- 1Department of Pharmacology, School of Basic Medical Sciences, Peking University Health Science Center, Beijing, China
- 2Beijing Key Laboratory on Drug Dependence Research, National Institute on Drug Dependence, Peking University, Beijing, China
- 3Department of Gastroenterology of Dapping Hospital, Third Military Medical University, Chongqing, China
- 4Key Laboratory of Molecular Epidemiology of Hunan Province, School of Medicine, Hunan Normal University, Changsha, China
- 5Institute of Mental Health, National Clinical Research Center for Mental Disorders, Key Laboratory of Mental Health and Peking University Sixth Hospital, Peking University, Beijing, China
- 6Peking University, Shenzhen Hospital, Shenzhen, China
Increasing evidence has indicated that circular RNAs (circRNAs) act as competing endogenous RNAs (ceRNAs) regulatory network to regulate the expression of target genes by sponging microRNAs (miRNAs), and therefore play an essential role in many neuropsychiatric disorders, including cocaine use disorder. However, the functional roles and regulatory mechanisms of circRNAs as ceRNAs in dorsolateral prefrontal cortex (dlPFC) of patients with cocaine use disorder remain to be determined. In this study, an expression profiling for dlPFC in 19 patients with cocaine use disorder and 17 controls from Gene Expression Omnibus datasets was used for the differentially expressed circRNAs analysis and the differentially expressed mRNAs analysis. Several tools were used to predict the miRNAs targeted by the circRNAs and the miRNAs targeted mRNAs, which then overlapped with the cocaine-associated differentially expressed mRNAs to determine the functional roles of circRNAs. Functional analysis for the obtained mRNAs was performed via Gene Ontology (GO) in Metascape database. Integrated bioinformatics analysis was conducted to further characterize the circRNA–miRNA–mRNA regulatory network and identify the functions of distinct circRNAs. We found a total of 41 differentially expressed circRNAs, and 98 miRNAs were targeted by these circRNAs. The overlapped mRNAs targeted by the miRNAs and the differentially expressed mRNAs constructed a circRNA–miRNA–mRNA regulation network including 24 circRNAs, 43 miRNAs, and 82 mRNAs in the dlPFC of patients with cocaine use disorder. Functional analysis indicated the regulation network mainly participated in cell response-related, receptor signaling-related, protein modification-related and axonogenesis-related pathways, which might be involved with cocaine use disorder. Additionally, we determined four hub genes (HSP90AA1, HSPA1B, YWHAG, and RAB8A) from the protein–protein interaction network and constructed a circRNA–miRNA-hub gene subnetwork based on the four hub genes. In conclusion, our findings provide a deeper understanding of the circRNAs-related ceRNAs regulatory mechanisms in the pathogenesis of cocaine use disorder.
Introduction
Cocaine addiction inflicts enormous health and economic costs to individuals, families, and society (Reid et al., 2012; United Nations Office on Drugs and Crime, 2020). Recently, significantly increased studies have focused on the field of neuroscience of cocaine use disorder, but its neurobiological mechanism is still unclear, and there is no effective clinical treatment for cocaine use disorder (Gawin and Ellinwood, 1989; Majewska, 1996a,b; Heal et al., 2014).
Epigenetic mechanisms can integrate both genetic and diverse environmental stimuli to exert potent and often long-lasting changes in gene expression (Jaenisch and Bird, 2003). Accumulating research has found epigenetic mechanism plays an important role in the drug addiction (Robison and Nestler, 2011; Nestler, 2014; Nestler and Lüscher, 2019). Non-coding RNAs, specifically long non-coding RNAs, circular RNAs (circRNAs), and small non-coding RNAs, are one type of common epigenetic regulators that play a vital role in many biological processes associated with diseases (Amin et al., 2019; Mehta et al., 2020).
Circular RNAs are vastly conserved non-coding RNAs formed by back-splicing and covalent fusion of RNA free ends into natural circles (Vicens and Westhof, 2014; Szabo and Salzman, 2016; Greene et al., 2017; Li et al., 2018). Because circRNAs lack poly(A) tails and cap structure, they are not affected by RNA exonuclease (Vicens and Westhof, 2014; Szabo and Salzman, 2016; Greene et al., 2017; Li et al., 2018). CircRNAs usually exert their functions as transcriptional and post-transcriptional regulators through various functional mechanisms, such as RNA binding protein (RBP) “sponges” (Du et al., 2016; Holdt et al., 2016), translated proteins (Legnini et al., 2017; Pamudurti et al., 2017), and RNA–RNA interaction (Li et al., 2015). At present, circRNAs function mainly by absorbing microRNAs (miRNAs) as competing endogenous RNAs (ceRNAs) regulatory network to regulate their target genes expression, which construct a functional circRNA–miRNA–mRNA regulation network (Hansen et al., 2013; Vicens and Westhof, 2014; Rybak-Wolf et al., 2015; Du et al., 2017; Greene et al., 2017; Li et al., 2018; Mehta et al., 2020). For example, knockdown of circHIPK2 expression significantly inhibited astrocyte activation induced by methamphetamine through the targeting of miR124 and SIGMAR1 (Huang et al., 2017). Another study reported that circTmeff-1 promotes incubation of context-induced morphine craving by sponging miR-541/miR-6934 in the nucleus accumbens (Yu et al., 2021).
Although several circRNAs have been identified as participating in cocaine addiction, the regulatory networks in patients with cocaine use disorder are still unknown. It is necessary to conduct the circRNA–miRNA–mRNA regulatory networks in patients with cocaine use disorder to help to advance our understanding of the molecular mechanism of cocaine use disorder. Dorsolateral prefrontal cortex (dlPFC), similar role to medial PFC in rodents (Seamans et al., 2008), is a crucial component brain region of inhibitory control (Gass and Chandler, 2013; Moeller et al., 2014), which undergoes significant changes after long-term cocaine use (Matochik et al., 2003; Moreno-Lopez et al., 2012) and is involved in compulsive drug-seeking behaviors, increasing drug intake and addiction severity (Chen B. T. et al., 2013; Conti and Nakamura-Palacios, 2014; Terraneo et al., 2016). In this study, we aimed to investigate the functional circRNA–miRNA–mRNA regulatory networks in the dlPFC of patients with cocaine use disorder. Lastly, we constructed a circRNA–miRNA–mRNA regulation network including 24 circRNAs, 43 miRNAs, and 82 mRNAs, which may reveal a novel molecular mechanism in pathogenesis of patients with cocaine use disorder.
Materials and Methods
Data Collection
The circRNAs expression data were obtained from GSE99349 in GEO database.1 The data were generated using RNA sequencing (RNA-seq) of human postmortem dlPFC neuronal nuclei for 19 patients with cocaine use disorder and 17 unaffected controls. All patients who met criteria for cocaine use disorder were identified sudden deaths due to the toxic effects of chronic cocaine abuse (Ribeiro et al., 2017). Unaffected controls, who were selected from homicides, accidental or natural deaths, were drug-free age-matched subjects. Post-mortem interval (PMI), RNA integrity number (RIN), age, and race are provided in the original paper and do not significantly differ between cases and controls (Ribeiro et al., 2017). In the original study, the authors analyzed the differentially expressed genes and non-coding linear RNAs, but did not analyze the circRNAs. We further analyzed the circRNAs using the data of GSE99349 and used the differentially expressed genes in the original study to overlap predicted genes.
In addition, we collected some differentially expressed mRNAs from PFC RNA-seq data of different cocaine addiction animal models (GSE124952 and GSE89572) (Li et al., 2017; Bhattacherjee et al., 2019).
Identification of Differentially Expressed Circular RNAs
Cutadapt (Martin, 2011) was used to remove the reads that contained adaptor contamination, low-quality bases, and undetermined bases. Next, sequence quality was verified using FastQC (Andrews, 2010). Bowtie 2 was used to map reads to the human genome hg37 (Langmead and Salzberg, 2012). CIRI2 was initially used for de novo assembly of the mapped reads into circRNAs (Gao et al., 2018); subsequently, back-splicing reads were identified in unmapped reads using CIRI2. The total reads and the number of mapped reads per sample is shown in Supplementary Table 1. The differentially expressed of circRNAs were calculated using R package edgeR (Robinson et al., 2010). Only the comparisons with P-value < 0.05 and fold change ≥ 1.5 were regarded as differential expressed circRNAs.
Target MicroRNAs and mRNAs Prediction and Regulatory Network Establishment
MicroRNAs targeted by circRNAs were predicted using miRDB (target score >80) (Liu and Wang, 2019; Chen and Wang, 2020). Putative miRNAs were listed based on competitive binding ability, the top five miRNAs for each circRNA were mainly considered as circRNA target (Lv et al., 2018) and selected for further targeted mRNA predictions using TargetScan (score <−0.4) (Agarwal et al., 2015), DIANA-microT (score >0.8) (Paraskevopoulou et al., 2013), Tarbase (Vergoulis et al., 2012), and miRDB (score >80) (Liu and Wang, 2019). TargetScan, DIANA-microT, and TarBase are based on DIANA-miRPath v.3 platform (Fromm et al., 2015; Vlachos and Hatzigeorgiou, 2017). Only the target mRNAs presented in at least 3 out of 4 databases were considered as target genes of the given miRNAs. The targeted mRNAs were then overlapped with the differentially expressed mRNA data of the dlPFC neurons of patients with cocaine use disorder (Ribeiro et al., 2017). Last, a circRNA–miRNA–mRNA regulatory network was constructed. Cytoscape (Shannon et al., 2003) (version 3.6.0) was used to delineate the cocaine-related gene regulatory network.
Gene Set Enrichment Analysis for mRNAs in the Regulatory Network
To assess functional enrichment, Metascape Gene Ontology (GO) terms were used to perform gene set enrichment analysis for the mRNAs in the circRNA–miRNA–mRNA network (Zhou et al., 2019). The thresholds of enrichment analysis were set as GO terms with P < 0.01 and the count of genes involved in the GO terms ≥3.
Establishment of Protein–Protein Interaction Network and Identification of Hub Genes
The protein–protein interaction (PPI) network of the mRNAs in the circRNA–miRNA–mRNA network was established using the STRING database (Szklarczyk et al., 2017; Doncheva et al., 2019), and then visualized using Cytoscape software (Shannon et al., 2003). Subsequently, cytoHubba app (Chin et al., 2014) of Cytoscape was used to determine the hub genes. According to the degree ranks of cytoHubba app, the nodes degree ≥5 were considered as hub genes. The structure pattern of several vital circRNAs associated with hub genes were drawn using the database CSCD (Feng et al., 2021), which can be used for predicting miRNA response element, RBP, and open reading frame to better explore the potentially functional mechanisms of the selected circRNA.
