- Division of Biological and Biomedical Sciences, College of Health and Life Sciences, Hamad Bin Khalifa University, Qatar Foundation, Doha, Qatar
Anti-COVID19 drugs, such as nirmatrelvir, have been developed targeting the SARS-CoV-2 main protease, Mpro, based on the critical requirement of its proteolytic processing of the viral polyproteins into functional proteins essential for viral replication. However, the emergence of SARS-CoV-2 variants with Mpro mutations has raised the possibility of developing resistance against these drugs, likely due to therapeutic targeting of the Mpro catalytic site. An alternative to these drugs is the development of drugs that target an allosteric site distant from the catalytic site in the protein that may reduce the chance of the emergence of resistant mutants. Here, we combine computational analysis with in vitro assay and report the discovery of a potential allosteric site and an allosteric inhibitor of SARS-CoV-2 Mpro. Specifically, we identified an Mpro metastable state with a deformed catalytic site harboring potential allosteric sites, raising the possibility that stabilization of this metastable state through ligand binding can lead to the inhibition of Mpro activity. We then performed a computational screening of a library (∼4.2 million) of drug-like compounds from the ZINC database and identified several candidate molecules with high predicted binding affinity. MD simulations showed stable binding of the three top-ranking compounds to the putative allosteric sites in the protein. Finally, we tested the three compounds in vitro using a BRET-based Mpro biosensor and found that one of the compounds (ZINC4497834) inhibited the Mpro activity. We envisage that the identification of a potential allosteric inhibitor of Mpro will aid in developing improved anti-COVID-19 therapy.
Introduction
The COVID-19 pandemic has tremendously affected human health and economic activities around the world (Cucinotta and Vanelli, 2020; Lamers and Haagmans, 2022; Wu et al., 2020). As of 17 March 2024, it has caused more than seven million deaths and 774 million infections, overwhelming the healthcare system even in countries with the best healthcare setup (WHO Coronavirus (COVID-19) Dashboard | WHO Coronavirus (COVID-19) Dashboard With Vaccination Data) (Miller et al., 2020; Cooper et al., 2023; Salje et al., 2020). The restrictions implemented to control the pandemic have resulted in substantial economic losses globally, possibly increasing losses due to the disease’s long-term impact on patient health (Jackson and Overview, 2022). At the heart of the problem is the high infectivity and rapid transmission of SARS-CoV-2 (Bian et al., 2021; Tao et al., 2021a). The viral infection cycle begins with the binding of the virus to receptors expressed on human host cells, leading to the internalization of the virus and release of its RNA genome into the host cell cytosol (V’kovski et al., 2021). This is followed by the translation of viral RNA into polyproteins containing non-structural proteins (NSPs) critical for the viral replication complex formation (Ludwig and Zarbock, 2020; Cevik et al., 2020; Harrison et al., 2020; Khalaf et al., 2020). However, to perform their function, these NSPs need to be cleaved into individual proteins through the proteolytic activity of the two SARS-CoV-2 proteases, the main protease (Mpro; chymotrypsin-like protease; 3CLpro; NSP5) (Jin et al., 2020; Hu et al., 2022; Legare et al., 2022) and papain-like protease (PLpro; NSP3) (Harrison et al., 2020), (Lee et al., 2022; Trougakos et al., 2021; Vicenti et al., 2021).
The rapid development of vaccines has indeed decelerated COVID-19-related death rates (Huespe et al., 2023; Golob et al., 2021; Hall et al., 2022; Robson, 2020). However, the continued emergence of SARS-CoV-2 variants with increased infection potential, disease severity, and resistance to antibody-mediated neutralization could make the currently administered vaccines ineffective (Jangra et al., 2021; Uwamino et al., 2022; Chen et al., 2022; McLean et al., 2022; Mistry et al., 2022; Bian et al., 2021; Tao et al., 2021a). Additionally, the emergence of novel infectious strains of coronaviruses remains a constant possibility (Tao et al., 2021a). Therefore, pharmacological targeting of SARS-CoV-2 proteases, among other essential viral proteins, remains a viable alternative for combating the pandemic (Schütz et al., 2020; Chiou et al., 2021; Jan et al., 2023a; Cheohen et al., 2023).
Given the importance of Mpro in SARS-CoV-2 replication and infection, several inhibitors have been developed so far (Jin et al., 2020; Günther et al., 2021; Samrat et al., 2022; Pang et al., 2023; Chan et al., 2021; Sabbah et al., 2021; Narayanan et al., 2022; Shree et al., 2022; Ge et al., 2022; Huff et al., 2022; Rossetti et al., 2022). These include competitive inhibitors such as TDZD-8 (Jin et al., 2020), ebselen (Jin et al., 2020), N3 (Arafet et al., 2020), 11a (Dai et al., 2020), and α-ketoamide (13b) (Zhang et al., 2020) that inhibit the activity of the protein by binding to its catalytic site. More importantly, the Mpro inhibitor nirmatrelvir, which is a part of Paxlovid®, has been approved by the FDA as a SARS-CoV-2 antiviral drug (Paciaroni et al., 2023). However, the competitive inhibition of Mpro places the catalytic site under constant evolutionary pressure to evolve in order to accommodate the substrate peptide into the catalytic site and proteolytic cleavage (Wenthur et al., 2014). This is especially relevant in the case of SARS-CoV-2 as its RNA genome is prone to mutations and the virus has already been reported to have evolved into many variants (Callaway, 2022; Tao et al., 2021b). Therefore, any mutation in the Mpro catalytic site may reduce the efficacy of antiviral drugs such as nirmatrelvir. Mutations of catalytic site residues, such as Q189K and G143S, have been reported to reduce nirmatrelvir efficacy (Noske et al., 2023). Therefore, it is necessary to continue SARS-CoV-2 antiviral drug discovery research with alternate approaches such as the development of allosteric modulators to regulate Mpro activity (Tee and Berezovsky, 2024).
Allosteric modulation or allostery can be defined as the regulation of the protein activity by the interaction of a ligand to a site that is distinct from the catalytic or substrate-binding site of the protein (Biswas, 2017; Astore et al., 2024; Wu et al., 2024; McCullagh et al., 2024). The ligand binding-induced allosteric modulation can be either activating or inhibitory depending on the nature of the impact of the allosteric ligand binding on the structure and structural dynamics of the protein (Biswas, 2017; Biswas K. et al., 2008; Biswas and Visweswariah, 2011; Biswas et al., 2015). For instance, an inhibitory allosteric ligand can induce conformational changes in the catalytic site or change the structural dynamics of the catalytic site in a way that stabilizes the protein in its inactive form (Bhat et al., 2022b; Yuce et al., 2021). Some previous studies have reported allosteric inhibitors that bind to allosteric sites in Mpro (Günther et al., 2021; Strömich et al., 2022; Tao X. et al., 2021; Verma and Pandey, 2021; Jiménez-Avalos et al., 2021). However, most of these allosteric inhibitors were identified through in silico docking studies using FDA-approved drugs, natural products, and small drug libraries (Bhat et al., 2022b; Yuce et al., 2021; Tumskiy et al., 2023).
The Mpro monomer is structurally divided into three domains: domain-I (10–96) and domain-II (102–180) constitute the catalytic pocket, while domain-III (200–303) is a C-terminal α-helical domain linked to domain-II by a linker loop (183–197) (Jin et al., 2020). The catalytic dyad residues (H41 and C145) lie between domain-I and domain-II (Jin et al., 2020). Additionally, electrostatic and hydrophobic interactions between domain-III of two monomers enable Mpro homodimerization, which is critical for its proteolytic activity (Paciaroni et al., 2023; Ferreira et al., 2022). Mutation-induced variations in Mpro structural dynamics have been shown to alter kinetic parameters such as Km and the catalytic efficiency (kcat/Km) of the enzyme (Chen et al., 2023). For instance, the V186F (7.2% in the gamma variant) and A260V (3.7% in alpha and 5% in delta variants) Mpro mutants showed an increase in their kcat/Km, while two of the highly prevalent mutants K90R (99.8% in beta variant) and P132H (99.9% in omicron) showed an increase in the Km and a decrease in their catalytic efficiency (kcat/Km) (Chen et al., 2023). However, the K90RP132H double-mutant showed the opposite (a decrease in Km and an increase in kcat/Km) results compared to the single mutants (Chen et al., 2023). Furthermore, several Mpro mutations have been reported to affect Mpro thermal stability (Chen et al., 2023). For instance, structural analysis of the P132H mutant Mpro revealed that the residue H132 allosterically enhances the dynamic flexibility of the catalytic pocket’s entry site, which resulted in a reduction in the thermal stability of this mutant (Bhat et al., 2024). Carli et al. (2021) reported that the accessibility of the Mpro catalytic dyad residues is also correlated with some key interactions that are distant from the catalytic site, such as the interaction of residue E47 with residue L57 and the interaction of residue Y118 with residue N142 (Carli et al., 2021). Additionally, these allosteric interactions were present in some of the Mpro metastable states and absent in others (Carli et al., 2021). This may also suggest differences in the proteolytic activity among various Mpro metastable states (Carli et al., 2021). Furthermore, structural analysis of the Mpro metastable states revealed the formation and deformation of certain pockets relative to the catalytic site structure in some of the metastable states (Carli et al., 2021). This raises the possibility of targeting these potential allosteric interactions in Mpro using chemical ligands to inhibit its proteolytic activity and, thus, develop an anti-SARS-CoV-2 drug.
In the present study, we intended to identify metastable states of Mpro that exhibit (i) a deformed catalytic site and (ii) a well-formed allosteric site that could be targeted using chemical ligands. For the identification of this metastable state, we analyzed the binding affinity of metastable states to the Mpro N-terminal auto-cleavage peptide (TSAVLQSGFRK) (Xue et al., 2008) and selected two (amongst a total of 18) Mpro metastable states, namely, m2_c5 and m1_c11, that showed decreased binding affinity to the substrate peptide. A comparative analysis of the catalytic sites of the Mpro metastable states m1_c11 and m2_c5 with a reference Mpro X-ray crystal structure (PDB: 6Y84; https://www.rcsb.org/structure/6Y84) revealed that the Mpro metastable state m2_c5 has a deformed catalytic pocket. Subsequently, we analyzed its structure to identify potential allosteric sites that show high druggability. Following the identification of the potential allosteric sites, we performed an in silico screening of a library of 4.2 million drug-like compounds obtained from the ZINC15 database (Irwin and Shoichet, 2005), targeting the potential allosteric sites, and validated high-affinity binders through additional, blind computational docking and molecular dynamics (MD) simulation analysis. Finally, in vitro assays using our in-house BRET-based Mpro biosensor (Geethakumari et al., 2022a) revealed that one of the three high-affinity allosteric site binders inhibited Mpro activity with micromolar affinity.
Materials and methods
Protein–peptide docking
The Mpro N-terminal auto-cleavage peptide, TSAVLQSGFRK, was docked on the catalytic site of all 18 Mpro metastable states (Carli et al., 2021) using AutoDockFR (Ravindranath et al., 2015) (ADFR) software suite, a docking software application for flexible receptor and ligand docking. For this, we took advantage of the Mpro metastable states reported by Carli et al. (2021) recently. In brief, the authors analyzed a total of 20,000 conformers of Mpro obtained from a 100-µs-long MD simulation (D. E. Shaw Research, “Molecular Dynamics Simulations Related to SARS-CoV-2,” D. E. Shaw Research Technical Data, 2020. https://www.deshawresearch.com/downloads/download_trajectory_sarscov2.cgi/) (Carli et al., 2021) of a dimeric Mpro structure (10,000 conformers from each monomer) and identified 18 metastable states (11 metastable states from one monomer and 7 metastable states from the second monomer of the Mpro dimer). The peptide coordinates used for docking with ADFR were obtained from the peptide-Mpro complex structure (PDB: 2Q6G) (Xue et al., 2008). The output of protein–peptide docking consisted of the binding energies and binding pose of the docked peptide on the Mpro metastable states. The docked complexes were ranked based on their binding energies, and the high-affinity binding poses of the Mpro N-terminal auto-cleavage peptide with Mpro metastable states were analyzed using PyMOL (WL, 2002; Yuan et al., 2017).
Mpro allosteric site prediction
From protein–peptide docking, we selected the Mpro metastable states m1_c11 and m2_c5, one from each monomer of the Mpro dimer, with the lowest binding affinity for the Mpro N-terminal auto-cleavage peptide. Next, we performed a structural analysis of the catalytic sites of the two Mpro metastable states (m1_c11 and m2_c5) against the reference structure (PDB: 6Y84; https://www.rcsb.org/structure/6Y84) using PyMOL (WL, 2002; Yuan et al., 2017). The primary goal of this structural analysis was to identify the Mpro metastable state with the most significant structural deformation, which leads to the identification of the Mpro metastable states m2_c5 that showed major structural variations compared to the reference structure. Thereafter, we utilized the structure of the Mpro metastable state m2_c5 for the identification of potential allosteric sites using the DoGSiteScorer (Volkamer et al., 2012) software application, which is an automated pocket detection tool available on the ProteinsPlus server (https://proteins.plus/help/dogsite). The identified potential allosteric sites were then further analyzed using PyMOL (WL, 2002; Yuan et al., 2017) and were used for in silico screening of a library of drug-like molecules for the identification of potential Mpro allosteric site binders.
Ligand library preparation
The ZINC15 chemical compounds library of approximately 4.2 million was obtained from the ZINC15 database website with the tranches specification of 3D representation, standard reactivity, in stock, LogP value in the range of 2–4.5, and molecular weight ranging from 400 to 500 Da (https://zinc.docking.org/tranches/home/) (Irwin and Shoichet, 2005). The chemical compound library was downloaded in the PDBQT format and was used in the same format for in silico chemical compound library screening (Sterling and Irwin, 2015).
