regression, and Bayesian fine-mapping have been used to first narrow down causal variants and then genes using variant-to-gene mapping (Schaid et al., 2018). More recently, GWAS data and chromatin conformation capture were used for variant-to-gene mapping to identify 88 candidate insomnia risk genes (Palermo et al., 2021). The orthologs of many of these genes were then functionally tested using RNAi knockdown in Drosophila, which identified a small number of genes whose loss resulted in a sleep phenotype. The function of one of these genes was tested using CRISPR/Cas9 knockdown in zebrafish, which resulted in a phenotype similar to that observed in Drosophila. Thus, while variant-to-gene mapping methods can narrow down a list of candidate genes (Chesi et al., 2019), these candidates must ultimately be validated using an animal model in order to attribute causality to a particular gene. Rodent models have provided many insights into mechanisms that underlie sleep (Saper and Fuller, 2017; Scammell et al., 2017; Liu and Dan, 2019), but are poorly suited for validating large numbers of candidate disease risk genes due to the high cost and labor associated with generating, maintaining, and testing large collections of rodent mutant lines. In contrast, the use of genetics to study sleep was largely pioneered using Drosophila (Hendricks et al., 2000; Shaw et al., 2000; Cirelli et al., 2005), which has been used to identify a large number of genes and neuronal circuits that regulate sleep (Allada et al., 2017; Artiushin and Sehgal, 2017). Unlike mice, Drosophila are well suited for high-throughput sleep studies that monitor sleep/wake states using beam breaks (Hendricks et al., 2000; Shaw et al., 2000) or, similar to zebrafish, a videotracking system (Guo et al., 2016). RNAi libraries for conditional gene knockdown in specific cell types (Dietzl et al., 2007) are available to quickly test many candidate genes for sleep phenotypes, an approach that has identified genes that affect sleep and the cells in which they function. However, Drosophila have several disadvantages as a sleep model. First, there is little anatomical or molecular homology between vertebrate and invertebrate brains (Lichtneckert and Reichert, 2005), which hinders translating Drosophila findings to mammals. In addition, some genes known to regulate vertebrate sleep lack clear invertebrate orthologs (Ferreira et al., 2017; de Lecea, 2021), while some genes that affect invertebrate sleep lack clear vertebrate orthologs (Koh et al., 2008; Toda et al., 2019). Finally, the sleep-wake cycle of Drosophila, with wakefulness peaks in the morning and evening and a mid-day siesta (Hendricks et al., 2000; Shaw et al., 2000), differs from the diurnal sleep-wake cycle of humans and some vertebrates, including zebrafish.
Figure 1. Examples of human GWAS data for excessive daytime sleepiness (EDS). Regional association plots for genome-wide significant associations for rs843372 (A) and rs1846644 (B) with EDS are shown. Genes within each region are shown in the lower panels. Blue lines indicate recombination rate. Filled circles show the −log10 P-value for each SNP, with the named SNP shown in purple. Additional SNPs are colored according to correlation (r2) with the lead SNP, estimated by LocusZoom based on the CEU HapMap haplotypes. Dashed black lines indicate the genome-wide significance threshold (P < 5 × 10−8). (A) rs843372 and nearby SNPs that have a genome-wide significant association with EDS are spread out over several different genes, making it difficult to predict which gene is associated with EDS. (B) rs1846644 and nearby SNPs that have a genome-wide significant association with EDS are located within introns of the KSR2 gene locus, suggesting that variation in KSR2 might be associated with EDS. Reproduced and modified from Lee et al. (2019).
A key strength of zebrafish is that technologies such as CRISPR/Cas9 (Hwang et al., 2013) and high-throughput behavioral assays (Prober et al., 2006; Thyme et al., 2019) allow large numbers of mutant lines to be generated and behaviorally tested efficiently and cost-effectively. Using zebrafish, one can systematically knock out and behaviorally phenotype each candidate gene within a genomic locus identified by GWAS in order to identify the causative gene. Indeed, a recent zebrafish screen used CRISPR/Cas9-induced targeted mutations to validate 132 candidate schizophrenia risk genes (Thyme et al., 2019) at 108 genomic loci that were identified by human GWAS (Schizophrenia Working Group of the Psychiatric Genomics, 2014). This targeted loss-of-function approach has also been used to characterize the swimming behavior of zebrafish containing mutations in the orthologs of 90 genes that have been associated with psychiatric disorders (Tang et al., 2020). Most recently, zebrafish were used to validate a candidate insomnia risk gene identified from a targeted Drosophila screen (Palermo et al., 2021) based on a human insomnia GWAS (Jansen et al., 2019). Automated annotation of the zebrafish genome found that over 70% of human genes have at least one zebrafish ortholog (Howe et al., 2013), and manual annotation suggests that this number is closer to 90% (Tran and Prober, unpublished observation). Thus, most human candidate genes will have at least one zebrafish ortholog.
Sleep can be defined on the basis of three behavioral criteria (Joiner, 2016). First, sleep is associated with behavioral quiescence that is rapidly reversible, which distinguishes it from paralysis or coma. Second, sleep is associated with an increased arousal threshold, which distinguishes sleep from quiet wakefulness. Third, sleep is controlled by a homeostatic process, such that sleep-deprived animals exhibit increased sleep need. Using these criteria, sleep-like states have been described in several animal models, including fruit flies, nematodes, jellyfish, and zebrafish (Hendricks et al., 2000; Shaw et al., 2000; Zhdanova et al., 2001; Prober et al., 2006; Van Buskirk and Sternberg, 2007; Yokogawa et al., 2007; Raizen et al., 2008; Nath et al., 2017). Since the first description of zebrafish sleep (Zhdanova et al., 2001), Danio rerio has emerged as a useful animal model for vertebrate sleep, with a large body of evidence demonstrating conserved genetic, pharmacological, neuronal, and anatomical aspects of mammalian sleep (Barlow and Rihel, 2017; Levitas-Djerbi and Appelbaum, 2017; Oikonomou and Prober, 2017). Larval zebrafish behavior can be studied using a high-throughput automated videotracking system (Figure 2A). Individual larval zebrafish are placed in each well of a 96-well plate, and the behavior of each animal is recorded using an infrared camera (Prober et al., 2006). Starting at 5-days post-fertilization (dpf), zebrafish exhibit robust diurnal rest/activity cycles.
Figure 2. Zebrafish sleep assays. (A) Schematic of sleep assay. Larval zebrafish at 4-dpf obtained from an in-cross of heterozygous mutant fish are individually placed in each well of a 96-well plate that is filled with E3 medium and is maintained at 28.5°C by recirculating heated water. The plate is placed in a videotracking system, and is illuminated from below with white light from 9 a.m. to 11 p.m. and continuously with infrared light. The behavior of each fish is monitored by an infrared camera for up to 72 h. Animals are genotyped by PCR after the behavioral experiment is complete. (B) Example of data generated using the sleep assay. Zebrafish are diurnal, and thus sleep more at night compared to the day. Treatment of WT larval zebrafish with an EGFR small molecule inhibitor results in reduced sleep during both the night and day compared to siblings treated with DMSO vehicle control. (C) Schematic of arousal threshold assay. The videotracking system shown in (A) is modified by adding an Arduino board to control two solenoids that deliver a mechano-acoustic tapping stimulus to the 96-well plate during the night. The stimuli are applied over a range of intensities in random order at 1-min intervals while behavior is monitored. (D) Example of data generated using the arousal threshold assay. The fraction of animals that exhibit a startle response to each stimulus is plotted against the stimulus intensity (arbitrary units, a.u.) to generate a stimulus-response curve. The effective tap power 50 (ETP50) is the stimulus intensity that elicits 50% of the maximal response. Treatment of WT zebrafish with an EGFR small molecule inhibitor results in a decreased ETP50 (red dashed line) compared to siblings treated with DMSO vehicle control (blue dashed line), indicating that inhibition of EGFR results in a decreased arousal threshold. (E) Schematic of sleep deprivation assay. Larval zebrafish are placed in each well of a 96-well plate that is cut in half, and both halves of the plate are placed in the videotracking system shown in (A). Behavior is first monitored for 24 h to quantify baseline sleep levels. On the second night, half of the plate is removed from the videotracker and is subjected to a complex mechano-acoustic perturbation in the dark for the first 6 h of the night, while the other half plate is left in the videotracker to serve as a non-perturbed control. The half-plate that is subjected to the perturbation is then returned to the videotracker, and the amount of recovery sleep is quantified by comparing the amount of sleep during the remaining 4 h of the night for the perturbed and non-perturbed animals. The amount of recovery sleep can also be normalized by comparison to the amount of sleep during the baseline night (not shown). (F–I) A sleep deprivation assay should result in increased sleep following perturbation during the normal sleep phase, but not following perturbation during the normal wake phase. Using this assay, there is an increase in sleep after the perturbation when it is applied at night (F,G) but not when it is applied during the day (H,I). Fish are maintained in darkness (gray shading) after the perturbation in order to avoid the arousing effect of light on behavior. Dashed boxes indicate the 4 h period during which the amount of recovery sleep is quantified. ***P < 0.005, n.s. = not significant, by Mann-Whitney test. Figures are reproduced and modified from Prober et al. (2006) and Lee et al. (2019).
In order to measure arousal threshold, several assays that use visual or mechano-acoustic stimuli have been developed (Zhdanova et al., 2001; Prober et al., 2006; Elbaz et al., 2012; Singh et al., 2015). For example, one of these assays uses the same 96-well plate and automated videotracking system that is used to monitor locomotor activity, but subjects the animals to a tapping stimulus that is applied over a wide range of intensities, with multiple trials at each intensity, for several hours at night (Figure 2C; Singh et al., 2015). The stimuli are applied at 1-minute intervals, which is long enough to prevent habituation (Burgess and Granato, 2007; Woods et al., 2014). By quantifying the fraction of animals that show a behavioral response at each stimulus intensity, the stimulus intensity that elicits 50% of the maximal response (effective tap power 50, ETP50) can be calculated and serves as a measure of arousal threshold. Behavioral responses to these stimuli can also be used to identify differences in sleep depth by comparing the fraction of sleeping animals of different genotypes that respond to the stimulus (Oikonomou et al., 2019). Assays that use different stimuli have all found that larval zebrafish show a large increase in arousal threshold following 1 minute of inactivity, indicating that 1 or more minutes of rest corresponds to a sleep-like state in larval zebrafish (Prober et al., 2006; Elbaz et al., 2012; Singh et al., 2015).