Results
Identification of Differentially Expressed Circular RNAs in Dorsolateral Prefrontal Cortex of Patients With Cocaine Use Disorder
A total 2,046 circRNAs were identified in the GSE99349 dataset, and exon-derived circRNA account for 77.4% (Figure 1A). Among these, 16 up-regulated circRNAs and 25 down-regulated circRNAs with fold changes ≥ 1.5 and P-values ≤ 0.05 were considered as significantly differentially expressed circRNAs (Figure 1B). Among the differentially expressed circRNAs, 65.9% had already existed in the circBase database (Glažar et al., 2014), 14 were de novo significantly differentially expressed circRNAs (Tables 1, 2). Of the differentially expressed circRNAs, 90.24% were covered in the exon of the genome (Figure 1C), others aligned with intron or other sequences. Interestingly, non-coding RNA MALAT1 produced seven circRNAs (named circMALAT1-1 to circMALAT1-7 in Tables 1, 2). Additionally, the chromosome distribution of the circRNAs showed no significant differences (Figure 1D).
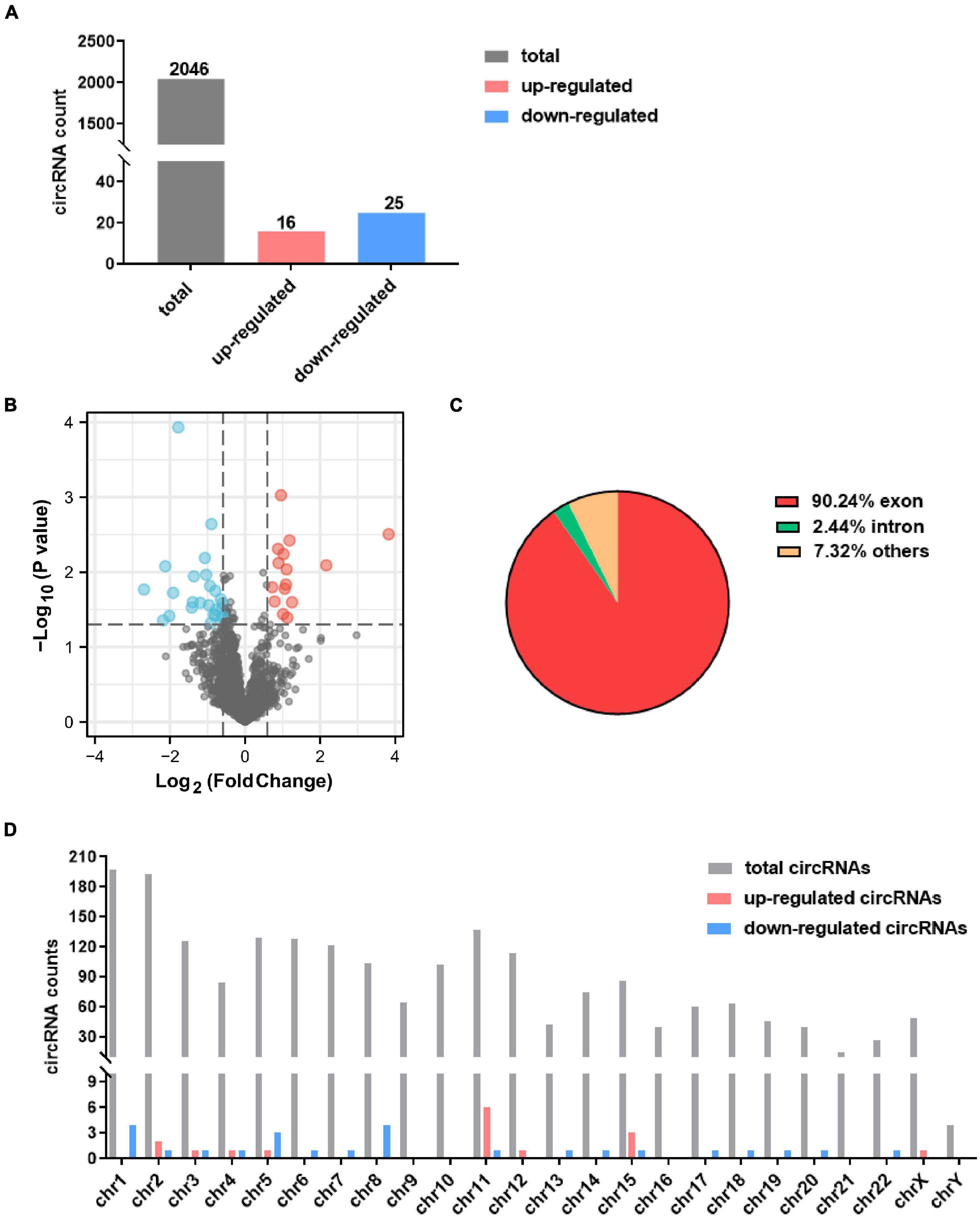
Figure 1. Identification of differentially expressed circRNAs in dorsolateral prefrontal cortex neurons of patients with cocaine use disorder. (A) The count of identified circRNAs. (B) Volcano plot showing circRNAs expression in patients with cocaine use disorder and unaffected controls. The red and blue dots represent circRNAs with statistically significant differences in expression. (C) Pie chart showing the percentage of circRNAs derived from different genomic regions. (D) Distributions of identified circRNAs along the chromosomes.
Construction of the circRNA–miRNA–mRNA ceRNAs Network
Given the potential regulatory roles of circRNAs on recruiting miRNAs to regulate the expression of target genes, we predicted the miRNA “sponges” of circRNA using miRDB database, and 98 miRNAs were found to be closely targeted by the differentially expressed circRNAs. These 98 miRNAs further targeted 2,115 mRNA genes, among which, 82 mRNAs were overlapped with the differentially expressed mRNA of dlPFC neurons of patients with cocaine use disorder (Ribeiro et al., 2017), including 22 up-regulated mRNAs and 60 down-regulated mRNAs (Figures 2A,B). Ultimately, the 82 target mRNAs were targeted by 43 miRNAs, and the miRNAs were further targeted by 24 circRNAs, which formed a circRNA–miRNA–mRNA network for further study (Figure 2C).
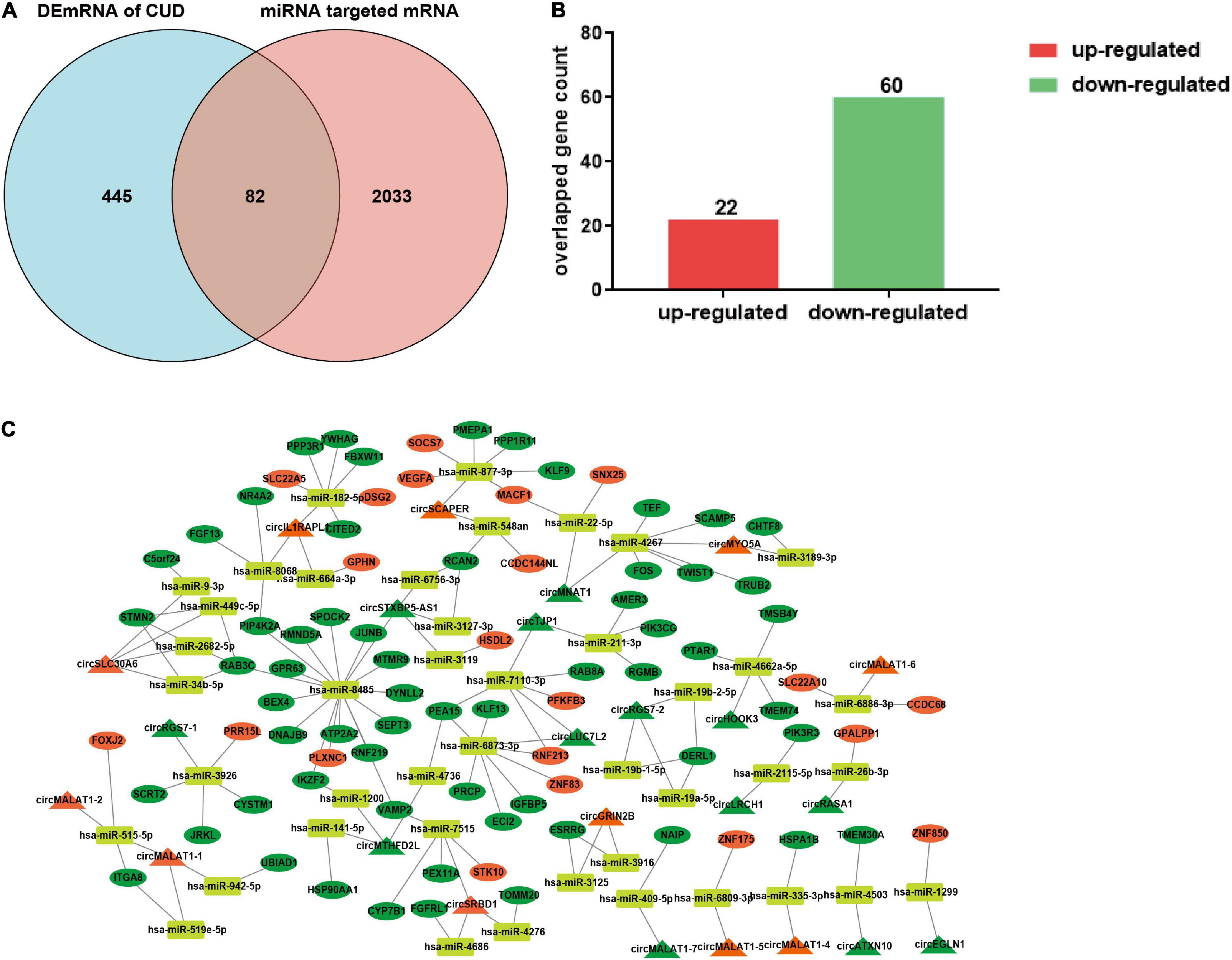
Figure 2. Construction of the ceRNA network. (A) The shared genes between differentially expressed mRNAs in dlPFC neurons of patients with cocaine use disorder and predicted mRNAs using the miRNAs targeted by circRNAs. (B) Histogram showing the up-regulated mRNAs and down-regulated mRNAs in shared genes. (C) The circRNA–miRNA–mRNA regulatory network. The triangle, ellipse, rectangle, respectively presents circRNAs, mRNAs, and miRNAs. Orange triangle and ellipse represents up-regulated circRNAs and mRNAs, respectively; green triangle and ellipse represents down-regulated circRNAs and mRNAs, respectively. DEmRNAs, differentially expressed mRNAs; CUD, cocaine use disorder.
