- 1Key Laboratory of Healthy Mariculture for the East China Sea, Ministry of Agriculture and Rural Affairs, Jimei University, Xiamen, China
- 2Zhejiang Fisheries Technical Extension Center, Hangzhou, China
- 3Key Lab of Mariculture and enhancement of Zhejiang Province, Zhejiang Marine fisheries Research institute, Zhoushan, China
The largemouth bass is a native species of North America that was first introduced to mainland China in the 1980s. In recent years, it has been extensively farmed in China due to its high meat quality and broad adaptability. In this study, we collected growth trait data from 1,066 largemouth bass individuals across two populations. We generated an average of approximately 7× sequencing coverage for these fish using Illumina sequencers. From the samples, we identified 2,695,687 SNPs and retained 1,809,116 SNPs for further analysis after filtering. To estimate the number of genome-wide effective SNPs, we performed LD pruning with PLINK software and identified 77,935 SNPs. Our GWAS revealed 15 SNPs associated with six growth traits. We identified a total of 24 genes related to growth, with three genes—igf1, myf5, and myf6—directly associated with skeletal muscle development and growth, located near the leading SNP on chromosome 23. Other candidate genes are involved in the development of tissues and organs or other physiological processes. These findings provide a valuable set of SNPs and genes that could be useful for genetic breeding programs aimed at enhancing growth in largemouth bass.
1 Introduction
Largemouth bass (Micropterus salmoides) is a freshwater fish native to rivers and lakes in America (Bai et al., 2008). Due to its strong adaptability, high meat quality, and absence of intermuscular bones, largemouth bass is now extensively farmed in China. First introduced to mainland China in 1983, its production has recently exceeded 700,000 tons (https://www.yearbookchina.com/downsoft-n3020013079.html), making it a crucial species in Chinese freshwater aquaculture (Xie et al., 2020).
Growth is a critical trait in aquaculture species due to its significant economic implications, and fast-growing species are prime targets for improvement (Li et al., 2017). Key growth traits, including body weight (BW), body length (BL), body height (BH), body thickness (BT), caudal peduncle length (CPL), caudal peduncle height (CPH), and condition factor (K) (Li et al., 2017; de Vries et al., 2020), are used to describe growth. These quantitative traits are influenced by polygenes with minor effects, environmental factors, and interactions between genes and the environment (Wang et al., 2006). According to Li et al. (2017), growth trait variation has a strong genetic basis and can be enhanced through phenotypic selection, with genetic gain accelerated by marker-assisted selection.
Genome-wide association study (GWAS) are now the standard method for identifying associations between single nucleotide polymorphisms (SNPs) and phenotypic traits at the whole-genome level, based on linkage disequilibrium (LD) (Visscher et al., 2012). By 2017, over 3,000 human GWAS had investigated more than 1,800 diseases and traits, revealing thousands of SNP associations (Buniello et al., 2019). GWAS has been widely applied to study genetic mechanisms of human diseases and, in aquaculture, to explore growth, disease resistance, meat quality traits, and behavioral traits in species such as Atlantic salmon, catfish, rainbow trout, and carp. For instance, Sodeland et al. (2013) used 5,650 SNPs to identify genetic variations related to fillet fat content and firmness in Norwegian Atlantic salmon, while Tsai et al. (2015) showed that multiple gene loci (pomt1, myh9, gapdh, notch3, etc.) influence growth traits in juvenile farmed Atlantic salmon through high-density SNP arrays. Wan et al. (2018) conducted a GWAS on n-3 highly unsaturated fatty acids (HUFA) and eviscerated weight (EW) in the large yellow croaker, identifying three candidate regions for each trait. Zhou et al. (2022) used 5,205 high-quality SNPs to perform a GWAS on six growth traits in 160 mandarin fish (Siniperca chuatsi), detecting 11 significant and 37 suggestive SNPs. Huang et al. (2022) identified candidate SNPs and genes associated with body length and weight in 125 female yellow catfish (Pelteobagrus fulvidraco), finding one BL-related SNP and three BW-related SNPs significantly associated with these traits.
To date, research on largemouth bass has focused on its distribution, migration, and growth performance with nutrients like chlorogenic acid (Yin et al., 2021). High-density linkage maps for largemouth bass have been constructed (Dong et al., 2019), and recent studies have mapped the sex determination region (He et al., 2022; Wen et al., 2022). However, GWAS studies on growth traits in largemouth bass have not yet been reported. This study aims to identify candidate genes or mutations associated with growth traits in largemouth bass, which could be leveraged for genetic breeding to enhance future yields.
2 Materials and methods
2.1 Ethics statement
The sample collection of largemouth bass and experiments in this study were approved by the Animal Care and Use Committee of Jimei University (No. JMU202003019).
2.2 Sample collection and phenotypic traits measurements
In October 2020 and November 2021, a total of 1,066 largemouth bass were collected from Dongjing Biotechnology Co., Ltd. in Longyan, Fujian Province, China, 688 fish in October 2020 (batch 1) and 378 fish in November 2021 (batch 2). Pectoral fins were sampled from each fish and preserved in 75% ethyl alcohol. We collected phenotypic data on several growth traits, including body length (BL), body weight (BW), body thickness (BT), body height (BH), caudal peduncle length (CPL), and caudal peduncle height (CPH) for each individual. Differences between males and females were assessed using Fisher’s exact test. The fish collected in October 2020 were dissected to determine their phenotypic sex through visual examination of the gonads. For the fish collected in November 2021, sex was determined using a sex-specific marker (He et al., 2022).
2.3 Genome sequencing and SNP calling
Genomic DNA was extracted from the pectoral fin using the FastPure® Cell/Tissue DNA Isolation Mini Kit (Vazyme, Nanjing, China). The DNA was sheared and end-repaired, and then ligated with Illumina sequencing adapters to construct sequencing libraries. The libraries were quantified, pooled, and sequenced with 150-bp paired-end mode on a NovaSeq X platform at Novogene Co., Ltd. (Beijing, China). In total, 688 individuals of batch 1 and 378 individuals of batch 2 were sequenced.