Several assays have been developed to measure sleep homeostasis in both larval (Zhdanova et al., 2001; Lee et al., 2019; Leung et al., 2019; Oikonomou et al., 2019; Reichert et al., 2019) and adult (Yokogawa et al., 2007) zebrafish. For example, one assay uses a 96-well plate that is cut in half (Figure 2E; Lee et al., 2019). After a night of baseline sleep is recorded using the videotracking system, the animals in one half-plate are subjected to a complex mechano-acoustic stimulus during the first 6 hours of the night, while animals in the other half-plate are not perturbed and serve as controls. Sleep rebound is then quantified by comparing the amount of sleep during the remaining 4 hours of the night between perturbed and non-perturbed animals. Importantly, sleep rebound is only observed when animals are perturbed at night and not when they are perturbed during the day (Figures 2F–I), indicating that rebound sleep is due to loss of sleep rather than other factors such as stress or fatigue.
The zebrafish has several features that make it a useful sleep model. Due to their small size and robust sleep/wake cycles that appear as early as 5-dpf, larval zebrafish are amenable to high-throughput screens that have identified genes (Chiu et al., 2016) and drugs (Rihel et al., 2010) that affect sleep. Indeed, screens have shown that most drugs that affect mammalian sleep have similar effects on larval zebrafish (Renier et al., 2007; Rihel et al., 2010), suggesting that similar mechanisms underlie mammalian and zebrafish sleep. Due to their small size, many larval zebrafish can be tested in parallel in 96-well plates, which provides sufficient statistical power to detect even subtle sleep phenotypes. The larval zebrafish brain shares many anatomical similarities with that of mammals (Tropepe and Sive, 2003), including structures thought to be most important for mammalian sleep, such as the hypothalamus and hindbrain, as well as several neuropeptidergic and neuromodulatory populations (Barlow and Rihel, 2017; Levitas-Djerbi and Appelbaum, 2017; Oikonomou and Prober, 2017). Indeed, neuropeptides and neuromodulators that affect mammalian sleep have similar functions in zebrafish (Prober et al., 2006; Singh et al., 2015; Chen A. et al., 2016, Chen S. et al., 2016, Chen S. et al., 2017; Chiu et al., 2016; Leung et al., 2019; Oikonomou et al., 2019; Reichert et al., 2019). There is also evidence that zebrafish sleep may be associated with brain oscillations that are similar to those observed during mammalian sleep (Leung et al., 2019). However, the larval zebrafish brain has >100 fold fewer neurons than the rodent brain, thus providing a more tractable system to study basic neuronal mechanisms that underlie vertebrate sleep. Zebrafish are also diurnal (Hurd et al., 1998), and thus may be a better model than commonly used nocturnal rodents for the circadian regulation of human sleep. Indeed, genetic studies in zebrafish provided long-sought proof that melatonin is required for circadian regulation of sleep in a diurnal vertebrate animal (Gandhi et al., 2015). Due to its transparency at embryonic and larval stages, zebrafish are amenable to non-invasive optogenetic stimulation of genetically specified neurons (Singh et al., 2015) and whole-brain neuronal activity monitoring (Ahrens et al., 2013) that can be used to identify and manipulate specific neuronal populations in behaving animals (Del Bene and Wyart, 2012; Singh et al., 2015; Orger and de Polavieja, 2017; Lee et al., 2020). Genetic techniques such as CRISPR/Cas9 mutagenesis (Hwang et al., 2013) and Tol2 transgenesis (Kawakami et al., 2000) allow rapid and cost-effective generation of mutant and transgenic lines.
Finally, there are several examples where mutation of a gene in rodents results in embryonic or perinatal lethality, but knocking out the zebrafish ortholog results in a sleep phenotype with no apparent developmental defects. As a result, it has been possible to use genetics to study the roles of some genes in sleep using zebrafish that has not been possible using rodents. For instance, mutation of dopamine beta hydroxylase (dbh), which is required for the synthesis of noradrenaline, is embryonic lethal in mice, while zebrafish dbh mutants show a large increase in sleep but no developmental defects (Singh et al., 2015). It is unclear why some genes are required for rodent but not zebrafish development, but this may be due in part to challenges of development in utero for rodents compared to ex utero for zebrafish, as well as the dependence of newborn rodents, but not zebrafish, on their mother for survival. Another example is melatonin, whose role in mammalian sleep has not been evaluated using genetics because most laboratory mouse strains produce little or no melatonin. This lack of melatonin is due to the mutation of genes that are required for melatonin synthesis (Goto et al., 1989), likely due to selection pressures in the laboratory setting. Thus, the use of zebrafish genetics has facilitated the discovery of important mechanisms that regulate sleep that has not been possible using rodents.
Despite numerous advantages of zebrafish for studying sleep, it has several limitations as a sleep model. Mammalian sleep is often defined based on recordings of electrical activity in the brain (electroencephalogram, EEG) and muscle (electromyogram, EMG) that can precisely define sleep and wake states. Although methods for non-invasive EEG recordings in larval (Hong et al., 2016) and adult (Cho et al., 2017) zebrafish have been developed, these methods have only been used to monitor large changes in brain activity that occur during seizures. However, several studies have shown that mammalian sleep can be studied using behavioral measures alone, similar to zebrafish. For example, behavioral assays that measure sleep duration in mice have been developed that have a high correlation with EEG/EMG measures of sleep (Pack et al., 2007; Fisher et al., 2012). Similarly, sleep in humans has been measured using actigraphy, with results similar to those obtained using EEG/EMG (Ancoli-Israel et al., 2003; Van de Water et al., 2011; Yetish et al., 2015). A limitation of this approach is that when sleep is defined using behavioral measures, it can be difficult to distinguish sleep from direct effects on the motor system. However, sleep is rapidly reversible and is associated with an increased arousal threshold, thus distinguishing it from paralysis. Another limitation of zebrafish is that it is more distantly related to humans than rodents, and thus rodents may provide a better model for genetic regulation of human sleep. However, as noted above, most human genes have at least one zebrafish ortholog, and most genes that affect mammalian sleep have similar effects on zebrafish behavior (Barlow and Rihel, 2017; Levitas-Djerbi and Appelbaum, 2017; Oikonomou and Prober, 2017). A final concern is that zebrafish lack some mammalian brain structures, such as a large cerebral cortex, that may play roles in sleep (Krone et al., 2021). However, the zebrafish dorsal telencephalon has similarities to mammalian cortical regions based on molecular markers (Wullimann and Mueller, 2004; Mueller et al., 2011) and functional assays (Aoki et al., 2013; Lal et al., 2018; Torigoe et al., 2021).
To perform a targeted screen for genes whose loss affects sleep, genome-editing techniques such as CRISPR/Cas9 (Hwang et al., 2013; Figure 3A) can be used to generate a large collection of zebrafish lines, each containing a predicted null mutation in the zebrafish ortholog of a candidate human sleep disorder risk gene. Tools such as CHOPCHOP (Labun et al., 2016, 2019) and CRISPRscan (Moreno-Mateos et al., 2015) can be used to identify single guide RNAs (sgRNAs) with high predicted activity and no predicted off-target sites in the genome. Ideally, one should select sgRNAs that target exons between or within domains that are essential for protein function. To generate mutants, an sgRNA and Cas9 mRNA (Hwang et al., 2013) or Cas9 protein (Jao et al., 2013) are microinjected into embryos at the 1-cell stage, and sgRNAs with high cleavage efficiency can be identified using a variety of methods, including T7 endonuclease assay (Hua et al., 2017), high-resolution melting curve analysis (Thomas et al., 2014), or high-throughput sequencing (Gagnon et al., 2014). Once an effective sgRNA is identified, embryos are injected with that sgRNA and Cas9 mRNA or protein and are raised to adulthood. These F0 fish are then outcrossed to wild-type (WT) fish, and their F1 progeny are screened for mutations at the embryonic or larval stage of development using PCR fragment analysis (Varshney et al., 2016) or other methods (Sentmanat et al., 2018). Once F0 fish carrying germline mutations are identified, they are again outcrossed to WT fish to generate F1 animals, which are raised to adulthood and genotyped by PCR to identify heterozygous mutant carriers. The specific nature of each mutation can then be determined by Sanger sequencing. Usually, mutants are selected that contain an insertion/deletion (indel) mutation that is predicted to cause a shift in the translational reading frame, since this changes the protein sequence and often introduces a premature stop codon, thus generating a truncated protein that lacks essential functional domains. Alternatively, the first exon or an entire gene can be deleted by co-injecting two sgRNAs that flank the region to be deleted (Chen et al., 2014), or sequences containing a stop codon can be inserted into the coding sequence of a gene (Gagnon et al., 2014), but these methods are less efficient than generating indel mutations. Ideally, two independent mutant lines should be established for each candidate gene in order to increase confidence that any phenotype is due to mutation of the gene of interest. Mutants should be outcrossed to the parental WT strain for at least two generations before behavioral assays are performed to minimize the presence of any off-target mutations that might affect behavior.
Figure 3. Using CRISPR/Cas9 to generate a predicted null mutation in the zebrafish egfra gene. (A) A schematic diagram of the CRISPR/Cas9 system. The Cas9/sgRNA complex targets a specific sequence in the genome based on a 20 base-pair target sequence in the sgRNA that is complementary to the genomic target next to a protospacer adjacent motif (PAM) and produces a double-stranded DNA break. (B) The coding sequence of a region of exon 6 from WT and mutant zebrafish egfra are shown. The mutant contains an 11 bp deletion (red font) and a 27 bp insertion (blue font) in the egfra gene. Yellow shading indicates the sgRNA target sequence. (C) An alignment of human EGFR (Hs), zebrafish WT EGFRA (Dr), and zebrafish mutant EGFRA (Dr mut) proteins is shown. Blue shading indicates amino acids that are identical in the human and zebrafish orthologs. Gray shading indicates frame shifted sequence in the zebrafish EGFRA mutant. The black line indicates the location of the transmembrane domain in the WT proteins. The mutant protein lacks the transmembrane domain and intracellular domains that are required to interact with downstream effector proteins, and thus should be non-functional. Reproduced and modified from Lee et al. (2019).
