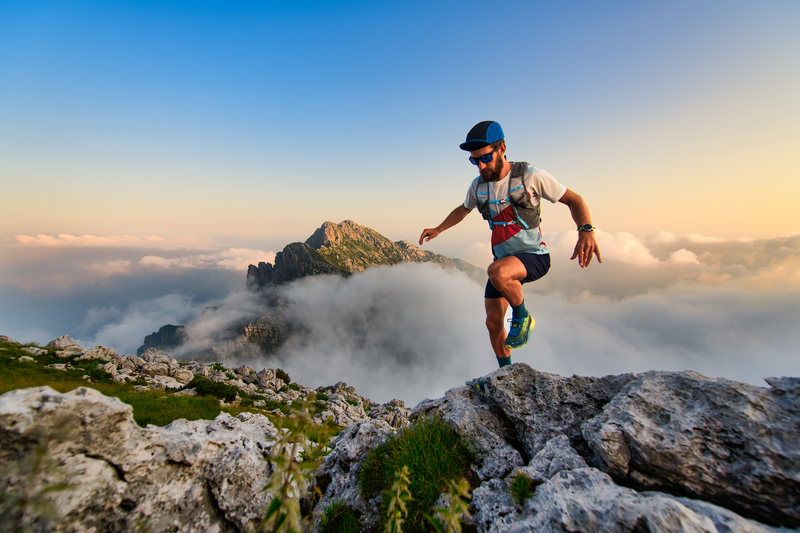
94% of researchers rate our articles as excellent or good
Learn more about the work of our research integrity team to safeguard the quality of each article we publish.
Find out more
ORIGINAL RESEARCH article
Front. Mol. Neurosci. , 10 February 2021
Sec. Brain Disease Mechanisms
Volume 14 - 2021 | https://doi.org/10.3389/fnmol.2021.619496
This article is part of the Research Topic The Biochemistry of Amyloids in Neurodegenerative Diseases, Volume I View all 11 articles
Besides amyloid fibrils, amyloid pores (APs) represent another mechanism of amyloid induced toxicity. Since hypothesis put forward by Arispe and collegues in 1993 that amyloid-beta makes ion-conducting channels and that Alzheimer's disease may be due to the toxic effect of these channels, many studies have confirmed that APs are formed by prefibrillar oligomers of amyloidogenic proteins and are a common source of cytotoxicity. The mechanism of pore formation is still not well-understood and the structure and imaging of APs in living cells remains an open issue. To get closer to understand AP formation we used predictive methods to assess the propensity of a set of 30 amyloid-forming proteins (AFPs) to form transmembrane channels. A range of amino-acid sequence tools were applied to predict AP domains of AFPs, and provided context on future experiments that are needed in order to contribute toward a deeper understanding of amyloid toxicity. In a set of 30 AFPs we predicted their amyloidogenic propensity, presence of transmembrane (TM) regions, and cholesterol (CBM) and ganglioside binding motifs (GBM), to which the oligomers likely bind. Noteworthy, all pathological AFPs share the presence of TM, CBM, and GBM regions, whereas the functional amyloids seem to show just one of these regions. For comparative purposes, we also analyzed a few examples of amyloid proteins that behave as biologically non-relevant AFPs. Based on the known experimental data on the β-amyloid and α-synuclein pore formation, we suggest that many AFPs have the potential for pore formation. Oligomerization and α-TM helix to β-TM strands transition on lipid rafts seem to be the common key events.
It is widely accepted and inherited cases confirm a notion that the major part of the pathology of neurodegenerative diseases is due to aberrant processes of protein misfolding and formation of amyloid fibrils by the amyloidogenic proteins concerned: α-synuclein in Parkinson's disease, β-amyloid (Aβ) in Alzheimer's disease, SOD1 and TDP-43 in amyotrophic lateral sclerosis, etc. Dobson (2002) discovered that these conformational transitions are not reserved to amyloidogenic proteins, but that under certain conditions all proteins can be converted into amyloid fibrils, even the very stable and α-helical myoglobin (Fandrich et al., 2001). However, the tendency to misfold and aggregate to amyloid at physiological pH and temperature is not the same for all proteins; certain proteins or their parts—after cleavage—are more susceptible to the formation of amyloid fibrils. Amyloidogenic proteins do not have common sequence motifs, but by comparing the protein sequences it can be predicted that some parts are hot spots that form a cross-β spine of amyloid-like fibrils (Nelson et al., 2005). The peptides, which are as short as hexapeptides, can form amyloid fibrils (Tenidis et al., 2000). From the molecular forces that determine the cross-β structure of the amyloid, the main chain hydrogen bonds, but also aromatic repetitive patterns (Gazit, 2007) seem to be of great importance, the latter probably undergoing Π stacking (Gazit, 2002, 2007; Reymer et al., 2014). The secondary structure in the native fold protein is important, but not directly correlated with the secondary structure of the amyloid fibrils. The over-prediction of α-helices compared to the X-ray structure derived α-helices indicates the propensity of α to β transition in the intermediate (Morillas et al., 2001), partially unfolded state and, for intrinsically disordered proteins, partially folded state.
The transition to amyloid fibrils is a reaction consisting of a lag, growth and plateau phases. The most common mechanism is nucleation via an oligomeric nucleus and the other spectrum is downhill polymerization (Žerovnik et al., 2011; Dovidchenko et al., 2014). In due course of amyloid fibrils formation the prefibrillar oligomers of different shapes can be formed; from rings as found at Aβ (Oxana, 2019), to globules, spheres, or stars. Some of these prefibrillar oligomers are on-pathway and determine the nucleus that assigns the lag phase, some are off-pathway. Some are benign some are toxic differing by subtle changes in conformation (Capitini et al., 2018; Sengupta and Udgaonkar, 2018). The toxic prefibrillar oligomers (Bucciantini et al., 2002; Leri et al., 2016) are thought to make pores into membranes, similar to antimicrobial peptides or bacterial toxins (Anderluh and Žerovnik, 2012; Last and Miranker, 2013). The “channel hypothesis” of AD is not new. It is based on electrophysiological measurements by the group of Arispe et al. (1993a,b); Arispe et al. (2014), Kawahara et al. (2000), and Diaz et al. (2009). Later, the same concept was increased to other amyloid proteins, among them α-synuclein (α-syn), by Lashuel et al. (2002) and Lashuel and Lansbury (2006). Amyloid pore (AP) formation (Kawahara et al., 2011; Di Scala et al., 2016; Kandel et al., 2017) is still not fully understood and has not been directly proven in living cells until recently (Jamasbi et al., 2018). Various in vitro studies on membrane vesicles, artificial lipid bilayers, and neuronal cell cultures were performed for Aβ and α-syn (Kawahara et al., 2000; Tsigelny et al., 2012; Chen et al., 2016; Di Scala et al., 2016; Kandel et al., 2017; Hannestad et al., 2020; Perissinotto et al., 2020). Recent, still in vitro study revealed the imaging of how α-syn forms the AP in membrane predominantly composed of anionic phospholipids, alike those making mitochondrial membranes. Since the interaction of neuronal α-syn with lipid membranes appears crucial in the context of Parkinson's disease, authors tried to explain the roles of different lipids in pathogenic protein aggregation and membrane disruption (Hannestad et al., 2020). Perissinotto et al. (2020) showed that metals (iron in particular) influence interaction of α-syn with lipid rafts. Kayed et al. (2020) has written a review on existence of endogenous oligomeric and multimeric species in α-synucleopathies. The association of α-syn with plasma membrane of hippocampal neurons was demonstrated to induce the formation of pore-like structures (Li et al., 2020). The analysis of Lee et al. (2017) has shown structure and conductance of oligomeric Aβ pores in a natural lipid membrane, which closely mimics the in vivo cellular environment. Recent studies also include interaction of Aβ with cellular membranes (Bode et al., 2017) and animal models (Julien et al., 2018), both confirming the hypothesis of membrane perforation. For example, in C. elegans the membrane repair response was turned on when Aβ was fed to animals (Julien et al., 2018).
Moreover, in last years the researchers have elucidated the X-ray crystallographic structures of oligomers derived from Aβ, α-synuclein, and β2-microglobulin (Kreutzer and Nowick, 2018). Out of these three amyloidogenic peptides/proteins, the Aβ β-hairpin mimics have provided the most insight into amyloid oligomers. Study has revealed a new mode of self-assembly, where three Aβ β-hairpin mimics assemble to form a triangular trimer which can pack together with other triangular trimers to form higher-order oligomers (hexamers and dodecamers). These higher-order oligomers can form annular pore-like assemblies and exhibit toxicity toward neuronally derived cells (Kreutzer and Nowick, 2018). Specific pore-forming β-barrel oligomers of Aβ42 in DPC micelle conditions were reported also by Serra-Batiste et al. (2016). Recently, another atomic level structures of β-sheet pore-forming Aβ(1-42) oligomers were obtained by nuclear magnetic resonance (NMR) and mass spectrometry (MS), and a mechanism for membrane disruption based on electrophysiology and simulation studies in membranes was provided (Ciudad et al., 2020). These structural findings are significant and address the gap in understanding the molecular basis of amyloid diseases.