After filtering out reads containing adaptor sequences or lower quality bases using Trimmomatic (v.0.36) (Bolger et al., 2014), we aligned the clean sequencing reads to the reference genome of largemouth bass (GenBank accession GCA_019677235.1) (Wen et al., 2022) using BWA (v 0.7.17) (Li and Durbin, 2009). We then sorted and indexed the alignment using Samtools (v1.11) (Danecek et al., 2021) and removed PCR duplicates with the Genome Analysis Toolkit (GATK, v4.2.0) (McKenna et al., 2010; DePristo et al., 2011). HaplotypeCaller function of GATK (v 4.2.0.0) was used to identify SNPs across all samples. PLINK (v1.9) (Yang et al., 2012) was used to filter the SNPs with following parameters: individual missing (geno) < 0.2, Hardy-Weinberg equilibrium (hwe) < 1e-5, minor allele frequency (maf) > 0.05, and SNPs missing rate <0.2. To impute missing sites, we used STITCH (v.1.6.6) with the parameters “nGen = 40, K = 30, ni = 30,” which were most suitable for our data (Davies et al., 2016).
2.4 Population structure analysis
Principal component analysis (PCA) of the sequenced fish was conducted using PLINK (v1.9) software. The twstats tool from EIGENSTRAT (v6.1.4) (Price et al., 2006) was employed to select significant principal components (PCs) for the GWAS. Additionally, to assess population kinship, we used Genome-wide Complex Trait Analysis (GCTA) software (v1.93.2) (Yang et al., 2011) to construct the genomic relationship matrix using the--make-grm parameter. A sparse genetic relationship matrix (GRM) was generated from the full-dense GRM using the--make-bK-sparse 0.05 parameter, with a cutoff value of 0.05.
2.5 Genome-wide association study (GWAS)
Association analysis was performed using the mixed linear model (MLM) of GCTA software (v1.93.2), the phenotypic data underwent normalization for transformation, and the covariates including the batch effect, gender and the results of PCA in the analysis (Jiang et al., 2019).
To determine the genome-wide significance cutoff for our GWAS results, we estimated the number of effective SNPs by pruning SNPs within a 50 kb window with an R2 ≥ 0.8 using PLINK (v1.9). We employed a 50-kb sliding window approach with a step size of 25 kb (Xiang et al., 2020; Wang et al., 2022). The Bonferroni correction was applied to establish the significance threshold, with genome-wide significance defined as 0.05 divided by the number of effective SNPs. The false discovery rate (FDR) was calculated using the Benjamini–Hochberg procedure to adjust the significance threshold (Benjamini and Hochberg, 2018). LD analysis plots were generated using LDBlockshow (v1.40) (Dong et al., 2021) and PopLDdecay (v3.41) (Zhang et al., 2019) software.
2.6 Heritability analysis
Heritability is defined as the proportion of variance in phenotype explained (PVE) by genetic variations (Young et al., 2022). The genetic relationship matrix (GRM) was constructed using GCTA, and heritability was calculated by a restricted maximum likelihood (REML) analysis with GCTA software. The PVE was calculated as follows (Shim et al., 2015; Young et al., 2022):
Where: N is the sample size, beta is the effect size for the genetic variant of interest, and MAF is the minor allele frequency for the genetic variant of interest, se (beta) is the standard error of effect size for the genetic variant of interest.
3 Results
3.1 Phenotypic statistic and correlations
The descriptive statistics results showed that the mean body weight (BW) of largemouth bass was 539.2 ± 111.21 g, with males averaging 548.2 g and females 530.6 g. The mean body length (BL) was 25.15 ± 1.96 cm, with males at 25.08 cm and females at 25.22 cm. The mean body height (BH) was 8.90 ± 0.81 cm, with males at 8.88 cm and females at 8.91 cm. The mean body thickness (BT) was 4.62 ± 0.51 cm, with males at 4.7 cm and females at 4.53 cm. The mean caudal peduncle length (CPL) was 3.17 ± 0.22 cm, with males at 3.15 cm and females at 3.18 cm. The mean caudal peduncle height (CPH) was 5.50 ± 0.66 cm, with males at 5.61 cm and females at 5.39 cm. Phenotypic differences between males and females were statistically significant for all traits (p-value range: 0.044–1.35e-08, Fisher’s exact test) except for CPH (p-value: 0.077) (Table 1). Correlation analysis revealed significant correlations between all growth traits (p < 0.01), except between CPL and BW and BT (Figure 1).
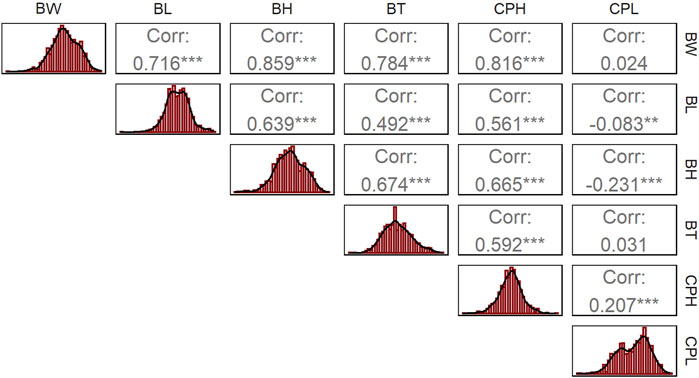
Figure 1. Correlation of each growth trait in largemouth bass. The superscripts of the coefficients indicate the significance of the Pearson correlation: * denotes p < 0.05, ** denotes p < 0.01, and *** denotes p < 0.001. The histogram on the left of each row shows the distribution of the phenotypic data for each trait, with the x-axis representing the trait value and the y-axis representing the frequency.
3.2 Sequencing and genotyping
We obtained a total of 4.9 TB of clean data through next-generation sequencing. The average sequencing depth for the samples was 6.9 × , with 10 samples sequenced at 11.1-12.3 × coverage. We used STITCH to impute the missing genotypes, achieving an imputation accuracy of 0.92, based on an assessment with the 10 high-coverage samples. After variant calling with GATK, we identified 2,695,687 SNPs. Following quality control, 685,037 variants were removed due to minor allele frequency thresholds (--maf), no individuals were removed due to missing genotype data (--mind), 124,328 variants were removed due to missing genotype data (--geno), and 77,156 variants were removed due to Hardy-Weinberg equilibrium exact test (--hwe). Ultimately, we retained 1,809,166 SNPs for subsequent analyses.