In silico screening
In order to identify potential Mpro allosteric site binders, we screened a library of approximately 4.2 drug-like chemical compounds obtained from the ZINC15 chemical compound library (Irwin and Shoichet, 2005). To screen the compounds, we performed a site-specific docking of the chemical compounds on the identified allosteric site in the Mpro metastable state m2_c5 using idock software (Li et al., 2012), which is a faster version of AutoDock Vina (Trott and Olson, 2010) and, thus, requires reduced computational resources. Ligands were ranked according to their predicted binding energies, and the top 400 compounds showing high energy binding to the potential allosteric site in the site-specific docking screen were further screened through blind docking using AutoDock Vina (Trott and Olson, 2010) software for their preference to bind to the identified allosteric sites in the Mpro metastable state m2_c5 structure. These led to the identification of three compounds, namely, ZINC11696924, ZINC12383815, and ZINC4497834, that were predicted to bind to the Mpro metastable state m2_c5 with high affinity and with a preference for the identified potential allosteric sites.
In silico physiochemical, ADME, and druglikeness prediction
To determine the efficacy and safety of the predicted potential Mpro allosteric binders ZINC11696924, ZINC12383815, and ZINC4497834, we determined their physicochemical properties, ADME, and druglikeness. These included features such as molecular weight, number of heavy atoms, aromatic heavy atoms, rotatable bonds, hydrogen-bond acceptors, hydrogen-bond donors, and topological polar surface area (TPSA), as well as pharmacokinetic properties like gastrointestinal absorption (GI absorption), blood–brain barrier permeability, P-glycoprotein substrate status, interactions with cytochrome P450 enzymes, and druglikeness as per Lipinski’s rule of five, the Ghose filter, Veber rules, and the bioavailability score. These parameters were predicted using the web tool SwissADME (http://www.swissadme.ch/) (Daina et al., 2017).
MD simulation and analysis
MD simulations of the Mpro metastable state m2_c5 in the apo and in complex with high-affinity allosteric binders (ZINC11696924, ZINC12383815, and ZINC4497834) were performed using NAMD 2.13 software (Phillips et al., 2005) using the CHARMM36 force field (Huang et al., 2017), largely as described previously (Geethakumari et al., 2022a; Jan et al., 2023b; Ahmed et al., 2022; Philip et al., 2023; Altamash et al., 2021; Uddin et al., 2024; Ahmed et al., 2024; Arshad et al., 2023). The topology and parameter files for the simulation were generated using the CHARMM-GUI online server (Jo et al., 2008). First, the complex was dissolved in an explicit solvent, employing the TIP3P cubic water box (Jorgensen et al., 1983). The box had a minimum distance of 10 Å between its edges and any of the atoms in the complex. Subsequently, 0.15 M NaCl was added to the solvated system. The simulation system had 149,117, 149,196, 149,199, and 149,187 atoms for the Mpro metastable state m2_c5 in apo and in complex with ZINC12383815, ZINC11696924, and ZINC4497834, respectively. Before the production run, the biomolecular simulation system was taken through energy minimization, thermal annealing, and equilibration, applying periodic boundary conditions as previously described. Subsequently, three independent 100 ns production simulations were run, with a time step of 2 fs, and trajectory frames were saved every 10,000 steps. For handling short-range non-bonded interactions, a 12 Å cut-off with a 10-Å switching distance was utilized. As for long-range non-bonded electrostatic interactions, the particle-mesh scheme at a 1 Å PME grid spacing was employed (Feller et al., 1995; Steinbach and Brooks, 1994; Essmann et al., 1995). Trajectory analysis was performed using the available tools in visual molecular dynamics (VMD) (Humphrey et al., 1996), including Cα atom root-mean-square deviation (RMSD) and root-mean-square fluctuation (RMSF) measurements. The free energy change of binding was estimated using the molecular mechanics Poisson–Boltzmann surface area (MM-PBSA) method (Kollman et al., 2000). This was achieved using the CaFE 1.0 (Liu and Hou, 2016) plugin in VMD (Humphrey et al., 1996). Hydrogen bond analysis was performed with a cut-off distance of 3.5 Å and an A-D-H angle of 20° using the “Hydrogen Bonds” analysis plugin in VMD (Humphrey et al., 1996). Dynamic cross-correlation (DCC) analysis based on the position of Cα atoms was performed using MD-task (Brown et al., 2017), a python script suite.
Expression and purification of SARS-CoV-2 Mpro
The BL21-CodonPlus (DE3) E. coli strain was chemically transformed with the SARS-CoV-2 Mpro bacterial expression plasmid, pETM33_NSP5_Mpro (Addgene #156475) (Mihalic et al., 2023). Transformed bacteria were grown in Luria broth (LB) media containing 50 μg/mL kanamycin and chloramphenicol overnight at 180 rotations per minute (rpm) and 37°C. The inoculum was transferred to the fresh LB media and incubated for 2 h at 37°C and 220 rpm, followed by protein expression induction using IPTG at a final concentration of 1 mM for 2.30 h at 37°C and 220 rpm. The cells were pelleted at 4,000×g at 4°C for 10 min and re-suspended in 10 mL lysis buffer [50 mM Tris (pH 8), 300 mM NaCl, 10 mM β-mercaptoethanol (β-ME), 1 mM PMSF, and 10% (v/v) glycerol], followed by sonication on ice for 15–30 min and centrifugation at 4,000× g at 4°C for 10 min. The supernatant was collected and centrifuged at 18,000×g at 4°C for 1 h. The supernatant was incubated with the GSH beads for 2 h, followed by washing with a buffer containing 50 mM Tris (pH 7), 150 mM NaCl, 10 mM β-mercaptoethanol (β-ME), 1 mM EDTA, 10% (v/v) glycerol, and 0.01% TritonX-100. The GSH beads containing Mpro were incubated with PreScission Protease (GE Healthcare # 27–0843–01) in cleavage buffer [50 mM Tris (pH 7), 150 mM NaCl, 1 mM EDTA, 1 mM DTT, 10% (v/v) glycerol, and 0.01% TritonX-100] for 16 h at 4°C. The supernatant containing SARS-CoV-2 Mpro obtained after centrifugation at 4°C and 500×g was aliquoted and stored at −80°C until further usage.
Cell culture and Mpro biosensor lysate preparation
To obtain cell lysates containing the Mpro biosensor for use in in vitro Mpro assays, HEK 293T cells were transfected with the pmNG-Mpro-Nter-auto-NLuc (Geethakumari et al., 2022b) plasmid DNA using polyethyleneimine (PEI) transfection, as described previously (Ahmed et al., 2024; Uddin et al., 2024; Altamash et al., 2021), (Biswas and Visweswariah, 2011), (Biswas et al., 2015), (Ahmed et al., 2024; Uddin et al., 2024; Altamash et al., 2021), (Biswas K. H. et al., 2008; Moovarkumudalvan et al., 2022; Geethakumari et al., 2022b). Cells were washed with cold Dulbecco’s phosphate-buffered saline (DPBS) 48 h post-transfection, and cells were lysed in a buffer containing 50 mM HEPES (pH 7.5), 50 mM NaCl, 0.1% Triton-X 100, 1 mM DTT, and 1 mM ethylenediamine tetraacetic acid (EDTA) (Grum-Tokars et al., 2008). Cell lysates were collected in a 1.5-mL Eppendorf tube and centrifuged at 4°C for 1 h at 14,000 rpm. The supernatant was collected and stored at −80°C for later usage.
In vitro, BRET-based Mpro proteolytic cleavage assay
The allosteric inhibitors at various concentrations (ranging from 10–4 to 10–13 M) were incubated with 2 μM of recombinantly purified SARS-CoV-2 Mpro for 2 h at 37°C in a buffer containing tris-buffered saline (TBS), 0.6 M sodium citrate, 1 mM EDTA, and 2 mM DTT, followed by the addition of cell lysates prepared from HEK293T cells expressing the Mpro biosensor (Geethakumari et al., 2022b). GC376 (GC376 sodium; AOBIOUS-AOB36447; stock solution prepared in 50% DMSO at a concentration of 10 mM) served as the control. Bioluminescence resonance energy transfer (BRET) measurements were performed at 37°C by the addition of furimazine (Promega, Wisconsin, United States) at a dilution of 1:200. The bioluminescence measurements at 467 and 533 nm were recorded using a Tecan SPARK multimode microplate reader and used to calculate BRET ratio (533 nm/467 nm). BRET ratios, obtained after 30 min of the initiation of the cleavage reaction through the addition of recombinant Mpro either in the absence or in the presence of increasing concentrations of the compounds, were fit to a sigmoidal dose–response curve to determine the IC50 values.
Data analysis and figure preparation
The output files from protein–peptide docking and protein–ligand docking were analyzed using a molecular visualization tool, PyMOL (Molecular Graphics System, Version 2.5.2, Schrödinger, LLC; pymol. org) (WL, 2002). For figure preparation, images were exported from PyMOL (WL, 2002) in a ray-traced, transparent background PNG format. Inkscape (Inkscape-1.1 version open-source software licensed under the GPL) and Microsoft PowerPoint software were used for assembling figures. GraphPad Prism (GraphPad Software, La Jolla, California, United States; www.graphpad.com), in combination with Microsoft Excel, was used for data analysis and graph preparation.
Results and discussions
Identification of an Mpro metastable state for potential allosteric targeting
In order to identify an allosteric site in the Mpro structure, we first attempted to identify a Mpro metastable with a deformed catalytic site such that it can no longer bind to its cleavage substrate peptides (Figure 1). For this, we took advantage of the structural analysis performed on a 100-µs-long MD simulation trajectory of Mpro reported by Carli et al. (2021). In brief, the authors analyzed a total of 20,000 configurations of Mpro obtained from the 100 µs-long MD simulation trajectory (D. E. Shaw Research, “Molecular Dynamics Simulations Related to SARS-CoV-2,” D. E. Shaw Research Technical Data, 2020. https://www.deshawresearch.com/downloads/download_trajectory_sarscov2.cgi/) (Carli et al., 2021) of a dimeric Mpro structure (10,000 configurations from each monomer) and identified a total of 18 metastable states (11 from monomer 1 and 7 from monomer 2; monomers were referred to as either m1 or m2). This high-dimensional analysis involved the calculation of the ψ backbone-dihedral distance and the mobile contact map distance to monitor variations in the protein backbone and the side chain rearrangements, respectively. The selected configurations, based on ψ backbone-dihedral distance and the mobile contact map distance, were used for free energy estimation. Furthermore, the authors defined a metastable state as a group of configurations with similar free energy minima that persisted for tens of nanoseconds throughout the 100-µs-long MD simulation trajectory (Carli et al., 2021).
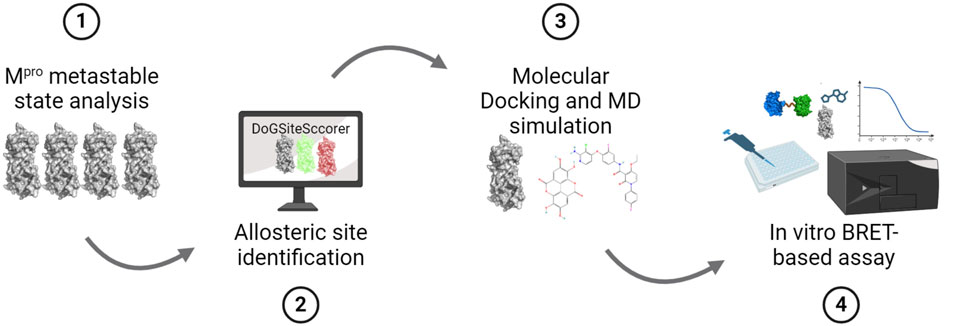
Figure 1. SARS-CoV-2 Mpro allosteric inhibitor discovery pipeline. Schematic representation showing the stepwise methodology employed in this study that includes (1) analysis of the Mpro metastable states to identify those that contain a deformed catalytic site such that it cannot bind the substrate peptide, (2) identification of allosteric sites in the selected Mpro metastable state, (3) performing in silico drug screening to identify potential ligands for the identified allosteric sites and perform MD simulation of top-scoring ligands to determine the stability of their binding, and (4) performing in vitro Mpro cleavage assay using a BRET-based biosensor to determine the inhibitory potency of identified allosteric site ligands.
To identify an Mpro metastable state with a deformed catalytic site, we performed protein–peptide docking using ADFR software (Ravindranath et al., 2015) to examine the binding affinity of all 18 metastable states for the Mpro N-terminal autocleavage substrate peptide (TSAVLQSGFRK) (Xue et al., 2008). We posited that differences in the binding energies, determined through the docking study, would provide insights into the differences in the catalytic site structures in these metastable states (Ferreira et al., 2021). These docking simulations revealed two metastable states, namely, m2_c5 and m1_c11, that showed markedly reduced predicted binding affinities for the Mpro substrate peptide (−12.1 and −10.7 kcal/mol, respectively) compared to that of all other metastable states (values ranging from 13.4 to 17.4 kcal/mol) (Figure 2).
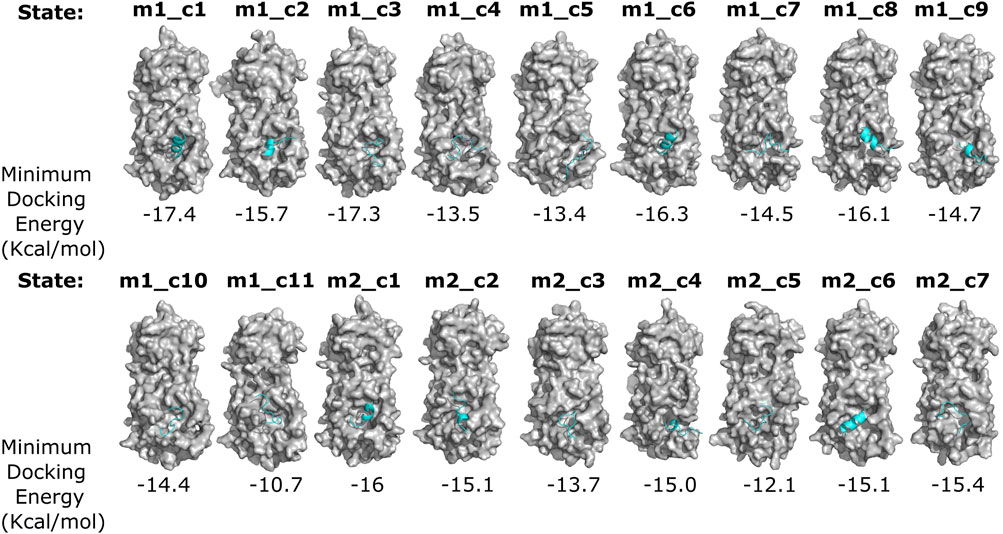
Figure 2. Differential binding affinity of the N-terminal Mpro autocleavage site peptide for various Mpro metastable states. Surface representation of the indicated Mpro metastable states (gray) and docked N-terminal autocleavage site peptide (cyan) obtained from flexible receptor–ligand docking analysis using the ADGFR suite (Ravindranath et al., 2015). Minimum docking energies (for the top-scoring poses) are indicated below each complex. Note the differential binding modes, secondary structure, and binding energies of the N-terminal autocleavage site peptide with various Mpro metastable states.
