- Department of Bioengineering, James H. Clark Center, Stanford University, Stanford, CA, United States
Advances in cryogenic electron microscopy (cryoEM) single particle analysis have revolutionized structural biology by facilitating the in vitro determination of atomic- and near-atomic-resolution structures for fully hydrated macromolecular complexes exhibiting compositional and conformational heterogeneity across a wide range of sizes. Cryogenic electron tomography (cryoET) and subtomogram averaging are rapidly progressing toward delivering similar insights for macromolecular complexes in situ, without requiring tags or harsh biochemical purification. Furthermore, cryoET enables the visualization of cellular and tissue phenotypes directly at molecular, nanometric resolution without chemical fixation or staining artifacts. This forward-looking review covers recent developments in cryoEM/ET and related technologies such as cryogenic focused ion beam milling scanning electron microscopy and correlative light microscopy, increasingly enhanced and supported by artificial intelligence algorithms. Their potential application to emerging concepts is discussed, primarily the prospect of complementing medical histopathology analysis. Machine learning solutions are poised to address current challenges posed by “big data” in cryoET of tissues, cells, and macromolecules, offering the promise of enabling novel, quantitative insights into disease processes, which may translate into the clinic and lead to improved diagnostics and targeted therapeutics.
1 Introduction
Pathology is the medical specialty that studies the nature and causes of disease and has played a pivotal role in understanding, diagnosing, and treating diseases since antiquity, when early healers and philosophers began to contemplate disease etiology (van den Tweel and Taylor, 2010). Although Renaissance scholars revolutionized anatomical studies with detailed autopsies and dissections, it was botanist Matthias Schleiden, zoologist Theodor Schwann, and pathologist Rudolf Virchow who pioneered the Cell Theory in the first half of the 19th century, thereby laying down the cornerstone for modern cellular pathology (Ribatti, 2018). At the microscopic level, histology and the field of cellular pathology, also referred to as histopathology, analyze patient tissues to understand the structural and functional alterations associated with diseases (Musumeci, 2014). While histopathology and the experimental laboratory techniques it routinely relies on are already being potentiated by rapid advances in computer science and artificial intelligence (AI) (de Matos et al., 2021), unique opportunities will be forthcoming to enhance cellular pathology as machine learning (ML) methods also extend the capabilities of cryogenic electron microscopy (cryoEM) and tomography (cryoET) and related techniques, facilitating the efficient, quantitative processing of patient derived imaging datasets.
1.1 Techniques in structural, cellular pathology
In terms of structural characterization, histologists and histopathologists most commonly use light and/or fluorescence microscopy (LM and FM, respectively), which can be complemented by electron microscopy (EM) to gain insights at higher resolution than that allowed by the former techniques (Chen et al., 2011). Light microscopy uses visible light to visualize overall tissue architecture and cellular morphology, sufficing to detect relatively large abnormal structures such as tumors or infectious agents including bacteria and parasites. Moreover, FM utilizes fluorescent dyes or proteins to visualize specific molecules or structures within cells and tissues. These microscopy methods are commonly complemented by various molecular biology techniques to enhance specificity and detail. For instance, immunohistochemistry (IHC) is frequently employed to identify specific proteins in samples using antibodies labeled with fluorescent or chromogenic markers (Hofman and Taylor, 2013). Additionally, in situ hybridization (ISH) enables the detection of specific nucleic acid sequences within tissue samples by hybridizing labeled nucleic acid probes to complementary target sequences in cells (Jensen, 2014). While IHC enables pathologists to identify cell types, characterize tumors, and assess protein expression patterns, ISH allows visualizing infectious agents as well as gene expression patterns and chromosomal abnormalities to diagnose genetic disorders.