3.3 Population structure
We used Plink (v1.9) to generate 20 principal components (PCs). After examining the PCA results with EIGENSTRAT’s twstats tool (v6.1.4), we selected 10 PCs with p-value <0.05 for further analysis. The PCA outcomes revealed that PC1 accounted for 16.95% of the genetic variation and PC2 accounted for 12.34% (Figure 2). Together, PC1 and PC2 explain a substantial portion of the dataset’s variability. By observing the distribution of these points in the principal component space, we can gain insights into the similarities and differences between different data batches and their trends along the principal components. Additionally, the genetic relationship matrix (GRM) indicated a certain level of population stratification (Supplementary Figure S1). After transforming the GRM into a sparse matrix, no unrelated individuals were removed. Overall, our findings revealed the existence of population stratification among the 1066 individuals collected in two batches.
3.4 Genome-wide association study
We used 10 principal components (PCs), sex, and batch effect as covariates in the genome-wide association study. The number of genome-wide effective SNPs was determined by pruning SNPs within 50 kb and with R2 ≥ 0.8 using PLINK (v1.9) with a sliding window approach, resulting in 77,935 effective SNPs. The genome-wide significance threshold was set at 6.42 × 10e-7 (0.05/77,935) based on the number of effective SNPs.
Using GCTA software, the estimated heritability for body weight (BW), body length (BL), body height (BH), body thickness (BT), caudal peduncle height (CPH), and caudal peduncle length (CPL) were 0.37, 0.20, 0.44, 0.29, 0.48, and 0.28, respectively (Table 2). According to the GWAS results (Figure 3; Supplementary Figure S2), six SNPs in BW, BH, and CPH reached genome-wide significance. For BW, significant SNPs were located on chromosome 23 at positions 8,370,508 bp and 20,121,867 bp where p-value reached 7.52 and 7.14, with phenotypic variance explained (PVE) of 3.35% and 2.64%, respectively (Figure 3A; Table 2). For BH, significant SNPs were located on chromosome 1 at 3,391,027 bp where p-value reached 6.28, with PVEs of 2.28%, respectively (Figure 3C; Table 2). For CPH, significant SNPs were located on chromosome 19 at 33,623,024 bp, on chromosome 23 at 8,414,614 bp, and on chromosome 18 at 26,787,765 bp where p-value reached 7.15, 6.87 and 6.37, with PVEs of 3.06%, 2.99%, and 2.85%, respectively (Figure 3E; Table 2). In addition, we found the false discovery rate (FDR) results were consistent with the pruning SNPs overall (Table 2).
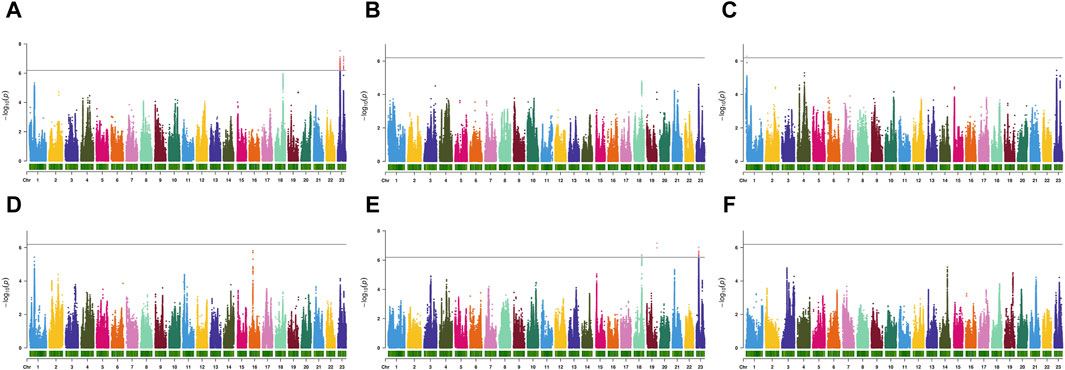
Figure 3. The manhattan plots of genome-wide association analysis of body weight (A), body length (B), body height (C), body thickness (D), caudal peduncle height (E), caudal peduncle length (F). The gray line represents the threshold p-value for genome-wide significance (-log10(p-value) = 6.19).
Additionally, we screened SNPs for growth traits of largemouth bass that reached suggestive significance (Table 2). We identified eight SNPs for BL, BT, and CPL traits in the GWAS results. For BL, significant SNPs were located on chromosomes 23, 18, and 21, with PVEs of 1.89%, 2.06%, and 1.5%, respectively (Figure 3B; Table 2). For BT, significant SNPs were located on chromosomes 16 and 1, with PVEs of 2.4% and 2.29%, respectively (Figure 3D; Table 2). For CPL, significant SNPs were located on chromosomes 14, 3, and 19, with PVEs of 2.09%, 2.06%, and 1.64%, respectively (Figure 3F; Table 2).
We investigated candidate genes associated with growth traits near the top SNPs and selected these genes based on linkage disequilibrium (LD) blocks surrounding the top SNPs (see Supplementary Figure S4). Following each of SNP, we ultimately identified 24 candidate genes related to growth traits (Table 2), and the gene function annotations were obtained using the ZFIN (https://zfin.org/), Alliance of Genome Resources (www.alliancegenome.org), and GeneCards (https://www.genecards.org/) web platforms.
For body weight (BW) and caudal peduncle height (CPH) traits, we identified four genes on chromosome 23: rerg, jup, etv6, and lrig3. The rerg gene inhibits cell proliferation (Finlin et al., 2001). The jup gene is involved in alpha-catenin and cadherin binding activities (Boutillon et al., 2022). The etv6 gene plays a role in embryonic hemopoiesis (De Braekeleer et al., 2012). The lrig3 gene may be involved in craniofacial and inner ear morphogenesis during embryonic development (Abraira et al., 2010). Additionally, for BW, we identified three genes around the top SNP on chromosome 23: myf6, myf5, and igf1. The myf6 gene is important for skeletal muscle tissue development (Wei et al., 2024). The myf5 gene is involved in mesoderm development, muscle organ development, and neural crest cell development (Wei et al., 2022). The igf1 gene exhibits insulin-like growth factor receptor binding activity, contributing to dorsal and ventral pattern formation and positive regulation of cell proliferation (Duran et al., 2022).