Various methods have been developed to calculate the propensity to form amyloid fibrils, such as AGGRESCAN, AGGRESCAN3D, TANGO, WALTZ, etc. The method AGGRESCAN3D (Pujols et al., 2018) takes into account the tertiary structure of proteins apart from their sequence. The overview of the available programs is described in the review paper of Pallarés and Ventura (2019). A preliminary screening of amyloidogenic sequence fragments can be performed with the RFAmy predictor (http://server.malab.cn/RFAmyloid) (Niu et al., 2018) and the AmyPro database (https://amypro.net) (Varadi et al., 2018). This database includes pathogenic amyloids as well as prions and functional amyloids, and allows users to screen their sequences against the entire collection of validated amyloidogenic sequence fragments. Further, AmyPred2 (Tsolis et al., 2013) (http://aias.biol.uoa.gr/AMYLPRED2) shows a CONSENSUS result of many methods. Previously, this program was successfully used to predict amyloid-prone regions in human stefin B wild-type and proline mutants (Hasanbasic et al., 2019). In this study a set of 30 potentially AFPs was selected and the amyloid-fibril propensity was calculated using various tools (Table 1).
In the interaction of prefibrillar oligomers with phospholipid membranes, the lipid rafts, i.e., the microdomains of membranes rich in gangliosides and cholesterol, play an important role (Jang et al., 2009, 2013; Di Scala et al., 2016; Kandel et al., 2019). There are some parallels to the entry of virus particles (Yahi and Fantini, 2014). For example, the spike protein of the coronavirus SARS-CoV-2 in the S2-part has a motif that binds to lipid rafts and thus enables the S1-part to attach and interact with the ACE2-receptor (Fantini et al., 2020). Of interest, the SARS coronavirus-(SARS CoV-1) protein E (E for envelope) was shown to form cation-selective membrane channels (Wilson et al., 2004; Verdiá-Báguena et al., 2012). The SARS-CoV-2 protein E thus likely functions as a “viroporin,” but also may have an important function in the infection process and subsequent inflammation (Pacheco et al., 2015).
Therefore, the main focus of our study was to determine in the set of 30 AFPs the domains that could be crucial for AP formation [TM regions, ganglioside (GBM) and cholesterol binding motifs (CBM)]. In this regard, several publicly available tools were used to assess whether the proteins under investigation have TM regions, either α-helices or β-strands. Detailed description and list of these tools are available in section Materials and Methods and Supplementary Table 1. Indeed, we were able to determine possible TM regions in some of the amyloidogenic proteins involved in neurodegenerative pathology. For others, we suspect that they can still form TM channels when in the oligomeric state. Further on, in the same set of sequences we looked for the motifs that represent signatures for the binding to gangliosides and cholesterol, GBM and CBM, respectively. Lipid rafts are rich in cholesterol and gangliosides (Figure 1) (Sezgin et al., 2017), and both are the sites where membrane interaction often begins; as seen in viruses (Wilson et al., 2004; Verdiá-Báguena et al., 2012) or in the direct pore formation through APs (Di Scala et al., 2016). The association of oligomeric α-synuclein with plasma membrane of hippocampal neurons was demonstrated to induce the formation of pore-like structures (Li et al., 2020). Furthermore, the results of Pacheco et al. (2015) go in line with the data of β-amyloid, another experimentally confirmed amyloidogenic pore forming peptide (Sepúlveda et al., 2014). Models of rather mobile Aβ channels have been proposed already in 2007 by the Nussinov group, who used molecular dynamics simulations (Jang et al., 2007, 2009, 2016; Capone et al., 2012). The simulations indicated that β-sheet channels might break into loosely associated mobile β-sheet subunits. The preferred channel sizes (16- to 24-mer) were compatible with electron microscopy/atomic force microscopy-derived dimensions (Jang et al., 2009).
Further in vitro experiments have shown that AP formation involves both membrane lipids, ganglioside and cholesterol, that physically interact with amyloid proteins through specific structural motifs (GBM and CBM) (Jang et al., 2007, 2009, 2013; Di Scala et al., 2016; Dong et al., 2017). Mutation or deletion of these motifs abolished pore formation in α-synuclein (Parkinson's disease) and Aβ (Alzheimer's disease). Moreover, both peptides did no longer form Ca2+-permeable pores in the presence of drugs that target either cholesterol or ganglioside or both membrane lipids, indicating that gangliosides and cholesterol cooperate to favor the formation of AP through a common molecular mechanism (Di Scala et al., 2016). Figure 2 highlights the α-synuclein and β-amyloid domains that were confirmed by in vitro experiments to be involved in AP formation. Based on studies of how the β-amyloid tetramer and α-synuclein octamer insert into membranes (Tsigelny et al., 2012; Ciudad et al., 2020) we propose a possible common mechanism of membrane AP formation for other AFPs (Figure 3).
Figure 2. Lipid-binding domains and transmembrane regions in β-amyloid and α-synuclein that are involved in amyloid pore formation; orange—ganglioside-binding motif (GBM), green—cholesterol-binding motif (CBM), line pattern—transmembrane region (TM). Representation is based on results of Tsigelny et al. (2012), Di Scala et al. (2016), and Ciudad et al. (2020).
Figure 3. Model of the amyloid-membrane disruption mechanism based on a case study of β-amyloid (Aβ). (A) General scheme of membrane disruption; monomer secondary structure transition with aggregation into oligomer(s) and pore formation. (B) Structural data of membrane disruption by Aβ; soluble monomer α-helix (PDB: 1IYT), β-sheet structure of Aβ tetramer arrangement in membrane environment (PDB: 6RHY), MD simulations in DPPC membrane bilayer of Aβ octamer (Ciudad et al., 2020).
The data set for this in silico experiment was generated by an extensive literature search for human proteins with a known amyloidogenic mode of action. A total of 30 proteins were selected for this study. The amino acid sequences of these proteins were compiled from UniProtKB database (https://www.uniprot.org/). The list of proteins and UniProtKB codes are shown in Table 1. The detailed protein descriptions and amino acid sequence representation and results of TM regions predictions, amyloidogenic regions, GBM and CBM regions are available in Supplementary Tables 1, 5).
Using the AmyPro database, we screened selected amino acid sequences against the entire collection of validated amyloidogenic sequence fragments to predict amyloidogenic regions within proteins (Varadi et al., 2018) (https://amypro.net). The database is publicly accessible and provides the boundaries of experimentally validated amyloidogenic sequence regions. Additional data are available, such as the functional relevance of the proteins and their amyloid state, experimental techniques used in the amyloid state studies, and relevant data transferred from the UniProt database.
Furthermore, the consensus method for the prediction of the amyloid propensityAmylPred2 (Tsolis et al., 2013) (http://aias.biol.uoa.gr/AMYLPRED2), was implemented in our data set. The FASTA format of the sequences was used as input. The consensus of different methods specifically developed for the prediction of features related to the formation of amyloid fibrils was generated for each protein. In this work a consensus of at least four methods was used.
RFAmyloid is a platform for protein sequence analysis based on machine learning approaches (Niu et al., 2018) (http://server.malab.cn/RFAmyloid). With the RFAmy classifier we estimated the propensity for amyloid based on the input of selected amino acid sequences in FASTA format. The predictions are based on the training set of original protein sequences from the Uniprot and AmyPro data sets and the technique of random forest for the classification of protein sequences ( ≤ 0.5 non-amyloid, >0.5 amyloid).
Several programs are available for the prediction of TM segments of proteins, either α-helices or β-strands. In this study different predictors were used, which are listed in Supplementary Table 2. All predictors are freely available online. PredαTM and PredβTM (Roy Choudhury and Novič, 2015) were developed in our laboratory and show reliable performance with reasonable predictions of α-helices or β-strands when compared to other predictors used. Reports of the benchmark analyzed are available in studies by Venko et al. (2017) and Roy Choudhury and Novič (2015). The PredαTM and PredβTM are two-layer predictors; the first layer is a classifier of TM segments, while the second layer is an adjustment of the border amino acids of the TM segments, based on the propensity of border amino acids in structurally solved TM proteins available in the PDB database (Roy Choudhury and Novič, 2009). The initial classifier for predicting α-helix TM segments was based on the artificial neural network algorithm (Pasquier et al., 1999), later both classifiers were upgraded by using the support vector machine algorithm (Venko et al., 2017). Algorithms PredαTM and PredβTM are using the sliding window approach (20 and 10 amino acids for α-helix and β-strand, respectively) and each segment is classified by the pre-developed SVM classifier as either transmembrane or non-transmembrane (Roy Choudhury and Novič, 2012).