The catalytic sites of these two states were then analyzed using PyMOL (WL, 2002; Yuan et al., 2017) to determine any differences in the position of the catalytic site residues compared to the Mpro reference protein structure (PDB: 6Y84; https://www.rcsb.org/structure/6Y84) (Figure 3A). The Mpro metastable state m1_c11 showed a reversed orientation of the side chains of residues C145 and N142. The residue C145 is a part of the catalytic dyad (residue C145 and residue H41) of Mpro19,45 and is critical for the proteolytic activity of the protein (Figure 3B). Additionally, residue N142 is reported to form vdW interactions with the glutamine residue (Q) of the substrate peptide (AVLQSGFR) (Shaqra et al., 2022). The Mpro metastable state m2_c5 also showed a change in the side chain orientation of residue Q189 (Figure 3B). However, no discernable difference in the position of residues T25, G143, and E166 was observed. The Mpro metastable state m2_c5, on the other hand, showed a difference in the Cα atom positions and alterations in the side chain orientations of the catalytic site residues. Furthermore, the distances between residues T25, H41, C145, and E166 were observed to be decreased, while residues N142 and G143 appeared to be distant from these catalytic site residues. Importantly, residue Q189 showed a shift in its location and appeared to move away from the catalytic site, with its side chain pointing away from the bulk of the protein (Figure 3C). The side chain of residue Q189 is critical for forming a cavity that positions the P2 residue of substrate peptide, leucine (L), in case of N-terminal Mpro substrate cleavage site (NSP4-5 = AVLQSGFR) (Shaqra et al., 2022). The side chain and the backbone of residue Q189 have been reported to form van der Waals (vdW) and hydrogen bond (H-bond) interactions with all Mpro substrate cleavage site peptides except for NSP14-15 (not reported for NSP14-15 as of yet) (Chan et al., 2021; Shaqra et al., 2022).
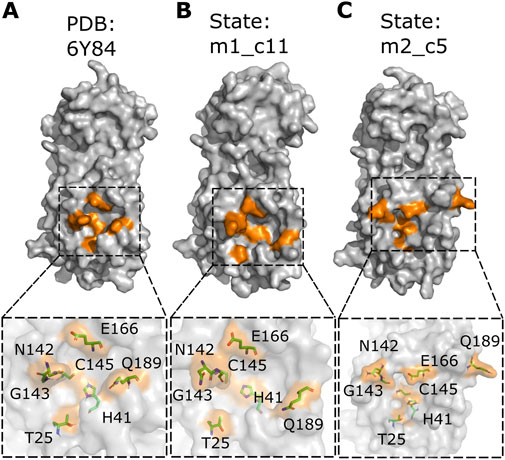
Figure 3. Altered position and orientation of catalytic site residues in the Mpro metastable states m1_c11 and m2_c5. (A–C) Surface representation of the Mpro crystal structure (PDB: 6Y84; https://www.rcsb.org/structure/6Y84) (A) and metastable states m1_c11 (B) and m2_c5 (C) highlighting the catalytic site residues in orange. Outsets show the location and orientation of the catalytic site residues (both side chain and main chain atoms) in the stick representation in the indicated complexes. Note the prominent alteration in the position of catalytic site residue Q189 in the Mpro metastable states m1_c11 (B) and m2_c5 (C). The structural analysis was performed using PyMOL (WL, 2002; Yuan et al., 2017).
Based on the structural differences discussed above, we selected the Mpro metastable state m2_c5 as a metastable state having a deformed catalytic site for further studies. Next, we attempted to find a potential druggable allosteric site on this metastable state using the DoGSiteScorer tool (Volkamer et al., 2012; Volkamer et al., 2010), which is an automated pocket detection and analysis tool to predict the potential binding pockets and sub-pockets in protein structures (Volkamer et al., 2012). We selected potential binding sites based on the drug score (Volkamer et al., 2012), an output parameter with values ranging from 0 to 1, which provides an estimate of the druggability of the predicted sites (the higher the score, the more druggable the site is) (Volkamer et al., 2012). The druggability or drug score is calculated for each (sub)pocket using the linear combination of the three descriptors describing volume, hydrophobicity, and enclosure (Volkamer et al., 2012). Initially, the site with the highest drug score was selected as the putative allosteric site, which we named as allosteric site 1a. The allosteric site 1a contained 38 residues (list of residues is given in Table 1) with the drug score of 0.83, enclosure (ratio of site hull to surface grid points) of 0.09, and a depth of 26.21 Å (Figure 4A; highlighted in orange). In addition to this site, another site was detected by DoGSiteScorer (Volkamer et al., 2012), which we named allosteric site 1b. Located near the allosteric site 1a, this was of a relatively small size and had a low drug score (0.26) but had a high polar residue ratio of 0.56. In the subsequent in silico screening, we utilized a relatively large grid box that included allosteric sites 1a and 1b (together formed the allosteric site 1) to ensure a maximum number of hit identifications (Figure 4A; highlighted in turquoise). Importantly, we note that some of the residues in the predicted allosteric site 1 (residues G29, L115, R131, G149, D197, and E290) have been reported to be sensitive to mutation for the Mpro proteolytic activity (Flynn et al., 2022).

Table 1. Druggability analysis of the potential allosteric sites in Mpro. Table showing the results of the druggability analysis and size and shape descriptors of the two potential allosteric sites predicted in the Mpro metastable state m2_c5 using DoGSiteScorer (Volkamer et al., 2012).
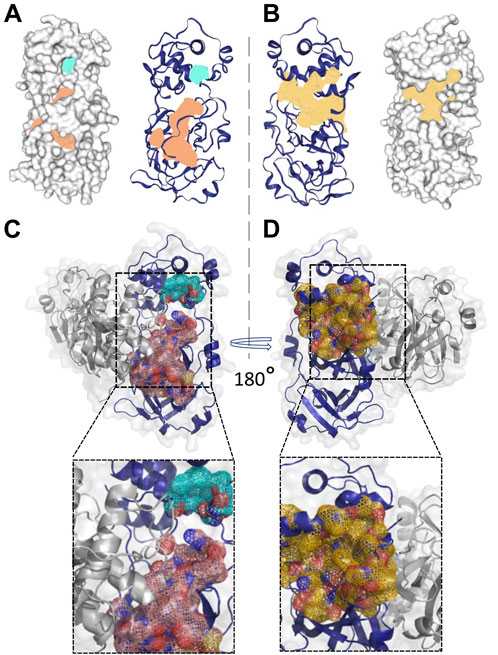
Figure 4. Location and shape of the two predicted potentially druggable allosteric sites in the Mpro metastable state m2_c5. (A, B) Surface (left panel) and cartoon (right panel) representation of two potential druggable allosteric sites in the Mpro metastable state m2_c5 with the shaded area representing allosteric site 1 (light orange, allosteric site 1 and light sea green, allosteric site1′) (A) and allosteric site 2 (pale yellow, allosteric site 2) (B) between domain II and domain III of m2_c5. (C, D) Cartoon and surface representations of the Mpro dimer structure showing the position of the potentially druggable allosteric sites 1 (C) and 2 (D). Outsets in C and D show zoomed-in views of the potentially druggable allosteric sites 1 and 2 in the Mpro dimer structure. The allosteric site 1 is partly located at the dimer interface, while allosteric site 2 is located opposite to the dimer interface of Mpro.
In addition to the allosteric site 1, the DoGSiteScorer (Volkamer et al., 2012) analysis also predicted another potential allosteric site, which we named allosteric site 2, which showed the second-highest drug score of 0.8. This site comprises 30 residues (the list of residues is provided in Table 1) (Figure 4B; highlighted in pale yellow). Some of the key residues of the allosteric site 2, such as residues I200 and H246, were also reported by Günther et al. (2021) to play a role in the allosteric regulation of Mpro proteolytic activity. However, the Mpro metastable state m2_c5 appeared to be more accessible with much less enclosure (0.16) compared to the previously reported site (enclosure: 0.72) at the same location (Alzyoud et al., 2022).
Additionally, we determined the location of the predicted allosteric sites in the Mpro dimer to ensure that these sites are accessible for ligand binding in the dimeric structure of Mpro, which is the catalytically competent form of the protein (Jin et al., 2020). This analysis revealed a relatively small portion of the allosteric site 1a to be located at the Mpro dimer interface.
Overall, the allosteric site 1 appeared to be accessible for ligand binding in the dimer form of Mpro (Figure 4C). On the other hand, the allosteric site 2 was located opposite to the dimer interface, making it completely accessible for ligand binding in the Mpro dimer (Figure 4D).
Identification of high-affinity compounds targeting the potential allosteric site
Having determined a metastable state with a deformed catalytic site and a potential allosteric ligand binding site, we performed an in silico screening of a drug-like compound library (∼4.2 million) obtained from the ZINC15 database (Irwin and Shoichet, 2005). For this, we first performed site-specific docking using the idock algorithm (Li et al., 2012) with grid parameters specified for allosteric site 1 in Mpro to identify potential binders for this site. Following in silico screening, we selected 400 top-ranking compounds showing binding energies lower than −9 kcal/mol and proceeded with a step of blind docking using AutoDock Vina (Trott and Olson, 2010) to determine whether these compounds prefer binding to the predicted allosteric sites. Following the docking, we analyzed the binding poses of all the 400 compounds using PyMOL (WL, 2002;Yuan et al., 2017) and found them to bind to three distinct sites on Mpro. These were the catalytic site and the potential allosteric sites 1 and 2. We then applied a selection criterion wherein we selected compounds that showed binding to the allosteric sites more than once in the nine binding poses generated in the blind, AutoDock Vina docking runs. Finally, we identified three compounds, namely, ZINC11696924, ZINC12383815, and ZINC4497834, having high affinity for the predicted allosteric sites and further analyzed them for their binding to the potential allosteric sites in Mpro.
First, the compound ZINC11696924 was found to bind to the allosteric site 2 in five docking poses and to the allosteric site 1 in only one docking pose out of nine docking poses. The top-ranking binding pose with a binding affinity of −9.9 kcal/mol showed polar interactions of ZINC11696924 with residues H246 and T292 in allosteric site 2 (Figure 5A). The residue H246 is one of the important histidine residues of Mpro and is crucial for the stability of the Mpro structure (Pavlova et al., 2021). On the other hand, the residue T292 has an allosteric effect on the catalytic activity of Mpro as mutation at this position with positively charged residues results in the loss of Mpro activity (Flynn et al., 2022).
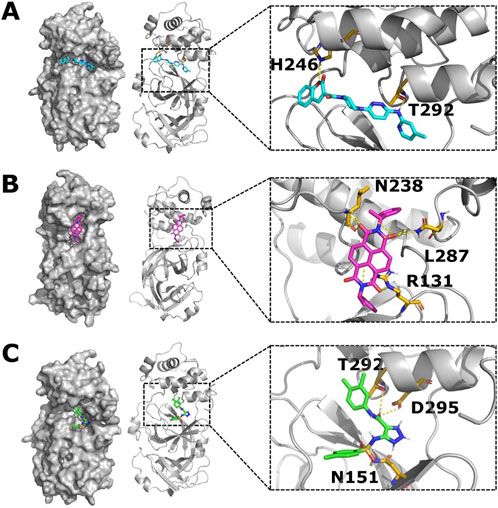
Figure 5. Top-ranking ligands (ZINC11696924, ZINC12383815, and ZINC4497834) are predicted to bind to the potential allosteric sites in the Mpro metastable state m2_c5. (A–C) Surface (left panel) and cartoon (right panel) representation of the Mpro metastable state m2_c5 showing docked ligands ZINC11696924 (A), ZINC12383815 (B), and ZINC4497834 (C). Outsets show polar interactions between the ligands and Mpro residues identified using PyMOL (WL, 2002; Yuan et al., 2017) software (ZINC11696924 interacts with residues H246 and T292; ZINC12383815 interacts with residues N238, L287, and R131; and ZINC4497834 interacts with residues T292, D295, and N151) in the respective complexes.
The second compound ZINC12383815 showed binding to the allosteric site 1 in two out of the nine docking poses. It showed polar interactions with residues R131, N283, and L287 of Mpro with a top affinity of −10.0 kcal/mol (Figure 5B). Out of these three residues that showed an interaction with ZINC12383815, residue R131 plays a critical role in the structural plasticity and flexibility of the Mpro structure due to the formation of a salt bridge with residue D289 (Flynn et al., 2022; Bhat et al., 2022a).
The third compound ZINC4497834 showed binding to the allosteric site 2 in five out of the nine docking poses and revealed polar interactions with residues N151, T292, and D295 in Mpro with the top predicted affinity of −8.2 kcal/mol (Figure 5C). The residue D295 is one of the salt bridge-forming residues, and any substitution at this position leads to a complete loss of Mpro activity (Flynn et al., 2022; Kaptan et al., 2022). Additionally, it has been reported that the Mpro nanobody, N2B4, which acts as an allosteric inhibitor of the protease, also interacts with the residue D295 (Sun et al., 2022). Together, these suggest that the compound ZINC4497834 can allosterically impact the catalytic activity of Mpro, given that it appears to bind to the potential allosteric site.
Overall, our in silico screening led to the identification of three potential high-affinity binders of the potential allosteric sites in Mpro. ADME profiles of these three compounds, as predicted by SwissADME, were found to be suitable. In addition, the three compounds showed a good pharmacokinetic profile and druglikeness (Table 2). Although the compound ZINC4497834 did not appear to violate any druglikeness criteria with a good pharmacokinetic profile, the compounds ZINC11696924 and ZINC12383815 appeared to violate one of Lipinski’s rule and also appear to inhibit a few of the cytochrome-P450 enzymes (Table 2). The chemical structures of ZINC11696924, ZINC12383815, and ZINC4497834 are given in Supplementary Figure S1.