For body length (BL) traits, we identified ntf3 on chromosome 23. The ntf3 gene is predicted to have growth factor activity and nerve growth factor receptor binding activity, and is involved in negative regulation of programmed cell death and peripheral nervous system development (Cacialli and Lucini, 2022). Additionally, two genes on chromosome 21 related to BL were highlighted: homer and znf703. The homer gene is involved in muscle structure development (Lin et al., 2013). The znf703 gene participates in cilium assembly and embryonic camera-type eye morphogenesis (Nakamura et al., 2008).
For body height (BH) traits, we identified the gene cavin4b on chromosome 1. The cavin4b gene is involved in T-tubule organization and skeletal muscle fiber development (Lo et al., 2021).
For body thickness (BT) traits, we identified two genes on chromosome 16: prdm16 and edn3b. The prdm16 gene is involved in cardiac muscle cell proliferation, embryonic cranial skeleton morphogenesis, and hematopoietic stem cell differentiation (Shull et al., 2022). The edn3b gene plays a role in melanocyte differentiation and pigment cell development (Krauss et al., 2014). Additionally, we identified three genes on chromosome 1 for BT: shisa2, flt3, and flt1. The shisa2 gene is involved in negative regulation of fibroblast growth factor receptor signaling (Katoh and Katoh, 2005). The flt3 gene is associated with macrophage differentiation and myeloid cell development (Peng et al., 2015). The flt1 gene regulates tip cell formation and arterial branching morphogenesis (Krueger et al., 2011).
For caudal peduncle length (CPL) traits, we identified igf2r on chromosome 14. We also identified two genes on chromosome 3 related to CPL: nhsl2 and irs2. The nhsl2 gene is predicted to be involved in cell differentiation (Brooks et al., 2010). The irs2 gene is predicted to have insulin receptor binding activity (Zasu et al., 2022). Additionally, three genes on chromosome 19 were identified for CPL: col22a1, paqr7a, and kdf1b. For caudal peduncle height (CPH) traits, we identified two genes on chromosome 18: cdk5 and fev. The cdk5 gene plays a role in nervous system development (Huang et al., 2019). The fev gene is involved in hematopoietic stem cell differentiation (Wang et al., 2013).
4 Discussion
Improving the growth of largemouth bass is a key objective in the industry, yet effective breeding methods for this species have been lacking. In this study, we utilized genome-wide association studies (GWAS) to identify chromosomal regions associated with growth traits in largemouth bass. Generally, growth traits are complex and influenced by minor genetic factors. Our analysis revealed 24 candidate genes related to growth, including genes involved in growth factors, myogenic factors, neurotrophic factors, and other growth-related functions.
False negatives can arise in GWAS when multiple hypothesis testing methods are employed to establish statistical significance. The two commonly used methods are the Bonferroni correction and the false discovery rate (FDR). However, overly conservative thresholds can lead to a high rate of false negatives. Initially, we applied the Bonferroni correction to set a significance threshold of 2.76e-8 (-log10(p-value) = 7.5), calculated as 0.05 divided by the number of tested SNPs (1,809,166). And no SNPs with high p-values met the significance criteria for any traits. To refine the significance level for our GWAS results, we focused on effective SNPs by pruning those based on high linkage disequilibrium (LD) to identify SNPs representing high LD values (Xiang et al., 2020; Wang et al., 2022). We determined a genome-wide significant threshold of 6.42e−7 (0.05 divided by the number of effective SNPs). Using this threshold, we identified 12 genes associated with specific growth traits. For the remaining growth traits that did not meet the significant threshold, we selected lead SNPs based on the p-values and clustering of SNPs.
Based on phenotypic correlations, body weight (BW) was found to be highly correlated with both body height (BH) and chest perimeter (CPH). Our GWAS results mapped these traits to two SNP clusters located on chromosome 23. Within these regions, we identified a total of six genes. BW and BH shared a common gene, while CPH was associated with one additional gene compared to BW and BH. The identified genes include rerg, jup, cd63, etv6, and lrig3.
Additionally, the lead SNPs for BW, BH, and CPH were found in another SNP cluster on chromosome 23, specifically at position 20,121,867. In this cluster, we identified three genes: myf6, myf5, and igf1. For body length (BL), the lead SNP, which did not reach the significant threshold on chromosome 23, was located at position 7,805,143. This region was associated with the ntf3 gene.
Previous studies have demonstrated that growth traits are controlled by multiple genes. Growth factors, which are polypeptides regulating cellular behaviors positively or negatively, play crucial roles in development (Junjie and Shengjie, 2019). Our study identified four key genes directly related to growth and muscle development: insulin-like growth factor I (igf1), cation-independent mannose-6-phosphate receptor (igf2r) (also known as the insulin-like growth factor 2 receptor), myogenic factor 5 (myf5), and myogenic factor 6 (myf6).
The growth hormone (GH)/insulin-like growth factor-I (IGF-1) endocrine axis is crucial for regulating growth in fish. IGF-1, produced in response to pituitary GH stimulation of the liver, mediates the growth-promoting effects of GH (Moriyama et al., 2000; Reinecke, 2010). Insulin-like growth factors 1 and 2 have also been linked to testicular germ cell proliferation and apoptosis in fish reproduction (Moreira et al., 2020), and IGF-1 has been shown to regulate muscle growth through integrative transcriptome and microRNAome analyses in Pacu (Piaractus mesopotamicus) (Duran et al., 2022). A transcriptomic study of largemouth bass suggested that the GH-IGF-1 axis and its signaling pathway are associated with muscle growth development (Li et al., 2017). This study observed that IGF2R was downregulated and IGFBP1 was upregulated in fast-growing largemouth bass, while IGF2R was upregulated in slow-growing fish (Li et al., 2017). Our investigation also identified the igf2r gene on chromosome 14 associated with caudal peduncle length (CPL), predicted to have IGF binding activity and involvement in lysosomal transport. The igf2r gene is predicted to have insulin-like growth factor binding activity (Rajeswari et al., 2022). Li et al. (2017) reported increased IGF2R expression in slow-growing largemouth bass. Similarly, Azizi et al. (2016) observed elevated IGF2R expression during myogenesis in gilthead sea bream, suggesting that IGF-2 stimulates muscle growth. In Atlantic salmon, IGF2R transcripts were upregulated during prolonged fasting and downregulated after 7 days of refeeding, indicating that IGF2R may act as a negative regulator for IGF-2 (Abu El-Magd et al., 2014). These findings suggest that both IGF1 and IGF2R play significant roles in the growth of largemouth bass.