By concept, α- or β-TM regions are segments of predominantly hydrophobic residues, which are energetically suitable for the hydrophobic membrane environment and have aromatic/charged residues at the membrane-water interface (terminal positions of the TM regions). In general, the identification of α- or β-TM regions can be approached by two different concepts: pattern-based or homology-based. By first, TM features are predicted based on algorithms using hydrophobicity scales or sequence similarity, by second, the prediction is based on algorithms that make a comparison with existing data from homologs. Therefore, in the first case the applicability for homologs and non-homologs is theoretically the same, while in the second case the probability of the prediction depends on the homology rate or is biased with it (Venko et al., 2017). Since all TM proteins with currently known high-resolution structures are strictly homomers and no mix assemblies of both TM segments have yet been determined, the predictors for each TM unit have been separated and developed separately to achieve a better precision in the predictions of the TM regions. Both types of predictors use different computational methods, which can generally be categorized into three classes: physico-chemical methods [PRED-TMR (Pasquier et al., 1999), BOMP (Berven et al., 2004)], statistical methods [TMpred (Hofmann and Stoffel, 1993)], and machine learning methods [HMMTOP (Tusnády and Simon, 2001), TMHMM (Krogh et al., 2001), MEMSAT-SVM (Nugent and Jones, 2009), PredαTM (Roy Choudhury and Novič, 2015), OCTOPUS (Viklund and Elofsson, 2008), B2TMPRED (Jacoboni et al., 2001), PRED-TMBB (Bagos et al., 2004), PredβTM (Roy Choudhury and Novič, 2015), TBBpred (Natt et al., 2004), BOCTOPUS2 (Hayat et al., 2016), PureseqTM (Wang et al., 2019), MPEx (Snider et al., 2009), ABTMpro (Cheng et al., 2005)]. In addition, it is proposed to apply a consensus approach for relevant predictions based on the analysis of the results of various currently available predictors. This type of consensus approach is already included in predictors such as CCTOP (Dobson et al., 2015), TOPCONS (Tsirigos et al., 2015) and ConBBPred (Bagos et al., 2005). Machine learning methods are regarding various performance analyses recognized as the most advanced and accurate (Roy Choudhury and Novič, 2015; Venko et al., 2017). Most often they are based on learning algorithms such as Support Vector Machines, Hidden Markov Models and Neural Networks. Interfacial hydropathy profile with White-Wimley scale was defined in MPEx (Snider et al., 2009). Further on, an FFPred3 (Cozzetto et al., 2016) server was used for feature-based function prediction and then a search for any membrane gene ontology domains was performed.
All protein sequences were manually screened for the presence of CBM and GBM, as suggested by Fantini and colleagues (Fantini and Barrantes, 2013; Yahi and Fantini, 2014; Fantini et al., 2020). Cholesterol interacts with membrane lipids and proteins at the molecular/atomic scale, thus the consensus cholesterol binding motifs CRAC and/or CARC were characterized (Fantini and Barrantes, 2013). The CRAC domain is generally referred as Cholesterol Recognition/interaction Amino acid Consensus sequence present in the TM segment. This is motif of mandatory amino acid residues (L/V)-X1–5-(Y)-X1–5-(K/R). The CARC domain is similar to the CRAC sequence, but exhibits the opposite orientation (K/R)-X1–5-(Y/F)-X1–5-(L/V) from the N-term to the C-term (an inverted CRAC domain) (Fantini and Barrantes, 2013). Aside, a possible universal GBM is a variation of motifs consisting of a triad of mandatory amino acid residues such as (K/R)-Xn-(F/Y/W)-Xn-(K/R). While the Xn are intercalating segments of usually four to five residues, which can contain any amino acid, but often glycine (G), proline (P), and/or serine (S) residues (Yahi and Fantini, 2014).
Table 1 lists 30 human AFPs that we have selected for analysis based on experimental evidence that 29 of them form amyloid aggregates. We also included secretin as a putative negative control, since it was experimentally shown not to form amyloid, however, by predictive methods it proved to be highly amyloidogenic (Table 1, Supplementary Table 5). The functional category for each of the studied proteins is also shown in Table 1. Particularly, in addition to the 19 pathological AFPs, we also included three biologically non-relevant, seven functional amyloids and one negative control. However, for amyloidogenic protein cathelicidin the contrasting results among different sources were reported.
The chosen AFPs were examined for their propensity to form amyloid using the RFAmy predictor and for possible amyloidogenic sequence fragments using the AmyPro database and the AmylPred2 predictor. The results are shown in Table 1. A detailed graphical sequence representation is available in Supplementary Table 5, where the AmyPro amyloidogenic validated sequence fragments are marked. The RFAmy program classified 28 proteins as amyloids (probability >0.5). Using the AmyPRO database, the amyloidogenic sequence fragments were determined for 25 of the selected proteins; whereas for myoglobin and α-phosphatidyl inositol 3-kinase, homologous sequences from other species represented in the AmyPRO database were used. The only exceptions were stefin A, α-crystallin B chain, α-1-antichymotrypsin, cathelicidin, and secretin. For the above cases, it was crucial to use AmylPred2 (Tsolis et al., 2013) a consensus approach to predict amyloidogenic sequence fragments.
An interesting observation was made for α-1-antichymotrypsin since on one side, this protein was found in the amyloid plaques from the hippocampus of Alzheimer disease brains (Shoji et al., 1991; Padmanabhan et al., 2006; Tyagi et al., 2013) and known to promote Aβ deposition in plaques (Ma et al., 1994; Eriksson et al., 1995; Nilsson et al., 2001), thus confirming its pathological role. On the other side, we found a sequence homology with urocortin, a functional amyloid (Maji et al., 2009). Moreover, the antibacterial peptide cathelicidin was reported to act as immunomodulator that can contribute to the development of autoimmune diseases (Kahlenberg and Kaplan, 2013) and promote inflammation (Takahashi et al., 2018). On the other side, its exhibits a protective role as an inhibitor of amyloid self-assembly of Aβ (De Lorenzi et al., 2017) and islet amyloid polypeptide (IAPP) (Armiento et al., 2020).
Potential TM regions, either α-helices or β-strands have been predicted from amino acid sequences of the 305 proteins. Interestingly, for almost all AFPs at least one α- or β-TM region was determined. Table 2 shows the regions that can form TM α-helices or β-strands. The results of the predictions for each TM predictor are shown in Supplementary Table 3. Table 2 lists only α- or β-TM regions that meet the following criteria: they are predicted with at least two or more TM predictors, or the predicted TM region characterizes the same residues and secondary structures as in experimentally solved 3D structure of the soluble native form. In Supplementary Table 3 we have highlighted the regions which may form TM α-helices (gray color) and the regions which may form TM β-strands (yellow color).
Table 2. Transmembrane α-helix and β-strand predictions for the set of 30 amyloid-forming proteins; in italics are TM regions with CBM.
For the majority of proteins, β-TM secondary structures were more likely, but the ATMBpro predictor favored α-helices in all cases. Compared to other TM predictors, the ATMBpro tool is more restrictive in predicting whether a protein has the potential to be TM or not, since only three of 30 proteins have a high probability of being TM proteins (>0.5), seven have a medium probability (≥0.1 and ≤ 0.5) and the rest have a very low probability (<0.1). Interesting are also the predictions of the MEMSAT-SVM predictor, which for 17 proteins emphasizes the α-TM regions as pore-lining helices. In general, only one α-TM region is predicted for the majority of proteins, while β-TM regions are predicted more frequently, usually up to three or even more regions per protein (Table 2, Supplementary Tables 3, 5). Furthermore, the interfacial hydropaty profiles of all analyzed proteins are represented in Supplementary Table 4. Noteworthy, the results of FFPred3 search for membrane feature-based functions showed some membrane gene ontology domains in almost all analyzed proteins (Supplementary Table 6).
For almost all 30 analyzed proteins cholesterol and ganglioside binding motifs were detected. In Table 3 the sequences of CBM and GBM according to codes [(L or V)-(1–5 residues)-(Y)-(1–5 residues)-(K or R)], [(K or R)-(1–5 residues)-(Y or F)-(1–5 residues)-(L or V)] (Fantini and Barrantes, 2013), and [(K or R)-(4–6 residues)-(F or Y or W)-(4–6 residues)-(K or R)] (Yahi and Fantini, 2014; Fantini et al., 2020) are listed. Moreover, on a schematic representation of each protein sequence, the GBM and CBM motifs are highlighted in orange and green colors, respectively (Supplementary Table 5).
Further on, the representative TM regions which include a CBM are underlined in Table 2. Namely, all 19 pathological AFPs were determined to possess at least one TM region, which fulfilled the criteria of including all three regions (TM, CBM, and GBM), while on the contrary; the functional AFPs and negative control do not satisfy above mentioned criteria (Table 4). Among the biologically non-relevant proteins α-phosphatidylinositol 3-kinase and stefin A possess TM regions with the fulfilled criteria (TM, CBM, and GBM), while myoglobin does not show TM regions, which would fulfill TM-CBM-GBM criteria.
Table 4. Representation of 30 amyloid-forming proteins (AFPs) according to amyloid category and fulfilled criteria of including all three domains (transmembrane, cholesterol, and ganglioside binding regions).
In accordance with the proposal of Dobson (2002) and Chiti and Dobson (2017) that any protein under proper conditions can transform into amyloid state, we determined the propensity to form amyloids for all 30 AFPs (Table 1). However, the kinetics of amyloid fibril formation is dictated by stability of the protein and its tendency to form folding intermediates (Dobson, 2017) as seen for example in the case of stefin B against stefin A (Jenko et al., 2004).
Similarly, it is believed that most if not all amyloid proteins can form oligomers, which exert toxicity via membrane binding and perforation (Bucciantini et al., 2002; Stefani and Dobson, 2003). The channel theory of Alzheimer's disease (AD) was proposed in 1993 by Arispe et al. (1993a,b), who stated that β-amyloid (Aβ) peptide perforates the plasma membrane, leading to the entry of Ca2+ ions and downstream signaling, which eventually causes cytotoxicity (Pacheco et al., 2015; Di Scala et al., 2016). Not long ago, the structure of the Aβ oligomer that could perforate the plasma membrane was proposed based on molecular dynamics and solid state NMR (Ciudad et al., 2020), which contributes to a better understanding of the possible mechanism of toxicity in AD (Press-Sandler and Miller, 2018). Meanwhile, Lashuel et al. (2002) and Lashuel and Lansbury (2006) describe that APs are formed by many amyloidogenic proteins and are a common source of amyloid-induced toxicity. The mechanism of their formation is still not well-understood and the imaging of pores in living cells remains an open issue. However, not so recent ago APs by Aβ were confirmed in living cells (Bode et al., 2017) and the membrane repair response was induced by Aβ in C. elegans model (Julien et al., 2018).