In order to reduce the amount of time and effort needed to generate and characterize mutant animals, several studies have proposed performing screens of somatic mutations in F0 injected embryos and larvae (Wu et al., 2018; Hoshijima et al., 2019; Kroll et al., 2021; Quick et al., 2021). This strategy involves injecting Cas9 protein along with multiple sgRNAs that target different regions of the same gene, with scrambled sgRNAs used as negative controls. This approach has been shown to work well when testing for developmental phenotypes such as loss of pigmentation (Kroll et al., 2021), loss of specific neuronal populations (Liu et al., 2015), and eye defects (Hoshijima et al., 2019). In proof-of-concept experiments, the potential utility of this approach to generate behavioral phenotypes was demonstrated by assaying behavioral responses to a chemical irritant and changes in locomotor activity in larval zebrafish (Kroll et al., 2021). Despite the efficiency of this strategy, it is unclear whether it will be useful for identifying sleep mutants for several reasons. First, in F0 injected embryos and larvae, the targeted gene is only knocked out in a subset of cells in an animal, and the number of such cells will vary among injected animals. Since sleep experiments require that behavioral data from many animals be analyzed as a group, and it is difficult to determine whether the targeted gene is knocked out in the relevant cells in each animal, phenotypes in F0 injected animals are likely to be smaller and more variable compared to those in germline mutants, where the targeted gene is mutated in every cell of an animal. This caveat is less of a problem for developmental assays, where mutant phenotypes can be detected in individual animals, including animals that are mosaic for mutant cells, and one can often directly observe mutant and WT cells in an animal (Liu et al., 2015; Kroll et al., 2021). Second, while computational tools are used to select sgRNAs that are predicted to lack off-target sites in the genome (Moreno-Mateos et al., 2015; Labun et al., 2016, 2019), co-injecting multiple sgRNAs nevertheless increases the likelihood of generating off-target mutations (Chen et al., 2014; McCarty et al., 2020) which can affect behavior. Third, the microinjection procedure can cause non-specific developmental defects that affect behavior but are too subtle to detect by visual inspection. This can result in fish that have abnormal behavior, which in some cases can be difficult to distinguish from sleep phenotypes. Indeed, the behavior of microinjected fish can be more variable than that of uninjected controls (Chiu et al., 2016), and robust sleep phenotypes observed in germline mutants may not be apparent in F0 microinjected fish.
A potential problem with using genetic approaches to study sleep is that mutants may have developmental defects that preclude or confound behavioral testing. Indeed, this has been a significant challenge for attempts to use genetics to study mammalian sleep (Thomas et al., 1995; Alenina et al., 2009). It is estimated that 1,400–2,500 zebrafish genes are essential for embryonic development (Driever et al., 1996; Haffter et al., 1996; Amsterdam et al., 2004), and there are likely many additional genes whose loss results in animals that are viable but have developmental defects. Thus, mutants must be carefully screened for developmental defects prior to testing them for sleep phenotypes. Even if a mutant does not show obvious developmental phenotypes, it may have subtle developmental defects or be developmentally delayed, which can result in phenotypes that may be difficult to distinguish from effects on sleep. This risk can be mitigated by testing mutants for muscle and motor defects by light touch with a blunt needle and by tapping the dish containing the animals, both of which should elicit a startle response. Ideally, mutant phenotypes should be confirmed using acute perturbations where effects on development are less of a concern, such as acute treatment with a drug that inhibits the targeted protein or its signaling pathway, or by targeted ablation of cells that express the protein. In addition, showing that an acute gain-of-function perturbation results in a phenotype opposite to that of the mutant increases confidence that a mutant phenotype is due to effects on behavior and not on development. This can be achieved using several approaches, including heat shock-induced overexpression of the gene of interest (Prober et al., 2006), and optogenetic (Singh et al., 2015) or chemogenetic (Chen S. et al., 2016) stimulation of neurons that express the gene of interest.
An important consideration when using loss-of-function mutations in zebrafish is that the teleost lineage underwent genome duplication events after its divergence from the mammalian lineage, and at least 20% of duplicated genes have been retained (Postlethwait et al., 2000). As a result, the zebrafish genome contains two or more paralogs of some human genes, which can have both advantages and disadvantages for genetic studies. One advantage is that some genes that are required for mammalian development or whose loss results in phenotypes that confound sleep analyses can be studied in zebrafish if the two zebrafish paralogs are at least partially redundant. For example, mutation of ATPase Na+/K+ transporting subunit alpha 3 (ATP1A3) in humans is associated with rapid-onset dystonia-Parkinsonism (de Carvalho Aguiar et al., 2004) and alternating hemiplegia of childhood (Heinzen et al., 2014). atp1a3 mutant mice exhibit seizures (Clapcote et al., 2009) and motor deficits (Kirshenbaum et al., 2011) which complicate the study of sleep. Zebrafish have two atp1a3 paralogs (atp1a3a and atp1a3b), and while fish that lack both paralogs apparently die during embryogenesis, loss of either gene alone has no apparent effect on development or gross motor functions. However, atp1a3a, but not atp1a3b, mutant zebrafish show a large reduction in sleep at night (Barlow et al., 2020). Thus, the study of atp1a3 in zebrafish revealed a function in sleep that would be difficult to detect in mammals. Another advantage arises when multiple zebrafish paralogs of a single mammalian gene are expressed in different cell populations, thus allowing the function of each population to be studied separately. For example, zebrafish have three paralogs of mammalian tryptophan hydroxylase (tph), the rate-limiting enzyme in the biosynthesis of serotonin. In zebrafish, tph1a is expressed in the retina, pineal gland, preoptic area, posterior tuberculum, and caudal hypothalamus, whereas tph1b is only transiently expressed in preoptic neurons of the hypothalamus during development (Bellipanni et al., 2002). In contrast, although tph2 is expressed in the pineal gland, similar to tph1a, it is also expressed in serotonergic raphe neurons, which do not express tph1a or tph1b (Teraoka et al., 2004). Mutation of tph2 and manipulation of tph2-expressing neurons revealed that serotonergic raphe neurons promote sleep, thus resolving a longstanding controversy in the field (Oikonomou et al., 2019).
A disadvantage of having multiple zebrafish paralogs of single mammalian genes is that if the paralogs are redundant, it is necessary to knock out both genes in order to observe a phenotype. For example, human gpr103, which acts as a receptor for the neuropeptide QRFP (Takayasu et al., 2006), has two paralogs in zebrafish (gpr103a and gpr103b; Chen A. et al., 2016). While mutation of either paralog has no effect on sleep in zebrafish, double mutants sleep less during the day compared to their WT siblings (Chen A. et al., 2016). Another disadvantage arises when a mutation causes nonsense-mediated decay (NMD) of the mutated gene’s mRNA, which often occurs for indel mutations that are generated using CRISPR/Cas9. NMD can trigger a compensatory response in which the expression of paralogs and other related genes are upregulated, thus potentially masking a mutant phenotype (Rossi et al., 2015; Anderson et al., 2017; El-Brolosy et al., 2019). The presence of NMD can be determined by performing RT-qPCR or in situ hybridization using a probe specific for the targeted gene and comparing its expression level in homozygous mutant animals to their WT siblings. If NMD is observed, and particularly if there is no mutant phenotype, other mutant alleles can be generated by using sgRNAs that target other regions of the gene, which may create mutations that do not result in the induction of NMD. Alternatively, NMD can be avoided by deleting the gene of interest from the genome by using a pair of sgRNAs that flank the coding sequence (El-Brolosy et al., 2019). However, this strategy will remove introns that might contain sequences that affect the expression of other genes, and thus deletion of only the first exon may be preferable. Ideally, loss of the targeted protein should be evaluated using an antibody that is specific for the mutated gene, although antibodies are not available for most zebrafish proteins.
To test zebrafish mutants for sleep defects, adult heterozygous mutant animals are in-crossed to generate homozygous mutant, heterozygous mutant, and WT sibling larvae. Individual 4-dpf fish are placed in each well of a 96-well plate filled with E3 medium and maintained at 28.5°C. A videotracking system (Figure 2A) is used to monitor the behavior of each animal for up to 72 hours, during which animals are exposed to white light from 9 a.m. to 11 p.m., while infrared light and an infrared camera are used to record behavior. Each animal is then genotyped by PCR to identify homozygous mutant, heterozygous mutant, and homozygous WT siblings. MATLAB scripts are used to quantify the duration of locomotor activity and sleep, the length and number of wake and sleep bouts, the latency to first sleep bout at night, and the amount of locomotor activity while awake. This method has been used for zebrafish behavioral screens (Rihel et al., 2010; Chiu et al., 2016; Thyme et al., 2019) and to characterize sleep phenotypes in mutant zebrafish (Gandhi et al., 2015; Singh et al., 2015, 2017; Yelin-Bekerman et al., 2015; Chen A. et al., 2016, Chen A. et al., 2017, Chen S. et al., 2017; Chiu et al., 2016; Hoffman et al., 2016; Lee et al., 2017, 2019; Ashlin et al., 2018; Leung et al., 2019; Oikonomou et al., 2019; Reichert et al., 2019; Levitas-Djerbi et al., 2020; Özcan et al., 2020; Kroll et al., 2021). This behavioral assay has also been modified to measure habituation to acoustic stimuli, prepulse inhibition, and behavioral responses to light, darkness, heat, and noxious chemicals (Prober et al., 2008; Chen S. et al., 2017; Thyme et al., 2019).
In a recent proof-of-concept study, GWAS analyses implicated the epidermal growth factor receptor (EGFR) signaling pathway in regulating sleep, a finding that was then validated using zebrafish (Lee et al., 2019). A human GWAS sleep trait analysis identified genome-wide significant signals near ERBB4 (Lee et al., 2019) and KSR2 (Figure 1B), which are known to participate in the EGFR signaling pathway (Cohen et al., 1996; Dhawan et al., 2016). Consistent with this observation, a separate GWAS analysis found that associations at the EGFR signaling pathway are enriched for daytime sleepiness in humans (Wang et al., 2019). To validate a role for EGFR signaling in sleep, zebrafish containing loss-of-function mutations in the zebrafish orthologs of epidermal growth factor receptor a (egfra; Figures 3B,C), or the EGFR ligands transforming growth factor alpha (tgfa) and epidermal growth factor (egf), resulted in decreased sleep, as did acute pharmacological inhibition of EGFR (Figure 2B), ERBB4, or KSR2 (Lee et al., 2019). Conversely, overexpression of tgfa resulted in increased sleep in an egfra-dependent manner. Arousal threshold and sleep deprivation assays revealed that EGFR signaling regulates both arousal threshold (Figure 2D) and sleep homeostasis (Lee et al., 2019). These results indicate that EGFR signaling functions to promote sleep in zebrafish, consistent with previous studies showing that EGFR signaling promotes sleep in Drosophila (Foltenyi et al., 2007; Donlea et al., 2009; Harbison et al., 2013, 2017) and C. elegans (Van Buskirk and Sternberg, 2007; Hill et al., 2014; Nelson et al., 2014; Nath et al., 2016). Thus, EGFR signaling has an evolutionarily conserved function to promote sleep.