Fish meat quality is influenced by the composition and content of nutrients such as amino acids and fatty acids. At the molecular level, the growth and differentiation of fish muscle are regulated by myogenic regulatory factors (MRFs). MRFs affect muscle growth and meat quality (Tan and Du, 2002).
Our study identified two MRFs genes, myf5 and myf6, related to the initiation and development of skeletal muscle. Myf5 is expressed primarily in satellite cells and muscle spindles (Beauchamp et al., 2000; Zammit et al., 2004), while myf6 is involved in muscle cell differentiation and has similar functions to MYOG (Lazure et al., 2020). Moghadam et al. (2007) investigated igf1, igf2, myf5, myf6, and GRF/PACAP in rainbow trout (Oncorhynchus mykiss), Atlantic salmon (Salmo salar), and Arctic charr (Salvelinus alpinus), demonstrating that igf1, myf5, and myf6 are critical for growth in these species.
We also identified genes related to CPL trait: nhsl2, irs2, col22a1, paqr7a, and kdf1b. The nhsl2 gene is predicted to be involved in cell differentiation (Brooks et al., 2010). The irs2 gene is predicted to have insulin receptor binding activity (Zasu et al., 2022). The col22a1 gene is involved in embryonic caudal fin morphogenesis, muscle attachment, and fin regeneration (Charvet et al., 2013). The paqr7a gene may be involved in oocyte maturation (Wang et al., 2021). The kdf1b gene is predicted to regulate epidermal cell division (Shamseldin et al., 2017).
In summary, we have identified significant SNPs and candidate genes associated with growth traits through GWAS. To validate these SNPs, future studies should include growth phenotypes and genotypes from additional largemouth bass cohorts. These SNPs can be employed in genomic selection (GS) to enhance growth in largemouth bass populations, offering a rapid and accurate method for assessing genetic value. Our functional annotations indicate that growth and myogenic factors directly impact the growth and development of largemouth bass, while other candidate genes are involved in tissue and organ development and various physiological processes. This GWAS provides a foundation for the selection and breeding of largemouth bass.
5 Conclusion
In this study, we utilized a total of 4.9 Terabytes of clean data from 1066 individuals and obtained 1,809,166 SNPs after variant calling and quality control. Based on this, we found 24 candidate genes (rerg, jup, etv6, lrig3, myf6, myf5, igf1, ntf3, homer, znf703, cavin4b, prdm16, edn3b, shisa2, flt3, flt1, igf2r, nhsl2, irs2, col22a1, paqr7a, kdf1b, cdk5,and fev) that are related to the growth traits of largemouth bass, as determined by the Genome-wide association study (GWAS) results. Three genes, namely, igf1, myf5, and myf6, which are directly associated with the development of skeletal muscle and individual growth, were located around the lead SNP on chromosome 23. These findings provide useful information for selection and breeding in largemouth bass.
Data availability statement
The datasets presented in this study can be found in online repositories. The names of the repository/repositories and accession number(s) can be found below: https://www.ncbi.nlm.nih.gov/bioproject, PRJNA748185.
Ethics statement
The animal study was approved by the Animal Care and Use Committee of Jimei University. The study was conducted in accordance with the local legislation and institutional requirements.
Author contributions
WH: Data curation, Formal Analysis, Investigation, Methodology, Resources, Writing–original draft, Writing–review and editing. MQ: Data curation, Investigation, Writing–original draft, Writing–review and editing. KY: Investigation, Resources, Writing–review and editing. QH: Methodology, Resources, Writing–review and editing. DY: Methodology, Resources, Writing–review and editing. DX: Data curation, Resources, Writing–review and editing. FH: Conceptualization, Formal Analysis, Investigation, Writing–original draft, Writing–review and editing. WL: Conceptualization, Formal Analysis, Funding acquisition, Supervision, Writing–original draft, Writing–review and editing.
Funding
The author(s) declare that financial support was received for the research, authorship, and/or publication of this article. This work was supported by the National Natural Science Foundation of China (No. 32072969) and Fujian Provincial Department of Education (No. JAT210242).
Acknowledgments
Chatgpt 3.5 of OpenAI was used for grammar checks.
Conflict of interest
The authors declare that the research was conducted in the absence of any commercial or financial relationships that could be construed as a potential conflict of interest.
Publisher’s note
All claims expressed in this article are solely those of the authors and do not necessarily represent those of their affiliated organizations, or those of the publisher, the editors and the reviewers. Any product that may be evaluated in this article, or claim that may be made by its manufacturer, is not guaranteed or endorsed by the publisher.