Functional and Pathway Enrichment Analyses
Gene Ontology pathway enrichment analysis for the 82 genes aberrantly expressed in the patients with cocaine use disorder and indirectly regulated by circRNAs revealed that the 22 up-regulated genes participated in vital biological processes including positive regulation of axonogenesis (GO: 0050772) and cell junction organization (GO: 0034330) (Figure 3A), which is consistent with current reports on the relationship between cocaine addiction and the synaptic transmission (Li et al., 2021; Wang et al., 2021; Zinsmaier et al., 2021). Moreover, the 62 down-regulated genes participated in vital biological processes including cellular response (GO:0032870, GO:0034605, and GO:0048511), protein modification and transport process (GO:0006986, GO:0046854, GO:0051258, GO:0031400, and GO:0017038), intracellular receptor and calcium-ion (GO:0030522, GO:0017156, and GO:0019722), cell and tissue morphogenesis (GO:0030099, GO:0001764, GO:0060538, GO:0048729, and GO:0030010), autophagy (GO:0006914), and positive regulation of cytokine production (GO:0001819) (Figure 3B), which implied that the etiology of cocaine use disorder may involve many biological processes.
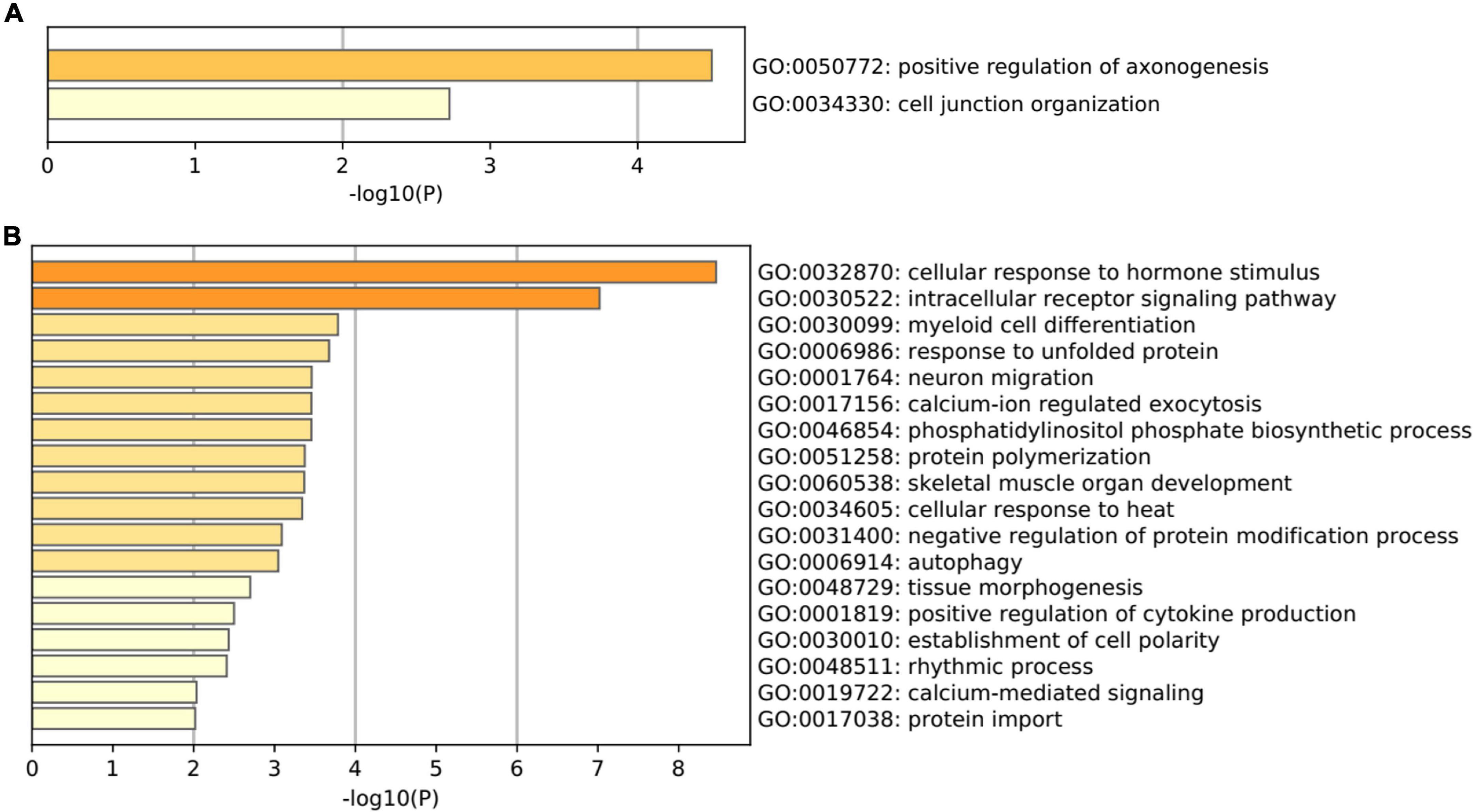
Figure 3. Gene Ontology terms enriched by the shared genes between differentially expressed mRNAs in dlPFC neurons of patients with cocaine use disorder and predicted mRNAs using the miRNAs targeted by circRNAs. (A) GO terms enriched by the up-regulated mRNAs. (B) GO terms enriched by the down-regulated mRNAs.
Establishment of Protein–Protein Interaction Network and Identification of Hub Genes
Based on the STRING database, among the 82 genes aberrantly expressed in the patients with cocaine use disorder and indirectly regulated by circRNAs, 38 genes formed a PPI network, containing 38 nodes and 47 edges (Figure 4A). The highest-scoring nodes (degree ≥5) were screened as hub genes: HSP90AA1, HSPA1B, YWHAG, and RAB8A (Figure 4B). It is well known that hub nodes with high degrees of connectivity have vital functions in biological networks (Han et al., 2004; Wang et al., 2018). Hence, we used these genes to construct a circRNA–miRNA-hub gene subnetwork (Figure 4C): circMTHFD2L/hsa-miR-141-5p/HSP90AA1, circMALAT1-4/hsa-miR-335-3p/HSPA1B, circIL1RAPL1/hsa-miR-182-5p/YWHAG, circTJP1/hsa-miR-7110-3p/RAB8A, and circLUC7L2/hsa-miR-7110-3p/RAB8A. Based on the circRNA–miRNA-hub gene subnetwork, five circRNAs (circMTHFD2L, circMALAT1-4, circIL1RAPL1, circTJP1, and circLUC7L2) were likely to play important roles in cocaine use disorder. In order to further recover the function of the five vital circRNAs, the structural patterns of these vital circRNAs were shown in Figure 5.
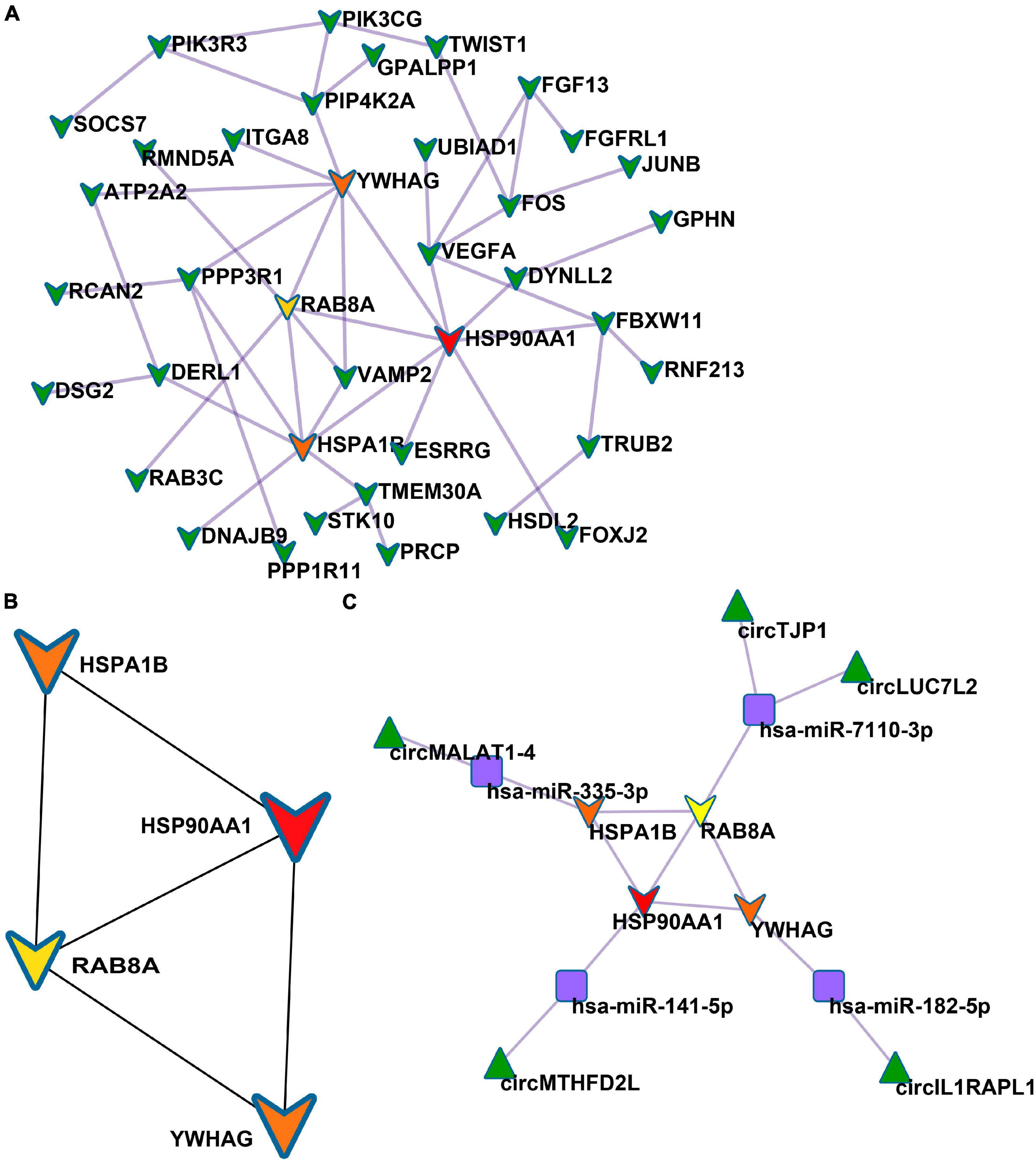
Figure 4. Identification of hub genes from the PPI network. (A) PPI network containing 38 nodes and 47 edges. (B) PPI network of four hub genes that extracted from the PPI network. (C) The circRNA–miRNA-hub gene network.