Once a role for EGFR signaling in zebrafish sleep was established, further work integrated this finding into a sleep-regulating mechanism that had been discovered by animal model studies. A previous study found that the hypothalamic neuropeptide VF (NPVF) promotes sleep in zebrafish (Lee et al., 2017), similar to EGFR signaling. When a potential link between these pathways was explored, it was found that EGFR signaling promotes both the expression of npvf and the activity of npvf-expressing neurons, and genetic epistasis experiments indicated that NPVF acts downstream of EGFR to promote sleep (Lee et al., 2019). Another study showed that NPVF signaling promotes sleep via the serotonergic raphe nuclei in zebrafish (Lee et al., 2020), which have a sleep-promoting function in both zebrafish and mice (Oikonomou et al., 2019). A sleep-promoting function for the serotonergic raphe in zebrafish and mice is consistent with human GWAS, which found that variants in genes participating in serotonergic signaling are enriched for association with human sleep traits (Lane et al., 2017, 2019; Dashti et al., 2019; Jones et al., 2019). These studies illustrate how human GWAS and animal model studies can be used to identify genes that underlie human sleep disorders and to determine the genetic and neuronal mechanisms through which these genes affect sleep.
Since the first GWAS was published 20 years ago (Ozaki et al., 2002), hundreds of thousands of genomic risk loci associated with thousands of human traits and diseases have been identified (MacArthur et al., 2017; Loos, 2020). This work has led to the development of comprehensive GWAS databases containing information on identified disease/trait-associated variants and nearby genes (Buniello et al., 2019; Wang et al., 2020). However, a bottleneck currently exists in the development of tools and animal models to identify the causative gene that is associated with each genomic risk locus. The use of rodent models is limited by the challenges of generating, maintaining, and testing large numbers of mutant lines, while zebrafish provide a useful alternative vertebrate model to validate human candidate disease risk genes efficiently and cost-effectively, and to then perform mechanistic studies to understand the involvement of each gene in disease. Indeed, zebrafish have been used to validate human candidate genes associated with insomnia (Palermo et al., 2021), schizophrenia (Thyme et al., 2019), diabetes (Adeyemo et al., 2019), abnormal liver function (Liu et al., 2013), idiopathic scoliosis (Mathieu et al., 2021), attention deficit hyperactivity disorder (ADHD; Dark et al., 2020), and other psychiatric disorders (Tang et al., 2020). The strategies described in this review are not limited to GWAS, but can also be applied to other disease gene discovery strategies. For example, whole-exome and whole-genome sequencing of individuals with neuropsychiatric disorders are increasingly being used to identify candidate disease risk genes (Sanders et al., 2017), but similar to GWAS it is often unclear whether any specific candidate gene is causative for disease. This is particularly the case for non-coding variants, which represent over 90% of disease-associated genomic risk loci identified through GWAS (Sanders et al., 2017). Thus, similar to GWAS, candidate disease risk genes identified using sequencing often require validation studies in an animal model. Zebrafish models have already been useful for this purpose, for example validating nr3c2 as an autism spectrum disorder risk gene based on human whole-genome sequencing and zebrafish functional assays (Ruzzo et al., 2019). Thus, the use of zebrafish models has the potential to accelerate the identification of disease risk genes, aid in the acquisition of mechanistic insights, and inform the development of new therapies.
ST and DAP both contributed to the writing of this manuscript. All authors contributed to the article and approved the submitted version.
This work was funded by NIH grants R35NS122172 and R01MH121601 to DAP and a SRSF Career Development Award, Banting Postdoctoral Fellowship, and NSERC Postdoctoral Fellowship awarded to ST.
The authors declare that the research was conducted in the absence of any commercial or financial relationships that could be construed as a potential conflict of interest.
All claims expressed in this article are solely those of the authors and do not necessarily represent those of their affiliated organizations, or those of the publisher, the editors and the reviewers. Any product that may be evaluated in this article, or claim that may be made by its manufacturer, is not guaranteed or endorsed by the publisher.
Adamantidis, A. R., Zhang, F., Aravanis, A. M., Deisseroth, K., and de Lecea, L. (2007). Neural substrates of awakening probed with optogenetic control of hypocretin neurons. Nature 450, 420–424. doi: 10.1038/nature06310
Adeyemo, A. A., Zaghloul, N. A., Chen, G., Doumatey, A. P., Leitch, C. C., Hostelley, T. L., et al. (2019). ZRANB3 is an African-specific type 2 diabetes locus associated with beta-cell mass and insulin response. Nat. Commun. 10:3195. doi: 10.1038/s41467-019-10967-7
Ahrens, M. B., Huang, K. H., Narayan, S., Mensh, B. D., and Engert, F. (2013). Two-photon calcium imaging during fictive navigation in virtual environments. Front. Neural Circuits 7:104. doi: 10.3389/fncir.2013.00104
Alenina, N., Kikic, D., Todiras, M., Mosienko, V., Qadri, F., Plehm, R., et al. (2009). Growth retardation and altered autonomic control in mice lacking brain serotonin. Proc. Natl. Acad. Sci. U S A 106, 10332–10337. doi: 10.1073/pnas.0810793106
Allada, R., Cirelli, C., and Sehgal, A. (2017). Molecular mechanisms of sleep homeostasis in flies and mammals. Cold Spring Harb. Perspect. Biol. 9:a027730. doi: 10.1101/cshperspect.a027730
Amsterdam, A., Nissen, R. M., Sun, Z., Swindell, E. C., Farrington, S., and Hopkins, N. (2004). Identification of 315 genes essential for early zebrafish development. Proc. Natl. Acad. Sci. U S A 101, 12792–12797. doi: 10.1073/pnas.0403929101
Ancoli-Israel, S., Cole, R., Alessi, C., Chambers, M., Moorcroft, W., and Pollak, C. P. (2003). The role of actigraphy in the study of sleep and circadian rhythms. Sleep 26, 342–392. doi: 10.1093/sleep/26.3.342
Anderson, J. L., Mulligan, T. S., Shen, M.-C., Wang, H., Scahill, C. M., Tan, F. J., et al. (2017). mRNA processing in mutant zebrafish lines generated by chemical and CRISPR-mediated mutagenesis produces unexpected transcripts that escape nonsense-mediated decay. PLoS Genet. 13:e1007105. doi: 10.1371/journal.pgen.1007105
Aoki, T., Kinoshita, M., Aoki, R., Agetsuma, M., Aizawa, H., Yamazaki, M., et al. (2013). Imaging of neural ensemble for the retrieval of a learned behavioral program. Neuron 78, 881–894. doi: 10.1016/j.neuron.2013.04.009
Artiushin, G., and Sehgal, A. (2017). The Drosophila circuitry of sleep-wake regulation. Curr. Opin. Neurobiol. 44, 243–250. doi: 10.1016/j.conb.2017.03.004
Ashlin, T. G., Blunsom, N. J., Ghosh, M., Cockcroft, S., and Rihel, J. (2018). Pitpnc1a regulates zebrafish sleep and wake behavior through modulation of insulin-like growth factor signaling. Cell Rep. 24, 1389–1396. doi: 10.1016/j.celrep.2018.07.012
Barlow, I. L., Mackay, E., Wheater, E., Goel, A., Lim, S., Zimmerman, S., et al. (2020). A genetic screen identifies dreammist as a regulator of sleep. bioRxiv [Preprint]. doi: 10.1101/2020.11.18.388736
Barlow, I. L., and Rihel, J. (2017). Zebrafish sleep: from geneZZZ to neuronZZZ. Curr. Opin. Neurobiol. 44, 65–71. doi: 10.1016/j.conb.2017.02.009
Bellipanni, G., Rink, E., and Bally-Cuif, L. (2002). Cloning of two tryptophan hydroxylase genes expressed in the diencephalon of the developing zebrafish brain. Mech. Dev. 119, S215–S220. doi: 10.1016/s0925-4773(03)00119-9
Buniello, A., MacArthur, J. A. L., Cerezo, M., Harris, L. W., Hayhurst, J., Malangone, C., et al. (2019). The NHGRI-EBI GWAS Catalog of published genome-wide association studies, targeted arrays and summary statistics 2019. Nucleic Acids Res. 47, D1005–D1012. doi: 10.1093/nar/gky1120
Burgess, H. A., and Granato, M. (2007). Sensorimotor gating in larval zebrafish. J. Neurosci. 27, 4984–4994. doi: 10.1523/JNEUROSCI.0615-07.2007
Bycroft, C., Freeman, C., Petkova, D., Band, G., Elliott, L. T., Sharp, K., et al. (2018). The UK Biobank resource with deep phenotyping and genomic data. Nature 562, 203–209. doi: 10.1038/s41586-018-0579-z
Chemelli, R. M., Willie, J. T., Sinton, C. M., Elmquist, J. K., Scammell, T., Lee, C., et al. (1999). Narcolepsy in orexin knockout mice: molecular genetics of sleep regulation. Cell 98, 437–451. doi: 10.1016/s0092-8674(00)81973-x