Supplementary material
The Supplementary Material for this article can be found online at: https://www.frontiersin.org/articles/10.3389/fmolb.2024.1443522/full#supplementary-material
References
Abraira, V. E., Satoh, T., Fekete, D. M., and Goodrich, L. V. (2010). Vertebrate Lrig3-ErbB interactions occur in vitro but are unlikely to play a role in Lrig3-dependent inner ear morphogenesis. PLoS One 5 (2), e8981. doi:10.1371/journal.pone.0008981
Abu El-Magd, M., Abo-Al-Ela, H. G., El-Nahas, A., Saleh, A. A., and Mansour, A. A. (2014). Effects of a novel SNP of IGF2R gene on growth traits and expression rate of IGF2R and IGF2 genes in gluteus medius muscle of Egyptian buffalo. Gene 540 (2), 133–139. doi:10.1016/j.gene.2014.02.059
Azizi, S., Nematollahi, M. A., Amiri, B. M., Vélez, E. J., Salmerón, C., Chan, S. J., et al. (2016). IGF-I and IGF-II effects on local IGF system and signaling pathways in gilthead sea bream (Sparus aurata) cultured myocytes. General Comp. Endocrinol. 232, 7–16. doi:10.1016/j.ygcen.2015.11.011
Bai, J. J., Lutz-Carrillo, D. J., Quan, Y. C., and Liang, S. X. (2008). Taxonomic status and genetic diversity of cultured largemouth bass Micropterus salmoides in China. Aquaculture 278 (1-4), 27–30. doi:10.1016/j.aquaculture.2008.03.016
Beauchamp, J. R., Heslop, L., Yu, D. S. W., Tajbakhsh, S., Kelly, R. G., Wernig, A., et al. (2000). Expression of CD34 and Myf5 defines the majority of quiescent adult skeletal muscle satellite cells. J. Cell Biol. 151 (6), 1221–1234. doi:10.1083/jcb.151.6.1221
Bolger, A. M., Lohse, M., and Usadel, B. (2014). Trimmomatic: a flexible trimmer for Illumina sequence data. Bioinformatics 30 (15), 2114–2120. doi:10.1093/bioinformatics/btu170
Boutillon, A., Escot, S., Elouin, A., Jahn, D., González-Tirado, S., Starruß, J., et al. (2022). Guidance by followers ensures long-range coordination of cell migration through α-catenin mechanoperception. Dev. Cell 57 (12), 1529–1544.e5. doi:10.1016/j.devcel.2022.05.001
Brooks, S. P., Coccia, M., Tang, H. R., Kanuga, N., Machesky, L. M., Bailly, M., et al. (2010). The Nance-Horan syndrome protein encodes a functional WAVE homology domain (WHD) and is important for co-ordinating actin remodelling and maintaining cell morphology. Hum. Mol. Genet. 19 (12), 2421–2432. doi:10.1093/hmg/ddq125
Buniello, A., MacArthur, J. A. L., Cerezo, M., Harris, L. W., Hayhurst, J., Malangone, C., et al. (2019). The NHGRI-EBI GWAS Catalog of published genome-wide association studies, targeted arrays and summary statistics 2019. Nucleic Acids Res. 47 (D1), D1005-D1012–D1012. doi:10.1093/nar/gky1120
Cacialli, P., and Lucini, C. (2022). Analysis of the expression of neurotrophins and their receptors in adult zebrafish kidney. Vet. Sci. 9 (6), 296. doi:10.3390/vetsci9060296
Charvet, B., Guiraud, A., Malbouyres, M., Zwolanek, D., Guillon, E., Bretaud, S., et al. (2013). Knockdown of col22a1 gene in zebrafish induces a muscular dystrophy by disruption of the myotendinous junction. Development 140 (22), 4602–4613. doi:10.1242/dev.096024
Danecek, P., Bonfield, J. K., Liddle, J., Marshall, J., Ohan, V., Pollard, M. O., et al. (2021). Twelve years of SAMtools and BCFtools. Gigascience 10 (2), giab008. doi:10.1093/gigascience/giab008
Davies, R. W., Flint, J., Myers, S., and Mott, R. (2016). Rapid genotype imputation from sequence without reference panels. Nat. Genet. 48(8), 965. 969. doi:10.1038/ng.3594
De Braekeleer, E., Douet-Guilbert, N., Morel, F., Le Bris, M. J., Basinko, A., and De Braekeleer, M. (2012). ETV6 fusion genes in hematological malignancies: a review. Leuk. Res. 36 (8), 945–961. doi:10.1016/j.leukres.2012.04.010
DePristo, M. A., Banks, E., Poplin, R., Garimella, K. V., Maguire, J. R., Hartl, C., et al. (2011). A framework for variation discovery and genotyping using next-generation DNA sequencing data. Nat. Genet. 43(5), 491. 498. doi:10.1038/ng.806
de Vries, A. N., Govoni, D., Árnason, S. H., and Carlsson, P. (2020). Microplastic ingestion by fish: body size, condition factor and gut fullness are not related to the amount of plastics consumed. Mar. Pollut. Bull. 151, 110827. doi:10.1016/j.marpolbul.2019.110827
Dong, C. J., Jiang, P., Zhang, J. F., Li, X. J., Li, S. J., Bai, J. J., et al. (2019). High-density linkage map and mapping for sex and growth-related traits of largemouth bass (Micropterus salmoides). Front. Genet. 10, 960. doi:10.3389/fgene.2019.00960
Duran, B. O. S., Zanella, B. T. T., Perez, E. S., Mareco, E. A., Blasco, J., Dal-Pai-Silva, M., et al. (2022). Amino acids and IGF1 regulation of fish muscle growth revealed by transcriptome and microRNAome integrative analyses of Pacu (piaractus mesopotamicus) myotubes. Int. J. Mol. Sci. 23 (3), 1180. doi:10.3390/ijms23031180
Finlin, B. S., Gau, C. L., Murphy, G. A., Shao, H., Kimel, T., Seitz, R. S., et al. (2001). RERG is a novel ras-related, estrogen-regulated and growth-inhibitory gene in breast cancer. J. Biol. Chem. 276 (45), 42259–42267. doi:10.1074/jbc.M105888200
He, Q. W., Ye, K., Han, W., Yekefenhazi, D., Sun, S., Xu, X. D., et al. (2022). Mapping sex-determination region and screening DNA markers for genetic sex identification in largemouth bass (Micropterus salmoides). Aquaculture 559, 738450. doi:10.1016/j.aquaculture.2022.738450
Huang, P. P., Guo, W. J., Wang, Y. H., Xiong, Y., Ge, S., Gong, G. R., et al. (2022). Genome-wide association study reveals the genetic basis of growth trait in yellow catfish with sexual size dimorphism. Genomics 114 (3), 110380. doi:10.1016/j.ygeno.2022.110380
Huang, V., Butler, A. A., and Lubin, F. D. (2019). Telencephalon transcriptome analysis of chronically stressed adult zebrafish. Sci. Rep. 9 (1), 1379. doi:10.1038/s41598-018-37761-7
Jiang, L. D., Zheng, Z. L., Qi, T., Kemper, K. E., Wray, N. R., Visscher, P. M., et al. (2019). A resource-efficient tool for mixed model association analysis of large-scale data. Nat. Genet. 51(12), 1749. doi:10.1038/s41588-019-0530-8
Junjie, B., and Shengjie, L. (2019) “The genetic parameters of growth traits and breeding value estimation in largemouth bass (Micropterus salmoides),” in Genetic breeding and molecular marker-assisted selective breeding of largemouth bass.