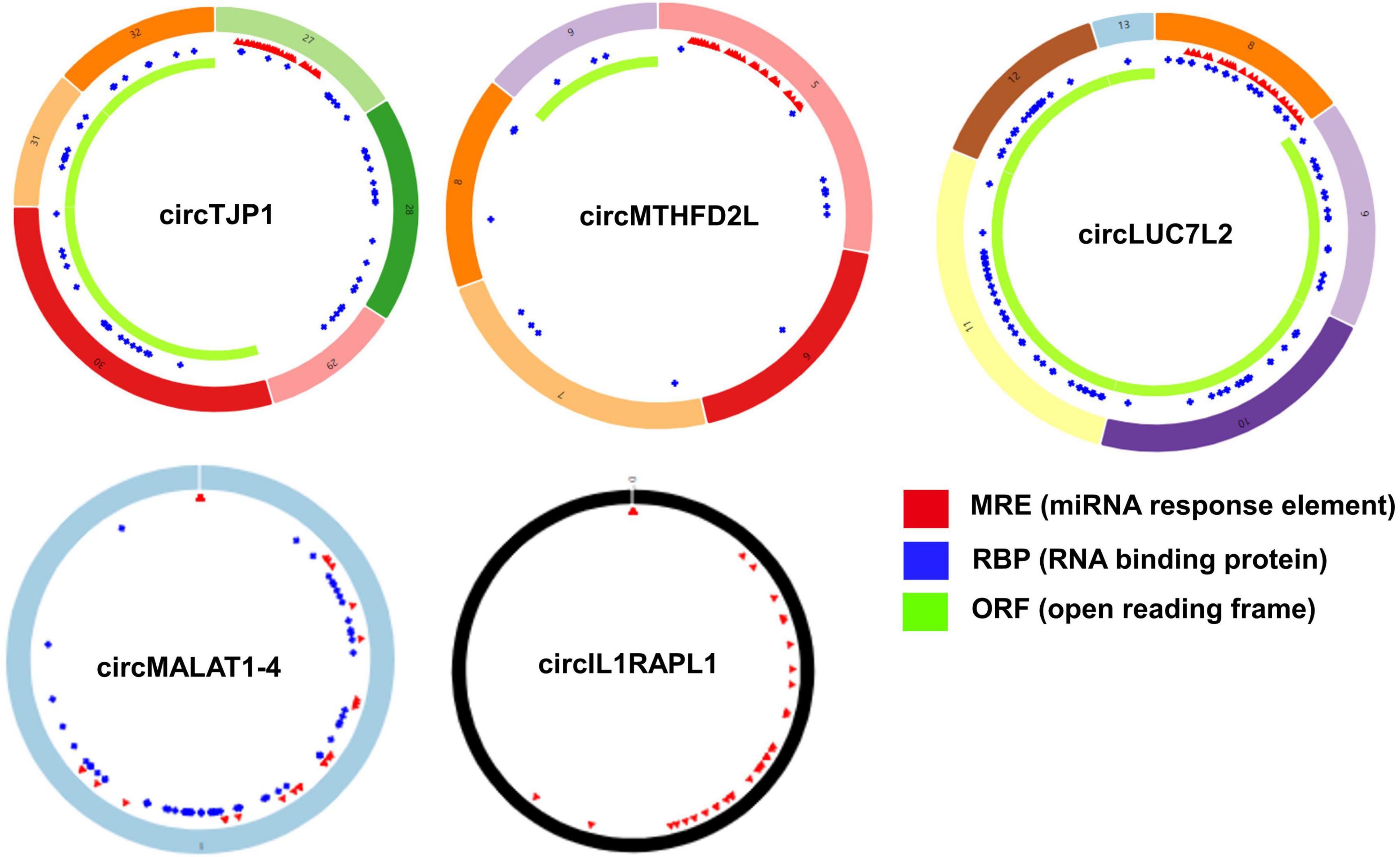
Figure 5. Structural patterns of the five important circRNAs from circRNA–miRNA-hub gene network. Structural patterns of circTJP1, circMTHFD2L, circLUC7L2, circMALAT1-4, and circIL1RAPL1. The colored circle represents the circRNAs that consist of exons. The numbers on the circRNAs mean the exon number. The red, blue, and green regions inside the circRNA molecule, respectively represent MRE (microRNA response element), RBP (RNA binding protein), and ORF (open reading frame).
Circular RNA–MicroRNA–mRNA Network Regulation in Different Cocaine Addiction Animal Model
To further verify the “sponge” function of circRNAs in cocaine addiction, we utilized the differentially expressed mRNAs from PFC RNA-seq data of different cocaine addiction models to overlap with the predicted mRNA indirectly regulated by circRNAs and differentially expressed mRNA in dlPFC of patients with cocaine use disorder. Many genes participate in the circRNA–miRNA–mRNA network regulation (Figure 6A) in the chronic cocaine exposure models with different withdrawal time points. However, only one gene, FOS, was overlapped in all different withdrawal time points (Table 3). Similarly, there were many genes in the circRNAs–miRNA–mRNA network involving in cocaine self-administration model (Figure 6B). As shown in Table 4, hub gene YWHAG and HSP90AA1 participated in cocaine maintains and withdrawal 15 days, respectively.
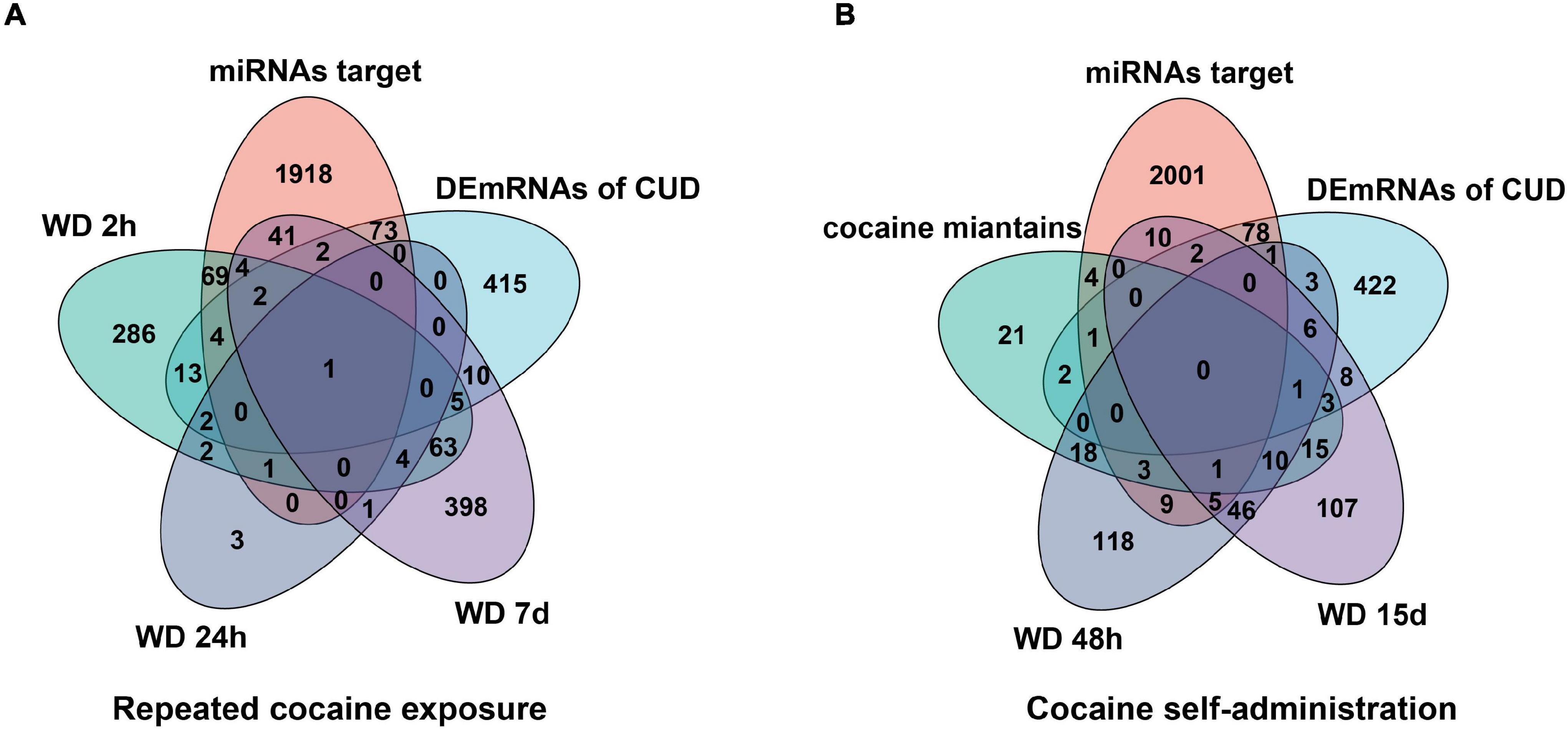
Figure 6. Overlap analysis for the mRNAs from the circRNAs–miRNA–mRNA network with the differentially expressed genes in different cocaine addiction animal model. (A) The shared genes between mRNAs in ceRNA network and differentially expressed mRNAs in different withdrawal time points after repeated cocaine exposure. (B) The shared genes between mRNAs in ceRNA network and differentially expressed mRNAs in different withdrawal time points after cocaine self-administration. miRNA target, predicted mRNAs using miRNAs targeted by circRNAs; human mRNA, differentially expressed mRNAs in dlPFC neuron of human with cocaine use disorder; WD 2h, withdrawal 2 h; WD 24h, withdrawal 24h; WD 48 h, withdrawal 48h; WD 7d, withdrawal 7 days; WD 15d, withdrawal 15 days.
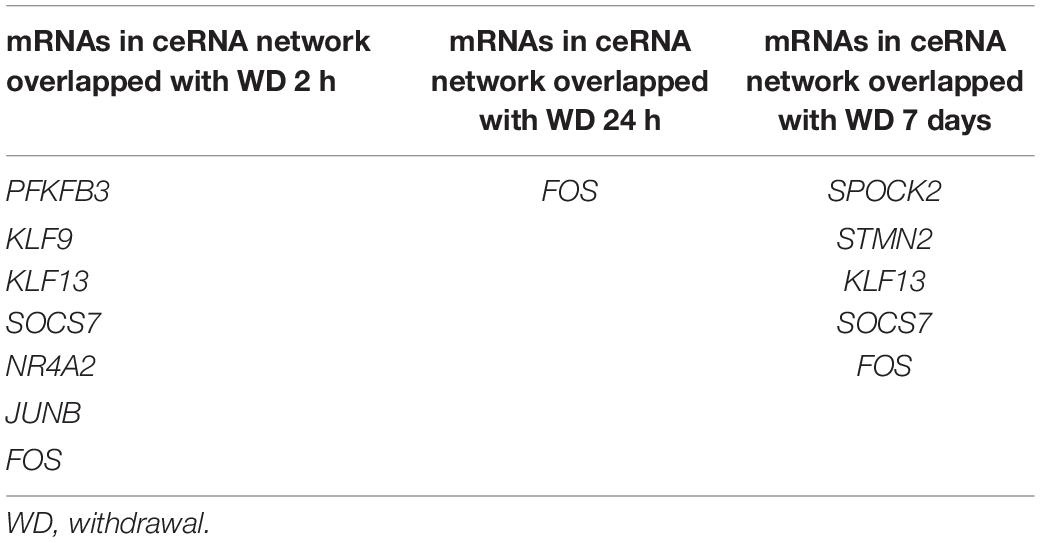
Table 3. The shared genes between mRNAs in ceRNA network and differentially expressed mRNAs in different withdrawal time points after repeated cocaine exposure.