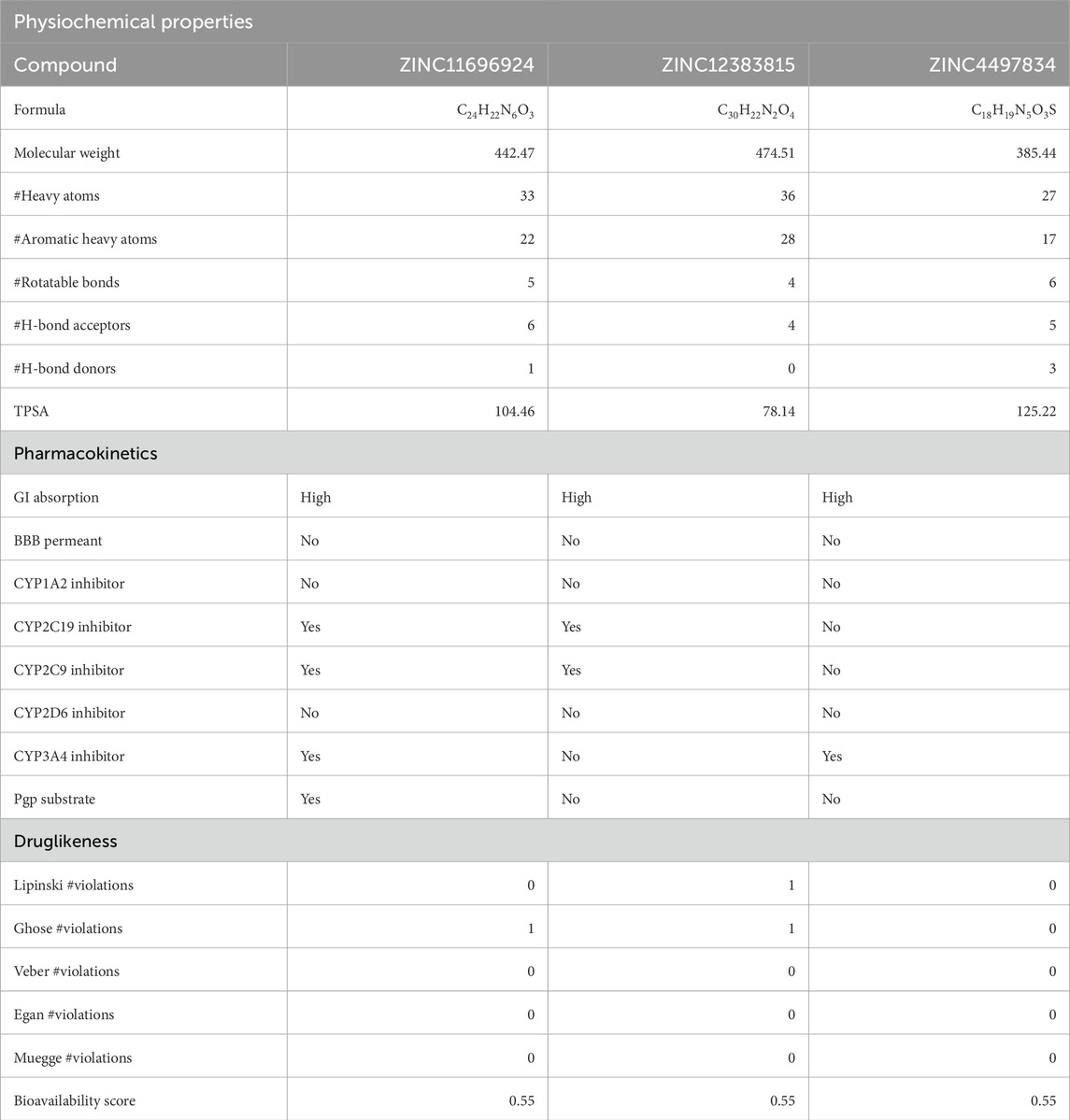
Table 2. Physiochemical properties, pharmacokinetics, and druglikeness of the top-ranking ligands. Table showing physiochemical properties, pharmacokinetics, and druglikeness of the top-ranking ligands predicted using the SwissADME web tool (Daina et al., 2017). TPSA, topological polar surface area; GI absorption, gastrointestinal absorption, BBB permeation, blood–brain barrier permeation; Pgp substrate, P-glycoprotein substrate.
Stable binding of ZINC11696924, ZINC12383815, and ZINC4497834 to the potential allosteric sites in Mpro
To investigate the binding stability of the three identified compounds to their respective allosteric sites, we performed three independent 100 ns, all-atom, explicit solvent, MD simulations of the apo Mpro metastable state m2_c5 and Mpro metastable state m2_c5 in complex with high-affinity allosteric binders, using NAMD2 (Phillips et al., 2005). Analysis of the MD trajectories showed that the ligands were retained in the respective allosteric sites across the 100 ns simulation time (Figure 6A). However, the change in orientation of the compounds was observed in the respective allosteric sites (Figure 6A). Additionally, RMSD analysis showed a general decrease in the structural dynamics of the protein as a result of ligand binding to the allosteric site (Figure 6B). Specifically, RMSF analysis of the Mpro metastable state m2_c5 bound with the compound ZINC11696924 showed an increase in the flexibility of a region in the middle of domain I (residues ranging from 46 to 51) and at the end of domain II (residues ranging from 178 to 199), respectively. The residues ranging from 46 to 51 are a part of the S2 subsite of the Mpro catalytic site (Parmar et al., 2022). Moreover, the end of the domain II (residues ranging from 178 to 199) region contains an alpha helix and a loop that connects domain II to domain III (Parmar et al., 2022) of the Mpro metastable state m2_c5, and the observed increase in the flexibility of this region may affect the catalytic activity of Mpro. On the other hand, the Mpro metastable state m2_c5 in complex with ZINC12383815 showed a decrease in RMSF values in the region containing residues ranging from C44 to D56. We note that the residue S46 has been shown to interact with the substrate peptide, while the residue M49 has been reported to form the ‘lid’ of the S2 hydrophobic subsite in the Mpro catalytic site (Parmar et al., 2022). Finally, the Mpro metastable state m2_c5 bound with the compound ZINC4497834 showed an overall increase in the RMSF values compared to that of the apo Mpro metastable state m2_c5 (Figure 6C), except for a decrease in the RMSF values of residues ranging from T45 to L50. Importantly, there was an increase in the flexibility of a region encompassing residues ranging from T135 to C145, which contains two catalytic site residues N142 and G143 and one Mpro catalytic dyad (Puhl et al., 2019) residue C145 (Figure 6C). The observed RMSF differences in the Mpro metastable state in apo and in complex with the compounds ZINC11696924, ZINC12383815, and ZINC4497834 suggest an altered structural dynamics of the Mpro metastable state m2_c5 upon binding to potential allosteric binders. Contrary to the differences observed in the RMSF analysis, intra-protein hydrogen bond (H-bond) analysis did not reveal any large differences with the average number of H-bonds of 69 ± 4, 69 ± 4, 67 ± 4, and 67 ± 4 for the apo Mpro metastable state m2_c5 and those that are bound to ZINC11696924, ZINC12383815, and ZINC4497834, respectively (Figure 6D).
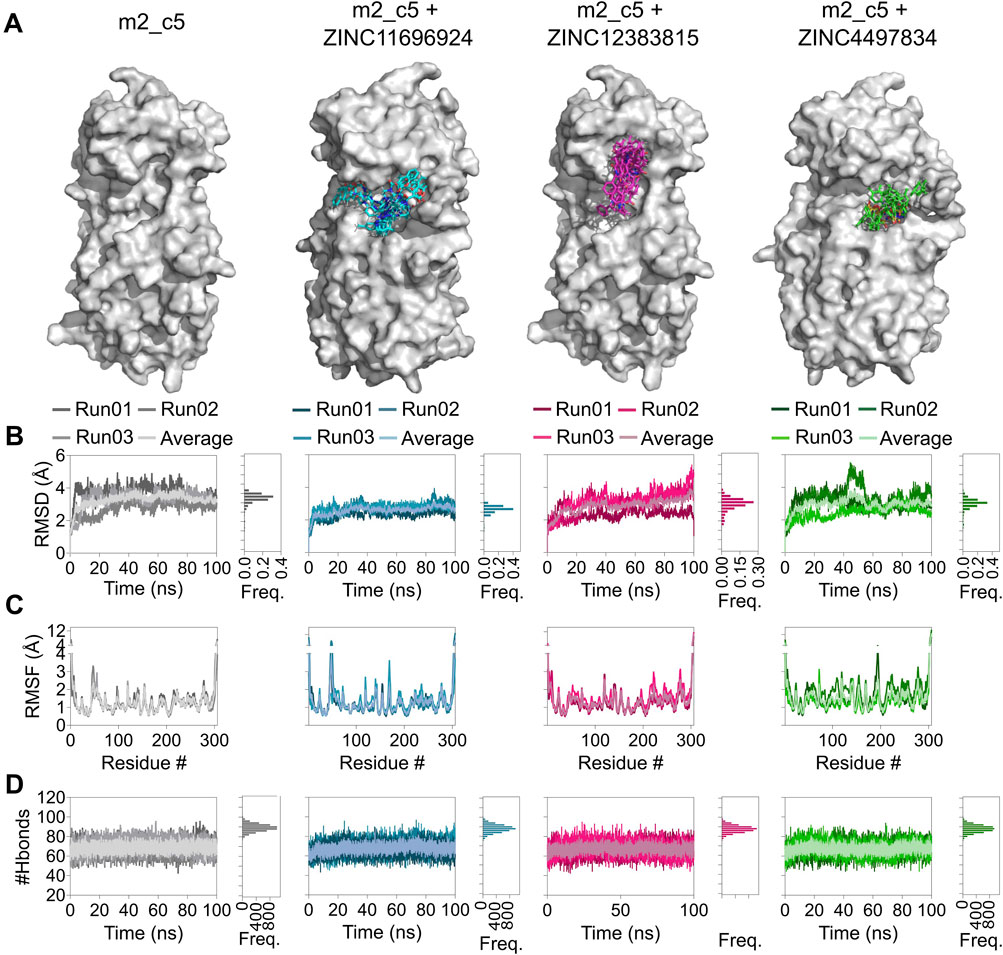
Figure 6. Altered structural dynamics of the Mpro metastable state m2_c5 in complex with the potential allosteric site binders ZINC11696924, ZINC12383815, and ZINC4497834. (A) Surface representation of the Mpro metastable state m2_c5 in the absence of ligand or in the presence of bound ligands ZINC11696924 (cyan), ZINC12383815 (magenta), and ZINC4497834 (green) showing orientations of the ligands obtained from 100-ns MD simulations, captured every 10 ns. (B) Graphs showing RMSD values (left panel) and frequency distribution of the RMSD values (right panel) of the Mpro metastable state m2_c5 in the absence of any ligand and in complex with ligands ZINC11696924 (cyan), ZINC12383815 (magenta), and ZINC4497834 (green) obtained from 100 ns MD simulations. (C) Graphs showing RMSF values of the Mpro metastable state m2_c5 in the absence of any ligand and in complex with ligands ZINC11696924 (cyan), ZINC12383815 (magenta), and ZINC4497834 (green) obtained from 100 ns MD simulations. (D) Graphs showing the number of H-bonds in the Mpro metastable state m2_c5 in the absence of any ligand (apo, gray) and in complex with ligands ZINC11696924 (cyan), ZINC12383815 (magenta), and ZINC4497834 (green). Data shown are obtained from three independent, 100 ns long, all-atom, explicit solvent MD simulations.
We then assessed the distance of the compounds from their interacting residues to determine if they remained bound to the potential allosteric sites over the 100 ns trajectories (Figure 7A). Overall, this analysis revealed that all three compounds remained bound to their allosteric sites in Mpro. A closure inspection revealed that the distance of the compound ZINC11696924 with its interacting residues H246 and T292 increased from 10 Å, measured at the beginning of the simulation, to approximately 13 Å over time (mean ± SD, 13 ± 2 Å) (Figure 7B). However, the distance of the compound ZINC12383815 with its interacting residues N238, L287, and R131 largely remained the same, with some increases observed during the initial stages of the simulations (27 ± 3 Å) (Figures 7C, D). On the other hand, the distance of the compound ZINC4497834 with its interacting residues N151, T292, and D295 decreased during the course of 100 ns MD simulations (8 ± 1 Å) (Figures 7E, F).
