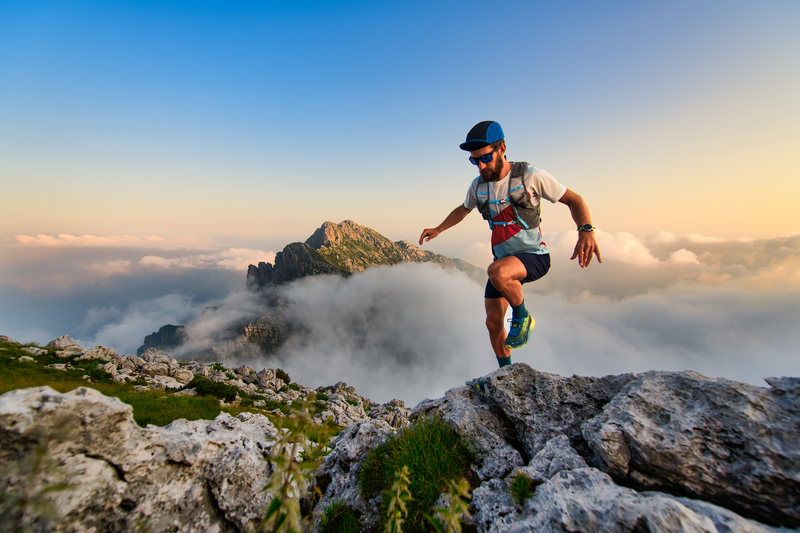
95% of researchers rate our articles as excellent or good
Learn more about the work of our research integrity team to safeguard the quality of each article we publish.
Find out more
EDITORIAL article
Front. Mol. Biosci. , 26 August 2024
Sec. Structural Biology
Volume 11 - 2024 | https://doi.org/10.3389/fmolb.2024.1479705
This article is part of the Research Topic Prediction of Protein-Protein Interactions (PPIs): The Next Frontier View all 6 articles
Editorial on the Research Topic
Prediction of protein-protein interactions (PPIs): the next frontier
Protein-protein interactions (PPIs) are essential for biological processes, and their study is pivotal for elucidating cellular mechanisms and identifying potential drug targets. In recent years, the field of structural biology has seen remarkable advancements, particularly in modeling proteins’ tertiary and quaternary structures. The introduction of Artificial Intelligence (AI) and Deep Learning (DL) methods has shown a significant increase in accuracy for structure prediction. The recently released software AlphaFold3 (Abramson et al., 2024) and RoseTTAFold (Krishna et al., 2024) significantly expand these capabilities to a broader range of biomolecular interactions, setting a new standard for the prediction of PPIs. This Research Topic aimed to highlight promising advanced computational and experimental approaches for the prediction and characterization of PPIs. It includes original research contributions that delve into specific aspects of protein interactions and protein-protein recognition.
The first research article published in this Research Topic, authored by Shin et al., introduces PPI-Surfer, a novel computational method for comparing and quantifying similarities between PPI interfaces. The method captures both the 3D shape and physicochemical properties of protein surfaces using overlapping surface patches described by three-dimensional Zernike descriptors (3DZDs), allowing for fast comparison of molecular surfaces. Unlike alignment-based methods, PPI-Surfer is alignment-free and can identify local surface similarities in PPIs by exploring combinations of similar surface patches. To comprehend a variety of protein-protein interactions in a proteome, PPI-Surfer can be a valuable tool for discovering similarities, classifying PPI sites, and assisting in protein-based PPI drug discovery.
The Original Research article by Miller et al. investigated antibody-antigen complexes and identified several features that are crucial for distinguishing strong and weak antibody-antigen binding affinity. The developed machine learning models demonstrated good predictive performance by ranking the relative importance of different features, which include energetic, statistical, network-based, and machine-learned features, in determining binding affinity. The insights gained from this research could potentially expedite progress in understanding the structural features of antibodies, classifying antibody affinity, and designing and optimizing antibodies.
The following two research articles focus on interactions between specific domains of different proteins. The experimental study conducted by Genera et al. provides insight into the interactions and structural determinants of Protein Tyrosine Phosphatase Non-Receptor Type 3 (PTPN3). The focus of the research is on the PDZ domain of PTPN3, which is involved in PPIs, as well as its interactions with both viral and cellular partners. The in silico investigations by Shah et al. utilized epitope mapping techniques to identify specific regions on the SARS-CoV-2 Omicron subvariants that are targeted by neutralizing antibodies (nAbs). This study provides valuable insight into how these subvariants interact with antibodies, which is vital for improving vaccine and therapeutic strategies.
The Original Research article by Olatona et al. identifies proteins that interact with the cytoskeleton in basal airway epithelial cells in vitro. Advanced proteomics techniques, including mass spectrometry, and bioinformatic tools were employed to analyze the proteomics data, yielding a database of candidates that may link the structural elements.
In conclusion, the articles presented here highlight diverse computational and experimental approaches that, when combined, have the potential to significantly advance our understanding of specific protein interactions and proteomics as a whole.
AS: Writing–original draft, Writing–review and editing. BN: Writing–review and editing. HN: Writing–review and editing.
The author(s) declare that no financial support was received for the research, authorship, and/or publication of this article.
The authors declare that the research was conducted in the absence of any commercial or financial relationships that could be construed as a potential conflict of interest.
The author(s) declared that they were an editorial board member of Frontiers, at the time of submission. This had no impact on the peer review process and the final decision.
All claims expressed in this article are solely those of the authors and do not necessarily represent those of their affiliated organizations, or those of the publisher, the editors and the reviewers. Any product that may be evaluated in this article, or claim that may be made by its manufacturer, is not guaranteed or endorsed by the publisher.
Abramson, J., Adler, J., Dunger, J., Evans, R., Green, T., Pritzel, A., et al. (2024). Accurate structure prediction of biomolecular interactions with AlphaFold 3. Nature 630 (8016), 493–500. doi:10.1038/s41586-024-07487-w
Keywords: protein-protein interaction (PPI), protein-protein docking, homolog modeling, drug design, structural biology, molecular recognition
Citation: Singh A, Nguyen BP and Ng HL (2024) Editorial: Prediction of protein-protein interactions (PPIs): the next frontier. Front. Mol. Biosci. 11:1479705. doi: 10.3389/fmolb.2024.1479705
Received: 12 August 2024; Accepted: 14 August 2024;
Published: 26 August 2024.
Edited and reviewed by:
Annalisa Pastore, King’s College London, United KingdomCopyright © 2024 Singh, Nguyen and Ng. This is an open-access article distributed under the terms of the Creative Commons Attribution License (CC BY). The use, distribution or reproduction in other forums is permitted, provided the original author(s) and the copyright owner(s) are credited and that the original publication in this journal is cited, in accordance with accepted academic practice. No use, distribution or reproduction is permitted which does not comply with these terms.
*Correspondence: Amar Singh, YW1hci5zaW5naEBrdS5lZHU=
Disclaimer: All claims expressed in this article are solely those of the authors and do not necessarily represent those of their affiliated organizations, or those of the publisher, the editors and the reviewers. Any product that may be evaluated in this article or claim that may be made by its manufacturer is not guaranteed or endorsed by the publisher.
Research integrity at Frontiers
Learn more about the work of our research integrity team to safeguard the quality of each article we publish.