In order to get a deeper understanding of amyloid membrane interaction, we used different bioinformatics and machine learning tools to predict amyloidogenic (Table 1) and TM regions (Table 2, Supplementary Table 3) in a set of 30 selected proteins, all associated with protein misfolding and aggregation into amyloid fibrils (Sawaya et al., 2007). Since machine learning approaches are best suited to solve problems in the absence of general theories (i.e., large amounts of data with noisy patterns), they are ideal for usage in the case of protein complexity. According to the results of the α-TM region predictions the Memsat-SVM predictor is one of the most sensitive, since this predictor is the only one that predicts α-TM regions in 25 proteins. However, Memsat-SVM predictor in benchmark analyses in deed performed as one of the best TM predictors. In particular performs well at predicting the correct number of TM helices (95% accuracy) and also has a balanced number of over- and under predictions, which is favorable to avoid bias toward either type of prediction, and suggests good sensitivity while avoiding over predicting helices. By statistical parameters has very low rate of false positives (4%), for in comparison to others predictors, which have in general rate of false positives >10% (Nugent and Jones 2009; Venko et al., 2017). The TMpred and TMHMM predictors estimated α-TM regions in about one third of proteins, while the remaining α-TM predictors estimated α-TM regions in only three of the 30 AFPs. Such a difference in the sensitivity of α-TM regions predictors is somewhat surprising, since most predictors for α-TM regions in benchmark analysis showed very high (≥90%) sensitivities (Venko et al., 2017). Anyhow, since of the amphipathic nature of the β-TM regions, the hydrophobicity alone is an inefficient differentiating factor, so in advanced β-TM predictors the inclusion of non-linear statistics and evolutionary profiles was added to optimize predictions (Bagos et al., 2005). The recent benchmark analysis for β-TM predictors presented in Venko et al. (2017) shows that the PredβTM predictor based on machine-learning methodology currently outperforms all state-of-art β-TM region prediction methods. Indeed, in 27 proteins β-strand TM regions were predicted with the PredβTM predictor. Some predictors were less sensitive (PRED-TMBB, B2Tmpred, MEPx-BB), while the remaining predictors did not predict any β-TM regions. This fact is consisted with the estimated sensitivity of the separate β-TM predictors in the study by Roy Choudhury and Novič (2015). In general, the comparison between amyloidogenicity and TM potential is evident for 21 AFPs. As shown in Table 2, at least one or more TM regions in each protein were predicted by several TM region predictors. However, the estimation with the ABTMpro predictor shows that most of them have a very low TM probability score. It is interesting that those ones which appear in amyloid or neurodegenerative diseases (such as Aβ, cystatin C and prion) have a high probability of behaving as TM proteins (Di Scala et al., 2016; Kandel et al., 2017). However, in most AFPs sequences both TM secondary structures α-helices and β-strands were predicted, thus it is hard to decide, which one is the preferred one in AP formation. Tsigelny et al. (2012) in their study of α-synuclein membrane interaction provided reasonable explanation of this ambiguity and pointed out that during membrane binding and TM transition both secondary structures possibly occur. Their computational analysis of α-synuclein TM scores predicted that the region including residues 64–79 resembles a TM helix, since this region contains a significant number of hydrophobic residues that could play a critical role during the process of membrane penetration. Further analysis shows that α-synuclein α-helical conformer penetrates the membrane and undergoes change in the secondary structure with portions of the α-helices converting into π-helices and eventually extending into β-strands (Sepúlveda et al., 2014).
For the 42 amino acids long β-amyloid (Aβ) they have recently by using a combination of molecular dynamics calculations and solid state NMR measurements determined the structure of the pore-forming oligomers in lipid environment [tetramers/octamers, PDB: 6RHY (Ciudad et al., 2020)] (Figure 3B). Using several TM predictors, we showed that for the Aβ peptide, both types of TM regions are possible (Table 2, Supplementary Table 3). Although the ATMBpro predictor seems to prefer the α-helix structure, Ciudad et al. (2020) showed in their semi-empirical study that β-strand structures might be involved in the oligomerization and pore formation by Aβ. The two regions (G9-A21 and K28-A42) were confirmed as TM segments and both in formation of β-strands (Ciudad et al., 2020). Thus, predictors defined the second β-strand segment (A30-V40) correctly, while the first segment was determined by B2TMpred (Y10-A21) and partly with PRED-TMBB (F4-H14) predictor.
The architecture of the Aβ tetramer [PDB: 6RHY (Ciudad et al., 2020)], which could form pores in membranes, showed that the secondary structure in the oligomer differs from that present in the soluble monomers. Two α-helices were determined in the monomer [PDB: 1IYT (Crescenzi et al., 2002)], while antiparallel β-strands are present in the tetramer. This seems to be consistent with α to β secondary structure transition on the membrane. Indeed, it is known that many amyloidogenic proteins transform into β-sheet conformation before aggregating into amyloid fibrils. This type of oligomers with higher β-structure of Hyp was shown to be more toxic (Evangelisti et al., 2016). It is possible that α-helical parts on the lipid rafts, rich in gangliosides, undergo a secondary structure transition from α to β. It is remarkable that possible ganglioside binding sites can be detected for 25 analyzed AFPs (Table 3, Supplementary Table 5). For example, comparing human stefins B and A, such a site is found at the end of the α-helical part of stefin B (K30-K39), whereas in stefin A it prolongs up to residue 44 (K30-K44) (which is an overpredicted α-helix (Žerovnik et al., 1999). Both proteins also demonstrate another potential ganglioside binding site from residues K56-R68 (stefin B) and R58-K71 (stefin A), which resides in the third β-strand of native soluble form [PDB: 1DVD, (Žerovnik et al., 2011)]. The importance of cholesterol and ganglioside-binding domains in AP formation was experimentally shown in study of Di Scala et al. (2016). Mutation or deletion of these motifs in α-synuclein and Aβ abolished pore formation. Therefore, in our study another remarkable property of AFPs was observed, namely, that also the cholesterol binding domains in TM regions were found in 25 AFPs (Table 3). In general the CRAC and/or CARC domains were detected in TM regions, but occasionally some mispredicted unrealistic cholesterol binding domains outside TM regions were also observed. This is in accordance with observations of Fantini and Barrantes (2013).
The schematic mechanism of Aβ pore formation based on the possible tetramer structure (Ciudad et al., 2020) is depicted in Figure 3B. Derived from the case of Aβ we propose a more general mechanism (Figure 3A). This may apply to most amyloidogenic proteins, including cystatin C and the stefins A and B, which are involved in the typical amyloid disease; the hereditary amyloid angiopathy (cystatin C) or in a progressive myoclonal epileptic syndrome EPM1 with features of neurodegeneration (stefin B) and are non-physiological (such as stefin A) serving as model proteins in our previous work on protein aggregation to amyloid fibrils (stefins A and B) (Žerovnik et al., 1999, 2010; Anderluh and Žerovnik, 2012). In Parkinson's disease, the calcium-permeable pores formed by small oligomers of α-synuclein are thought the primary pathological species (Sepúlveda et al., 2014; Di Scala et al., 2016; Press-Sandler and Miller, 2018; Li et al., 2020). Our predictions for α-synuclein (residues 30–80) are in concordance with previously experimentally confirmed AP domains (Figure 2) (Sepúlveda et al., 2014). For the islet amyloid polypeptide and calcitonin experiments have also been conducted, which further confirm our assumption that many amyloidogenic proteins have potential to induce toxicity via pore formation (Press-Sandler and Miller, 2018). The islet amyloid polypeptide (IAPP or amylin) is a highly amyloidogenic peptide, and it has been hypothesized that transient membrane-bound α-helical structures of human IAPP are precursors of the amyloid deposits formation. The high-resolution structure of rat IAPP in the membrane-mimicking detergent micelles composed of dodecylphosphocholine was solved and α-TM region (A5-S23) was characterized (Nanga et al., 2009). The characterized regions are almost identical to our TM predicted regions; α-helix (A13-S28) or β-strand (C7-L16). While the MD simulations of possible structures of “amylin membrane channels” in various lipid bilayers using relatively large sizes of oligomers (12–36-mers) have been investigated and demonstrate the β-strands interfacing with the pore (Press-Sandler and Miller, 2018). The amphipathic α-helix was also experimentally determined in the membrane environment for the hormone calcitonin (T6-Y22) (Motta et al., 1991) and (S5-L19) (Hashimoto et al., 1999). The characterized regions are consistent with our TM predicted region for β-strand (L4-L12).