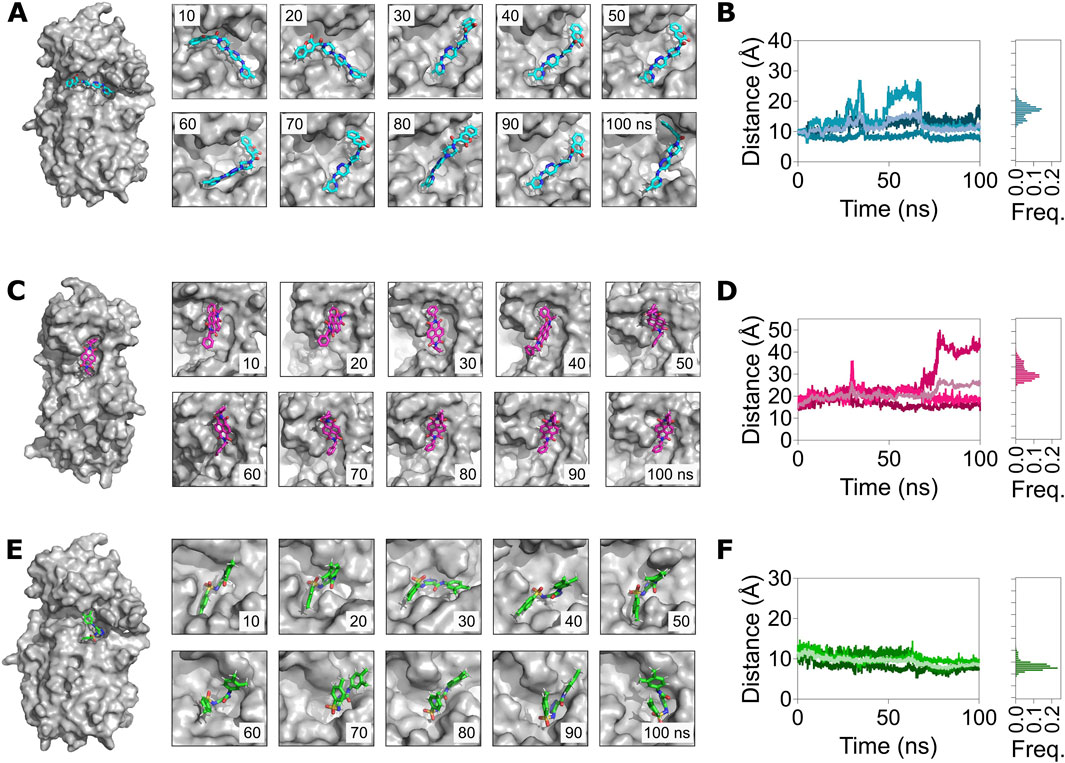
Figure 7. MD simulations reveal a stable binding of the allosteric site binders ZINC11696924, ZINC12383815, and ZINC4497834 with the predicted allosteric sites in the Mpro metastable state m2_c5. (A,C,E) Left panels: surface representation of the Mpro metastable state m2_c5 bound to ZINC11696924 (A), ZINC12383815 (C), and ZINC4497834 (E). Right panels: snapshots of the allosteric site complex with ligands ZINC11696924 (A), ZINC12383815 (C), and ZINC4497834 (E) captured every 10 ns of a 100 ns MD simulation trajectory. (B,D,F) Left panels: graphs showing the center-of-mass distance between ligand interacting residues in the pocket (H246 and T292) and ZINC11696924 (A), residues (N238, L287, and R131) and ZINC12383815 (C), and residues (N151, T292, and D295) and ZINC4497834 (E). Right panels: frequency distribution of the measured distance of the respective Mpro metastable state m2_c5 with allosteric binders. Data shown are obtained from three independent 100 ns long, all-atom, explicit solvent MD simulations.
Having found a generally stable binding of the compounds to the Mpro metastable state m2_c5, we then analyzed the MD simulation trajectories for any changes in correlated motions of the residue in the protein upon binding the potential allosteric binders using the dynamic cross-correlation (DCC) algorithm available as a part of the MD-TASK suite of Python scripts (Brown et al., 2017). This analysis revealed a general reduction in cross-correlated motions between residues in the intra-domain regions of the Mpro metastable state m2_c5 in the presence of all three potential allosteric binders (Figure 8). Specifically, the DCC values of residues in domain I (highlighted using pink boxes) and domain II (highlighted using blue boxes) were high in the apo Mpro metastable state m2_c5 but showed a reduction when the protein was bound to either of the three compounds ZINC11696924, ZINC12383815, and ZINC4497834 (Figures 8A–D), suggesting that the binding of these ligands results in a decrease in correlated motions in the individual domains of the protein. Aside from the intra-domain cross-correlation, residues in domain III showed a negative cross-correlation with residues in domains I and II. Interestingly, these negatively correlated motions appeared to be decreased in the presence of the compound ZINC11696924 (Figures 8A, B). Similarly, a reduction in the negatively correlated motions of residues in domain III with those in domain II was observed in the presence of the compounds ZINC12383815 and ZINC4497834 (Figures 8A, C; highlighted using red boxes). Additionally, an increase in the negatively correlated motions was observed between residues in domain III with those in domain II in the presence of compound ZINC4497834 compared to the apo Mpro metastable state m2_c5 (Figures 8A, D; highlighted using green boxes). Overall, these results are suggestive of an effect of these potential allosteric binders on the structural dynamics of the Mpro metastable state m2_c5 that might lead to an alteration in the Mpro proteolytic activity.
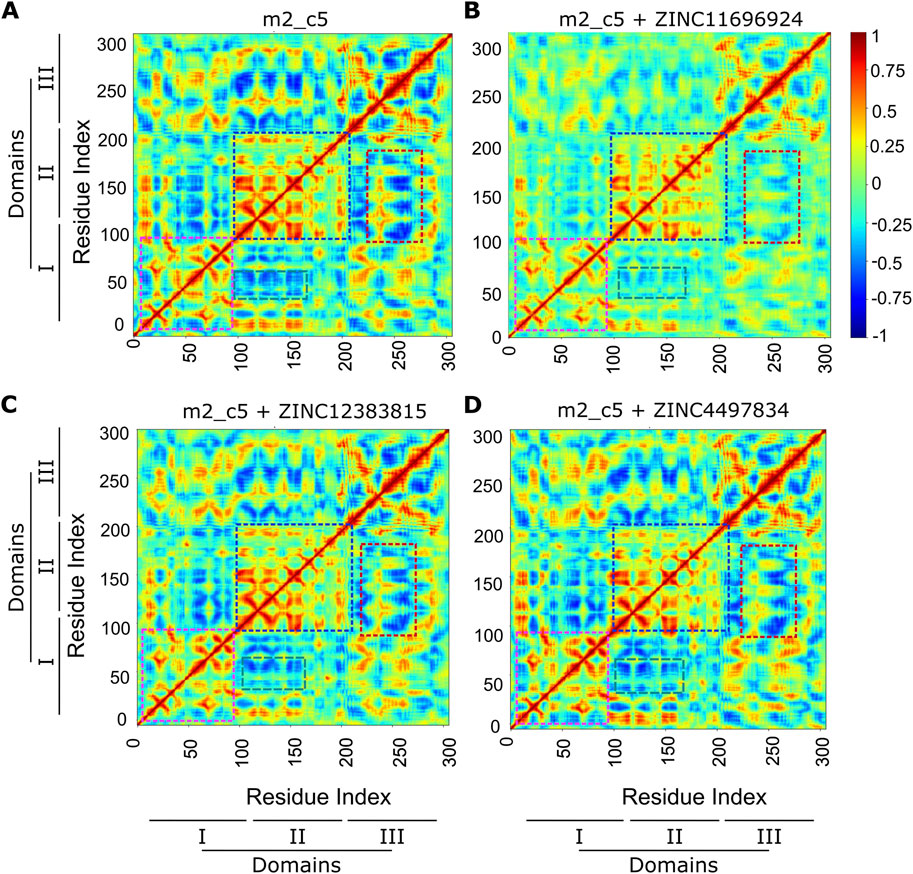
Figure 8. Altered cross-correlated motions of the Mpro metastable state m2_c5 in complex with ligands ZINC4497834, ZINC11696924, and ZINC12383815. (A–D) Heat maps showing average inter-residue dynamic cross-correlation (DCC) values of the Mpro metastable state m2_c5 in the apo (A) and in complex with ZINC11696924 (B), ZINC12383815 (C), and ZINC4497834 (D) obtained from three independent, 100 ns long MD simulations. Regions showing differences in DCC between the Mpro metastable state m2_c5 in the apo (A) and in complex with ZINC11696924 (B), ZINC12383815 (C), and ZINC4497834 (D) are highlighted with color boxes. Pink boxes, DCC of Mpro domain I residues; blue boxes, DCC of Mpro domain II residues; red boxes, DCC between Mpro domain III and II residues; green boxes, DCC between Mpro domain II and I residues.
Next, we determined the binding affinity of the potential allosteric binders through the calculation of free energy change of binding of the compounds ZINC11696924, ZINC12383815, and ZINC4497834 with the Mpro metastable state m2_c5 using MD simulation trajectories and the CaFE 1.0 tool (Liu and Hou, 2016) in conjunction with VMD (Humphrey et al., 1996). This analysis revealed generally high-affinity binding of the potential allosteric compounds with free energy change of binding (∆G) values of −11 ± 4, −13 ± 2, and −16 ± 3 kcal/mol for ZINC11696924, ZINC12383815, and ZINC4497834, respectively (Figure 9).
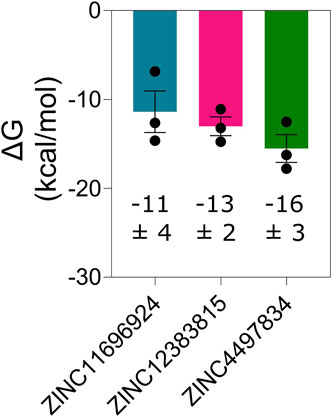
Figure 9. Mpro metastable state m2_c5 binds ligands ZINC11696924, ZINC12383815, and ZINC4497834 at the allosteric site with high affinity. Graph showing free energy change of binding (ΔG) of the Mpro metastable state m2_c5 with the indicated predicted allosteric site binders. Data shown are mean ± SD obtained from three independent, 100 ns long MD simulations determined at an interval of 20 ns.
ZINC4497834 inhibits the Mpro proteolytic activity in vitro
Having established stable and high-affinity binding of the three potential allosteric binders using computational methods, we aimed to determine the impact of these compounds (ZINC11696924, ZINC12383815, and ZINC4497834) on the proteolytic activity of Mpro in an in vitro assay. For this, we utilized our recently reported BRET-based Mpro biosensor (Geethakumari et al., 2022a). The biosensor consists of mNG (BRET acceptor) on the N-terminal side, Mpro N-terminal auto-cleavage sequence (AVLQSGFR) in the middle, and NLuc luciferase (BRET donor) on the C-terminal side. It shows high BRET in the absence of Mpro activity due to the proximity of NLuc and mNG, while it shows a reduction in BRET in the presence of Mpro activity due to the physical separation of NLuc and mNG (Figure 10A). We incubated lysates prepared from HEK 293T cells expressing the biosensor with a recombinantly purified Mpro with increasing concentrations of the potential allosteric compounds and monitored BRET after addition of the NLuc luciferase substrate (Figure 10). Incubation of Mpro with the known Mpro covalent inhibitor, GC376 (Geethakumari et al., 2022a; Sharun et al., 2021; Lu et al., 2022; Luan et al., 2023), resulted in a concentration-dependent decrease in Mpro activity with an IC50 value of 4.3 ± 5.5 µM (Figure 10B). Importantly, incubation of Mpro with the compounds ZINC11696924 and ZINC12383815 did not show any notable decrease in its activity (albeit the compound ZINC11696924 appeared to show some increase in Mpro activity at lower concentrations) (Figures 10C, D). On the other hand, incubation of Mpro with the compound ZINC4497834 resulted in a concentration-dependent decrease in its activity with an IC50 value of 43 ± 39 µM (Figure 10E), which is similar to the IC50 value (25.16 µM) of the recently reported allosteric inhibitor AT7519 (Günther et al., 2021). Importantly, AT7519 (Günther et al., 2021) was shown to bind to allosteric site 2, which is also the site where the compound ZINC4497834 was found to be stably bound in our computational studies (Figure 10E) (Günther et al., 2021). Together, these results suggest that the compound ZINC4497834 inhibits SARS-CoV-2 Mpro, likely through an allosteric mechanism.
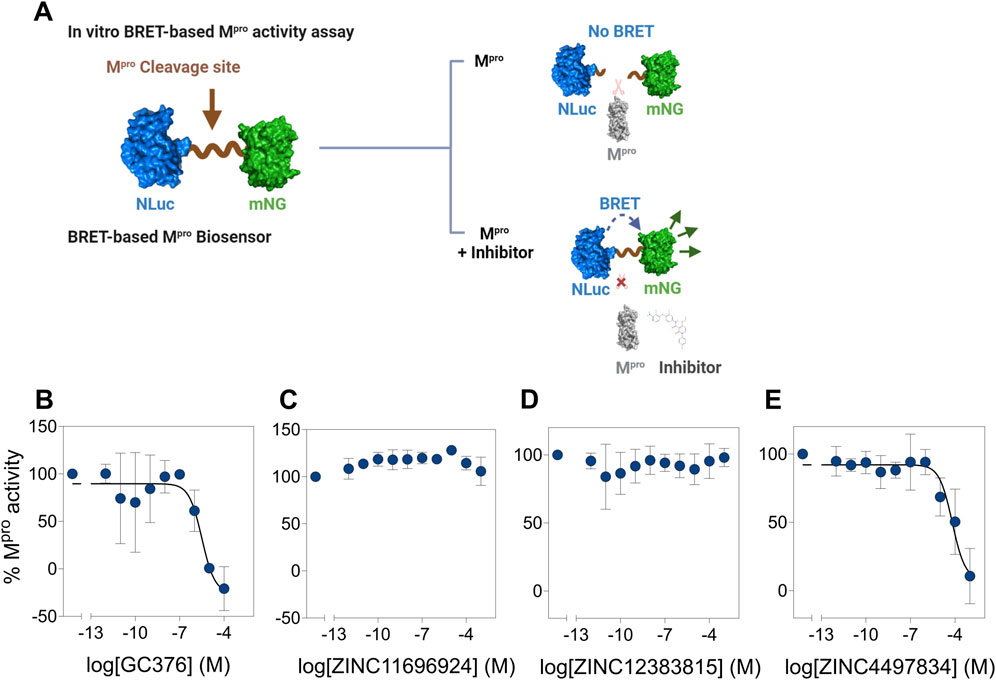
Figure 10. Potential allosteric site binding ligand ZINC4497834 inhibits Mpro activity in vitro. (A) Schematic representation showing the working of a BRET-based Mpro biosensor containing the Mpro N-terminal autocleavage sequence between mNG (BRET acceptor) and NLuc (BRET donor). The biosensor shows high BRET (ratio of mNG fluorescence and NLuc bioluminescence) in the absence of Mpro, while Mpro-mediated cleavage results in the decrease in the BRET due to physical separation of mNG and NLuc. However, in the presence of an inhibitor, cleavage of the biosensor will be abrogated and result in the maintenance of high BRET. (B–E) Graphs showing the percentage Mpro proteolytic cleavage activity at various concentrations of the covalent inhibitor GC376 (B) and potential allosteric ligands ZINC11696924 (C), ZINC12383815 (D), and ZINC4497834 (E). All BRET ratios in a given experiment were first normalized with those obtained in the absence of Mpro, and the normalized BRET ratios were used for determining % Mpro activities. Data shown are mean ± SD obtained from two (B) or three (C–E) independent experiments.
