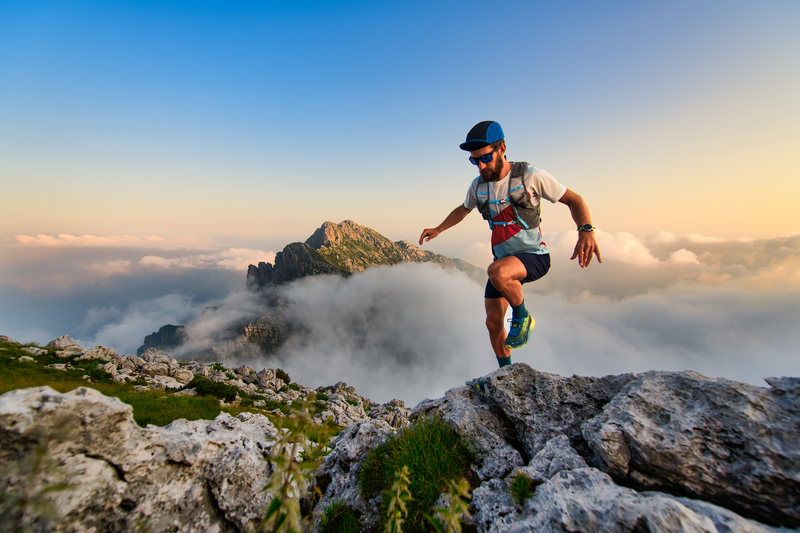
95% of researchers rate our articles as excellent or good
Learn more about the work of our research integrity team to safeguard the quality of each article we publish.
Find out more
ORIGINAL RESEARCH article
Front. Mol. Biosci.
Sec. Molecular Diagnostics and Therapeutics
Volume 12 - 2025 | doi: 10.3389/fmolb.2025.1570860
This article is part of the Research Topic Medical Knowledge-Assisted Machine Learning Technologies in Individualized Medicine Volume II View all 13 articles
The final, formatted version of the article will be published soon.
You have multiple emails registered with Frontiers:
Please enter your email address:
If you already have an account, please login
You don't have a Frontiers account ? You can register here
Background: Medulloblastoma (MB) and Ependymoma (EM) in children share similarities in age group, tumor location, and clinical presentation. Distinguishing between them through clinical diagnosis is challenging. Purpose: This study aims to explore the effectiveness of T2WI-based deep learning combined with clinical imaging features to differentiate MB and EM. Methods: Axial T2-weighted MRI sequences from 201 patients across three centers were utilized for model training and testing. Regions of interest (ROIs) were manually delineated by an experienced neuroradiologist, with oversight from a senior radiologist. We developed a deep learning (DL) classifier using a pretrained AlexNet architecture, which was fine-tuned on our dataset. To mitigate class imbalance, we implemented data augmentation techniques and employed K-fold cross-validation to enhance model generalizability. For patient classification, we utilized two voting strategies: the hard voting strategy, which selected the majority prediction from individual image slices, and the soft voting strategy, which averaged prediction scores across slices with a threshold of 0.5. Additionally, a multimodality fusion model was constructed, integrating the DL classifier with clinical and imaging features. Model performance was assessed using a 7:3 random split for training and validation. Key metrics, including sensitivity, specificity, positive predictive value (PPV), negative predictive value (NPV), F1 score, area under the ROC curve (AUC), and accuracy, were calculated, with statistical comparisons performed using the DeLong test. Medulloblastoma (MB) was classified as positive and ependymoma (EM) as negative. Results: The deep learning model utilizing a hard voting strategy achieved an AUC of 0.712 (95% CI: 0.625-0.797) on the training set and 0.689 (95% CI: 0.554-0.826) on the test set. In contrast, the multi-modality fusion model demonstrated superior performance with an AUC of 0.987 (95% CI: 0.974-0.996) on the training set and 0.889 (95% CI: 0.803-0.949) on the test set. The DeLong test indicated a statistically significant improvement in AUC for the fusion model compared to the DL model (p < 0.001), highlighting its enhanced discriminative ability. Conclusion: T2WI-based deep learning combined with multimodal clinical and imaging features could effectively differentiate MB and EM. The decision tree structure in the decision tree classifier could greatly assist clinicians in daily clinical practice.
Keywords: deep learning, Magnetic Resonance Imaging, Medulloblastoma, Ependymoma, T2WI
Received: 04 Feb 2025; Accepted: 03 Apr 2025.
Copyright: © 2025 Yimit, Yasin, Hao, Tuersun, Huang, Wang, Zou, Qiu, Wang and Nijiati. This is an open-access article distributed under the terms of the Creative Commons Attribution License (CC BY). The use, distribution or reproduction in other forums is permitted, provided the original author(s) or licensor are credited and that the original publication in this journal is cited, in accordance with accepted academic practice. No use, distribution or reproduction is permitted which does not comply with these terms.
* Correspondence:
Mayidili Nijiati, Fourth Affiliated Hospital, Xinjiang Medical University, Urumqi, China
Disclaimer: All claims expressed in this article are solely those of the authors and do not necessarily represent those of their affiliated organizations, or those of the publisher, the editors and the reviewers. Any product that may be evaluated in this article or claim that may be made by its manufacturer is not guaranteed or endorsed by the publisher.
Research integrity at Frontiers
Learn more about the work of our research integrity team to safeguard the quality of each article we publish.