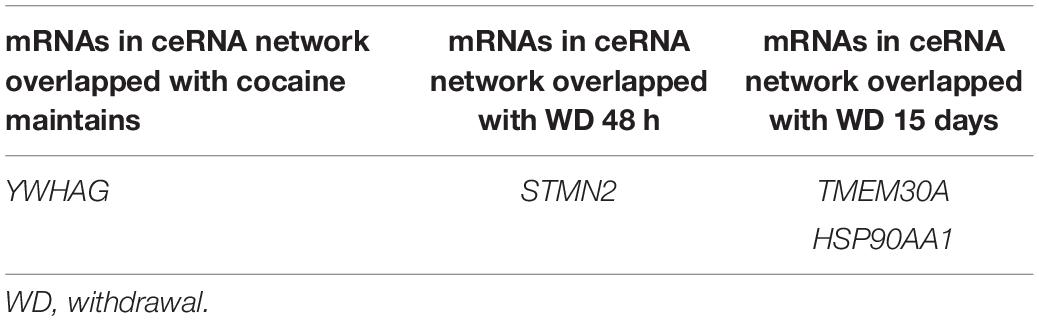
Table 4. The shared genes between mRNAs in ceRNA network and differentially expressed mRNAs in different withdrawal time points after cocaine self-administration.
Discussion
Most of previous research on the mechanism of addiction was based on animal models, or the peripheral blood of patients with substance use disorders; however, the studies do not truly portray the changes that occur in the brains of patients with substance use disorders, which may be an important obstruction to the study of drugs for the treatment of substance use disorders. The circRNA–miRNA–mRNA regulatory network we constructed will enhance the understanding of the addiction mechanism in the brain of patients with cocaine use disorder.
Through the functional enrichment analysis of the mRNAs in the network, we found that the up-regulated mRNAs were mainly involved in regulation of axonogenesis and cell junction, which suggests that our up-regulated mRNA may have a close connection with synaptic transmission, and previous studies have confirmed that abnormal synaptic transmission is a very critical factor for cocaine addiction (Khibnik et al., 2016; Martínez-Rivera et al., 2017; Li et al., 2021). Down-regulated mRNAs were found to be involved in many biological processes, including cellular response to hormone stimulus, response to unfolded protein, cellular response to heat, intracellular receptor signaling pathway, myeloid cell differentiation, calcium-ion regulated exocytosis, calcium-mediated signaling, and autophagy. All the biological processes related to down-regulated mRNAs in the circRNA–miRNA–mRNA regulatory network have been involved with cocaine addiction. For example, clinical trials have investigated that cocaine associated cues could significantly increase adrenocorticotropic hormone and cortisol (Berger et al., 1996). A single dose of cocaine can cause the accumulation of different heat shock proteins (Salminen et al., 1997), which leads to blood-brain barrier breakdown and brain edema formation thereby promoting cocaine intoxication (Sharma et al., 2009). Apart from this, Cocaine has the propensity to cause hyperthermia which increases the mortality rates to cocaine (Crandall et al., 2002). These are also evidences reported that various intracellular receptors, especially dopamine receptors and glutamate receptors, are all critical for cocaine addiction (Ellenbroek, 2013; Howell and Cunningham, 2015; Smaga et al., 2019). In addition, brain myeloid cells, particularly microglia, presented in the brain parenchyma, serve as a surveillance function for neuroinflammation and neurodegeneration in the central nervous system (Ransohoff and Cardona, 2010; Ajami et al., 2018; Jordão et al., 2019). Addictive drugs, especially cocaine, have been consistently shown to activate microglia both in vitro and in vivo (Guo et al., 2015; Liao et al., 2016). In rodents, inhibiting glial cell activation was shown to block cocaine-mediated behavioral changes (Chen et al., 2009). In humans, cocaine exposure can reduce microglial cells viability and inhibit the expression of extracellular vesicle-associated proteins disrupting cellular signaling and cell-to-cell communication (Kumar et al., 2020). Therefore, it can be considered that circRNAs were thought to play an important role in the multiple addiction-related networks in dlPFC of patients with cocaine use disorder.
Circular RNAs are endogenous non-coding RNAs with widespread distribution and various cellular function (Hansen et al., 2013; Vicens and Westhof, 2014; Rybak-Wolf et al., 2015; Du et al., 2017; Greene et al., 2017; Li et al., 2018; Mehta et al., 2020). Numerous studies have shown that circRNAs have an important influence on many complicated neuropsychiatric disorders (Cui et al., 2016; Zhang et al., 2018; An et al., 2019; Liu et al., 2019; Mahmoudi et al., 2019; Huang et al., 2020; Zhang Y. et al., 2020; Zimmerman et al., 2020), including drug addiction (Huang et al., 2017; Bu et al., 2019; Li et al., 2019, 2020; Zhang H. et al., 2020). Knockdown of circHomer1 ameliorates methamphetamine-induced neuronal injury through inhibiting Bbc3 expression (Li et al., 2020). The abnormal expression of mmu_circRNA_002381 in striatum was induced by cocaine self-administration and cocaine-induced locomotor activity model (Bu et al., 2019). Interestingly, siRNA-mediated mmu_circRNA_002381 down-regulation increased the expressions of limk1 and bdnf, which are the targets of miR-138 associated with synaptic plasticity. Additionally, some studies predicted that circRNAs are involved in the progress and development of many addictive drug models by sponging miRNA to regulate downstream targets (Li et al., 2017, 2020; Bu et al., 2019; Zhang H. et al., 2020). In our study, 24 circRNAs were identified to be involved in the circRNA–miRNA–mRNA regulatory network. Among these, 15 were identified previously in the mammalian brain as being dysregulated during neuronal differentiation and highly enriched in synapses (Rybak-Wolf et al., 2015). It was suggested that circRNAs in the circRNA–miRNA–mRNA regulatory network may play their regulatory functions in the neurons or synapses of patients with cocaine use disorder.
Several studies have revealed that circRNAs contain multiple miRNA response elements and can bind to miRNAs, often termed as “miRNA sponges,” decreasing cytoplasmic levels of miRNAs and liberating their respective downstream target mRNAs (Memczak et al., 2013; Piwecka et al., 2017; Kleaveland et al., 2018; Zhang Y. et al., 2020). Here, we constructed a circRNA–miRNA–mRNA regulation network involved in cocaine use disorder, including 24 circRNAs, 42 miRNAs, and 82 mRNAs. CircSLC30A6 was down-regulated in the dlPFC of patients with cocaine use disorder. Based on the analysis of circRNA–miRNA–mRNA network, we found that circSLC30A6 interacts with hsa-miR-9-3p. Interestingly, it has been known that hsa-miR-9-3p mediates the dynamic regulation of neural progenitor proliferation during neurogenesis (Pascale et al., 2020). Moreover, hsa-miR-9-3p is significantly increased in serums of patients with methamphetamine use disorder compared with normal controls (Gu et al., 2020). Therefore, we assume that circSLC30A6 up-regulation induced by the cocaine may be involved in cocaine effect through interacting with hsa-miR-9-3p. CircRASA1 and circMNAT1 were down-regulated in the dlPFC of patients with cocaine use disorder. Through the analysis of circRNA–miRNA–mRNA network, we found that circRASA1 interacts with hsa-miR-26b-3p and circMNAT1 interacts with hsa-miR-22-5p. miR-26b was found to be up-regulated in hippocampus following the acquisition and extinction but miR-22 was only up-regulated during extinction of cocaine-induced conditioned place preference in rats (Chen C. L. et al., 2013). Therefore, it is conceivable that circSLC30A6, circRASA1, and circMNAT1 might play an important role in cocaine use disorder through modulating their target miRNA.
To further identify the key circRNAs participating in the regulatory network, we established a PPI network and screened four hub genes, including HSP90AA1, HSPA1B, YWHAG, and RAB8A. Accordingly, we constructed a circRNA–miRNA-hub gene subnetwork. In the cocaine self-administration model, HSP90AA1 had a significant decrease in PFC after withdrawal of 15 days (Bhattacherjee et al., 2019), however, the expression of HSP90AA1 in posterior hippocampus increased significantly after 28 days of withdrawal (García-Fuster et al., 2012). These delayed neurobiological effects of HSP90AA1 likely contribute to sustained vulnerability to cocaine relapse, which may be regulated by circMTHFD2L. HSPA1B gene is one of heat shock protein 70 (HSP70)-encoding transcripts, and it is reported that HSPA1B expression was increased in the postmortem brains of patients with cocaine use disorder exhibiting excited delirium in comparison with other (non-excited delirium) cocaine-related deaths and drug-free controls, concluding that elevated HSPA1B provides a reliable forensic biomarker for the identification of excited delirium (Mash et al., 2009; Johnson et al., 2012). The structure of circRNA makes them more stable and has a longer half-life, so it is considered to be a more ideal marker (Enuka et al., 2016). Previous studies reported that cocaine exposure dysregulated the expression of YWHAG (Bhattacherjee et al., 2019), and reduced YWHAG can lead to neuronal hyperexcitability, and normalization of hyperexcitability can rescue memory deficits (Roy et al., 2021). RAB8A is a member of the RAS superfamily, which are key regulators of intracellular membrane trafficking from the formation of transport vesicles to their fusion with membranes, and involve in polarized vesicular trafficking, and neurotransmitter release (Núñez et al., 2009; Esseltine et al., 2012; Sellier et al., 2016; Nüchel et al., 2018). Although no studies have shown that RAB8A has a direct effect on cocaine addiction, the biological process in which it participates is very important in cocaine addiction (Periyasamy et al., 2016; Harraz et al., 2021). Here, we identified five circRNA–miRNA-hub gene axes, indicating competitive regulatory relationships of five circRNAs with the four genes in cocaine use disorder. Nevertheless, the expression of downstream genes may be regulated by multiple circRNAs and miRNAs, the expression of the five key circRNAs may be not significantly correlated with its potential downstream gene targets in the sequencing data. Cocaine use disorder is a complex brain disease in which many factors, such as cell subtype specificity, synaptic plasticity, and neural circuit, can influence genes expression. The bioinformatics analysis, which integrated several datasets, could only provide a possible research direction, how the circRNAs contributes to the specific mechanism of cocaine use disorder requires more in-depth studies.