Chen, S., Chiu, C. N., McArthur, K. L., Fetcho, J. R., and Prober, D. A. (2016). TRP channel mediated neuronal activation and ablation in freely behaving zebrafish. Nat. Methods 13, 147–150. doi: 10.1038/nmeth.3691
Chen, A., Chiu, C. N., Mosser, E. A., Kahn, S., Spence, R., and Prober, D. A. (2016). QRFP and its receptors regulate locomotor activity and sleep in zebrafish. J. Neurosci. 36, 1823–1840. doi: 10.1523/JNEUROSCI.2579-15.2016
Chen, S., Reichert, S., Singh, C., Oikonomou, G., Rihel, J., and Prober, D. A. (2017). Light-dependent regulation of sleep and wake states by prokineticin 2 in zebrafish. Neuron 95, 153–168.e6. doi: 10.1016/j.neuron.2017.06.001
Chen, A., Singh, C., Oikonomou, G., and Prober, D. A. (2017). Genetic analysis of histamine signaling in larval zebrafish sleep. eNeuro 4: ENEURO.0286-16.2017. doi: 10.1523/JNEUROSCI.2579-15.2016
Chen, X., Xu, F., Zhu, C., Ji, J., Zhou, X., Feng, X., et al. (2014). Dual sgRNA-directed gene knockout using CRISPR/Cas9 technology in Caenorhabditis elegans. Sci. Rep. 4:7581. doi: 10.1038/srep07581
Chesi, A., Wagley, Y., Johnson, M. E., Manduchi, E., Su, C., Lu, S., et al. (2019). Genome-scale capture C promoter interactions implicate effector genes at GWAS loci for bone mineral density. Nat. Commun. 10:1260. doi: 10.1038/s41467-019-09302-x
Chiu, C. N., Rihel, J., Lee, D. A., Singh, C., Mosser, E. A., Chen, S., et al. (2016). A zebrafish genetic screen identifies neuromedin U as a regulator of sleep/wake states. Neuron 89, 842–856. doi: 10.1016/j.neuron.2016.01.007
Cho, S.-J., Byun, D., Nam, T.-S., Choi, S.-Y., Lee, B.-G., Kim, M.-K., et al. (2017). Zebrafish as an animal model in epilepsy studies with multichannel EEG recordings. Sci. Rep. 7:3099. doi: 10.1038/s41598-017-03482-6
Cirelli, C., Bushey, D., Hill, S., Huber, R., Kreber, R., Ganetzky, B., et al. (2005). Reduced sleep in Drosophila Shaker mutants. Nature 434, 1087–1092. doi: 10.1038/nature03486
Clapcote, S. J., Duffy, S., Xie, G., Kirshenbaum, G., Bechard, A. R., Rodacker Schack, V., et al. (2009). Mutation I810N in the alpha3 isoform of Na+,K+-ATPase causes impairments in the sodium pump and hyperexcitability in the CNS. Proc. Natl. Acad. Sci. U S A 106, 14085–14090. doi: 10.1073/pnas.0904817106
Claussnitzer, M., Dankel, S. N., Kim, K.-H., Quon, G., Meuleman, W., Haugen, C., et al. (2015). FTO obesity variant circuitry and adipocyte browning in humans. N. Engl. J. Med. 373, 895–907. doi: 10.1056/NEJMoa1502214
Cohen, B. D., Green, J. M., Foy, L., and Fell, H. P. (1996). HER4-mediated biological and biochemical properties in NIH 3T3 cells evidence for HER1-HER4 heterodimers. J. Biol. Chem. 271, 4813–4818. doi: 10.1074/jbc.271.9.4813
Colten, H. R., and Altevogt, B. M. (2006). Sleep Disorders and Sleep Deprivation: An Unmet Public Health Problem. Washington, DC: National Academies Press (US). doi: 10.17226/11617
Cox, R. C., and Olatunji, B. O. (2016). A systematic review of sleep disturbance in anxiety and related disorders. J. Anxiety Disord. 37, 104–129. doi: 10.1016/j.janxdis.2015.12.001
Dark, C., Williams, C., Bellgrove, M. A., Hawi, Z., and Bryson-Richardson, R. J. (2020). Functional validation of CHMP7 as an ADHD risk gene. Transl. Psychiatry 10:385. doi: 10.1038/s41398-020-01077-w
Dashti, H. S., Daghlas, I., Lane, J. M., Huang, Y., Udler, M. S., Wang, H., et al. (2021). Genetic determinants of daytime napping and effects on cardiometabolic health. Nat. Commun. 12:900. doi: 10.1038/s41467-020-20585-3
Dashti, H. S., Jones, S. E., Wood, A. R., Lane, J. M., van Hees, V. T., Wang, H., et al. (2019). Genome-wide association study identifies genetic loci for self-reported habitual sleep duration supported by accelerometer-derived estimates. Nat. Commun. 10:1100. doi: 10.1038/s41467-019-08917-4
de Carvalho Aguiar, P., Sweadner, K. J., Penniston, J. T., Zaremba, J., Liu, L., Caton, M., et al. (2004). Mutations in the Na+/K+-ATPase α3 gene ATP1A3 are associated with rapid-onset dystonia parkinsonism. Neuron 43, 169–175. doi: 10.1016/j.neuron.2004.06.028
de Lecea, L. (2021). Twenty-three years of hypocretins: the “rosetta stone” of sleep/arousal circuits. Front. Neurol. Neurosci. 45, 1–10. doi: 10.1159/000514961
Del Bene, F., and Wyart, C. (2012). Optogenetics: a new enlightenment age for zebrafish neurobiology. Dev. Neurobiol. 72, 404–414. doi: 10.1002/dneu.20914
Dhawan, N. S., Scopton, A. P., and Dar, A. C. (2016). Small molecule stabilization of the KSR inactive state antagonizes oncogenic ras signalling. Nature 537, 112–116. doi: 10.1038/nature19327
Dietzl, G., Chen, D., Schnorrer, F., Su, K.-C., Barinova, Y., Fellner, M., et al. (2007). A genome-wide transgenic RNAi library for conditional gene inactivation in Drosophila. Nature 448, 151–156. doi: 10.1038/nature05954
Doherty, A., Smith-Byrne, K., Ferreira, T., Holmes, M. V., Holmes, C., Pulit, S. L., et al. (2018). GWAS identifies 14 loci for device-measured physical activity and sleep duration. Nat. Commun. 9:5257. doi: 10.1038/s41467-018-07743-4
Donlea, J. M., Ramanan, N., and Shaw, P. J. (2009). Use-dependent plasticity in clock neurons regulates sleep need in Drosophila. Science 324, 105–108. doi: 10.1126/science.1166657
Driever, W., Solnica-Krezel, L., Schier, A. F., Neuhauss, S. C., Malicki, J., Stemple, D. L., et al. (1996). A genetic screen for mutations affecting embryogenesis in zebrafish. Dev. 123, 37–46. doi: 10.1242/dev.123.1.37
Elbaz, I., Yelin-Bekerman, L., Nicenboim, J., Vatine, G., and Appelbaum, L. (2012). Genetic ablation of hypocretin neurons alters behavioral state transitions in zebrafish. J. Neurosci. 32, 12961–12972. doi: 10.1523/JNEUROSCI.1284-12.2012
El-Brolosy, M. A., Kontarakis, Z., Rossi, A., Kuenne, C., Gunther, S., Fukuda, N., et al. (2019). Genetic compensation triggered by mutant mRNA degradation. Nature 568, 193–197. doi: 10.1038/s41586-019-1064-z
Eriksson, N., Macpherson, J. M., Tung, J. Y., Hon, L. S., Naughton, B., Saxonov, S., et al. (2010). Web-based, participant-driven studies yield novel genetic associations for common traits. PLoS Genet. 6:e1000993. doi: 10.1371/journal.pgen.1000993
Fernandez-Mendoza, J., and Vgontzas, A. N. (2013). Insomnia and its impact on physical and mental health. Curr. Psychiatry Rep. 15:418. doi: 10.1007/s11920-013-0418-8
Ferreira, J. G. P., Bittencourt, J. C., and Adamantidis, A. (2017). Melanin-concentrating hormone and sleep. Curr. Opin. Neurobiol. 44, 152–158. doi: 10.1016/j.conb.2017.04.008
Fisher, S. P., Godinho, S. I. H., Pothecary, C. A., Hankins, M. W., Foster, R. G., and Peirson, S. N. (2012). Rapid assessment of sleep-wake behavior in mice. J. Biol. Rhythms 27, 48–58. doi: 10.1177/0748730411431550
Foltenyi, K., Greenspan, R. J., and Newport, J. W. (2007). Activation of EGFR and ERK by rhomboid signaling regulates the consolidation and maintenance of sleep in Drosophila. Nat. Neurosci. 10, 1160–1167. doi: 10.1038/nn1957
Frayling, T. M., Timpson, N. J., Weedon, M. N., Zeggini, E., Freathy, R. M., Lindgren, C. M., et al. (2007). A common variant in the FTO gene is associated with body mass index and predisposes to childhood and adult obesity. Science 316, 889–894. doi: 10.1126/science.1141634
Gagnon, J. A., Valen, E., Thyme, S. B., Huang, P., Akhmetova, L., Pauli, A., et al. (2014). Efficient mutagenesis by Cas9 protein-mediated oligonucleotide insertion and large-scale assessment of single-guide RNAs. PLoS One 9:e98186. doi: 10.1371/journal.pone.0098186
Gandhi, A. V., Mosser, E. A., Oikonomou, G., and Prober, D. A. (2015). Melatonin is required for the circadian regulation of sleep. Neuron 85, 1193–1199. doi: 10.1016/j.neuron.2015.02.016
Garfield, V. (2021). Sleep duration: a review of genome-wide association studies (GWAS) in adults from 2007 to 2020. Sleep Med. Rev. 56:101413. doi: 10.1016/j.smrv.2020.101413
Gentry, N. W., Ashbrook, L. H., Fu, Y.-H., and Ptáček, L. J. (2021). Human circadian variations. J. Clin. Invest. 131:e148282. doi: 10.1172/JCI148282
Goto, M., Oshima, I., Tomita, T., and Ebihara, S. (1989). Melatonin content of the pineal gland in different mouse strains. J. Pineal Res. 7, 195–204. doi: 10.1111/j.1600-079x.1989.tb00667.x
Gottlieb, D. J., Hek, K., Chen, T.-H., Watson, N. F., Eiriksdottir, G., Byrne, E. M., et al. (2015). Novel loci associated with usual sleep duration: the CHARGE consortium genome-wide association Study. Mol. Psychiatry 20, 1232–1239. doi: 10.1038/mp.2014.133
Grove, J., Ripke, S., Als, T. D., Mattheisen, M., Walters, R. K., Won, H., et al. (2019). Identification of common genetic risk variants for autism spectrum disorder. Nat. Genet. 51, 431–444. doi: 10.1038/s41588-019-0344-8
Guo, F., Yu, J., Jung, H. J., Abruzzi, K. C., Luo, W., Griffith, L. C., et al. (2016). Circadian neuron feedback controls the Drosophila sleep–activity profile. Nature 536, 292–297. doi: 10.1038/nature19097