Furthermore, by using molecular dynamics and other computational methods the toxicity mechanism of transactive response DNA-binding protein 43 (TDP-43), which has the prion-like C-terminal domain (residues 258–414) and is believed to be a major component of neuronal inclusion bodies in amyotrophic lateral sclerosis, was studied. By the unbiased atomic-detailed molecular dynamics simulations, the C-terminal fragments of TDP-43 were observed to aggregate and form disordered-toroidal pores in a lipid bilayer (Chen et al., 2016). Apart, the interaction of tau protein with membranes was recently investigated experimentally, in aim to characterize the effect of the tau-membrane interactions on the function, aggregation, and toxicity of tau in neuronal cultures. Although, the atomic structures of tau oligomers are unknown and currently it seems that the lack of the structure might delay the future studies on tau oligomers on membrane surfaces (Press-Sandler and Miller, 2018). Interestingly, the only relevant region predicted in tau protein is β-TM region (324–334) and is located in exon 10, which contains the microtubule-binding region and is only expressed in 4-repeat (4R) tau isoforms, while 3-repeat (3R) tau isoforms are produced without exon 10 (Kametani and Hasegawa, 2018). The rest of the proteins that were analyzed in our study, up to our knowledge, do not have experimentally solved 3D structures of their oligomers in membrane environments.
It is worth to highlight a comparison of amyloidogenic peptides with the antimicrobial ones. Although they do not share common sequences, typical secondary structures, or the same biological activity, both exhibit membrane-disruption ability to induce cytotoxicity (Zhang et al., 2014). The interactions with membranes may be on the surface or within the cell membrane. Consequently, membrane interactions may affect the structure of the amyloid species and at the same time, the structure of the membrane that leads to cytotoxicity. Despite the existence of different membrane disruption mechanisms, the formation of TM pores appears to be a generic mechanism applicable to both antimicrobial and amyloidogenic membrane interacting peptides (Zhang et al., 2014; Press-Sandler and Miller, 2018). A comparison of different computationally modeled and experimental observed amyloid channels reveals several common features in channel structure and activity. Amyloid membranes channels preferably contain a U-shaped β-strand—turn-β-strand conformation (Zhang et al., 2014). In general, three models for the mechanism of membrane interaction/perforation by amyloid or antimicrobial peptides have been proposed: pore model, carpeting model, and detergent-like model (Zhang et al., 2014; Press-Sandler and Miller, 2018). However, the understanding of the molecular mechanisms of amyloidogenic proteins interaction with membranes remains a challenge to both experimental and computational studies.
Even though all proteins may under certain conditions form amyloid state (according to Dobson, 2002), they differ in the propensity and likehood to form such a state, depending on thermodynamic and kinetic factors and environment, such as temperature, pH, reactive oxidative species—i.e., free radicals (ROS) and the crowding milieu. In our analysis we cannot predict all these factors but get by using various predictive methods a number expressing the propensity to transform into amyloid state (Table 1). From the functional point of view, among the 30 analyzed amyloid forming proteins (AFPs), we can differentiate those, which are a hallmark of disease and are termed “pathological” (19 cases), those that are biologically non-relevant (3), those that are “functional” (7) and a putative negative control (Table 1). The results of our study confirm a common feature of AFPs to possess regions of TM segments, either α-TM helices or β-TM strands, as proposed by several TM predictors (Table 2). Moreover, interactions of amyloidogenic proteins with membranes via lipid rafts rich in gangliosides and cholesterol are indicated (Table 3), as the predictions confirm such binding sites in all of the 19 pathological AFPs, while they are not fully present in functional amyloids (Table 4). Based on the membrane interaction and structural data of a generic oligomer type of an AFP (Aβ) leading to AP (Tsigelny et al., 2012; Ciudad et al., 2020), we suggest, that such mechanism of induced toxicity via AP formation could be indeed a generic property (Bucciantini et al., 2002). Since Ciudad et al. (2020) emphasize that toxicity arises from the hydrophilic residues located on the edges of the β-sheets, which lead to the formation of lipid-stabilized pores, the oligomerization and the α-TM helix or β-TM strand transition on the membrane surface (on lipid rafts) seem to be the common key events. Hopefully, in the near future stable TM regions that were defined in this study will be further confirmed experimentally for several amyloidogenic proteins. Thus, potentially, all AFPs can under certain circumstances form APs and become toxic. It depends, where and how this happens and if it leads to pathology or is transient, perhaps signaling proteotoxic stress to cells (Protter and Parker, 2016).
The original contributions presented in the study are included in the article/Supplementary Materials, further inquiries can be directed to the corresponding authors.
EŽ, VS, and MN: conceptualization. KV and VS: methodology and analysis. KV: software and visualization. KV, VS, and EŽ: investigation. KV and EŽ: writing—original draft preparation. MN and VS: writing—review and editing. VS: supervision. MN: project administration. MN and EŽ: funding acquisition. All authors have read and agreed to the published version of the manuscript.
This research was funded by Slovenian Research Agency, ARRS program grant P1-0017 (led by MN) and P1-0140 (led by prof. B. Turk).
The authors declare that the research was conducted in the absence of any commercial or financial relationships that could be construed as a potential conflict of interest.
The Supplementary Material for this article can be found online at: https://www.frontiersin.org/articles/10.3389/fnmol.2021.619496/full#supplementary-material
AP, amyloid pore; APFs, amyloid-forming proteins; CBM, cholesterol binding motif; GBM, ganglioside binding motif; TM, transmembrane.
Anderluh, G., and Žerovnik, E. (2012). Pore formation by human stefin B in its native and oligomeric states and the consequent amyloid induced toxicity. Front. Mol. Neurosci. 5:85. doi: 10.3389/fnmol.2012.00085
Arispe, N., Pollard, H. B., and Rojas, E. (1993b). Giant multilevel cation channels formed by Alzheimer disease amyloid beta-protein [A beta P-(1-40)] in bilayer membranes. Proc. Natl. Acad. Sci. U.S.A. 90, 10573–10577. doi: 10.1073/pnas.90.22.10573
Arispe, N., Rojas, E., and Pollard, H. B. (1993a). Alzheimer disease amyloid beta protein forms calcium channels in bilayer membranes: blockade by tromethamine and aluminum. Proc. Natl. Acad. Sci. U.S.A. 90, 567–571. doi: 10.1073/pnas.90.2.567
Arispe, N., Williams, M. R., Rivera, I., Lin, H. P., Cauvi, D. M., and De Maio, A. (2014). Calcium channel blockers used as anti-hypertension agents affect the toxicity of Aβ peptides on neurons. Biophys. J. 106:295a. doi: 10.1016/j.bpj.2013.11.1721
Armiento, V., Hille, K., Naltsas, D., Lin, J. S., Barron, A. E., and Kapurniotu, A. (2020). The human host-defense peptide cathelicidin LL-37 is a nanomolar inhibitor of amyloid self-assembly of islet amyloid polypeptide (IAPP). Angew. Chem. Int. Ed. Engl. 59, 12837–12841. doi: 10.1002/anie.202000148
Bagos, P. G., Liakopoulos, T. D., and Hamodrakas, S. J. (2005). Evaluation of methods for predicting the topology of beta-barrel outer membrane proteins and a consensus prediction method. BMC Bioinform. 6:7. doi: 10.1186/1471-2105-6-7
Bagos, P. G., Liakopoulos, T. D., Spyropoulos, I. C., and Hamodrakas, S. J. (2004). A Hidden Markov Model method, capable of predicting and discriminating beta-barrel outer membrane proteins. BMC Bioinform. 5:29. doi: 10.1186/1471-2105-5-29
Berven, F. S., Flikka, K., Jensen, H. B., and Eidhammer, I. (2004). BOMP: a program to predict integral beta-barrel outer membrane proteins encoded within genomes of Gram-negative bacteria. Nucleic Acids Res. 32, W394–399. doi: 10.1093/nar/gkh351