Katoh, Y., and Katoh, M. (2005). Comparative genomics on Shisa orthologs. Int. J. Mol. Med. 16 (1), 181–185. doi:10.3892/ijmm.16.1.181
Krauss, J., Frohnhöfer, H. G., Walderich, B., Maischein, H. M., Weiler, C., Irion, U., et al. (2014). Endothelin signalling in iridophore development and stripe pattern formation of zebrafish. Biol. Open 3 (6), 503–509. doi:10.1242/bio.20148441
Krueger, J., Liu, D., Scholz, K., Zimmer, A., Shi, Y., Klein, C., et al. (2011). Flt1 acts as a negative regulator of tip cell formation and branching morphogenesis in the zebrafish embryo. Development 138 (10), 2111–2120. doi:10.1242/dev.063933
Lazure, F., Blackburn, D. M., Corchado, A. H., Sahinyan, K., Karam, N., Sharanek, A., et al. (2020). Myf6/MRF4 is a myogenic niche regulator required for the maintenance of the muscle stem cell pool. Embo Rep. 21 (12), e49499. doi:10.15252/embr.201949499
Li, H., and Durbin, R. (2009). Fast and accurate short read alignment with Burrows-Wheeler transform. Bioinformatics 25 (14), 1754–1760. doi:10.1093/bioinformatics/btp324
Li, S. J., Liu, H., Bai, J. J., and Zhu, X. P. (2017). Transcriptome assembly and identification of genes and SNPs associated with growth traits in largemouth bass (Micropterus salmoides). Genetica 145 (2), 175–187. doi:10.1007/s10709-017-9956-z
Lin, C. Y., Chen, J. S., Loo, M. R., Hsiao, C. C., Chang, W. Y., and Tsai, H. J. (2013). MicroRNA-3906 regulates fast muscle differentiation through modulating the target gene homer-1b in zebrafish embryos. PLoS One 8 (7), e70187. doi:10.1371/journal.pone.0070187
Lo, H. P., Lim, Y. W., Xiong, Z., Martel, N., Ferguson, C., Ariotti, N., et al. (2021). Cavin4 interacts with Bin1 to promote T-tubule formation and stability in developing skeletal muscle. J. Cell Biol. 220 (12), e201905065. doi:10.1083/jcb.201905065
McKenna, A., Hanna, M., Banks, E., Sivachenko, A., Cibulskis, K., Kernytsky, A., et al. (2010). The Genome Analysis Toolkit: a MapReduce framework for analyzing next-generation DNA sequencing data. Genome Res. 20 (9), 1297–1303. doi:10.1101/gr.107524.110
Moghadam, H. K., Ferguson, M. M., Rexroad, C. E., Coulibaly, I., and Danzmann, R. G. (2007). Genomic organization of the IGF1, IGF2, MYF5, MYF6 and GRF/PACAP genes across Salmoninae genera. Anim. Genet. 38 (5), 527–532. doi:10.1111/j.1365-2052.2007.01645.x
Moreira, D. P., Melo, R. M. C., Weber, A. A., and Rizzo, E. (2020). Insulin-like growth factors 1 and 2 are associated with testicular germ cell proliferation and apoptosis during fish reproduction. Reproduction Fertil. Dev. 32 (11), 988–998. doi:10.1071/rd20128
Moriyama, S., Ayson, F. G., and Kawauchi, H. (2000). Growth regulation by insulin-like growth factor-I in fish. Biosci. Biotechnol. Biochem. 64 (8), 1553–1562. doi:10.1271/bbb.64.1553
Nakamura, M., Choe, S. K., Runko, A. P., Gardner, P. D., and Sagerström, C. G. (2008). Nlz1/Znf703 acts as a repressor of transcription. BMC Dev. Biol. 8, 108. doi:10.1186/1471-213x-8-108
Peng, X., Dong, M., Ma, L., Jia, X. E., Mao, J., Jin, C., et al. (2015). A point mutation of zebrafish c-cbl gene in the ring finger domain produces a phenotype mimicking human myeloproliferative disease. Leukemia 29 (12), 2355–2365. doi:10.1038/leu.2015.154
Rajeswari, J. J., Vélez, E. J., and Unniappan, S. (2022). Liver and muscle-specific effects of phoenixin-20 on the insulin-like growth factor system mRNAs in zebrafish. Growth Horm. IGF Res. 63, 101456. doi:10.1016/j.ghir.2022.101456
Reinecke, M. (2010). Insulin-like growth factors and fish reproduction. Biol. Reproduction 82 (4), 656–661. doi:10.1095/biolreprod.109.080093
Shamseldin, H. E., Khalifa, O., Binamer, Y. M., Almutawa, A., Arold, S. T., Zaidan, H., et al. (2017). KDF1, encoding keratinocyte differentiation factor 1, is mutated in a multigenerational family with ectodermal dysplasia. Hum. Genet. 136 (1), 99–105. doi:10.1007/s00439-016-1741-z
Shim, H., Chasman, D. I., Smith, J. D., Mora, S., Ridker, P. M., Nickerson, D. A., et al. (2015). A multivariate genome-wide association analysis of 10 LDL subfractions, and their response to statin treatment, in 1868 caucasians. Plos One 10 (4), e0120758. doi:10.1371/journal.pone.0120758
Shull, L. C., Lencer, E. S., Kim, H. M., Goyama, S., Kurokawa, M., Costello, J. C., et al. (2022). PRDM paralogs antagonistically balance Wnt/β-catenin activity during craniofacial chondrocyte differentiation. Development 149 (4), dev200082. doi:10.1242/dev.200082
Sodeland, M., Gaarder, M., Moen, T., Thomassen, M., Kjoglum, S., Kent, M., et al. (2013). Genome-wide association testing reveals quantitative trait loci for fillet texture and fat content in Atlantic salmon. Aquaculture 408, 169–174. doi:10.1016/j.aquaculture.2013.05.029
Tan, X. G., and Du, S. J. (2002). Differential expression of two MyoD genes in fast and slow muscles of gilthead seabream (Sparus aurata). Dev. Genes Evol. 212 (5), 207–217. doi:10.1007/s00427-002-0224-5
Tsai, H. Y., Hamilton, A., Tinch, A. E., Guy, D. R., Gharbi, K., Stear, M. J., et al. (2015). Genome wide association and genomic prediction for growth traits in juvenile farmed Atlantic salmon using a high density SNP array. Bmc Genomics 16, 969. doi:10.1186/s12864-015-2117-9