Haffter, P., Granato, M., Brand, M., Mullins, M. C., Hammerschmidt, M., Kane, D. A., et al. (1996). The identification of genes with unique and essential functions in the development of the zebrafish, Danio rerio. Dev. 123, 1–36. doi: 10.1242/dev.123.1.1
Hammerschlag, A. R., Stringer, S., de Leeuw, C. A., Sniekers, S., Taskesen, E., Watanabe, K., et al. (2017). Genome-wide association analysis of insomnia complaints identifies risk genes and genetic overlap with psychiatric and metabolic traits. Nat. Genet. 49, 1584–1592. doi: 10.1038/ng.3888
Harbison, S. T., McCoy, L. J., and Mackay, T. F. C. (2013). Genome-wide association study of sleep in Drosophila melanogaster. BMC Genomics 14:281. doi: 10.1186/1471-2164-14-281
Harbison, S. T., Serrano Negron, Y. L., Hansen, N. F., and Lobell, A. S. (2017). Selection for long and short sleep duration in Drosophila melanogaster reveals the complex genetic network underlying natural variation in sleep. PLoS Genet. 13:e1007098. doi: 10.1371/journal.pgen.1007098
Heinzen, E. L., Arzimanoglou, A., Brashear, A., Clapcote, S. J., Gurrieri, F., Goldstein, D. B., et al. (2014). Distinct neurological disorders with ATP1A3 mutations. Lancet Neurol. 13, 503–514. doi: 10.1016/S1474-4422(14)70011-0
Hendricks, J. C., Finn, S. M., Panckeri, K. A., Chavkin, J., Williams, J. A., Sehgal, A., et al. (2000). Rest in Drosophila is a sleep-like state. Neuron 25, 129–138. doi: 10.1016/s0896-6273(00)80877-6
Hill, A. J., Mansfield, R., Lopez, J. M. N. G., Raizen, D. M., and Van Buskirk, C. (2014). Cellular stress induces a protective sleep-like state in C. elegans. Curr. Biol. 24, 2399–2405. doi: 10.1016/j.cub.2014.08.040
Hoffman, E. J., Turner, K. J., Fernandez, J. M., Cifuentes, D., Ghosh, M., Ijaz, S., et al. (2016). Estrogens suppress a behavioral phenotype in zebrafish mutants of the autism risk gene, CNTNAP2. Neuron 89, 725–733. doi: 10.1016/j.neuron.2015.12.039
Hong, S., Lee, P., Baraban, S. C., and Lee, L. P. (2016). A novel long-term, multi-channel and non-invasive electrophysiology platform for zebrafish. Sci. Rep. 6:28248. doi: 10.1038/srep28248
Hoshijima, K., Jurynec, M. J., Klatt Shaw, D., Jacobi, A. M., Behlke, M. A., and Grunwald, D. J. (2019). Highly efficient CRISPR-Cas9-based methods for generating deletion mutations and F0 embryos that lack gene function in zebrafish. Dev. Cell 51, 645–657.e4. doi: 10.1016/j.devcel.2019.10.004
Howe, K., Clark, M. D., Torroja, C. F., Torrance, J., Berthelot, C., Muffato, M., et al. (2013). The zebrafish reference genome sequence and its relationship to the human genome. Nature 496, 498–503. doi: 10.1038/nature12111
Hu, Y., Shmygelska, A., Tran, D., Eriksson, N., Tung, J. Y., and Hinds, D. A. (2016). GWAS of 89,283 individuals identifies genetic variants associated with self-reporting of being a morning person. Nat. Commun. 7:10448. doi: 10.1038/ncomms10448
Hua, Y., Wang, C., Huang, J., and Wang, K. (2017). A simple and efficient method for CRISPR/Cas9-induced mutant screening. J. Genet. Genomics 44, 207–213. doi: 10.1016/j.jgg.2017.03.005
Hurd, M. W., Debruyne, J., Straume, M., and Cahill, G. M. (1998). Circadian rhythms of locomotor activity in zebrafish. Physiol. Behav. 65, 465–472. doi: 10.1016/s0031-9384(98)00183-8
Hwang, W. Y., Fu, Y., Reyon, D., Maeder, M. L., Tsai, S. Q., Sander, J. D., et al. (2013). Efficient genome editing in zebrafish using a CRISPR-Cas system. Nat. Biotechnol. 31, 227–229. doi: 10.1038/nbt.2501
Jansen, P. R., Watanabe, K., Stringer, S., Skene, N., Bryois, J., Hammerschlag, A. R., et al. (2019). Genome-wide analysis of insomnia in 1,331,010 individuals identifies new risk loci and functional pathways. Nat. Genet. 51, 394–403. doi: 10.1038/s41588-018-0333-3
Jao, L.-E., Wente, S. R., and Chen, W. (2013). Efficient multiplex biallelic zebrafish genome editing using a CRISPR nuclease system. Proc. Natl. Acad. Sci. U S A 110, 13904–13909. doi: 10.1073/pnas.1308335110
Joiner, W. J. (2016). Unraveling the evolutionary determinants of sleep. Curr. Biol. 26, R1073–R1087. doi: 10.1016/j.cub.2016.08.068
Jones, S. E., Tyrrell, J., Wood, A. R., Beaumont, R. N., Ruth, K. S., Tuke, M. A., et al. (2016). Genome-wide association analyses in 128,266 individuals identifies new morningness and sleep duration loci. PLoS Genet. 12:e1006125. doi: 10.1371/journal.pgen.1006125
Jones, S. E., van Hees, V. T., Mazzotti, D. R., Marques-Vidal, P., Sabia, S., van der Spek, A., et al. (2019). Genetic studies of accelerometer-based sleep measures yield new insights into human sleep behaviour. Nat. Commun. 10:1585. doi: 10.1038/s41467-019-09576-1
Kawakami, K., Shima, A., and Kawakami, N. (2000). Identification of a functional transposase of the Tol2 element, an Ac-like element from the Japanese medaka fish and its transposition in the zebrafish germ lineage. Proc. Natl. Acad. Sci. U S A 97, 11403–11408. doi: 10.1073/pnas.97.21.11403
Kirshenbaum, G. S., Clapcote, S. J., Duffy, S., Burgess, C. R., Petersen, J., Jarowek, K. J., et al. (2011). Mania-like behavior induced by genetic dysfunction of the neuron-specific Na+,K+-ATPase α3 sodium pump. Proc. Natl. Acad. Sci. U S A 108, 18144–18149. doi: 10.1073/pnas.1108416108
Koh, K., Joiner, W. J., Wu, M. N., Yue, Z., Smith, C. J., and Sehgal, A. (2008). Identification of SLEEPLESS, a sleep-promoting factor. Science 321, 372–376. doi: 10.1126/science.1155942
Kroll, F., Powell, G. T., Ghosh, M., Gestri, G., Antinucci, P., Hearn, T. J., et al. (2021). A simple and effective F0 knockout method for rapid screening of behaviour and other complex phenotypes. eLife 10:e59683. doi: 10.7554/eLife.59683
Krone, L. B., Yamagata, T., Blanco-Duque, C., Guillaumin, M. C. C., Kahn, M. C., van der Vinne, V., et al. (2021). A role for the cortex in sleep-wake regulation. Nat. Neurosci. 24, 1210–1215. doi: 10.1038/s41593-021-00894-6
Krystal, A. D. (2012). Psychiatric disorders and sleep. Neurol. Clin. 30, 1389–1413. doi: 10.1016/j.ncl.2012.08.018
Labun, K., Montague, T. G., Gagnon, J. A., Thyme, S. B., and Valen, E. (2016). CHOPCHOP v2: a web tool for the next generation of CRISPR genome engineering. Nucleic Acids Res. 44, W272–276. doi: 10.1093/nar/gkw398
Labun, K., Montague, T. G., Krause, M., Torres Cleuren, Y. N., Tjeldnes, H., and Valen, E. (2019). CHOPCHOP v3: expanding the CRISPR web toolbox beyond genome editing. Nucleic Acids Res. 47, W171–W174. doi: 10.1093/nar/gkz365
Lal, P., Tanabe, H., Suster, M. L., Ailani, D., Kotani, Y., Muto, A., et al. (2018). Identification of a neuronal population in the telencephalon essential for fear conditioning in zebrafish. BMC Biol. 16:45. doi: 10.1186/s12915-018-0502-y
Lane, J. M., Jones, S. E., Dashti, H. S., Wood, A. R., Aragam, K. G., van Hees, V. T., et al. (2019). Biological and clinical insights from genetics of insomnia symptoms. Nat. Genet. 51, 387–393. doi: 10.1038/s41588-019-0361-7
Lane, J. M., Liang, J., Vlasac, I., Anderson, S. G., Bechtold, D. A., Bowden, J., et al. (2017). Genome-wide association analyses of sleep disturbance traits identify new loci and highlight shared genetics with neuropsychiatric and metabolic traits. Nat. Genet. 49, 274–281. doi: 10.1038/ng.3749
Lane, J. M., Vlasac, I., Anderson, S. G., Kyle, S. D., Dixon, W. G., Bechtold, D. A., et al. (2016). Genome-wide association analysis identifies novel loci for chronotype in 100,420 individuals from the UK Biobank. Nat. Commun. 7:10889. doi: 10.1038/ncomms10889
Lauderdale, D. S., Knutson, K. L., Yan, L. L., Liu, K., and Rathouz, P. J. (2008). Self-reported and measured sleep duration: how similar are they? Epidemiology 19, 838–845. doi: 10.1097/EDE.0b013e318187a7b0
Lee, D. A., Andreev, A., Truong, T. V., Chen, A., Hill, A. J., Oikonomou, G., et al. (2017). Genetic and neuronal regulation of sleep by neuropeptide VF. eLife 6:e25727. doi: 10.7554/eLife.25727
Lee, D. A., Liu, J., Hong, Y., Lane, J. M., Hill, A. J., Hou, S. L., et al. (2019). Evolutionarily conserved regulation of sleep by epidermal growth factor receptor signaling. Sci. Adv. 5:eaax4249. doi: 10.1126/sciadv.aax4249
Lee, D. A., Oikonomou, G., Cammidge, T., Andreev, A., Hong, Y., Hurley, H., et al. (2020). Neuropeptide VF neurons promote sleep via the serotonergic raphe. eLife 9:e54491. doi: 10.7554/eLife.54491
Leung, L. C., Wang, G. X., Madelaine, R., Skariah, G., Kawakami, K., Deisseroth, K., et al. (2019). Neural signatures of sleep in zebrafish. Nature 571, 198–204. doi: 10.1038/s41586-019-1336-7
Levey, D. F., Stein, M. B., Wendt, F. R., Pathak, G. A., Zhou, H., Aslan, M., et al. (2021). Bi-ancestral depression GWAS in the million veteran program and meta-analysis in >1.2 million individuals highlight new therapeutic directions. Nat. Neurosci. 24, 954–963. doi: 10.1038/s41593-021-00860-2