Bode, D. C., Baker, M. D., and Viles, J. H. (2017). Ion channel formation by amyloid-beta 42 oligomers but not amyloid-beta 40 in cellular membranes. J. Biol. Chem. 292, 1404–1413. doi: 10.1074/jbc.M116.762526
Bucciantini, M., Giannoni, E., Chiti, F., Baroni, F., Formigli, L., Zurdo, J., et al. (2002). Inherent toxicity of aggregates implies a common mechanism for protein misfolding diseases. Nature 416, 507–511. doi: 10.1038/416507a
Capitini, C., Patel, J. R., Natalello, A., D'Andrea, C., Relini, A., Jarvis, J. A., et al. (2018). Structural differences between toxic and nontoxic Hyp F-N oligomers. Chem. Commun. 54, 8637–8640. doi: 10.1039/C8CC03446J
Capone, R., Jang, H., Kotler, S. A., Connelly, L., Arce, F. T., Ramachandran, S., et al. (2012). All-d-enantiomer of β-amyloid peptide forms ion channels in lipid bilayers. J. Chem. Theory Comput. 8, 1143–1152. doi: 10.1021/ct200885r
Chen, C. H., Khan, A., Huang, J. J. T., and Ulmschneider, M. B. (2016). Mechanisms of membrane pore formation by amyloidogenic peptides in amyotrophic lateral sclerosis. Chem. Eur. J. 22, 9958–9961. doi: 10.1002/chem.201601765
Cheng, J., Randall, A., Sweredoski, M., and Baldi, P. (2005). SCRATCH: a protein structure and structural feature prediction server. Nucleic Acids Res. 33, w72–76. doi: 10.1093/nar/gki396
Chiti, F., and Dobson, C. M. (2017). Protein misfolding, amyloid formation, and human disease: a summary of progress over the last decade. Ann. Rev. Biochem. 86, 27–68. doi: 10.1146/annurev-biochem-061516-045115
Ciudad, S., Puig, E., Botzanowski, T., Meigooni, M., Arango, A. S., Do, J., et al. (2020). Aβ(1-42) tetramer and octamer structures reveal edge conductivity pores as a mechanism for membrane damage. Nat. Commun. 11:3014. doi: 10.1038/s41467-020-16566-1
Cozzetto, D., Minneci, F., Currant, H., and Jones, D. T. (2016). FFPred 3: feature-based function prediction for all Gene Ontology domains. Sci. Rep. 6:31865. doi: 10.1038/srep31865
Crescenzi, O., Tomaselli, S., Guerrini, R., Salvadori, S., D'Ursi, A. M., Temussi, P. A., et al. (2002). Solution structure of the Alzheimer amyloid beta-peptide (1-42) in a polar microenvironment. Similarity with a virus fusion domain. Eur. J. Biochem. 269, 5642–5648. doi: 10.1046/j.1432-1033.2002.03271.x
De Lorenzi, E., Chiari, M., Colombo, R., Cretich, M., Sola, L., Vanna, R., et al. (2017). Evidence that the human innate immune peptide LL-37 may be a binding partner of Amyloid-β and inhibitor of fibril assembly. J. Alzheimers Dis. 59, 1213–1226. doi: 10.3233/JAD-170223
Di Scala, C., Yahi, N., Boutemeur, S., Flores, A., Rodriguez, L., Chahinian, H., et al. (2016). Common molecular mechanism of amyloid pore formation by Alzheimer's beta-amyloid peptide and alpha-synuclein. Sci. Rep. 6:28781. doi: 10.1038/srep28781
Diaz, J. C., Simakova, O., Jacobson, K. A., Arispe, N., and Pollard, H. B. (2009). Small molecule blockers of the Alzheimer Aβ calcium channel potently protect neurons from Aβ cytotoxicity. Proc. Natl. Acad. Sci. U.S.A. 106, 3348–3353. doi: 10.1073/pnas.0813355106
Dobson, C. M. (2002). Protein-misfolding diseases: getting out of shape. Nature 418, 729–730. doi: 10.1038/418729a
Dobson, C. M. (2017). The amyloid phenomenon and its links with human disease. Cold Spring Harb. Perspect. Biol. 9:a023648. doi: 10.1101/cshperspect.a023648
Dobson, L., Reményi, I., and Tusnády, G. E. (2015). CCTOP: A Consensus Constrained TOPology prediction web server. Nucleic Acids Res. 43, W408–W412. doi: 10.1093/nar/gkv451
Dong, X., Sun, Y., Wei, G., Nussinov, R., and Ma, B. (2017). Binding of protofibrillar Abeta trimers to lipid bilayer surface enhances Abeta structural stability and causes membrane thinning. Phys. Chem. Chem. Phys. 19, 27556–27569. doi: 10.1039/C7CP05959K
Dovidchenko, N. V., Leonova, E. I., and Galzitskaya, O. V. (2014). Mechanisms of amyloid fibril formation. Biochemistry 79, 1515–1527. doi: 10.1134/S0006297914130057
Eriksson, S., Janciauskiene, S., and Lannfelt, L. (1995). Alpha 1-antichymotrypsin regulates Alzheimer beta-amyloid peptide fibril formation. Proc. Natl. Acad. Sci. U.S.A. 92, 2313–2317. doi: 10.1073/pnas.92.6.2313
Evangelisti, E., Cascella, R., Becatti, M., Marrazza, G., Dobson, C. M., Chiti, F., et al. (2016). Binding affinity of amyloid oligomers to cellular membranes is a generic indicator of cellular dysfunction in protein misfolding diseases. Sci. Rep. 6:32721. doi: 10.1038/srep32721
Fandrich, M., Fletcher, M. A., and Dobson, C. M. (2001). Amyloid fibrils from muscle myoglobin. Nature 410, 165–166. doi: 10.1038/35065514
Fantini, J., and Barrantes, F. J. (2013). How cholesterol interacts with membrane proteins: an exploration of cholesterol-binding sites including CRAC, CARC, and tilted domains. Front. Physiol. 4:31. doi: 10.3389/fphys.2013.00031
Fantini, J., Di Scala, C., Chahinian, H., and Yahi, N. (2020). Structural and molecular modelling studies reveal a new mechanism of action of chloroquine and hydroxychloroquine against SARS-CoV-2 infection. Int. J. Antimicrob. Agents 55:105960. doi: 10.1016/j.ijantimicag.2020.105960
Gazit, E. (2002). A possible role for pi-stacking in the self-assembly of amyloid fibrils. FASEB J. 16, 77–83. doi: 10.1096/fj.01-0442hyp
Gazit, E. (2007). Self assembly of short aromatic peptides into amyloid fibrils and related nanostructures. Prion 1, 32–35. doi: 10.4161/pri.1.1.4095
Hannestad, J. K., Rocha, S., Agnarsson, B., Vladimir, P., Zhdanov, V. P., Wittung-Stafshede, P., et al. (2020). Single-vesicle imaging reveals lipid-selective and stepwise membrane disruption by monomeric α-synuclein. Proc. Natl. Acad. Sci. U.S.A. 117, 14178–14186. doi: 10.1073/pnas.1914670117
Hasanbasic, S., Taler-Vercic, A., Puizdar, V., Stoka, V., Tusek Znidaric, M., Vilfan, A., et al. (2019). Prolines affect the nucleation phase of amyloid fibrillation reaction; Mutational analysis of human stefin B. ACS Chem. Neurosci. 10, 2730–2740. doi: 10.1021/acschemneuro.8b00621
Hashimoto, Y., Toma, K., Nishikido, J., Yamamoto, K., Haneda, K., Inazu, T., et al. (1999). Effects of glycosylation on the structure and dynamics of eel calcitonin in micelles and lipid bilayers determined by nuclear magnetic resonance spectroscopy. Biochemistry 38, 8377–8384. doi: 10.1021/bi983018j
Hayat, S., Peters, C., Shu, N., Tsirigos, K. D., and Elofsson (2016). A. Inclusion of dyad-repeat pattern improves topology prediction of transmembrane β-barrel proteins. Bioinformatics 32, 1571–1573. doi: 10.1093/bioinformatics/btw025
Hofmann, K., and Stoffel, W. (1993). TMbase - a database of membrane spanning proteins segments. Biol. Chem. Hoppe Seyler 374:166.
Jacoboni, I., Martelli, P. L., Fariselli, P., De Pinto, V., and Casadio, R. (2001). Prediction of the transmembrane regions of beta barrel membrane proteins with a neural network based predictor. Prot. Sci. 10, 779–787. doi: 10.1110/ps.37201
Jamasbi, E., Hossain, M. A., Tan, M., Separovic, F., and Ciccotosto, G. D. (2018). Fluorescence imaging of the interaction of amyloid beta 40 peptides with live cells and model membrane. Biochim. Biophys. Acta Biomembr. 1860, 1609–1615. doi: 10.1016/j.bbamem.2018.01.024
Jang, H., Arce, F. T., Capone, R., Ramachandran, S., Lal, R., and Nussinov, R. (2009). Misfolded amyloid ion channels present mobile β-sheet subunits in contrast to conventional ion channels. Biophys. J. 97, 3029–3037. doi: 10.1016/j.bpj.2009.09.014
Jang, H., Arce, F. T., Lee, J., Alan, L., Gillman, A. L., Ramachandran, S., et al. (2016). Computational methods for structural and functional studies of Alzheimer's amyloid ion channels. Methods Mol. Biol. 1345, 251–268. doi: 10.1007/978-1-4939-2978-8_16
Jang, H., Connelly, L., Arce, F. T., Ramachandran, S., Bruce, L., Kagan, B. L., et al. (2013). Mechanisms for the insertion of toxic, fibril-like β-amyloid oligomers into the membrane. J. Chem. Theory Comput. 9, 822–833. doi: 10.1021/ct300916f
Jang, H., Zheng, J., and Nussinov, R. (2007). Models of β-amyloid ion channels in the membrane suggest that channel formation in the bilayer is a dynamic process. Biophys. J. 93, 1938–1949. doi: 10.1529/biophysj.107.110148
Jenko, S., Skarabot, M., Kenig, M., Guncar, G., Musevic, I., Turk, D., et al. (2004). Different propensity to form amyloid fibrils by two homologous proteins-Human stefins A and B: searching for an explanation. Proteins 55, 417–425. doi: 10.1002/prot.20041
