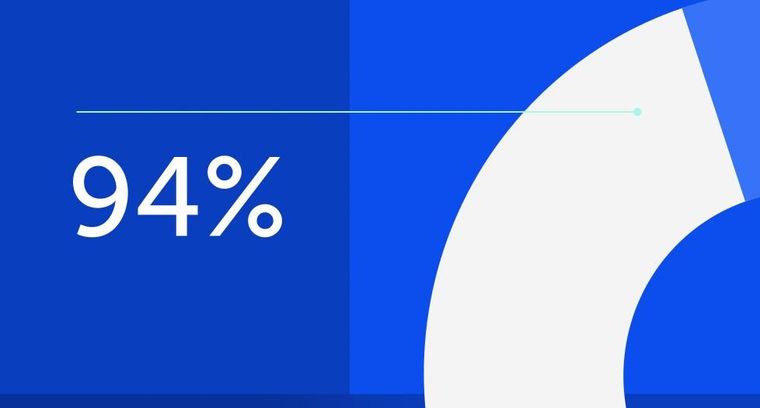
94% of researchers rate our articles as excellent or good
Learn more about the work of our research integrity team to safeguard the quality of each article we publish.
Find out more
ORIGINAL RESEARCH article
Front. Mol. Biosci.
Sec. Genome Organization and Dynamics
Volume 12 - 2025 | doi: 10.3389/fmolb.2025.1547231
This article is part of the Research TopicIntegration of Single-Cell Multi-Omics DataView all articles
The final, formatted version of the article will be published soon.
Select one of your emails
You have multiple emails registered with Frontiers:
Notify me on publication
Please enter your email address:
If you already have an account, please login
You don't have a Frontiers account ? You can register here
Graph Neural Networks (GNNs) have emerged as powerful tools for analyzing structured data, particularly in domains where relationships and interactions between entities are key. By leveraging the inherent graph structure in datasets, GNNs excel in capturing complex dependencies and patterns that traditional neural networks might miss. This advantage is especially pronounced in the field of computational biology, where the intricate connections between biological entities play a crucial role. In this context, Our work explores the application of GNNs to single-cell RNA sequencing (scRNA-seq) data, a domain characterized by complex and heterogeneous relationships. By extracting ligand-receptor (L-R) associations from LIANA and constructing Cell-Cell association networks with varying edge homophily ratios, based on L-R information, we enhance the biological relevance and accuracy of depicting cellular communication pathways. While standard GNN models like Graph Convolutional Networks (GCN), GraphSAGE, Graph Attention Networks (GAT), and MixHop often assume homophily (similar nodes are more likely to be connected), this assumption does not always hold in biological networks. To address this, we explore advanced GNN methods, such as H2GCN and Gated Bi-Kernel GNNs (GBK-GNN), that are specifically designed to handle heterophilic data. Our study spans across six diverse datasets, enabling a thorough comparison between heterophily-aware GNNs and traditional homophily-assuming models, including MLP, which disregards graph structure entirely. Our findings highlight the importance of considering data-specific characteristics in GNN applications, demonstrating that heterophily-focused methods can effectively decipher the complex patterns within scRNA-seq data. By integrating multi-omics data, including gene expression profiles and L-R interactions, we pave the way for more accurate and insightful analyses in computational biology, offering a more comprehensive understanding of cellular environments and interactions.
Keywords: Graph neural networks, single-cell RNA sequencing, cell-cell communication, Heterophily, homophily, cell type prediction
Received: 18 Dec 2024; Accepted: 07 Apr 2025.
Copyright: © 2025 Duan, Hashemi, Ngom and Rueda. This is an open-access article distributed under the terms of the Creative Commons Attribution License (CC BY). The use, distribution or reproduction in other forums is permitted, provided the original author(s) or licensor are credited and that the original publication in this journal is cited, in accordance with accepted academic practice. No use, distribution or reproduction is permitted which does not comply with these terms.
* Correspondence:
Lian Duan, University of Windsor, Windsor, Canada
Mahshad Hashemi, University of Windsor, Windsor, Canada
Alioune Ngom, University of Windsor, Windsor, Canada
Luis Rueda, University of Windsor, Windsor, Canada
Disclaimer: All claims expressed in this article are solely those of the authors and do not necessarily represent those of their affiliated organizations, or those of the publisher, the editors and the reviewers. Any product that may be evaluated in this article or claim that may be made by its manufacturer is not guaranteed or endorsed by the publisher.
Supplementary Material
Research integrity at Frontiers
Learn more about the work of our research integrity team to safeguard the quality of each article we publish.