Levitas-Djerbi, T., and Appelbaum, L. (2017). Modeling sleep and neuropsychiatric disorders in zebrafish. Curr. Opin. Neurobiol. 44, 89–93. doi: 10.1016/j.conb.2017.02.017
Levitas-Djerbi, T., Sagi, D., Lebenthal-Loinger, I., Lerer-Goldshtein, T., and Appelbaum, L. (2020). Neurotensin enhances locomotor activity and arousal and inhibits melanin-concentrating hormone signaling. Neuroendocrinology 110, 35–49. doi: 10.1159/000500590
Lichtneckert, R., and Reichert, H. (2005). Insights into the urbilaterian brain: conserved genetic patterning mechanisms in insect and vertebrate brain development. Heredity (Edinb) 94, 465–477. doi: 10.1038/sj.hdy.6800664
Lin, L., Faraco, J., Li, R., Kadotani, H., Rogers, W., Lin, X., et al. (1999). The sleep disorder canine narcolepsy is caused by a mutation in the hypocretin (orexin) receptor 2 gene. Cell 98, 365–376. doi: 10.1016/s0092-8674(00)81965-0
Liu, D., and Dan, Y. (2019). A motor theory of sleep-wake control: arousal-action circuit. Annu. Rev. Neurosci. 42, 27–46. doi: 10.1146/annurev-neuro-080317-061813
Liu, L. Y., Fox, C. S., North, T. E., and Goessling, W. (2013). Functional validation of GWAS gene candidates for abnormal liver function during zebrafish liver development. Dis. Model. Mech. 6, 1271–1278. doi: 10.1242/dmm.011726
Liu, J., Merkle, F. T., Gandhi, A. V., Gagnon, J. A., Woods, I. G., Chiu, C. N., et al. (2015). Evolutionarily conserved regulation of hypocretin neuron specification by Lhx9. Development 142, 1113–1124. doi: 10.1242/dev.117424
Loos, R. J. F. (2020). 15 years of genome-wide association studies and no signs of slowing down. Nat. Commun. 11:5900. doi: 10.1038/s41467-020-19653-5
Luyster, F. S., Strollo, P. J. J., Zee, P. C., and Walsh, J. K. (2012). Sleep: a health imperative. Sleep 35, 727–734. doi: 10.5665/sleep.1846
MacArthur, J., Bowler, E., Cerezo, M., Gil, L., Hall, P., Hastings, E., et al. (2017). The new NHGRI-EBI Catalog of published genome-wide association studies (GWAS Catalog). Nucleic Acids Res. 45, D896–D901. doi: 10.1093/nar/gkw1133
Mahoney, C. E., Cogswell, A., Koralnik, I. J., and Scammell, T. E. (2019). The neurobiological basis of narcolepsy. Nat. Rev. Neurosci. 20, 83–93. doi: 10.1038/s41583-018-0097-x
Mathieu, H., Patten, S. A., Aragon-Martin, J. A., Ocaka, L., Simpson, M., Child, A., et al. (2021). Genetic variant of TTLL11 gene and subsequent ciliary defects are associated with idiopathic scoliosis in a 5-generation UK family. Sci. Rep. 11:11026. doi: 10.1038/s41598-021-90155-0
Matthews, K. A., Patel, S. R., Pantesco, E. J., Buysse, D. J., Kamarck, T. W., Lee, L., et al. (2018). Similarities and differences in estimates of sleep duration by polysomnography, actigraphy, diary and self-reported habitual sleep in a community sample. Sleep Health 4, 96–103. doi: 10.1016/j.sleh.2017.10.011
McCarty, N. S., Graham, A. E., Studená, L., and Ledesma-Amaro, R. (2020). Multiplexed CRISPR technologies for gene editing and transcriptional regulation. Nat. Commun. 11:1281. doi: 10.1038/s41467-020-15053-x
Moreno-Mateos, M. A., Vejnar, C. E., Beaudoin, J.-D., Fernandez, J. P., Mis, E. K., Khokha, M. K., et al. (2015). CRISPRscan: designing highly efficient sgRNAs for CRISPR-Cas9 targeting in vivo. Nat. Methods 12, 982–988. doi: 10.1038/nmeth.3543
Moschen, A. R., Tilg, H., and Raine, T. (2019). IL-12, IL-23 and IL-17 in IBD: immunobiology and therapeutic targeting. Nat. Rev. Gastroenterol. Hepatol. 16, 185–196. doi: 10.1038/s41575-018-0084-8
Mueller, T., Dong, Z., Berberoglu, M. A., and Guo, S. (2011). The dorsal pallium in zebrafish, Danio rerio (Cyprinidae, Teleostei). Brain Res. 1381, 95–105. doi: 10.1016/j.brainres.2010.12.089
Nath, R. D., Bedbrook, C. N., Abrams, M. J., Basinger, T., Bois, J. S., Prober, D. A., et al. (2017). The jellyfish cassiopea exhibits a sleep-like state. Curr. Biol. 27, 2984–2990.e3. doi: 10.1016/j.cub.2017.08.014
Nath, R. D., Chow, E. S., Wang, H., Schwarz, E. M., and Sternberg, P. W. (2016). C. elegans stress-induced sleep emerges from the collective action of multiple neuropeptides. Curr. Biol. 26, 2446–2455. doi: 10.1016/j.cub.2016.07.048
Nelson, M. D., Lee, K. H., Churgin, M. A., Hill, A. J., Van Buskirk, C., Fang-Yen, C., et al. (2014). FMRFamide-like FLP-13 neuropeptides promote quiescence following heat stress in Caenorhabditis elegans. Curr. Biol. 24, 2406–2410. doi: 10.1016/j.cub.2014.08.037
Oikonomou, G., Altermatt, M., Zhang, R.-W., Coughlin, G. M., Montz, C., Gradinaru, V., et al. (2019). The serotonergic raphe promote sleep in zebrafish and mice. Neuron 103, 686–701.e8. doi: 10.1016/j.neuron.2019.05.038
Oikonomou, G., and Prober, D. A. (2017). Attacking sleep from a new angle: contributions from zebrafish. Curr. Opin. Neurobiol. 44, 80–88. doi: 10.1016/j.conb.2017.03.009
Orger, M. B., and de Polavieja, G. G. (2017). Zebrafish behavior: opportunities and challenges. Annu. Rev. Neurosci. 40, 125–147. doi: 10.1146/annurev-neuro-071714-033857
Ozaki, K., Ohnishi, Y., Iida, A., Sekine, A., Yamada, R., Tsunoda, T., et al. (2002). Functional SNPs in the lymphotoxin-α gene that are associated with susceptibility to myocardial infarction. Nat. Genet. 32, 650–654. doi: 10.1038/ng1047
Özcan, G. G., Lim, S., Leighton, P. L., Allison, W. T., and Rihel, J. (2020). Sleep is bi-directionally modified by amyloid beta oligomers. eLife 9:e53995. doi: 10.7554/eLife.53995
Pack, A. I., Galante, R. J., Maislin, G., Cater, J., Metaxas, D., Lu, S., et al. (2007). Novel method for high-throughput phenotyping of sleep in mice. Physiol. Genomics 28, 232–238. doi: 10.1152/physiolgenomics.00139.2006
Palermo, J., Chesi, A., Zimmerman, A., Sonti, S., Lasconi, C., Brown, E. B., et al. (2021). Variant-to-gene-mapping followed by cross-species genetic screening identifies GPI-anchor biosynthesis as novel regulator of sleep. bioRxiv [Preprint]. doi: 10.1101/2021.12.19.472248
Porcu, E., Rüeger, S., Lepik, K., Santoni, F. A., Reymond, A., and Kutalik, Z. (2019). Mendelian randomization integrating GWAS and eQTL data reveals genetic determinants of complex and clinical traits. Nat. Commun. 10:3300. doi: 10.1038/s41467-019-10936-0
Postlethwait, J. H., Woods, I. G., Ngo-Hazelett, P., Yan, Y. L., Kelly, P. D., Chu, F., et al. (2000). Zebrafish comparative genomics and the origins of vertebrate chromosomes. Genome Res. 10, 1890–1902. doi: 10.1101/gr.164800
Prober, D. A., Rihel, J., Onah, A. A., Sung, R.-J., and Schier, A. F. (2006). Hypocretin/orexin overexpression induces an insomnia-like phenotype in zebrafish. J. Neurosci. 26, 13400–13410. doi: 10.1523/JNEUROSCI.4332-06.2006
Prober, D. A., Zimmerman, S., Myers, B. R., McDermott, B. M. J., Kim, S.-H., Caron, S., et al. (2008). Zebrafish TRPA1 channels are required for chemosensation but not for thermosensation or mechanosensory hair cell function. J. Neurosci. 28, 10102–10110. doi: 10.1523/JNEUROSCI.2740-08.2008
Quick, R. E., Buck, L. D., Parab, S., Tolbert, Z. R., and Matsuoka, R. L. (2021). Highly efficient synthetic CRISPR RNA/Cas9-based mutagenesis for rapid cardiovascular phenotypic screening in F0 zebrafish. Front. Cell Dev. Biol. 9:735598. doi: 10.3389/fcell.2021.735598
Raizen, D. M., Zimmerman, J. E., Maycock, M. H., Ta, U. D., You, Y., Sundaram, M. V., et al. (2008). Lethargus is a Caenorhabditis elegans sleep-like state. Nature 451, 569–572. doi: 10.1038/nature06535
Ram, S., Seirawan, H., Kumar, S. K. S., and Clark, G. T. (2010). Prevalence and impact of sleep disorders and sleep habits in the United States. Sleep Breath. 14, 63–70. doi: 10.1007/s11325-009-0281-3
Reichert, S., Pavon Arocas, O., and Rihel, J. (2019). The neuropeptide galanin is required for homeostatic rebound sleep following increased neuronal activity. Neuron 104, 370–384.e5. doi: 10.1016/j.neuron.2019.08.010
Renier, C., Faraco, J. H., Bourgin, P., Motley, T., Bonaventure, P., Rosa, F., et al. (2007). Genomic and functional conservation of sedative-hypnotic targets in the zebrafish. Pharmacogenet. Genomics 17, 237–253. doi: 10.1097/FPC.0b013e3280119d62
Rihel, J., Prober, D. A., Arvanites, A., Lam, K., Zimmerman, S., Jang, S., et al. (2010). Zebrafish behavioral profiling links drugs to biological targets and rest/wake regulation. Science 327, 348–351. doi: 10.1126/science.1183090
Rossi, A., Kontarakis, Z., Gerri, C., Nolte, H., Holper, S., Kruger, M., et al. (2015). Genetic compensation induced by deleterious mutations but not gene knockdowns. Nature 524, 230–233. doi: 10.1038/nature14580
Ruzzo, E. K., Perez-Cano, L., Jung, J.-Y., Wang, L.-K., Kashef-Haghighi, D., Hartl, C., et al. (2019). Inherited and de novo genetic risk for autism impacts shared networks. Cell 178, 850–866.e26. doi: 10.1016/j.cell.2019.07.015