Julien, C., Tomberlin, C., Roberts, C. M., Akram, A., Stein, G. H., Silverman, M. A., et al. (2018). In vivo induction of membrane damage by β-amyloid peptide oligomers. Acta Neuropatholog. Commun. 6:131. doi: 10.1186/s40478-018-0634-x
Kahlenberg, J. M., and Kaplan, M. J. (2013). Little peptide, big effects: the role of LL-37 in inflammation and autoimmune disease. J. Immunol. 191, 4895–4901. doi: 10.4049/jimmunol.1302005
Kametani, F., and Hasegawa, M. (2018). Reconsideration of amyloid hypothesis and tau hypothesis in Alzheimer's disease. Front. Neurosci. 12:25. doi: 10.3389/fnins.2018.00025
Kandel, N., Matos, J. O., and Tatulian, S. A. (2019). Structure of amyloid β25–35 in lipid environment and cholesterol dependent membrane pore formation. Sci. Rep. 9:2689. doi: 10.1038/s41598-019-38749-7
Kandel, N., Zheng, T., Huo, Q., and Tatulian, S. A. (2017). Membrane binding and pore formation by a cytotoxic fragment of amyloid β peptide. J. Phys. Chem. B 121, 10293–10305. doi: 10.1021/acs.jpcb.7b07002
Kawahara, M., Kuroda, Y., Arispe, N., and Rojas, E. (2000). Alzheimer's beta-amyloid, human islet amylin, and prion protein fragment evoke intracellular free calcium elevations by a common mechanism in a hypothalamic GnRH neuronal cell line. J. Biol. Chem. 275, 14077–14083. doi: 10.1074/jbc.275.19.14077
Kawahara, M., Ohtsuka, I., Yokoyama, S., Kato-Negishi, M., and Sadakane, Y. (2011). Membrane incorporation, channel formation, and disruption of calcium homeostasis by Alzheimer's β-amyloid protein. Internatl. J. Alzheimer's Dis. 2011:304583. doi: 10.4061/2011/304583
Kayed, R., Dettmer, U., and Lesne, S. E. (2020). Soluble endogenous oligomeric alpha-synuclein species in neurodegenerative diseases: expression, spreading, and cross-talk. J. Parkinsons Dis. 10, 791–818. doi: 10.3233/JPD-201965
Kreutzer, A. G., and Nowick, J. S. (2018). Elucidating the structures of amyloid oligomers with macrocyclic β-hairpin peptides: insights into Alzheimer's disease and other amyloid diseases. Acc. Chem. Res. 51, 706–718. doi: 10.1021/acs.accounts.7b00554
Krogh, A., Larsson, B., von Heijne, G., and Sonnhammer, E. L. L. (2001). Predicting transmembrane protein topology with a hidden Markov model: application to complete genomes. J. Mol. Biol. 305, 567–580. doi: 10.1006/jmbi.2000.4315
Lashuel, H. A., Hartley, D., Petre, B. M., Walz, T., Peter, T., and Lansbury, P. T. (2002). Neurodegenerative disease: amyloid pores from pathogenic mutations affiliations. Nature 418:291. doi: 10.1038/418291a
Lashuel, H. A., and Lansbury, P. T. (2006). Are amyloid diseases caused by protein aggregates that mimic bacterial pore-forming toxins? Q. Rev. Biophys. 39, 167–201. doi: 10.1017/S0033583506004422
Last, N. B., and Miranker, A. D. (2013). Common mechanism unites membrane poration by amyloid and antimicrobial peptides. Proc. Natl. Acad. Sci. U.S.A. 110, 6382–6387. doi: 10.1073/pnas.1219059110
Lee, J., Kim, Y. H., Arce, F. T., Gillman, A. L., Jang, H., Kagan, B. L., et al. (2017). Amyloid beta ion channels in a membrane comprising brain total lipid extracts. ACS Chem. Neurosci. 8, 1348–1357. doi: 10.1021/acschemneuro.7b00006
Leri, M., Bemporad, F., Oropesa-Nunez, R., Canale, C., Calamai, M., Nosi, D., et al. (2016). Molecular insights into cell toxicity of a novel familial amyloidogenic variant of beta 2-microglobulin. J. Cell. Mol. Med. 20, 1443–1456. doi: 10.1111/jcmm.12833
Li, S., Yuan, L., Dai, G., Chen, R. A., Liu, D. X., and Fung, T. S. (2020). Regulation of the ER stress response by the ion channel activity of the infectious bronchitis Coronavirus envelope protein modulates virion release, apoptosis, viral fitness, and pathogenesis. Front. Microbiol. 10:3022. doi: 10.3389/fmicb.2019.03022
Ma, J., Yee, A., Brewer, B. H. Jr, Das, S., and Potter, H. (1994). Amyloid-associated proteins α1-antichymotrypsin and apolipoprotein E promote assembly of Alzheimer β-protein into filaments. Nature 372, 92–94. doi: 10.1038/372092a0
Maji, S. K., Perrin, M. H., Sawaya, M. R., Jessberger, S., Vadodaria, K., Rissman, R. A., et al. (2009). Functional amyloids as natural storage of peptide hormones in pituitary secretory granules. Science 325, 328–332. doi: 10.1126/science.1173155
Morillas, M., Vanik, D. L., and Surewicz, W. K. (2001). On the mechanism of α-helix to β-sheet transition in the recombinant prion protein. Biochemistry 40, 6982–6987. doi: 10.1021/bi010232q
Motta, A., Pastore, A., Goud, N. A., and Castiglione Morelli, M. A. (1991). Solution conformation of salmon calcitonin in sodium dodecyl sulfate micelles as determined by two-dimensional NMR and distance geometry calculations. Biochemistry 30, 10444–10450. doi: 10.1021/bi00107a012
Nanga, R. P. R., Brender, J. R., Xu, J., Hartman, K., Subramanian, V., and Ramamoorthy, A. (2009). Three-dimensional structure and orientation of rat islet amyloid polypeptide protein in a membrane environment by solution NMR spectroscopy. J. Am. Chem. Soc. 131, 8252–8261. doi: 10.1021/ja9010095
Natt, N. K., Kaur, H., and Raghava, G. P. S. (2004). Prediction of Transmembrane regions of beta-barrel proteins using ANN and SVM based method. Prot. Struct. Funct. Bioinform. 56, 11–18. doi: 10.1002/prot.20092
Nelson, R., Sawaya, M. R., and Balbirnie, M. (2005). Structure of the cross-beta spine of amyloid-like fibrils. Nature 435, 773–778. doi: 10.1038/nature03680
Nilsson, L. N., Bales, K. R., DiCarlo, G., Gordon, M. N., Morgan, D., Paul, S. M., et al. (2001). Alpha-1-antichymotrypsin promotes beta-sheet amyloid plaque deposition in a transgenic mouse model of Alzheimer's disease. J. Neurosci. 21, 1444–1451. doi: 10.1523/JNEUROSCI.21-05-01444.2001
Niu, M., Li, Y., Wang, C., and Han, K. (2018). RFAmyloid: A web server for predicting amyloid proteins. Int. J. Mol. Sci. 19:2071. doi: 10.3390/ijms19072071
Nugent, T., and Jones, D. T. (2009). Transmembrane protein topology prediction using support vector machines. BMC Bioinform. 10:159. doi: 10.1186/1471-2105-10-159
Oxana, G. (2019). New mechanism of amyloid fibril formation. Current Prot. Pept. Sci. 20, 630–640. doi: 10.2174/1389203720666190125160937
Pacheco, C. R., Morales, C. N., Ramírez, A. E., Muñoz, F. J., Gallegos, S. S., Caviedes, P. A., et al. (2015). Extracellular α-synuclein alters synaptic transmission in brain neurons by perforating the neuronal plasma membrane. J. Neurochem. 132, 731–741, doi: 10.1111/jnc.13060
Padmanabhan, J., Levy, M., Dickson, D. W., and Potter, H. (2006). Alpha1-antichymotrypsin, an inflammatory protein overexpressed in Alzheimer's disease brain, induces tau phosphorylation in neurons. Brain 129, 3020–3034. doi: 10.1093/brain/awl255
Pallarés, I., and Ventura, S. (2019). Advances in the prediction of protein aggregation propensity. Curr. Med. Chem. 26, 3911–3920. doi: 10.2174/0929867324666170705121754
Pasquier, C., Promponas, V. J., Palaios, G. A., and Hamodrakas, J. S. (1999). A novel method for predicting transmembrane segments in proteins based on a statistical analysis of the SwissProt database: the PRED-TMR algorithm. Protein Eng. 12, 381–385. doi: 10.1093/protein/12.5.381
Perissinotto, F., Stani, C., De Cecco, E., Vaccari, L., Rondelli, V., Posocco, P., et al. (2020). Iron-mediated interaction of alpha synuclein with lipid raft model membranes. Nanoscale 12, 7631–7640. doi: 10.1039/D0NR00287A
Press-Sandler, O., and Miller, Y. (2018). Molecular mechanisms of membrane-associated amyloid aggregation: computational perspective and challenges. BBA Biomem. 1860, 1889–1905. doi: 10.1016/j.bbamem.2018.03.014
Protter, D. S. W., and Parker, R. (2016). Principles and properties of stress granules. Trends Cell Biol. 26, 668–679. doi: 10.1016/j.tcb.2016.05.004
Pujols, J., Peña-Díaz, S., and Ventura, S. (2018). AGGRESCAN3D: Toward the prediction of the aggregation propensities of protein structures. Methods Mol. Biol. 1762, 427–443. doi: 10.1007/978-1-4939-7756-7_21
Reymer, A., Frederick, K. K., Rocha, S., Beke-Somfai, T., Kitts, C. C., Lindquist, S., et al. (2014). Orientation of aromatic residues in amyloid cores: structural insights into prion fiber diversity. Proc. Natl. Acad. Sci. U.S.A. 111, 17158–17163. doi: 10.1073/pnas.1415663111
Roy Choudhury, A., and Novič, M. (2009). Data-driven model for the prediction of protein transmembrane regions. SAR QSAR Environ. Res. 20, 741–754. doi: 10.1080/10629360903438602
Roy Choudhury, A., and Novič, M. (2012). Amino acid distribution in transmembrane regions: a statistical analysis and comparison with globular proteins. Int. J. Chem. Model. 4, 205–219.