After establishing high-affinity binding and subsequent inhibition of Mpro activity by the compound ZINC4497834 in the BRET-based in vitro assay (Figures 5C, 6, 7E), we aimed to explore the probable mechanism underlying this inhibition. For this, we first revisited the MD simulation trajectories and analyzed the difference in the average RMSF values of each residue in the apo- and compound ZINC4497834-bound form of the Mpro metastable state m2_c5. This analysis revealed an overall increase in the fluctuations of all the residues of the Mpro metastable state m2_c5 bound with ZINC4497834 (Figures 11A). Importantly, five catalytic site residues T25 (p = 0.043), H41 (p = 0.013), N142 (p = 0.012), G143 (p = 0.039), and C145 (p = 0.020) showed a significant increase in the RMSF in the Mpro metastable state m2_c5 bound with ZINC4497834 (Figure 11B). Interestingly, however, there was no significant difference in the fluctuations of the ZINC4497834-interacting allosteric site 2 residues N151, T292, and D295 in the apo and ZINC4497834-bound Mpro metastable state m2_c5 (Figure 11B). Second, we performed a closure inspection of the dynamic cross-correlation values of the ZINC4497834-interacting allosteric site 2 residues N151, T292, and D295 with all other residues in the apo and ZINC4497834-bound Mpro metastable state m2_c5 (Figure 11C). This analysis revealed a significant decrease in the positive cross-correlated motions of N151 with catalytic site residues N142 (p = 0.034) and G143 (p = 0.04) (Figure 11D, left panel, highlighted with red box). Third, we would like to note that the allosteric inhibitor AT7519, identified through X-ray screening by Günther et al. (2021), also binds to the allosteric site 2 that we have identified here and interacts with the residue D153, which is close to residues N151 and D295 (Günther et al., 2021). Finally, a comprehensive deep mutational scanning of Mpro by Flynn et al. (2022) revealed that residue D295 has a low mutation tolerance (Flynn et al., 2022). Together, these provide a probable mechanism underlying the inhibition of Mpro due to the binding of ZINC4497834 to the predicted allosteric site in the protein.
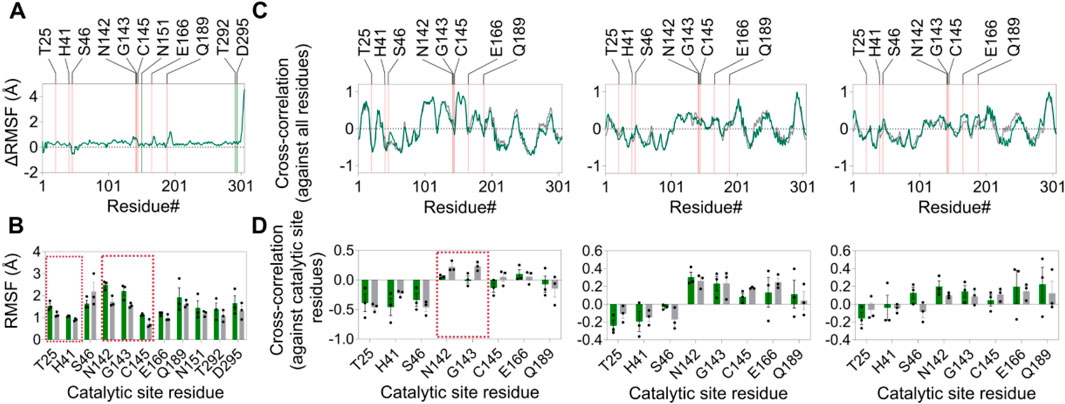
Figure 11. Altered structural dynamics of the Mpro metastable state m2_c5 induced by ZINC4497834. (A) Graphs showing the change in average RMSF (ΔRMSF) of residues in the Mpro metastable state m2_c5 in the presence of bound ZINC4497834 (orange and green lines indicate the location of catalytic site residues and ligand interacting residues on the x-axis, respectively). ΔRMSF values were determined by subtracting the average RMSF values of residues in the apo Mpro metastable state m2_c5 from the average RMSF values of residues in the Mpro metastable state m2_c5 bound to ZINC4497834. (B) Graph showing the RMSF(Å) of catalytic site residues and ZINC4497834-interacting residues in the apo (gray bars) and ZINC4497834-bound (green bars) Mpro metastable state m2_c5. (C) Graphs showing the average DCC values of residues N151 (right panel), T292 (middle panel), and D295 (left panel) against all residues in the protein (orange lines indicate the location of catalytic site residues on the x-axis). (D) Graphs showing the DCC values of residue N151 (left panel), T292 (middle panel), and D295 (right panel) with catalytic site residues. The dashed red boxes in B and D highlight the residues with significant difference in RMSF (B) and DCC values (D) between apo and the ZINC4497834-bound Mpro metastable state m2_c5, respectively. Data shown are obtained from three independent, 100 ns-long MD simulations.
Conclusion
To conclude, we performed a structural analysis of metastable states of Mpro identified from MD simulation and identified an Mpro metastable state (m2_c5) having a deformed catalytic site based on its affinity for the Mpro N-terminal auto-cleavage sequence. Furthermore, we identified two potential druggable allosteric sites on the Mpro metastable state m2_c5 and performed in silico screening (molecular docking) of a library of drug-like compounds obtained from the ZINC15 database, which resulted in the identification of three potential high-affinity allosteric site-binding compounds. Additional computational analysis, MD simulations, revealed a stable binding of selected compounds to their respective allosteric sites and a change in the Mpro metastable state m2_c5 structural dynamics upon binding to high-affinity allosteric site compounds. Importantly, one of the three selected compounds, ZINC4497834, inhibited SARS-CoV-2 Mpro activity in BRET-based in vitro assay, thus suggesting that this compound could act as an allosteric inhibitor of SARS-CoV-2 Mpro. We envisage that this compound can be taken further down the drug discovery pipeline as an allosteric inhibitor of SARS-CoV-2 Mpro after further research, including mutational analysis, live cell, and in vivo experiments. Moreover, the allosteric sites identified in this study can be targeted for the discovery of highly potent Mpro allosteric inhibitors.
Data availability statement
The original contributions presented in the study are included in the article/Supplementary Material; further inquiries can be directed to the corresponding author.
Author contributions
AF: data curation, formal analysis, investigation, methodology, writing–original draft. AG: data curation, formal analysis, investigation, writing–review and editing. WA: data curation, formal analysis, investigation, writing–review and editing. KB: conceptualization, formal analysis, investigation, methodology, supervision, writing–original draft, writing–review and editing, funding acquisition, project administration resources.
Funding
The author(s) declare that financial support was received for the research, authorship, and/or publication of this article. This work was supported by a grant from the HBKU Thematic Research Grant Program (VPR-TG01-007) and internal funding from the College of Health and Life Sciences, Hamad Bin Khalifa University, a member of the Qatar Foundation. Some of the computational research work reported in the manuscript was performed using high-performance computer resources and services provided by the Research Computing group at Texas A&M University in Qatar. Research computing is funded by the Qatar Foundation for Education, Science, and Community Development (http://www.qf.org.qa).
Conflict of interest
The authors declare that the research was conducted in the absence of any commercial or financial relationships that could be construed as a potential conflict of interest.
Publisher’s note
All claims expressed in this article are solely those of the authors and do not necessarily represent those of their affiliated organizations, or those of the publisher, the editors, and the reviewers. Any product that may be evaluated in this article, or claim that may be made by its manufacturer, is not guaranteed or endorsed by the publisher.
Supplementary material
The Supplementary Material for this article can be found online at: https://www.frontiersin.org/articles/10.3389/fmolb.2024.1451280/full#supplementary-material
References
Ahmed, W. S., Biswas, K. H., and Philip, A. M. (2022). Decreased interfacial dynamics caused by the N501Y mutation in the SARS-CoV-2 S1 spike: ACE2 complex. Front. Mol. Biosci. 506. doi:10.3389/fmolb.2022.846996
Ahmed, W. S., Geethakumari, A. M., Sultana, A., Fatima, A., Philip, A. M., Uddin, S. M. N., et al. (2024). A slow but steady nanoLuc: R162A mutation results in a decreased, but stable, nanoLuc activity. Int. J. Biol. Macromol. 269, 131864. doi:10.1016/j.ijbiomac.2024.131864
Altamash, T., Ahmed, W., Rasool, S., and Biswas, K. H. (2021). Intracellular ionic strength sensing using NanoLuc. Int. J. Mol. Sci. 22, 677. doi:10.3390/ijms22020677
Alzyoud, L., Ghattas, M. A., and Atatreh, N. (2022). Allosteric binding sites of the SARS-CoV-2 main protease: potential targets for broad-spectrum anti-coronavirus agents. Drug Des. Devel Ther. 16, 2463–2478. doi:10.2147/dddt.S370574
Arafet, K., Serrano-Aparicio, N., Lodola, A., Mulholland, A. J., González, F. V., Świderek, K., et al. (2020). Mechanism of inhibition of SARS-CoV-2 M(pro) by N3 peptidyl Michael acceptor explained by QM/MM simulations and design of new derivatives with tunable chemical reactivity. Chem. Sci. 12, 1433–1444. doi:10.1039/d0sc06195f
Arshad, N., Laurent-Rolle, M., Ahmed, W. S., Hsu, J. C. C., Mitchell, S. M., Pawlak, J., et al. (2023). SARS-CoV-2 accessory proteins ORF7a and ORF3a use distinct mechanisms to down-regulate MHC-I surface expression. Proc. Natl. Acad. Sci. U. S. A. 120, e2208525120. doi:10.1073/pnas.2208525120
Astore, M. A., Pradhan, A. S., Thiede, E. H., and Hanson, S. M. (2024). Protein dynamics underlying allosteric regulation. Curr. Opin. Struct. Biol. 84, 102768. doi:10.1016/j.sbi.2023.102768
Bhat, Z. A., Chitara, D., Iqbal, J., Sanjeev, B., and Madhumalar, A. (2022a). Targeting allosteric pockets of SARS-CoV-2 main protease Mpro. J. Biomol. Struct. Dyn. 40, 6603–6618. doi:10.1080/07391102.2021.1891141
Bhat, Z. A., Chitara, D., Iqbal, J., Sanjeev, B. S., and Madhumalar, A. (2022b). Targeting allosteric pockets of SARS-CoV-2 main protease M(pro). J. Biomol. Struct. Dyn. 40, 6603–6618. doi:10.1080/07391102.2021.1891141
Bhat, Z. A., Khan, M. M., Rehman, A., Iqbal, J., Sanjeev, B. S., and Madhumalar, A. (2024). MD simulations indicate Omicron P132H of SARS-CoV-2 M(pro) is a potential allosteric mutant involved in modulating the dynamics of catalytic site entry loop. Int. J. Biol. Macromol. 262, 130077. doi:10.1016/j.ijbiomac.2024.130077
Bian, L., Gao, F., Zhang, J., He, Q., Mao, Q., Xu, M., et al. (2021). Effects of SARS-CoV-2 variants on vaccine efficacy and response strategies. Expert Rev. vaccines. 20, 365–373. doi:10.1080/14760584.2021.1903879
Biswas, K., Sopory, S., and Visweswariah, S. (2008a). The GAF domain of the cGMP-binding, cGMP-specific phosphodiesterase (PDE5) is a sensor and a sink for cGMP. Biochemistry 47, 3534–3543. doi:10.1021/bi702025w
Biswas, K. H., Badireddy, S., Rajendran, A., Anand, G. S., and Visweswariah, S. S. (2015). Cyclic nucleotide binding and structural changes in the isolated GAF domain of Anabaena adenylyl cyclase, CyaB2. PeerJ 3, e882. doi:10.7717/peerj.882
Biswas, K. H. (2017). Allosteric regulation of proteins: A historical perspective on the development of concepts and techniques. Resonance. 22(1). 37–50. doi:10.1007/s12045-017-0431-z
Biswas, K. H., Sopory, S., and Visweswariah, S. S. (2008b). The GAF domain of the cGMP-binding, cGMP-specific phosphodiesterase (PDE5) is a sensor and a sink for cGMP. Biochemistry. 47, 3534–3543. doi:10.1021/bi702025w