Conclusion
In conclusion, our research is the first to use dlPFC circRNAs and mRNA of patients with cocaine use disorder via bioinformatic tools to identify a circRNA–miRNA–mRNA regulatory network in the patients with cocaine use disorder. The circRNA–miRNA-hub genes regulatory sub-network uncovered five important circRNAs that might be involved in cocaine use disorder, providing new insight into the pathogenesis of cocaine use disorder and suggesting potential therapeutic targets that warrant further investigation.
Data Availability Statement
Publicly available datasets were analyzed in this study. This data can be found here: http://www.ncbi.nlm.nih.gov/geo/, GSE99349, GSE124952, and GSE89572.
Author Contributions
YC, JS, and SC contributed toward conception and design of research. YC and XL analyzed the data and wrote the manuscript together. YC, JS, SM, SC, XL, and SH interpreted the results and revised the manuscript. All authors have read and approved the final version of the manuscript.
Funding
This research was funded by the Ministry of Science and Technology of China (2021ZD0202100), National Natural Science Foundation of China (U1802283 and 82130040), and Beijing Municipal Science & Technology Commission (Z181100001518005).
Conflict of Interest
The authors declare that the research was conducted in the absence of any commercial or financial relationships that could be construed as a potential conflict of interest.
Publisher’s Note
All claims expressed in this article are solely those of the authors and do not necessarily represent those of their affiliated organizations, or those of the publisher, the editors and the reviewers. Any product that may be evaluated in this article, or claim that may be made by its manufacturer, is not guaranteed or endorsed by the publisher.
Supplementary Material
The Supplementary Material for this article can be found online at: https://www.frontiersin.org/articles/10.3389/fnmol.2022.839233/full#supplementary-material
Footnotes
References
Agarwal, V., Bell, G. W., Nam, J. W., and Bartel, D. P. (2015). Predicting effective microRNA target sites in mammalian mRNAs. Elife 4:e05005. doi: 10.7554/eLife.05005
Ajami, B., Samusik, N., Wieghofer, P., Ho, P. P., Crotti, A., Bjornson, Z., et al. (2018). Single-cell mass cytometry reveals distinct populations of brain myeloid cells in mouse neuroinflammation and neurodegeneration models. Nat. Neurosci. 21, 541–551. doi: 10.1038/s41593-018-0100-x
Amin, N., McGrath, A., and Chen, Y.-P. P. (2019). Evaluation of deep learning in non-coding RNA classification. Nat. Mach. Intell. 1, 246–256. doi: 10.1038/s42256-019-0051-2
An, T., He, Z. C., Zhang, X. Q., Li, J., Chen, A. L., Tan, F., et al. (2019). Baduanjin exerts anti-diabetic and anti-depression effects by regulating the expression of mRNA, lncRNA, and circRNA. Chin. Med. 14:3. doi: 10.1186/s13020-019-0225-1
Berger, S. P., Hall, S., Mickalian, J. D., Reid, M. S., Crawford, C. A., Delucchi, K., et al. (1996). Haloperidol antagonism of cue-elicited cocaine craving. Lancet 347, 504–508. doi: 10.1016/s0140-6736(96)91139-3
Bhattacherjee, A., Djekidel, M. N., Chen, R., Chen, W., Tuesta, L. M., and Zhang, Y. (2019). Cell type-specific transcriptional programs in mouse prefrontal cortex during adolescence and addiction. Nat. Commun. 10:4169. doi: 10.1038/s41467-019-12054-3
Bu, Q., Long, H., Shao, X., Gu, H., Kong, J., Luo, L., et al. (2019). Cocaine induces differential circular RNA expression in striatum. Transl. Psychiatry 9:199. doi: 10.1038/s41398-019-0527-1
Chen, B. T., Yau, H. J., Hatch, C., Kusumoto-Yoshida, I., Cho, S. L., Hopf, F. W., et al. (2013). Rescuing cocaine-induced prefrontal cortex hypoactivity prevents compulsive cocaine seeking. Nature 496, 359–362. doi: 10.1038/nature12024
Chen, C. L., Liu, H., and Guan, X. (2013). Changes in microRNA expression profile in hippocampus during the acquisition and extinction of cocaine-induced conditioned place preference in rats. J. Biomed. Sci. 20:96. doi: 10.1186/1423-0127-20-96
Chen, H., Uz, T., and Manev, H. (2009). Minocycline affects cocaine sensitization in mice. Neurosci. Lett. 452, 258–261. doi: 10.1016/j.neulet.2009.01.078
Chen, Y., and Wang, X. (2020). MIRDB: an online database for prediction of functional microRNA targets. Nucleic Acids Res. 48, D127–D131. doi: 10.1093/nar/gkz757
Chin, C. H., Chen, S. H., Wu, H. H., Ho, C. W., Ko, M. T., and Lin, C. Y. (2014). CytoHubba: identifying hub objects and sub-networks from complex interactome. BMC Syst. Biol. 8, (Suppl. 4):S11. doi: 10.1186/1752-0509-8-S4-S11
Conti, C. L., and Nakamura-Palacios, E. M. (2014). Bilateral transcranial direct current stimulation over dorsolateral prefrontal cortex changes the drug-cued reactivity in the anterior cingulate cortex of crack-cocaine addicts. Brain Stimul. 7, 130–132. doi: 10.1016/j.brs.2013.09.007
Crandall, C. G., Vongpatanasin, W., and Victor, R. G. (2002). Mechanism of cocaine-induced hyperthermia in humans. Ann. Intern. Med. 136, 785–791. doi: 10.7326/0003-4819-136-11-200206040-00006
Cui, X., Niu, W., Kong, L., He, M., Jiang, K., Chen, S., et al. (2016). Hsa_circRNA_103636: potential novel diagnostic and therapeutic biomarker in Major depressive disorder. Biomark Med. 10, 943–952. doi: 10.2217/bmm-2016-0130
Doncheva, N. T., Morris, J. H., Gorodkin, J., and Jensen, L. J. (2019). Cytoscape stringapp: network analysis and visualization of proteomics data. J. Proteome Res. 18, 623–632. doi: 10.1021/acs.jproteome.8b00702
Du, W. W., Yang, W., Chen, Y., Wu, Z. K., Foster, F. S., Yang, Z., et al. (2017). Foxo3 circular RNA promotes cardiac senescence by modulating multiple factors associated with stress and senescence responses. Eur. Heart J. 38, 1402–1412. doi: 10.1093/eurheartj/ehw001
Du, W. W., Yang, W., Liu, E., Yang, Z., Dhaliwal, P., and Yang, B. B. (2016). Foxo3 circular RNA retards cell cycle progression via forming ternary complexes with p21 and CDK2. Nucleic Acids Res. 44, 2846–2858. doi: 10.1093/nar/gkw027
Ellenbroek, B. A. (2013). Histamine H(3) receptors, the complex interaction with dopamine and its implications for addiction. Br. J. Pharmacol. 170, 46–57. doi: 10.1111/bph.12221
Enuka, Y., Lauriola, M., Feldman, M. E., Sas-Chen, A., Ulitsky, I., and Yarden, Y. (2016). Circular RNAs are long-lived and display only minimal early alterations in response to a growth factor. Nucleic Acids Res. 44, 1370–1383. doi: 10.1093/nar/gkv1367
Esseltine, J. L., Ribeiro, F. M., and Ferguson, S. S. (2012). Rab8 modulates metabotropic glutamate receptor subtype 1 intracellular trafficking and signaling in a protein kinase C-dependent manner. J. Neurosci. 32, 16933a–16942a. doi: 10.1523/JNEUROSCI.0625-12.2012
Feng, J., Chen, W., Dong, X., Wang, J., Mei, X., Deng, J., et al. (2021). CSCD2: an integrated interactional database of cancer-specific circular RNAs. Nucleic Acids Res. 50, D1179–D1183. doi: 10.1093/nar/gkab830
Fromm, B., Billipp, T., Peck, L. E., Johansen, M., Tarver, J. E., King, B. L., et al. (2015). A uniform system for the annotation of vertebrate microRNA genes and the evolution of the human microRNAome. Annu. Rev. Genet. 49, 213–242. doi: 10.1146/annurev-genet-120213-092023
Gao, Y., Zhang, J., and Zhao, F. (2018). Circular RNA identification based on multiple seed matching. Brief Bioinform. 19, 803–810. doi: 10.1093/bib/bbx014
García-Fuster, M. J., Flagel, S. B., Mahmood, S. T., Watson, S. J., and Akil, H. (2012). Cocaine withdrawal causes delayed dysregulation of stress genes in the hippocampus. PLoS One 7:e42092. doi: 10.1371/journal.pone.0042092
Gass, J. T., and Chandler, L. J. (2013). The plasticity of extinction: contribution of the prefrontal cortex in treating addiction through inhibitory learning. Front. Psychiatry 4:46. doi: 10.3389/fpsyt.2013.00046
Gawin, F. H., and Ellinwood, E. H. Jr. (1989). Cocaine dependence. Annu. Rev. Med. 40, 149–161. doi: 10.1146/annurev.me.40.020189.001053
Glažar, P., Papavasileiou, P., and Rajewsky, N. (2014). Circbase: a database for circular RNAs. RNA 20, 1666–1670. doi: 10.1261/rna.043687.113
Greene, J., Baird, A. M., Brady, L., Lim, M., Gray, S. G., McDermott, R., et al. (2017). Circular RNAs: biogenesis, function and role in human diseases. Front. Mol. Biosci. 4:Artn38. doi: 10.3389/Fmolb.2017.00038
Gu, W. J., Zhang, C., Zhong, Y., Luo, J., Zhang, C. Y., Zhang, C., et al. (2020). Altered serum microRNA expression profile in subjects with heroin and methamphetamine use disorder. Biomed. Pharmacother. 125:109918. doi: 10.1016/j.biopha.2020.109918
Guo, M. L., Liao, K., Periyasamy, P., Yang, L., Cai, Y., Callen, S. E., et al. (2015). Cocaine-mediated microglial activation involves the ER stress-autophagy axis. Autophagy 11, 995–1009. doi: 10.1080/15548627.2015.1052205
Han, J. D., Bertin, N., Hao, T., Goldberg, D. S., Berriz, G. F., Zhang, L. V., et al. (2004). Evidence for dynamically organized modularity in the yeast protein-protein interaction network. Nature 430, 88–93. doi: 10.1038/nature02555