Sanders, S. J., Neale, B. M., Huang, H., Werling, D. M., An, J.-Y., Dong, S., et al. (2017). Whole genome sequencing in psychiatric disorders: the WGSPD consortium. Nat. Neurosci. 20, 1661–1668. doi: 10.1038/s41593-017-0017-9
Saper, C. B., and Fuller, P. M. (2017). Wake-sleep circuitry: an overview. Curr. Opin. Neurobiol. 44, 186–192. doi: 10.1016/j.conb.2017.03.021
Scammell, T. E., Arrigoni, E., and Lipton, J. O. (2017). Neural circuitry of wakefulness and sleep. Neuron 93, 747–765. doi: 10.1016/j.neuron.2017.01.014
Schaid, D. J., Chen, W., and Larson, N. B. (2018). From genome-wide associations to candidate causal variants by statistical fine-mapping. Nat. Rev. Genet. 19, 491–504. doi: 10.1038/s41576-018-0016-z
Schizophrenia Working Group of the Psychiatric Genomics. (2014). Biological insights from 108 schizophrenia-associated genetic loci. Nature 511, 421–427. doi: 10.1038/nature13595
Sentmanat, M. F., Peters, S. T., Florian, C. P., Connelly, J. P., and Pruett-Miller, S. M. (2018). A survey of validation strategies for CRISPR-Cas9 editing. Sci. Rep. 8:888. doi: 10.1038/s41598-018-19441-8
Shaw, P. J., Cirelli, C., Greenspan, R. J., and Tononi, G. (2000). Correlates of sleep and waking in Drosophila melanogaster. Science 287, 1834–1837. doi: 10.1126/science.287.5459.1834
Siminovitch, K. A. (2004). PTPN22 and autoimmune disease. Nat. Genet. 36, 1248–1249. doi: 10.1038/ng1204-1248
Singh, C., Oikonomou, G., and Prober, D. A. (2015). Norepinephrine is required to promote wakefulness and for hypocretin-induced arousal in zebrafish. eLife 4:e07000. doi: 10.7554/eLife.07000
Singh, C., Rihel, J., and Prober, D. A. (2017). Neuropeptide Y regulates sleep by modulating noradrenergic signaling. Curr. Biol. 27, 3796–3811.e5. doi: 10.1016/j.cub.2017.11.018
Smemo, S., Tena, J. J., Kim, K.-H., Gamazon, E. R., Sakabe, N. J., Gómez-Marín, C., et al. (2014). Obesity-associated variants within FTO form long-range functional connections with IRX3. Nature 507, 371–375. doi: 10.1038/nature13138
Stranges, S., Tigbe, W., Gómez-Olivé, F. X., Thorogood, M., and Kandala, N.-B. (2012). Sleep problems: an emerging global epidemic? Findings from the INDEPTH WHO-SAGE study among more than 40,000 older adults from 8 countries across Africa and Asia. Sleep 35, 1173–1181. doi: 10.5665/sleep.2012
Sun, Y., Tisdale, R. K., and Kilduff, T. S. (2021). Hypocretin/orexin receptor pharmacology and sleep phases. Front. Neurol. Neurosci. 45, 22–37. doi: 10.1159/000514963
Takayasu, S., Sakurai, T., Iwasaki, S., Teranishi, H., Yamanaka, A., Williams, S. C., et al. (2006). A neuropeptide ligand of the G protein-coupled receptor GPR103 regulates feeding, behavioral arousal and blood pressure in mice. Proc. Natl. Acad. Sci. U S A 103, 7438–7443. doi: 10.1073/pnas.0602371103
Tang, W., Davidson, J. D., Zhang, G., Conen, K. E., Fang, J., Serluca, F., et al. (2020). Genetic control of collective behavior in zebrafish. iScience 23:100942. doi: 10.1016/j.isci.2020.100942
Teraoka, H., Russell, C., Regan, J., Chandrasekhar, A., Concha, M. L., Yokoyama, R., et al. (2004). Hedgehog and Fgf signaling pathways regulate the development of tphR-expressing serotonergic raphe neurons in zebrafish embryos. J. Neurobiol. 60, 275–288. doi: 10.1002/neu.20023
Thomas, S. A., Matsumoto, A. M., and Palmiter, R. D. (1995). Noradrenaline is essential for mouse fetal development. Nature 374, 643–646. doi: 10.1038/374643a0
Thomas, H. R., Percival, S. M., Yoder, B. K., and Parant, J. M. (2014). High-throughput genome editing and phenotyping facilitated by high resolution melting curve analysis. PLoS One 9:e114632. doi: 10.1371/journal.pone.0114632
Thyme, S. B., Pieper, L. M., Li, E. H., Pandey, S., Wang, Y., Morris, N. S., et al. (2019). Phenotypic landscape of schizophrenia-associated genes defines candidates and their shared functions. Cell 177, 478–491.e20. doi: 10.1016/j.cell.2019.01.048
Toda, H., Williams, J. A., Gulledge, M., and Sehgal, A. (2019). A sleep-inducing gene, nemuri, links sleep and immune function in Drosophila. Science 363, 509–515. doi: 10.1126/science.aat1650
Torigoe, M., Islam, T., Kakinuma, H., Fung, C. C. A., Isomura, T., Shimazaki, H., et al. (2021). Zebrafish capable of generating future state prediction error show improved active avoidance behavior in virtual reality. Nat. Commun. 12:5712. doi: 10.1038/s41467-021-26010-7
Tropepe, V., and Sive, H. L. (2003). Can zebrafish be used as a model to study the neurodevelopmental causes of autism? Genes Brain Behav. 2, 268–281. doi: 10.1034/j.1601-183x.2003.00038.x
Uffelmann, E., Huang, Q. Q., Munung, N. S., de Vries, J., Okada, Y., Martin, A. R., et al. (2021). Genome-wide association studies. Nat. Rev. Methods Primers 1:59. doi: 10.1038/s43586-021-00056-9
Van Buskirk, C., and Sternberg, P. W. (2007). Epidermal growth factor signaling induces behavioral quiescence in Caenorhabditis elegans. Nat. Neurosci. 10, 1300–1307. doi: 10.1038/nn1981
Van de Water, A. T. M., Holmes, A., and Hurley, D. A. (2011). Objective measurements of sleep for non-laboratory settings as alternatives to polysomnography – a systematic review. J. Sleep Res. 20, 183–200. doi: 10.1111/j.1365-2869.2009.00814.x
Varshney, G. K., Carrington, B., Pei, W., Bishop, K., Chen, Z., Fan, C., et al. (2016). A high-throughput functional genomics workflow based on CRISPR/Cas9-mediated targeted mutagenesis in zebrafish. Nat. Protoc. 11, 2357–2375. doi: 10.1038/nprot.2016.141
Wang, J., Huang, D., Zhou, Y., Yao, H., Liu, H., Zhai, S., et al. (2020). CAUSALdb: a database for disease/trait causal variants identified using summary statistics of genome-wide association studies. Nucleic Acids Res. 48, D807–D816. doi: 10.1093/nar/gkz1026
Wang, H., Lane, J. M., Jones, S. E., Dashti, H. S., Ollila, H. M., Wood, A. R., et al. (2019). Genome-wide association analysis of self-reported daytime sleepiness identifies 42 loci that suggest biological subtypes. Nat. Commun. 10:3503. doi: 10.1038/s41467-019-11456-7
Woods, I. G., Schoppik, D., Shi, V. J., Zimmerman, S., Coleman, H. A., Greenwood, J., et al. (2014). Neuropeptidergic signaling partitions arousal behaviors in zebrafish. J. Neurosci. 34, 3142–3160. doi: 10.1523/JNEUROSCI.3529-13.2014
Wu, R. S., Lam, I. I., Clay, H., Duong, D. N., Deo, R. C., and Coughlin, S. R. (2018). A rapid method for directed gene knockout for screening in G0 zebrafish. Dev. Cell 46, 112–125.e4. doi: 10.1016/j.devcel.2018.06.003
Wullimann, M. F., and Mueller, T. (2004). Teleostean and mammalian forebrains contrasted: evidence from genes to behavior. J. Comp. Neurol. 475, 143–162. doi: 10.1002/cne.20183
Yelin-Bekerman, L., Elbaz, I., Diber, A., Dahary, D., Gibbs-Bar, L., Alon, S., et al. (2015). Hypocretin neuron-specific transcriptome profiling identifies the sleep modulator Kcnh4a. eLife 4:e08638. doi: 10.7554/eLife.08638
Yetish, G., Kaplan, H., Gurven, M., Wood, B., Pontzer, H., Manger, P. R., et al. (2015). Natural sleep and its seasonal variations in three pre-industrial societies. Curr. Biol. 25, 2862–2868. doi: 10.1016/j.cub.2015.09.046
Yokogawa, T., Marin, W., Faraco, J., Pézeron, G., Appelbaum, L., Zhang, J., et al. (2007). Characterization of sleep in zebrafish and insomnia in hypocretin receptor mutants. PLoS Biol. 5:e277. doi: 10.1371/journal.pbio.0050277
Keywords: zebrafish, sleep, behavior, GWAS, CRISPR/Cas9, genetic screen
Citation: Tran S and Prober DA (2022) Validation of Candidate Sleep Disorder Risk Genes Using Zebrafish. Front. Mol. Neurosci. 15:873520. doi: 10.3389/fnmol.2022.873520
Received: 10 February 2022; Accepted: 14 March 2022;
Published: 07 April 2022.
Edited by:
Robert Gerlai, University of Toronto, CanadaReviewed by:
Subhabrata Sanyal, California Life Company (Calico), United StatesCopyright © 2022 Tran and Prober. This is an open-access article distributed under the terms of the Creative Commons Attribution License (CC BY). The use, distribution or reproduction in other forums is permitted, provided the original author(s) and the copyright owner(s) are credited and that the original publication in this journal is cited, in accordance with accepted academic practice. No use, distribution or reproduction is permitted which does not comply with these terms.
*Correspondence: David A. Prober, ZHByb2JlckBjYWx0ZWNoLmVkdQ==
Disclaimer: All claims expressed in this article are solely those of the authors and do not necessarily represent those of their affiliated organizations, or those of the publisher, the editors and the reviewers. Any product that may be evaluated in this article or claim that may be made by its manufacturer is not guaranteed or endorsed by the publisher.
Research integrity at Frontiers
Learn more about the work of our research integrity team to safeguard the quality of each article we publish.