Roy Choudhury, A., and Novič, M. (2015). PredβTM: A novel β-transmembrane region prediction algorithm. PLoS ONE 10:e0145564. doi: 10.1371/journal.pone.0145564
Sawaya, M. R., Sambashivan, S., Nelson, R., Ivanova, M. I., Sievers, S. A., Apostol, M. I., et al. (2007). Atomic structures of amyloid cross-beta spines reveal varied steric zippers. Nature 447, 453–457. doi: 10.1038/nature05695
Sengupta, I., and Udgaonkar, J. B. (2018). Structural mechanisms of oligomer and amyloid fibril formation by the prion protein. Chem. Commun. 54, 6230–6242. doi: 10.1039/C8CC03053G
Sepúlveda, F. J., Fierro, H., Fernandez, E., Castillo, C., Peoples, R. W., Opazo, C., et al. (2014). Nature of the neurotoxic membrane actions of amyloid-ß on hippocampal neurons in Alzheimer's disease. Neurobiol. Aging 35, 472–481. doi: 10.1016/j.neurobiolaging.2013.08.035
Serra-Batiste, M., Ninot-Pedrosa, M., Bayoumi, M., Gairí, M., Maglia, G., and Carulla, N. (2016). Aβ42 assembles into specific β-barrel pore-forming oligomers in membrane-mimicking environments. Proc. Natl. Acad. Sci. U.S.A. 113, 10866–10871. doi: 10.1073/pnas.1605104113
Sezgin, E., Levental, I., Mayor, S., and Eggeling, C. (2017). The mystery of membrane organization: composition, regulation and roles of lipid rafts. Nat. Rev. Mol. Cell. Biol. 18, 361–374. doi: 10.1038/nrm.2017.16
Shoji, M., Hirai, S., Yamaguchi, H., Harigaya, Y., Ishiguro, K., and Matsubara, E. (1991). Alpha 1-antichymotrypsin is present in diffuse senile plaques. A comparative study of beta-protein and alpha 1-antichymotrypsin immunostaining in the Alzheimer brain. Am. J. Pathol. 138, 247–257.
Snider, C., Jayasinghe, S., Hristova, K., and White, S. H. (2009). MPEx: a tool for exploring membrane proteins. Protein Sci. 18, 2624–2628. doi: 10.1002/pro.256
Stefani, M., and Dobson, C. M. (2003). Protein aggregation and aggregate toxicity: new insights into protein folding, misfolding diseases and biological evolution. J. Mol. Med. 81, 678–699. doi: 10.1007/s00109-003-0464-5
Takahashi, T., Kulkarni, N. N., Lee, E. Y., Zhang, L. J., Wong, G. C. L., and Gallo, RL. (2018). Cathelicidin promotes inflammation by enabling binding of self-RNA to cell surface scavenger receptors. Sci. Rep. 8:4032. doi: 10.1038/s41598-018-22409-3
Tenidis, K., Waldner, M., Bernhagen, J., Fischle, W., Bergmann, M., Weber, M., et al. (2000). Identification of a penta- and hexapeptide of islet amyloid polypeptide (IAPP) with amyloidogenic and cytotoxic properties. J. Mol. Biol. 295, 1055–1071. doi: 10.1006/jmbi.1999.3422
Tsigelny, I. F., Sharikov, Y., Wrasidlo, W., Gonzalez, T., Desplats, P. A., Crews, L., et al. (2012). Role of α-synuclein penetration into the membrane in the mechanisms of oligomer pore formation. FEBS J. 279, 1000–1013. doi: 10.1111/j.1742-4658.2012.08489.x
Tsirigos, K. D., Peters, C., Shu, N., Käll, L., and Elofsson, A. (2015). The TOPCONS web server for combined membrane protein topology and signal peptide prediction. Nucleic Acids Res. 43, W401–W407. doi: 10.1093/nar/gkv485
Tsolis, A. C., Papandreou, N. C., Iconomidou, V. A., and Hamodrakas, S. J. (2013). A consensus method for the prediction of ‘aggregation-prone' peptides in globular proteins. PLoS ONE 8:e54175. doi: 10.1371/journal.pone.0054175
Tusnády, G. E., and Simon, I. (2001). The HMMTOP transmembrane topology prediction server. Bioinformatics 17, 849–850. doi: 10.1093/bioinformatics/17.9.849
Tyagi, E., Fiorelli, T., Norden, M., and Padmanabhan, J. (2013). Alpha 1-Antichymotrypsin, an inflammatory protein overexpressed in the brains of patients with Alzheimer's disease, induces Tau hyperphosphorylation through c-Jun N-Terminal kinase activation. Int. J. Alzheimers Dis. 2013:606083. doi: 10.1155/2013/606083
Varadi, M., De Baets, G., Vranken, W. F., Tompa, P., and Pancsa, R. (2018). AmyPro: a database of proteins with validated amyloidogenic regions. Nucleic Acids Res. 46, D387–D392. doi: 10.1093/nar/gkx950
Venko, K., Roy Choudhury, A., and Novič, M. (2017). Computational approaches for revealing the structure of membrane transporters: Case study on bilitranslocase. Comp. Struct. Biotechnol. J. 15, 232–242. doi: 10.1016/j.csbj.2017.01.008
Verdiá-Báguena, C., Nieto-Torres, J. L., Alcaraz, A., De Diego, M. L., Torres, J., Aguilella, V. M., et al. (2012). Coronavirus E protein forms ion channels with functionally and structurally involved membrane lipids. Virology 432, 485–494. doi: 10.1016/j.virol.2012.07.005
Viklund, H., and Elofsson, A. (2008). Improving topology prediction by two-track ANN-based preference scores and an extended topological grammar. Bioinformatics 24,1662–1668. doi: 10.1093/bioinformatics/btn221
Wang, Q., Ni, C.M., Li, Z., Li, X.F., Han, R.M., Zhao, F., et al. (2019). PureseqTM: efficient and accurate prediction of transmembrane topology from amino acid sequence only. bioRxiv [Preprint]. doi: 10.1101/627307
Wilson, L., McKinlay, C., Gage, P., and Ewart, G. (2004). SARS coronavirus E protein forms cation-selective ion channels. Virology 330, 322–331. doi: 10.1016/j.virol.2004.09.033
Yahi, N., and Fantini, J. (2014). Deciphering the glycolipid code of Alzheimer's and Parkinson's amyloid proteins allowed the creation of a universal ganglioside-binding peptide. PLoS ONE 9:e104751. doi: 10.1371/journal.pone.0104751
Žerovnik, E., Staniforth, R. A., and Turk, D. (2010). Amyloid fibril formation by human stefins: Structure, mechanism & putative functions. Biochime 92, 1597–1607. doi: 10.1016/j.biochi.2010.05.012
Žerovnik, E., Stoka, V., Mirtic, A., Guncar, G., Grdadolnik, J., Staniforth, R., et al. (2011). Mechanisms of amyloid fibril formation - focus on domain-swapping. FEBS J. 278, 2263–2282. doi: 10.1111/j.1742-4658.2011.08149.x
Žerovnik, E., Virden, R., Jerala, R., Kroon-Zitko, L., Turk, V., and Waltho, J.P. (1999). Differences in the effects of TFE on the folding pathways of human stefins A and B. Prot. Struct. Func. Bioinform. 36, 205–216. doi: 10.1002/(SICI)1097-0134(19990801)36:2<205::AID-PROT6>3.0.CO;2-4
Keywords: amyloid-forming proteins, amyloidogenic regions, transmembrane regions, amino-acid sequence predictors, cholesterol and ganglioside binding motifs, amyloid pore
Citation: Venko K, Novič M, Stoka V and Žerovnik E (2021) Prediction of Transmembrane Regions, Cholesterol, and Ganglioside Binding Sites in Amyloid-Forming Proteins Indicate Potential for Amyloid Pore Formation. Front. Mol. Neurosci. 14:619496. doi: 10.3389/fnmol.2021.619496
Received: 20 October 2020; Accepted: 12 January 2021;
Published: 10 February 2021.
Edited by:
Jinghui Luo, Paul Scherrer Institut (PSI), SwitzerlandReviewed by:
Alex Peralvarez-Marin, Autonomous University of Barcelona, SpainCopyright © 2021 Venko, Novič, Stoka and Žerovnik. This is an open-access article distributed under the terms of the Creative Commons Attribution License (CC BY). The use, distribution or reproduction in other forums is permitted, provided the original author(s) and the copyright owner(s) are credited and that the original publication in this journal is cited, in accordance with accepted academic practice. No use, distribution or reproduction is permitted which does not comply with these terms.
*Correspondence: Eva Žerovnik, ZXZhLnplcm92bmlrQGlqcy5zaQ==; Katja Venko, a2F0amEudmVua29Aa2kuc2k=
Disclaimer: All claims expressed in this article are solely those of the authors and do not necessarily represent those of their affiliated organizations, or those of the publisher, the editors and the reviewers. Any product that may be evaluated in this article or claim that may be made by its manufacturer is not guaranteed or endorsed by the publisher.
Research integrity at Frontiers
Learn more about the work of our research integrity team to safeguard the quality of each article we publish.