Biswas, K. H., and Visweswariah, S. S. (2011). Distinct allostery induced in the cyclic GMP-binding, cyclic GMP-specific phosphodiesterase (PDE5) by cyclic GMP, sildenafil, and metal ions. J. Biol. Chem. 286, 8545–8554. doi:10.1074/jbc.M110.193185
Brown, D. K., Penkler, D. L., Sheik Amamuddy, O., Ross, C., Atilgan, A. R., Atilgan, C., et al. (2017). MD-TASK: a software suite for analyzing molecular dynamics trajectories. Bioinformatics 33, 2768–2771. doi:10.1093/bioinformatics/btx349
Callaway, E. (2022). Fast-evolving COVID variants complicate vaccine updates. Nature 607, 18–19. doi:10.1038/d41586-022-01771-3
Carli, M., Sormani, G., Rodriguez, A., and Laio, A. (2021). Candidate binding sites for allosteric inhibition of the SARS-CoV-2 main protease from the analysis of large-scale molecular dynamics simulations. J. Phys. Chem. Lett. 12, 65–72. doi:10.1021/acs.jpclett.0c03182
Cevik, M., Kuppalli, K., Kindrachuk, J., and Peiris, M. (2020). Virology, transmission, and pathogenesis of SARS-CoV-2. BMJ 371, m3862. doi:10.1136/bmj.m3862
Chan, H. T. H., Moesser, M. A., Walters, R. K., Malla, T. R., Twidale, R. M., John, T., et al. (2021). Discovery of SARS-CoV-2 Mpro peptide inhibitors from modelling substrate and ligand binding. Chem. Sci. 12, 13686–13703. doi:10.1039/D1SC03628A
Chen, L. L., Lu, L., Choi, C. Y. K., Cai, J. P., Tsoi, H. W., Chu, A. W. H., et al. (2022). Impact of severe acute respiratory syndrome coronavirus 2 (SARS-CoV-2) variant-associated receptor binding domain (RBD) mutations on the susceptibility to serum antibodies elicited by coronavirus disease 2019 (COVID-19) infection or vaccination. Clin. Infect. Dis. 74, 1623–1630. doi:10.1093/cid/ciab656
Chen, S. A., Arutyunova, E., Lu, J., Khan, M. B., Rut, W., Zmudzinski, M., et al. (2023). SARS-CoV-2 Mpro protease variants of concern display altered viral substrate and cell host target galectin-8 processing but retain sensitivity toward antivirals. ACS Central Sci. 9, 696–708. doi:10.1021/acscentsci.3c00054
Cheohen, C. F. d. A. R., Esteves, M. E. A., da Fonseca, T. S., Leal, C. M., Assis, F. d. L. F., Campos, M. F., et al. (2023). In silico screening of phenylethanoid glycosides, a class of pharmacologically active compounds as natural inhibitors of SARS-CoV-2 proteases. Comput. Struct. Biotechnol. J. 21, 1461–1472. doi:10.1016/j.csbj.2023.02.020
Chiou, W. C., Hsu, M. S., Chen, Y. T., Yang, J. M., Tsay, Y. G., Huang, H. C., et al. (2021). Repurposing existing drugs: identification of SARS-CoV-2 3C-like protease inhibitors. J. Enzyme Inhib. Med. Chem. 36, 147–153. doi:10.1080/14756366.2020.1850710
Cooper, B. S., Evans, S., Jafari, Y., Pham, T. M., Mo, Y., Lim, C., et al. (2023). The burden and dynamics of hospital-acquired SARS-CoV-2 in England. Nature 623, 132–138. doi:10.1038/s41586-023-06634-z
Cucinotta, D., and Vanelli, M. (2020). WHO declares COVID-19 a pandemic. Acta Biomed. 91, 157–160. doi:10.23750/abm.v91i1.9397
Dai, W., Zhang, B., Jiang, X. M., Su, H., Li, J., Zhao, Y., et al. (2020). Structure-based design of antiviral drug candidates targeting the SARS-CoV-2 main protease. Science 368, 1331–1335. doi:10.1126/science.abb4489
Daina, A., Michielin, O., and Zoete, V. (2017). SwissADME: a free web tool to evaluate pharmacokinetics, drug-likeness and medicinal chemistry friendliness of small molecules. Sci. Rep. 7, 42717. doi:10.1038/srep42717
Essmann, U., Perera, L., Berkowitz, M. L., Darden, T., Lee, H., and Pedersen, L. G. (1995). A smooth particle mesh Ewald method. J. Chem. Phys. 103, 8577–8593. doi:10.1063/1.470117
Feller, S. E., Zhang, Y., Pastor, R. W., and Brooks, B. R. (1995). Constant pressure molecular dynamics simulation: the Langevin piston method. J. Chem. Phys. 103, 4613–4621. doi:10.1063/1.470648
Ferreira, J. C., Fadl, S., and Rabeh, W. M. (2022). Key dimer interface residues impact the catalytic activity of 3CLpro, the main protease of SARS-CoV-2. J. Biol. Chem. 298, 102023. doi:10.1016/j.jbc.2022.102023
Ferreira, J. C., Fadl, S., Villanueva, A. J., and Rabeh, W. M. (2021). Catalytic dyad residues His41 and Cys145 impact the catalytic activity and overall conformational fold of the main SARS-CoV-2 protease 3-chymotrypsin-like protease. Front. Chem. 9, 692168. doi:10.3389/fchem.2021.692168
Flynn, J. M., Samant, N., Schneider-Nachum, G., Barkan, D. T., Yilmaz, N. K., Schiffer, C. A., et al. (2022). Comprehensive fitness landscape of SARS-CoV-2 Mpro reveals insights into viral resistance mechanisms. eLife 11, e77433. doi:10.7554/eLife.77433
Ge, R., Shen, Z., Yin, J., Chen, W., Zhang, Q., An, Y., et al. (2022). Discovery of SARS-CoV-2 main protease covalent inhibitors from a DNA-encoded library selection. SLAS Discov. 27, 79–85. doi:10.1016/j.slasd.2022.01.001
Geethakumari, A. M., Ahmed, W. S., Rasool, S., Fatima, A., Nasir Uddin, S. M., Aouida, M., et al. (2022a). A genetically encoded BRET-based SARS-CoV-2 M(pro) protease activity sensor. Commun. Chem. 5, 117. doi:10.1038/s42004-022-00731-2
Geethakumari, A. M., Ahmed, W. S., Rasool, S., Fatima, A., Uddin, S. N., Aouida, M., et al. (2022b). A Genetically encoded BRET-based SARS-CoV-2 Mpro protease activity sensor. bioRxiv 2022.2001.2031, 478460. doi:10.1101/2022.01.31.478460
Golob, J. L., Lugogo, N., Lauring, A. S., and Lok, A. S. (2021). SARS-CoV-2 vaccines: a triumph of science and collaboration. JCI insight 6, e149187. doi:10.1172/jci.insight.149187
Grum-Tokars, V., Ratia, K., Begaye, A., Baker, S. C., and Mesecar, A. D. (2008). Evaluating the 3C-like protease activity of SARS-Coronavirus: recommendations for standardized assays for drug discovery. Virus Res. 133, 63–73. doi:10.1016/j.virusres.2007.02.015
Günther, S., Reinke, P. Y. A., Fernández-García, Y., Lieske, J., Lane, T. J., Ginn, H. M., et al. (2021). X-ray screening identifies active site and allosteric inhibitors of SARS-CoV-2 main protease. Science 372, 642–646. doi:10.1126/science.abf7945
Hall, V., Foulkes, S., Insalata, F., Kirwan, P., Saei, A., Atti, A., et al. (2022). Protection against SARS-CoV-2 after Covid-19 vaccination and previous infection. N. Engl. J. Med. 386, 1207–1220. doi:10.1056/NEJMoa2118691
Harrison, A. G., Lin, T., and Wang, P. (2020). Mechanisms of SARS-CoV-2 transmission and pathogenesis. Trends Immunol. 41, 1100–1115. doi:10.1016/j.it.2020.10.004
Hu, Q., Xiong, Y., Zhu, G. H., Zhang, Y. N., Zhang, Y. W., Huang, P., et al. (2022). The SARS-CoV-2 main protease (Mpro): structure, function, and emerging therapies for COVID-19. MedComm 3, e151. doi:10.1002/mco2.151
Huang, J., Rauscher, S., Nawrocki, G., Ran, T., Feig, M., de Groot, B. L., et al. (2017). CHARMM36m: an improved force field for folded and intrinsically disordered proteins. Nat. methods 14, 71–73. doi:10.1038/nmeth.4067
Huespe, I. A., Ferraris, A., Lalueza, A., Valdez, P. R., Peroni, M. L., Cayetti, L. A., et al. (2023). COVID-19 vaccines reduce mortality in hospitalized patients with oxygen requirements: differences between vaccine subtypes. A multicontinental cohort study. J. Med. Virol. 95, e28786. doi:10.1002/jmv.28786
Huff, S., Kummetha, I. R., Tiwari, S. K., Huante, M. B., Clark, A. E., Wang, S., et al. (2022). Discovery and mechanism of SARS-CoV-2 main protease inhibitors. J. Med. Chem. 65, 2866–2879. doi:10.1021/acs.jmedchem.1c00566
Humphrey, W., Dalke, A., and Schulten, K. (1996). VMD: visual molecular dynamics. J. Mol. Graph. 14, 33–38. doi:10.1016/0263-7855(96)00018-5
Irwin, J. J., and Shoichet, B. K. (2005). ZINC--a free database of commercially available compounds for virtual screening. J. Chem. Inf. Model 45, 177–182. doi:10.1021/ci049714+
Jackson, J. K.Overview (2022). in Global economic effects of COVID-19. Available at: https://crsreports.congress.gov/product/pdf/R/R46270/84.
Jan, Z., Ahmed, W. S., Biswas, K. H., and Jithesh, P. V. (2023b). Identification of a potential DNA methyltransferase (DNMT) inhibitor. J. Biomol. Struct. and Dyn. 1-15. doi:10.1080/07391102.2023.2233637
Jan, Z., Geethakumari, A. M., Biswas, K. H., and Jithesh, P. V. (2023a). Protegrin-2, a potential inhibitor for targeting SARS-CoV-2 main protease Mpro. Comput. Struct. Biotechnol. J. 21, 3665–3671. doi:10.1016/j.csbj.2023.07.020
Jangra, S., Ye, C., Rathnasinghe, R., Stadlbauer, D., Krammer, F., Simon, V., et al. (2021). SARS-CoV-2 spike E484K mutation reduces antibody neutralisation. Lancet Microbe 2, e283–e284. doi:10.1016/s2666-5247(21)00068-9
Jiménez-Avalos, G., Vargas-Ruiz, A. P., Delgado-Pease, N. E., Olivos-Ramirez, G. E., Sheen, P., Fernández-Díaz, M., et al. (2021). Comprehensive virtual screening of 4.8 k flavonoids reveals novel insights into allosteric inhibition of SARS-CoV-2 M(PRO). Sci. Rep. 11, 15452. doi:10.1038/s41598-021-94951-6
Jin, Z., Du, X., Xu, Y., Deng, Y., Liu, M., Zhao, Y., et al. (2020). Structure of Mpro from SARS-CoV-2 and discovery of its inhibitors. Nature 582, 289–293. doi:10.1038/s41586-020-2223-y
Jo, S., Kim, T., Iyer, V. G., and Im, W.CHARMM.-G. U. I. (2008). CHARMM-GUI: a web-based graphical user interface for CHARMM. J. Comput. Chem. 29, 1859–1865. doi:10.1002/jcc.20945
Jorgensen, W. L., Chandrasekhar, J., Madura, J. D., Impey, R. W., and Klein, M. L. (1983). Comparison of simple potential functions for simulating liquid water. J. Chem. Phys. 79, 926–935. doi:10.1063/1.445869
Kaptan, S., Girych, M., Enkavi, G., Kulig, W., Sharma, V., Vuorio, J., et al. (2022). Maturation of the SARS-CoV-2 virus is regulated by dimerization of its main protease. Comput. Struct. Biotechnol. J. 20, 3336–3346. doi:10.1016/j.csbj.2022.06.023
Khalaf, K., Papp, N., Chou, J. T. T., Hana, D., Mackiewicz, A., and Kaczmarek, M. (2020). SARS-CoV-2: pathogenesis, and advancements in diagnostics and treatment. Front. Immunol. 11, 570927. doi:10.3389/fimmu.2020.570927
Kollman, P., Massova, I., Reyes, C., Kuhn, B., Huo, S., Chong, L., et al. (2000). Calculating structures and free energies of complex molecules: combining molecular mechanics and continuum models. Acc. Chem. Res. 33, 889–897. doi:10.1021/ar000033j
Lamers, M. M., and Haagmans, B. L. (2022). SARS-CoV-2 pathogenesis. Nat. Rev. Microbiol. 20, 270–284. doi:10.1038/s41579-022-00713-0
Lee, J., Kenward, C., Worrall, L. J., Vuckovic, M., Gentile, F., Ton, A. T., et al. (2022). X-ray crystallographic characterization of the SARS-CoV-2 main protease polyprotein cleavage sites essential for viral processing and maturation. Nat. Commun. 13, 5196. doi:10.1038/s41467-022-32854-4
Legare, S., Heide, F., Bailey-Elkin, B. A., and Stetefeld, J. (2022). Improved SARS-CoV-2 main protease high-throughput screening assay using a 5-carboxyfluorescein substrate. J. Biol. Chem. 298, 101739. doi:10.1016/j.jbc.2022.101739
Li, H., Leung, K. S., and Wong, M. H. (2012). in 2012 IEEE symposium on computational intelligence in bioinformatics and computational biology (CIBCB), 77–84.