Hansen, T. B., Jensen, T. I., Clausen, B. H., Bramsen, J. B., Finsen, B., Damgaard, C. K., et al. (2013). Natural RNA circles function as efficient microRNA sponges. Nature 495, 384–388. doi: 10.1038/nature11993
Harraz, M. M., Guha, P., Kang, I. G., Semenza, E. R., Malla, A. P., Song, Y. J., et al. (2021). Cocaine-induced locomotor stimulation involves autophagic degradation of the dopamine transporter. Mol. Psychiatry 26, 370–382. doi: 10.1038/s41380-020-00978-y
Heal, D. J., Smith, S. L., and Henningfield, J. E. (2014). CNS stimulants. Neuropharmacology 87, 1–3. doi: 10.1016/j.neuropharm.2014.09.025
Holdt, L. M., Stahringer, A., Sass, K., Pichler, G., Kulak, N. A., Wilfert, W., et al. (2016). Circular non-coding RNA ANRIL modulates ribosomal RNA maturation and atherosclerosis in humans. Nat. Commun. 7:12429. doi: 10.1038/ncomms12429
Howell, L. L., and Cunningham, K. A. (2015). Serotonin 5-HT2 receptor interactions with dopamine function: implications for therapeutics in cocaine use disorder. Pharmacol. Rev. 67, 176–197. doi: 10.1124/pr.114.009514
Huang, R., Zhang, Y., Bai, Y., Han, B., Ju, M., Chen, B., et al. (2020). N(6)-methyladenosine modification of fatty acid amide hydrolase messenger RNA in circular RNA STAG1-Regulated astrocyte dysfunction and depressive-like behaviors. Biol. Psychiatry 88, 392–404. doi: 10.1016/j.biopsych.2020.02.018
Huang, R., Zhang, Y., Han, B., Bai, Y., Zhou, R., Gan, G., et al. (2017). Circular RNA HIPK2 regulates astrocyte activation via cooperation of autophagy and ER stress by targeting MIR124-2HG. Autophagy 13, 1722–1741. doi: 10.1080/15548627.2017.1356975
Jaenisch, R., and Bird, A. (2003). Epigenetic regulation of gene expression: how the genome integrates intrinsic and environmental signals. Nat. Genet. 33, (Suppl.) 245–254. doi: 10.1038/ng1089
Johnson, M. M., David, J. A., Michelhaugh, S. K., Schmidt, C. J., and Bannon, M. J. (2012). Increased heat shock protein 70 gene expression in the brains of cocaine-related fatalities may be reflective of postdrug survival and intervention rather than excited delirium. J. Forensic Sci. 57, 1519–1523. doi: 10.1111/j.1556-4029.2012.02212.x
Jordão, M. J. C., Sankowski, R., Brendecke, S. M., Sagar, I., Locatelli, G., Tai, Y. H., et al. (2019). Single-cell profiling identifies myeloid cell subsets with distinct fates during neuroinflammation. Science 363:eaat7554. doi: 10.1126/science.aat7554
Khibnik, L. A., Beaumont, M., Doyle, M., Heshmati, M., Slesinger, P. A., Nestler, E. J., et al. (2016). Stress and cocaine trigger divergent and cell type-specific regulation of synaptic transmission at single spines in nucleus accumbens. Biol. Psychiatry 79, 898–905. doi: 10.1016/j.biopsych.2015.05.022
Kleaveland, B., Shi, C. Y., Stefano, J., and Bartel, D. P. (2018). A network of noncoding regulatory RNAs acts in the mammalian brain. Cell 174, 350.e–362.e. doi: 10.1016/j.cell.2018.05.022
Kumar, S., Matthews, Q. L., and Sims, B. (2020). Effects of cocaine on human glial-derived extracellular vesicles. Front. Cell Dev. Biol. 8:563441. doi: 10.3389/fcell.2020.563441
Langmead, B., and Salzberg, S. L. (2012). Fast gapped-read alignment with Bowtie 2. Nat. Methods 9, 357–359. doi: 10.1038/nmeth.1923
Legnini, I., Di Timoteo, G., Rossi, F., Morlando, M., Briganti, F., Sthandier, O., et al. (2017). Circ-ZNF609 is a circular RNA that can be translated and functions in myogenesis. Mol. Cell 66, 22.e9–39.e9. doi: 10.1016/j.molcel.2017.02.017
Li, J., Shi, Q., Wang, Q., Tan, X., Pang, K., Liu, X., et al. (2019). Profiling circular RNA in methamphetamine-treated primary cortical neurons identified novel circRNAs related to methamphetamine addiction. Neurosci. Lett. 701, 146–153. doi: 10.1016/j.neulet.2019.02.032
Li, J., Sun, Q., Zhu, S., Xi, K., Shi, Q., Pang, K., et al. (2020). Knockdown of circHomer1 ameliorates METH-induced neuronal injury through inhibiting Bbc3 expression. Neurosci. Lett. 732:135050. doi: 10.1016/j.neulet.2020.135050
Li, M., Xu, P., Xu, Y., Teng, H., Tian, W., Du, Q., et al. (2017). Dynamic expression changes in the transcriptome of the prefrontal cortex after repeated exposure to cocaine in mice. Front. Pharmacol. 8:142. doi: 10.3389/fphar.2017.00142
Li, X., Yang, L., and Chen, L. L. (2018). The biogenesis, functions, and challenges of circular RNAs. Mol. Cell 71, 428–442. doi: 10.1016/j.molcel.2018.06.034
Li, Y., Simmler, L. D., Van Zessen, R., Flakowski, J., Wan, J. X., Deng, F., et al. (2021). Synaptic mechanism underlying serotonin modulation of transition to cocaine addiction. Science 373, 1252–1256. doi: 10.1126/science.abi9086
Li, Z., Huang, C., Bao, C., Chen, L., Lin, M., Wang, X., et al. (2015). Exon-intron circular RNAs regulate transcription in the nucleus. Nat. Struct. Mol. Biol. 22, 256–264. doi: 10.1038/nsmb.2959
Liao, K., Guo, M., Niu, F., Yang, L., Callen, S. E., and Buch, S. (2016). Cocaine-mediated induction of microglial activation involves the ER stress-TLR2 axis. J. Neuroinflammation 13:33. doi: 10.1186/s12974-016-0501-2
Liu, W., and Wang, X. (2019). Prediction of functional microRNA targets by integrative modeling of microRNA binding and target expression data. Genome Biol. 20:18. doi: 10.1186/s13059-019-1629-z
Liu, Z., Ran, Y., Tao, C., Li, S., Chen, J., and Yang, E. (2019). Detection of circular RNA expression and related quantitative trait loci in the human dorsolateral prefrontal cortex. Genome Biol. 20:99. doi: 10.1186/s13059-019-1701-8
Lv, C., Sun, L., Guo, Z., Li, H., Kong, D., Xu, B., et al. (2018). Circular RNA regulatory network reveals cell-cell crosstalk in acute myeloid leukemia extramedullary infiltration. J. Transl. Med. 16:361. doi: 10.1186/s12967-018-1726-x
Mahmoudi, E., Fitzsimmons, C., Geaghan, M. P., Shannon Weickert, C., Atkins, J. R., Wang, X., et al. (2019). Circular RNA biogenesis is decreased in postmortem cortical gray matter in schizophrenia and may alter the bioavailability of associated miRNA. Neuropsychopharmacology 44, 1043–1054. doi: 10.1038/s41386-019-0348-1
Majewska, M. D. (1996b). Neurotoxicity and neuropathology associated with chronic cocaine abuse. NIDA Res. Monogr. 162, 70–72.
Majewska, M. D. (1996a). Cocaine addiction as a neurological disorder: implications for treatment. NIDA Res. Monogr. 163, 1–26.
Martin, M. (2011). Cutadapt removes adapter sequences from high-throughput sequencing reads. Embnet J. 17:3. doi: 10.14806/ej.17.1.200
Martínez-Rivera, A., Hao, J., Tropea, T. F., Giordano, T. P., Kosovsky, M., Rice, R. C., et al. (2017). Enhancing VTA Cav1.3 L-type Ca(2+) channel activity promotes cocaine and mood-related behaviors via overlapping AMPA receptor mechanisms in the nucleus accumbens. Mol. Psychiatry 22, 1735–1745. doi: 10.1038/mp.2017.9
Mash, D. C., Duque, L., Pablo, J., Qin, Y., Adi, N., Hearn, W. L., et al. (2009). Brain biomarkers for identifying excited delirium as a cause of sudden death. Forensic Sci. Int. 190, e13–e19. doi: 10.1016/j.forsciint.2009.05.012
Matochik, J. A., London, E. D., Eldreth, D. A., Cadet, J. L., and Bolla, K. I. (2003). Frontal cortical tissue composition in abstinent cocaine abusers: a magnetic resonance imaging study. Neuroimage 19, 1095–1102. doi: 10.1016/s1053-8119(03)00244-1
Mehta, S. L., Dempsey, R. J., and Vemuganti, R. (2020). Role of circular RNAs in brain development and CNS diseases. Prog. Neurobiol. 186:101746. doi: 10.1016/j.pneurobio.2020.101746
Memczak, S., Jens, M., Elefsinioti, A., Torti, F., Krueger, J., Rybak, A., et al. (2013). Circular RNAs are a large class of animal RNAs with regulatory potency. Nature 495, 333–338. doi: 10.1038/nature11928
Moeller, S. J., Frobose, M. I., Konova, A. B., Misyrlis, M., Parvaz, M. A., Goldstein, R. Z., et al. (2014). Common and distinct neural correlates of inhibitory dysregulation: stroop fMRI study of cocaine addiction and intermittent explosive disorder. J. Psychiatr. Res. 58, 55–62. doi: 10.1016/j.jpsychires.2014.07.016
Moreno-Lopez, L., Catena, A., Fernandez-Serrano, M. J., Delgado-Rico, E., Stamatakis, E. A., Perez-Garcia, M., et al. (2012). Trait impulsivity and prefrontal gray matter reductions in cocaine dependent individuals. Drug Alcohol Depend. 125, 208–214. doi: 10.1016/j.drugalcdep.2012.02.012
Nestler, E. J. (2014). Epigenetic mechanisms of drug addiction. Neuropharmacology 76 Pt B, 259–268. doi: 10.1016/j.neuropharm.2013.04.004
Nestler, E. J., and Lüscher, C. (2019). The molecular basis of drug addiction: linking epigenetic to synaptic and circuit mechanisms. Neuron 102, 48–59. doi: 10.1016/j.neuron.2019.01.016
Nüchel, J., Ghatak, S., Zuk, A. V., Illerhaus, A., Morgelin, M., Schonborn, K., et al. (2018). TGFB1 is secreted through an unconventional pathway dependent on the autophagic machinery and cytoskeletal regulators. Autophagy 14, 465–486. doi: 10.1080/15548627.2017.1422850