Visscher, P. M., Brown, M. A., McCarthy, M. I., and Yang, J. (2012). Five years of GWAS discovery. Am. J. Hum. Genet. 90 (1), 7–24. doi:10.1016/j.ajhg.2011.11.029
Wan, L., Dong, L. S., Xiao, S. J., Han, Z. F., Wang, X. Q., and Wang, Z. Y. (2018). Genomewide association study for economic traits in the large yellow croaker with different numbers of extreme phenotypes. J. Genet. 97 (4), 887–895. doi:10.1007/s12041-018-0973-1
Wang, C. M., Lo, L. C., Zhu, Z. Y., and Yue, G. H. (2006). A genome scan for quantitative trait loci affecting growth-related traits in an F1 family of Asian seabass (Lates calcarifer). Bmc Genomics 7, 274. doi:10.1186/1471-2164-7-274
Wang, L., Liu, T., Xu, L., Gao, Y., Wei, Y., Duan, C., et al. (2013). Fev regulates hematopoietic stem cell development via ERK signaling. Blood 122 (3), 367–375. doi:10.1182/blood-2012-10-462655
Wang, Y., Luo, T., Zheng, L., Huang, J., Zheng, Y., and Li, J. (2021). PAQR7: an intermediary mediating nongenomic progesterone action in female reproductive tissue. Reprod. Biol. 21 (3), 100529. doi:10.1016/j.repbio.2021.100529
Wang, Y. Y., Wang, X. L., Sun, S., Jin, C. Z., Su, J. M., Wei, J. P., et al. (2022). GWAS, MWAS and mGWAS provide insights into precision agriculture based on genotype-dependent microbial effects in foxtail millet. Nat. Commun. 13 (1), 5913. doi:10.1038/s41467-022-33238-4
Wei, L. J., Xiao, W., Chen, B. L., Zou, Z. Y., Zhu, J. L., Li, D. Y., et al. (2024). Single nucleotide polymorphisms in the MRFs gene family associated with growth in Nile tilapia. Mol. Biol. Rep. 51 (1), 128. doi:10.1007/s11033-023-08955-8
Wen, M., Zhang, Y. X., Wang, S. Y., Hu, F. Z., Tang, C. J., Li, Q., et al. (2022). Sex locus and sex markers identification using whole genome pool-sequencing approach in the largemouth bass (Micropterus Salmoides L.). Aquaculture 559, 738375. doi:10.1016/j.aquaculture.2022.738375
Xiang, R. D., van den Berg, I., MacLeod, I. M., Daetwyler, H. D., and Goddard, M. E. (2020). Effect direction meta-analysis of GWAS identifies extreme, prevalent and shared pleiotropy in a large mammal. Commun. Biol. 3 (1), 88. doi:10.1038/s42003-020-0823-6
Xie, S. W., Yin, P., Tian, L. X., Yu, Y. Y., Liu, Y. J., and Niu, J. (2020). Dietary supplementation of astaxanthin improved the growth performance, antioxidant ability and immune response of juvenile largemouth bass (Micropterus salmoides) fed high-fat diet. Mar. Drugs 18 (12), 642. doi:10.3390/md18120642
Yang, B., Wu, Y.-J., Zhu, M., Fan, S.-B., Lin, J., Zhang, K., et al. (2012). Identification of cross-linked peptides from complex samples. Nat. Methods 9 (9), 904–906. doi:10.1038/nmeth.2099
Yang, J. A., Lee, S. H., Goddard, M. E., and Visscher, P. M. (2011). GCTA: a tool for genome-wide complex trait analysis. Am. J. Hum. Genet. 88 (1), 76–82. doi:10.1016/j.ajhg.2010.11.011
Yin, P., Xie, S. W., Zhuang, Z. X., Fang, H. H., Tian, L. X., Liu, Y. J., et al. (2021). Chlorogenic acid improves health in juvenile largemouth bass (Micropterus salmoides) fed high-fat diets: involvement of lipid metabolism, antioxidant ability, inflammatory response, and intestinal integrity. Aquaculture 545, 737169. doi:10.1016/j.aquaculture.2021.737169
Young, W. J., Lahrouchi, N., Isaacs, A., Duong, T. V., Foco, L., Ahmed, F., et al. (2022). Genetic analyses of the electrocardiographic QT interval and its components identify additional loci and pathways. Nat. Commun. 13 (1), 5144. doi:10.1038/s41467-022-32821-z
Zammit, P. S., Carvajal, J. J., Golding, J. P., Morgan, J. E., Summerbell, D., Zolnerciks, J., et al. (2004). Myf5 expression in satellite cells and spindles in adult muscle is controlled by separate genetic elements. Dev. Biol. 273 (2), 454–465. doi:10.1016/j.ydbio.2004.05.038
Zasu, A., Hishima, F., Thauvin, M., Yoneyama, Y., Kitani, Y., Hakuno, F., et al. (2022). NADPH-oxidase derived hydrogen peroxide and Irs2b facilitate Re-oxygenation-Induced catch-up growth in zebrafish embryo. Front. Endocrinol. (Lausanne) 13, 929668. doi:10.3389/fendo.2022.929668
Keywords: GWAS, growth traits, largemouth bass, IGF1, breeding
Citation: Han W, Qi M, Ye K, He Q, Yekefenhazi D, Xu D, Han F and Li W (2024) Genome-wide association study for growth traits with 1066 individuals in largemouth bass (Micropterus salmoides). Front. Mol. Biosci. 11:1443522. doi: 10.3389/fmolb.2024.1443522
Received: 04 June 2024; Accepted: 12 September 2024;
Published: 25 September 2024.
Edited by:
Ying Lu, Shanghai Ocean University, ChinaReviewed by:
Suxu Tan, Qingdao University, ChinaIrina Nakashidze, Shota Rustaveli State University, Georgia
Copyright © 2024 Han, Qi, Ye, He, Yekefenhazi, Xu, Han and Li. This is an open-access article distributed under the terms of the Creative Commons Attribution License (CC BY). The use, distribution or reproduction in other forums is permitted, provided the original author(s) and the copyright owner(s) are credited and that the original publication in this journal is cited, in accordance with accepted academic practice. No use, distribution or reproduction is permitted which does not comply with these terms.
*Correspondence: Fang Han, aGFuZmFuZ3ljQGptdS5lZHUuY24=; Wanbo Li, bGkud2FuYm9AZm94bWFpbC5jb20=
†These authors have contributed equally to this work