Liu, H., and Hou, T. (2016). CaFE: a tool for binding affinity prediction using end-point free energy methods. Bioinforma. Oxf. Engl. 32, 2216–2218. doi:10.1093/bioinformatics/btw215
Lu, J., Chen, S. A., Khan, M. B., Brassard, R., Arutyunova, E., Lamer, T., et al. (2022). Crystallization of feline coronavirus Mpro with GC376 reveals mechanism of inhibition. Front. Chem. 10, 852210. doi:10.3389/fchem.2022.852210
Luan, X.-d., Chen, B. X., Shang, W. J., Yin, W. C., Jin, Y., Zhang, L. K., et al. (2023). Structure basis for inhibition of SARS-CoV-2 by the feline drug GC376. Acta Pharmacol. Sin. 44, 255–257. doi:10.1038/s41401-022-00929-z
Ludwig, S., and Zarbock, A. (2020). Coronaviruses and SARS-CoV-2: a brief overview. Anesth. analgesia 131, 93–96. doi:10.1213/ANE.0000000000004845
McCullagh, M., Zeczycki, T. N., Kariyawasam, C. S., Durie, C. L., Halkidis, K., Fitzkee, N. C., et al. (2024). What is allosteric regulation? Exploring the exceptions that prove the rule. J. Biol. Chem. 300, 105672. doi:10.1016/j.jbc.2024.105672
McLean, G., Kamil, J., Lee, B., Moore, P., Schulz, T. F., Muik, A., et al. (2022). The impact of evolving SARS-CoV-2 mutations and variants on COVID-19 vaccines. mBio 13, e0297921. doi:10.1128/mbio.02979-21
Mihalic, F., Benz, C., Kassa, E., Lindqvist, R., Simonetti, L., Inturi, R., et al. (2023). Identification of motif-based interactions between SARS-CoV-2 protein domains and human peptide ligands pinpoint antiviral targets. Nat. Commun. 14, 5636. doi:10.1038/s41467-023-41312-8
Miller, I. F., Becker, A. D., Grenfell, B. T., and Metcalf, C. J. E. (2020). Disease and healthcare burden of COVID-19 in the United States. Nat Med. doi:10.1038/s41591-020-0952-y
Mistry, P., Barmania, F., Mellet, J., Peta, K., Strydom, A., Viljoen, I. M., et al. (2022). SARS-CoV-2 variants, vaccines, and host immunity. Front. Immunol. 12, 809244. doi:10.3389/fimmu.2021.809244
Moovarkumudalvan, B., Geethakumari, A. M., Ramadoss, R., Biswas, K. H., and Mifsud, B. (2022). Structure-based virtual screening and functional validation of potential hit molecules targeting the SARS-CoV-2 main protease. Biomolecules 12, 1754. doi:10.3390/biom12121754
Narayanan, A., Narwal, M., Majowicz, S. A., Varricchio, C., Toner, S. A., Ballatore, C., et al. (2022). Identification of SARS-CoV-2 inhibitors targeting Mpro and PLpro using in-cell-protease assay. Commun. Biol. 5, 169. doi:10.1038/s42003-022-03090-9
Noske, G. D., de Souza Silva, E., de Godoy, M. O., Dolci, I., Fernandes, R. S., Guido, R. V. C., et al. (2023). Structural basis of nirmatrelvir and ensitrelvir activity against naturally occurring polymorphisms of the SARS-CoV-2 main protease. J. Biol. Chem. 299, 103004. doi:10.1016/j.jbc.2023.103004
Paciaroni, A., Libera, V., Ripanti, F., Orecchini, A., Petrillo, C., Francisci, D., et al. (2023). Stabilization of the dimeric state of SARS-CoV-2 main protease by GC376 and nirmatrelvir. Int. J. Mol. Sci. 24, 6062. doi:10.3390/ijms24076062
Pang, X., Xu, W., Liu, Y., Li, H., and Chen, L. (2023). The research progress of SARS-CoV-2 main protease inhibitors from 2020 to 2022. Eur. J. Med. Chem. 257, 115491. doi:10.1016/j.ejmech.2023.115491
Parmar, M., Thumar, R., Patel, B., Athar, M., Jha, P. C., and Patel, D. (2022). Structural differences in 3C-like protease (Mpro) from SARS-CoV and SARS-CoV-2: molecular insights revealed by Molecular Dynamics Simulations. Struct. Chem., 1–18. doi:10.1007/s11224-022-02089-6
Pavlova, A., Lynch, D. L., Daidone, I., Zanetti-Polzi, L., Smith, M. D., Chipot, C., et al. (2021). Inhibitor binding influences the protonation states of histidines in SARS-CoV-2 main protease. Chem. Sci. 12, 1513–1527. doi:10.1039/d0sc04942e
Philip, A. M., Ahmed, W. S., and Biswas, K. H. (2023). Reversal of the unique Q493R mutation increases the affinity of Omicron S1-RBD for ACE2. Comput. Struct. Biotechnol. J. 21, 1966–1977. doi:10.1016/j.csbj.2023.02.019
Phillips, J. C., Braun, R., Wang, W., Gumbart, J., Tajkhorshid, E., Villa, E., et al. (2005). Scalable molecular dynamics with NAMD. J. Comput. Chem. 26, 1781–1802. doi:10.1002/jcc.20289
Puhl, A. C., Zorn, K. M., Lane, T. R., Russo, D. P., Klein, J. J., et al. (2019). Exploiting machine learning for end-to-end drug discovery and development. Nat. Mater. 18, 435–441. doi:10.1038/s41563-019-0338-z
Ravindranath, P. A., Forli, S., Goodsell, D. S., Olson, A. J., and Sanner, M. F. (2015). AutoDockFR: advances in protein-ligand docking with explicitly specified binding site flexibility. PLoS Comput. Biol. 11, e1004586. doi:10.1371/journal.pcbi.1004586
Robson, B. (2020). Computers and viral diseases. Preliminary bioinformatics studies on the design of a synthetic vaccine and a preventative peptidomimetic antagonist against the SARS-CoV-2 (2019-nCoV, COVID-19) coronavirus. Comput. Biol. Med. 119, 103670. doi:10.1016/j.compbiomed.2020.103670
Rossetti, G. G., Ossorio, M. A., Rempel, S., Kratzel, A., Dionellis, V. S., Barriot, S., et al. (2022). Non-covalent SARS-CoV-2 M(pro) inhibitors developed from in silico screen hits. Sci. Rep. 12, 2505. doi:10.1038/s41598-022-06306-4
Sabbah, D. A., Hajjo, R., Bardaweel, S. K., and Zhong, H. A. (2021). An updated review on SARS-CoV-2 main proteinase (M(pro)): protein structure and small-molecule inhibitors. Curr. Top. Med. Chem. 21, 442–460. doi:10.2174/1568026620666201207095117
Salje, H., Tran Kiem, C., Lefrancq, N., Courtejoie, N., Bosetti, P., Paireau, J., et al. (2020). Estimating the burden of SARS-CoV-2 in France. Science 369, 208–211. doi:10.1126/science.abc3517
Samrat, S. K., Xu, J., Xie, X., Gianti, E., Chen, H., Zou, J., et al. (2022). Allosteric inhibitors of the main protease of SARS-CoV-2. Antivir. Res. 205, 105381. doi:10.1016/j.antiviral.2022.105381
Schütz, D., Ruiz-Blanco, Y. B., Münch, J., Kirchhoff, F., Sanchez-Garcia, E., and Müller, J. A. (2020). Peptide and peptide-based inhibitors of SARS-CoV-2 entry. Adv. Drug Deliv. Rev. 167, 47–65. doi:10.1016/j.addr.2020.11.007
Shaqra, A. M., Zvornicanin, S. N., Huang, Q. Y. J., Lockbaum, G. J., Knapp, M., Tandeske, L., et al. (2022). Defining the substrate envelope of SARS-CoV-2 main protease to predict and avoid drug resistance. Nat. Commun. 13, 3556. doi:10.1038/s41467-022-31210-w
Sharun, K., Tiwari, R., and Dhama, K. (2021). Protease inhibitor GC376 for COVID-19: lessons learned from feline infectious peritonitis. Ann. Med. Surg. (Lond) 61, 122–125. doi:10.1016/j.amsu.2020.12.030
Shree, P., Mishra, P., Selvaraj, C., Singh, S. K., Chaube, R., Garg, N., et al. (2022). Targeting COVID-19 (SARS-CoV-2) main protease through active phytochemicals of ayurvedic medicinal plants - withania somnifera (Ashwagandha), Tinospora cordifolia (Giloy) and Ocimum sanctum (Tulsi) - a molecular docking study. J. Biomol. Struct. Dyn. 40, 1–14. doi:10.1080/07391102.2020.1810778
Steinbach, P. J., and Brooks, B. R. (1994). New spherical-cutoff methods for long-range forces in macromolecular simulation. J. Comput. Chem. 15, 667–683. doi:10.1002/jcc.540150702
Sterling, T., and Irwin, J. J. (2015). ZINC 15--ligand discovery for everyone. J. Chem. Inf. Model 55, 2324–2337. doi:10.1021/acs.jcim.5b00559
Strömich, L., Wu, N., Barahona, M., and Yaliraki, S. N. (2022). Allosteric hotspots in the main protease of SARS-CoV-2. J. Mol. Biol. 434, 167748. doi:10.1016/j.jmb.2022.167748
Sun, Z., Wang, L., Li, X., Fan, C., Xu, J., Shi, Z., et al. (2022). An extended conformation of SARS-CoV-2 main protease reveals allosteric targets. Proc. Natl. Acad. Sci. 119, e2120913119. doi:10.1073/pnas.2120913119
Tao, K., Tzou, P. L., Nouhin, J., Gupta, R. K., de Oliveira, T., Kosakovsky Pond, S. L., et al. (2021a). The biological and clinical significance of emerging SARS-CoV-2 variants. Nat. Rev. Genet. 22, 757–773. doi:10.1038/s41576-021-00408-x
Tao, K., Tzou, P. L., Nouhin, J., Gupta, R. K., de Oliveira, T., Kosakovsky Pond, S. L., et al. (2021b). The biological and clinical significance of emerging SARS-CoV-2 variants. Nat. Rev. Genet. 22, 757–773. doi:10.1038/s41576-021-00408-x
Tao, X., Zhang, L., Du, L., Liao, R., Cai, H., Lu, K., et al. (2021c). Allosteric inhibition of SARS-CoV-2 3CL protease by colloidal bismuth subcitrate. Chem. Sci. 12, 14098–14102. doi:10.1039/d1sc03526f
Tee, W. V., and Berezovsky, I. N. (2024). Allosteric drugs: new principles and design approaches. Curr. Opin. Struct. Biol. 84, 102758. doi:10.1016/j.sbi.2023.102758
Trott, O., and Olson, A. J. (2010). AutoDock Vina: improving the speed and accuracy of docking with a new scoring function, efficient optimization, and multithreading. J. Comput. Chem. 31, 455–461. doi:10.1002/jcc.21334
Trougakos, I. P., Stamatelopoulos, K., Terpos, E., Tsitsilonis, O. E., Aivalioti, E., Paraskevis, D., et al. (2021). Insights to SARS-CoV-2 life cycle, pathophysiology, and rationalized treatments that target COVID-19 clinical complications. J. Biomed. Sci. 28 (9), 9. doi:10.1186/s12929-020-00703-5
Tumskiy, R. S., Tumskaia, A. V., Klochkova, I. N., and Richardson, R. J. (2023). SARS-CoV-2 proteases Mpro and PLpro: design of inhibitors with predicted high potency and low mammalian toxicity using artificial neural networks, ligand-protein docking, molecular dynamics simulations, and ADMET calculations. Comput. Biol. Med. 153, 106449. doi:10.1016/j.compbiomed.2022.106449
Uddin, S. M. N., Rasool, S., Geethakumari, A. M., Ahmed, W. S., and Biswas, K. H. (2024). Engineering β-catenin-derived peptides for α-catenin binding. Emergent Mater. doi:10.1007/s42247-024-00663-8
Uwamino, Y., Yokoyama, T., Shimura, T., Nishimura, T., Sato, Y., Wakui, M., et al. (2022). The effect of the E484K mutation of SARS-CoV-2 on the neutralizing activity of antibodies from BNT162b2 vaccinated individuals. Vaccine 40, 1928–1931. doi:10.1016/j.vaccine.2022.02.047
Verma, S., and Pandey, A. K. (2021). Factual insights of the allosteric inhibition mechanism of SARS-CoV-2 main protease by quercetin: an in silico analysis. 3 Biotech. 11, 67. doi:10.1007/s13205-020-02630-6
Vicenti, I., Zazzi, M., and Saladini, F. (2021). SARS-CoV-2 RNA-dependent RNA polymerase as a therapeutic target for COVID-19. Expert Opin. Ther. Pat. 31, 325–337. doi:10.1080/13543776.2021.1880568
V’kovski, P., Kratzel, A., Steiner, S., Stalder, H., and Thiel, V. (2021). Coronavirus biology and replication: implications for SARS-CoV-2. Nat. Rev. Microbiol. 19, 155–170. doi:10.1038/s41579-020-00468-6
Volkamer, A., Griewel, A., Grombacher, T., and Rarey, M. (2010). Analyzing the topology of active sites: on the prediction of pockets and subpockets. J. Chem. Inf. Model. 50, 2041–2052. doi:10.1021/ci100241y
Volkamer, A., Kuhn, D., Rippmann, F., and Rarey, M. (2012). DoGSiteScorer: a web server for automatic binding site prediction, analysis and druggability assessment. Bioinformatics 28, 2074–2075. doi:10.1093/bioinformatics/bts310
Wenthur, C. J., Gentry, P. R., Mathews, T. P., and Lindsley, C. W. (2014). Drugs for allosteric sites on receptors. Annu. Rev. Pharmacol. Toxicol. 54, 165–184. doi:10.1146/annurev-pharmtox-010611-134525
Wl, D. (2002). The PyMOL molecular graphics system. Available at: http://www.pymol.org.
Wu, D., Wu, T., Liu, Q., and Yang, Z. Z. (2020). The SARS-CoV-2 outbreak: What we know. Int J Infect Dis. 94:44–48. doi:10.1016/j.ijid.2020.03.004
Wu, N., Barahona, M., and Yaliraki, S. N. (2024). Allosteric communication and signal transduction in proteins. Curr. Opin. Struct. Biol. 84, 102737. doi:10.1016/j.sbi.2023.102737
Xue, X., Yu, H., Yang, H., Xue, F., Wu, Z., Shen, W., et al. (2008). Structures of two coronavirus main proteases: implications for substrate binding and antiviral drug design. J. Virol. 82, 2515–2527. doi:10.1128/jvi.02114-07
Yuan, S., Chan, H. S., and Hu, Z. (2017). Using PyMOL as a platform for computational drug design. Wiley Interdiscip. Rev. Comput. Mol. Sci. 7, e1298. doi:10.1002/wcms.1298
Yuce, M., Cicek, E., Inan, T., Dag, A. B., Kurkcuoglu, O., and Sungur, F. A. (2021). Repurposing of FDA-approved drugs against active site and potential allosteric drug-binding sites of COVID-19 main protease. Proteins 89, 1425–1441. doi:10.1002/prot.26164
Keywords: COVID-19, allosteric regulator, MD simulation, metastable states, Mpro, ZINC15
Citation: Fatima A, Geethakumari AM, Ahmed WS and Biswas KH (2024) A potential allosteric inhibitor of SARS-CoV-2 main protease (Mpro) identified through metastable state analysis. Front. Mol. Biosci. 11:1451280. doi: 10.3389/fmolb.2024.1451280
Received: 18 June 2024; Accepted: 14 August 2024;
Published: 06 September 2024.
Edited by:
Yonglan Liu, National Cancer Institute at Frederick (NIH), United StatesReviewed by:
Mohamed Shehata, University of California, San Diego, United StatesAditya K. Padhi, Riken Yokohama, Japan
Copyright © 2024 Fatima, Geethakumari, Ahmed and Biswas. This is an open-access article distributed under the terms of the Creative Commons Attribution License (CC BY). The use, distribution or reproduction in other forums is permitted, provided the original author(s) and the copyright owner(s) are credited and that the original publication in this journal is cited, in accordance with accepted academic practice. No use, distribution or reproduction is permitted which does not comply with these terms.
*Correspondence: Kabir H. Biswas, a2Jpc3dhc0BoYmt1LmVkdS5xYQ==
†ORCID: Asma Fatima, orcid.org/0009-0004-7191-5109; Anupriya M. Geethakumari, orcid.org/0000-0002-8932-9114; Wesam S. Ahmed, orcid.org/0000-0002-3441-2631; Kabir H. Biswas, orcid.org/0000-0001-9194-4127