Núñez, E., Perez-Siles, G., Rodenstein, L., Alonso-Torres, P., Zafra, F., Jimenez, E., et al. (2009). Subcellular localization of the neuronal glycine transporter GLYT2 in brainstem. Traffic 10, 829–843. doi: 10.1111/j.1600-0854.2009.00911.x
Pamudurti, N. R., Bartok, O., Jens, M., Ashwal-Fluss, R., Stottmeister, C., Ruhe, L., et al. (2017). Translation of circRNAs. Mol. Cell 66, 9–21. doi: 10.1016/j.molcel.2017.02.021
Paraskevopoulou, M. D., Georgakilas, G., Kostoulas, N., Vlachos, I. S., Vergoulis, T., Reczko, M., et al. (2013). DIANA-microT web server v5.0: service integration into miRNA functional analysis workflows. Nucleic Acids Res. 41, W169–W173. doi: 10.1093/nar/gkt393
Pascale, E., Beclin, C., Fiorenzano, A., Andolfi, G., Erni, A., De Falco, S., et al. (2020). Long non-coding RNA T-UCstem1 controls progenitor proliferation and neurogenesis in the postnatal mouse olfactory bulb through interaction with miR-9. Stem Cell Rep. 15, 836–844. doi: 10.1016/j.stemcr.2020.08.009
Periyasamy, P., Guo, M. L., and Buch, S. (2016). Cocaine induces astrocytosis through ER stress-mediated activation of autophagy. Autophagy 12, 1310–1329. doi: 10.1080/15548627.2016.1183844
Piwecka, M., Glazar, P., Hernandez-Miranda, L. R., Memczak, S., Wolf, S. A., Rybak-Wolf, A., et al. (2017). Loss of a mammalian circular RNA locus causes miRNA deregulation and affects brain function. Science 357:eaam8526. doi: 10.1126/science.aam8526
Ransohoff, R. M., and Cardona, A. E. (2010). The myeloid cells of the central nervous system parenchyma. Nature 468, 253–262. doi: 10.1038/nature09615
Reid, A. G., Lingford-Hughes, A. R., Cancela, L. M., and Kalivas, P. W. (2012). Substance abuse disorders. Handb. Clin. Neurol. 106, 419–431. doi: 10.1016/B978-0-444-52002-9.00024-3
Ribeiro, E. A., Scarpa, J. R., Garamszegi, S. P., Kasarskis, A., Mash, D. C., and Nestler, E. J. (2017). Gene network dysregulation in dorsolateral prefrontal cortex neurons of humans with cocaine use disorder. Sci. Rep. 7:5412. doi: 10.1038/s41598-017-05720-3
Robinson, M. D., McCarthy, D. J., and Smyth, G. K. (2010). EdgeR: a Bioconductor package for differential expression analysis of digital gene expression data. Bioinformatics 26, 139–140. doi: 10.1093/bioinformatics/btp616
Robison, A. J., and Nestler, E. J. (2011). Transcriptional and epigenetic mechanisms of addiction. Nat. Rev. Neurosci. 12, 623–637. doi: 10.1038/nrn3111
Roy, D. S., Zhang, Y., Aida, T., Choi, S., Chen, Q., Hou, Y., et al. (2021). Anterior thalamic dysfunction underlies cognitive deficits in a subset of neuropsychiatric disease models. Neuron 109, 2590.e13–2603.e13. doi: 10.1016/j.neuron.2021.06.005
Rybak-Wolf, A., Stottmeister, C., Glazar, P., Jens, M., Pino, N., Giusti, S., et al. (2015). Circular RNAs in the mammalian brain are highly abundant, conserved, and dynamically expressed. Mol. Cell 58, 870–885. doi: 10.1016/j.molcel.2015.03.027
Salminen, W. F. Jr., Roberts, S. M., Fenna, M., and Voellmy, R. (1997). Heat shock protein induction in murine liver after acute treatment with cocaine. Hepatology 25, 1147–1153. doi: 10.1002/hep.510250517
Seamans, J. K., Lapish, C. C., and Durstewitz, D. (2008). Comparing the prefrontal cortex of rats and primates: insights from electrophysiology. Neurotox. Res. 14, 249–262. doi: 10.1007/BF03033814
Sellier, C., Campanari, M. L., Julie Corbier, C., Gaucherot, A., Kolb-Cheynel, I., Oulad-Abdelghani, M., et al. (2016). Loss of C9ORF72 impairs autophagy and synergizes with polyQ Ataxin-2 to induce motor neuron dysfunction and cell death. EMBO J. 35, 1276–1297. doi: 10.15252/embj.201593350
Shannon, P., Markiel, A., Ozier, O., Baliga, N. S., Wang, J. T., Ramage, D., et al. (2003). Cytoscape: a software environment for integrated models of biomolecular interaction networks. Genome Res. 13, 2498–2504. doi: 10.1101/gr.1239303
Sharma, H. S., Muresanu, D., Sharma, A., and Patnaik, R. (2009). Cocaine-induced breakdown of the blood-brain barrier and neurotoxicity. Int. Rev. Neurobiol. 88, 297–334. doi: 10.1016/S0074-7742(09)88011-2
Smaga, I., Sanak, M., and Filip, M. (2019). Cocaine-induced changes in the expression of NMDA receptor subunits. Curr. Neuropharmacol. 17, 1039–1055. doi: 10.2174/1570159X17666190617101726
Szabo, L., and Salzman, J. (2016). Detecting circular RNAs: bioinformatic and experimental challenges. Nat. Rev. Genet. 17, 679–692. doi: 10.1038/nrg.2016.114
Szklarczyk, D., Morris, J. H., Cook, H., Kuhn, M., Wyder, S., Simonovic, M., et al. (2017). The STRING database in 2017: quality-controlled protein-protein association networks, made broadly accessible. Nucleic Acids Res. 45, D362–D368. doi: 10.1093/nar/gkw937
Terraneo, A., Leggio, L., Saladini, M., Ermani, M., Bonci, A., and Gallimberti, L. (2016). Transcranial magnetic stimulation of dorsolateral prefrontal cortex reduces cocaine use: a pilot study. Eur. Neuropsychopharmacol. 26, 37–44. doi: 10.1016/j.euroneuro.2015.11.011
United Nations Office on Drugs and Crime (2020). UNODC World Drug Report 2020: Global drug use rising; while COVID-19 has far reaching impact on global drug markets. Vienna: United Nations Office on Drugs and Crime.
Vergoulis, T., Vlachos, I. S., Alexiou, P., Georgakilas, G., Maragkakis, M., Reczko, M., et al. (2012). TarBase 6.0: capturing the exponential growth of miRNA targets with experimental support. Nucleic Acids Res. 40, D222–D229. doi: 10.1093/nar/gkr1161
Vicens, Q., and Westhof, E. (2014). Biogenesis of circular RNAs. Cell 159, 13–14. doi: 10.1016/j.cell.2014.09.005
Vlachos, I. S., and Hatzigeorgiou, A. G. (2017). Functional analysis of miRNAs using the DIANA Tools online suite. Methods Mol. Biol. 1517, 25–50. doi: 10.1007/978-1-4939-6563-2_2
Wang, J., Li, K. L., Shukla, A., Beroun, A., Ishikawa, M., Huang, X., et al. (2021). Cocaine triggers astrocyte-mediated synaptogenesis. Biol. Psychiatry 89, 386–397. doi: 10.1016/j.biopsych.2020.08.012
Wang, Q., Cai, J., Fang, C., Yang, C., Zhou, J., Tan, Y., et al. (2018). Mesenchymal glioblastoma constitutes a major ceRNA signature in the TGF-beta pathway. Theranostics 8, 4733–4749. doi: 10.7150/thno.26550
Yu, H., Xie, B., Zhang, J., Luo, Y., Galaj, E., Zhang, X., et al. (2021). The role of circTmeff-1 in incubation of context-induced morphine craving. Pharmacol. Res. 170:105722. doi: 10.1016/j.phrs.2021.105722
Zhang, H., Chen, Z., Zhong, Z., Gong, W., and Li, J. (2018). Total saponins from the leaves of Panax notoginseng inhibit depression on mouse chronic unpredictable mild stress model by regulating circRNA expression. Brain Behav. 8:e01127. doi: 10.1002/brb3.1127
Zhang, H., Wang, Q., Wang, Q., Liu, A., Qin, F., Sun, Q., et al. (2020). Circular RNA expression profiling in the nucleus accumbens: effects of electroacupuncture treatment on morphine-induced conditioned place preference. Addict Biol. 25:e12794. doi: 10.1111/adb.12794
Zhang, Y., Du, L., Bai, Y., Han, B., He, C., Gong, L., et al. (2020). CircDYM ameliorates depressive-like behavior by targeting miR-9 to regulate microglial activation via HSP90 ubiquitination. Mol. Psychiatry 25, 1175–1190. doi: 10.1038/s41380-018-0285-0
Zhou, Y., Zhou, B., Pache, L., Chang, M., Khodabakhshi, A. H., Tanaseichuk, O., et al. (2019). Metascape provides a biologist-oriented resource for the analysis of systems-level datasets. Nat. Commun. 10:1523. doi: 10.1038/s41467-019-09234-6
Zimmerman, A. J., Hafez, A. K., Amoah, S. K., Rodriguez, B. A., Dell’Orco, M., Lozano, E., et al. (2020). A psychiatric disease-related circular RNA controls synaptic gene expression and cognition. Mol. Psychiatry 25, 2712–2727. doi: 10.1038/s41380-020-0653-4
Keywords: cocaine use disorder, circRNAs, ceRNAs regulatory network, protein–protein interaction network, hub genes, integrated bioinformatics analysis
Citation: Chen Y, Li X, Meng S, Huang S, Chang S and Shi J (2022) Identification of Functional CircRNA–miRNA–mRNA Regulatory Network in Dorsolateral Prefrontal Cortex Neurons of Patients With Cocaine Use Disorder. Front. Mol. Neurosci. 15:839233. doi: 10.3389/fnmol.2022.839233
Received: 19 December 2021; Accepted: 01 March 2022;
Published: 14 April 2022.
Edited by:
Jianfeng Liu, Texas A&M University, United StatesReviewed by:
Min Zhao, Shanghai Changning Mental Health Center, ChinaBiao Yan, Fudan University, China
Copyright © 2022 Chen, Li, Meng, Huang, Chang and Shi. This is an open-access article distributed under the terms of the Creative Commons Attribution License (CC BY). The use, distribution or reproduction in other forums is permitted, provided the original author(s) and the copyright owner(s) are credited and that the original publication in this journal is cited, in accordance with accepted academic practice. No use, distribution or reproduction is permitted which does not comply with these terms.
*Correspondence: Jie Shi, c2hpamllQGJqbXUuZWR1LmNu; Suhua Chang, Y2hhbmdzaEBiam11LmVkdS5jbg==