Pathologists also routinely use traditional transmission electron microscopy (TEM) and, occasionally, scanning EM (SEM) to visualize thin tissue sections (Graham and Orenstein, 2007). TEM provides detailed images of internal cellular structures, making it particularly valuable for investigating ultrastructural alterations suggestive of disease. Moreover, EM can be integrated with LM/FM through multi-scale correlative approaches that facilitate molecular assignments in high-resolution EM images by aligning them with fluorophore signals (Giepmans et al., 2005). While high-magnification EM can routinely achieve atomic resolution visualization (∼1 Å) for samples in material sciences, including those that form strong, repetitive lattices (Spence, 1999; Egerton and Watanabe, 2022), biological specimens are more susceptible to deformation from radiation damage even when stained or fixed (Luther, 2006), and are typically visualized at lower magnifications that can capture large sections of tissues, entire cells, organelles, and macromolecular complexes, yielding resolutions at nanometric scale. Furthermore, since IHC and ISH rely on fluorescent signals, histopathological observations using these methodologies are diffraction-limited to ∼200–500 nm resolution, depending on the wavelength of light used in the optical microscope.
Although traditional tissue-based evaluations of histopathology slides through direct microscopy observation, typically using single biomarkers, do not lend themselves to complex quantitative analyses, advances in staining techniques allowing for multiplex immunohistochemistry and immunofluorescence have enhanced the diagnostic accuracy and specificity of cellular pathology and will soon become routine in the clinic (Harms et al., 2023). Furthermore, the digital revolution of the last few decades has permeated every facet of medicine, and pathology is no exception. With the emergence of digital pathology (Hanna and Pantanowitz, 2019) and whole-slide imaging (Madabhushi and Lee, 2016), encompassing the digitization and computational visualization of histological slides, modern examinations are increasingly occurring virtually without being tethered to the microscope or the laboratory. This has ushered in a new era of telemedicine, efficiency, and remote collaborations in tissue-based diagnostics, and arguably represents the way of the future for the field (Pallua et al., 2020). Importantly, this digital revolution has rendered a plethora of histopathology data amenable to automated quantitative image analysis (Gurcan et al., 2009), thereby giving rise to computational pathology (Louis et al., 2014), which uses mathematical models and computational methods to extract relevant, clinically actionable information from various sources of raw data. The computational methods used include modern AI approaches (Niazi et al., 2019), such as ML leveraging deep convolutional neural networks (CNNs) (Banerji and Mitra, 2022). These applications are making their way into the clinic (van der Laak et al., 2021) and allowing for large-scale data mining to improve validation and derive population-level insights. These techniques streamline workflow efficiency by automating routine tasks, such as tissue segmentation and feature extraction, thereby augmenting pathologists’ diagnostic capabilities, speed, and accuracy.
1.2 Volume EM as a bridge toward high-resolution 3D histopathology
Volume electron microscopy (volume EM or VEM) refers to a group of cutting-edge imaging techniques that generate serial images of resin-embedded cellular or tissue specimens that are at least ∼1 μm thick (Peddie and Collinson, 2014). Collinson et al., 2023 highlighted this technique in a recent Nature Methods review, deeming it “a revolution in the making”. This recognition is well founded since VEM techniques are yielding unprecedented insights into cellular and subcellular architecture through large volumes of biological samples, in 3D, at the nanoscale level, in contrast to traditional EM, which typically provides two-dimensional (2D) projection images of thin samples. Indeed, while the number of publications containing VEM-related terms is still small, it’s increasing exponentially (Figure 1). Moreover, the number of publications containing terms referring to the main VEM techniques, namely, focused ion beam (FIB) scanning electron microscopy (SEM) and serial block-face (SBF) SEM, are much larger (Figure 1A). Another powerful albeit less common VEM technique is array tomography (Smith, 2018), for which publication numbers are also growing, although at a slower pace. Interestingly, when analyzing the scientific areas represented in the combined publications including terms for VEM, FIB-SEM, and SBF-SEM, “Medicine” is extremely underrepresented, accounting for merely 5.6% among the total number of publications using these techniques (Figure 1B). While cost, accessibility, and throughput remain a concern, such factors don’t seem to have impeded a more active use of these technologies in other fields. While this may be in part due to FIB milling being originally developed for room-temperature applications in material sciences, it also points to a relatively untapped trove of opportunities for biomedical imaging as VEM methods are made more accessible and efficient, especially through integration with AI-based strategies (Kievits et al., 2022), as demonstrated in a recent study analyzing hepatoblastoma patient tissues using ML for comprehensive segmentation of SBF-SEM reconstructions (de Senneville et al., 2021).
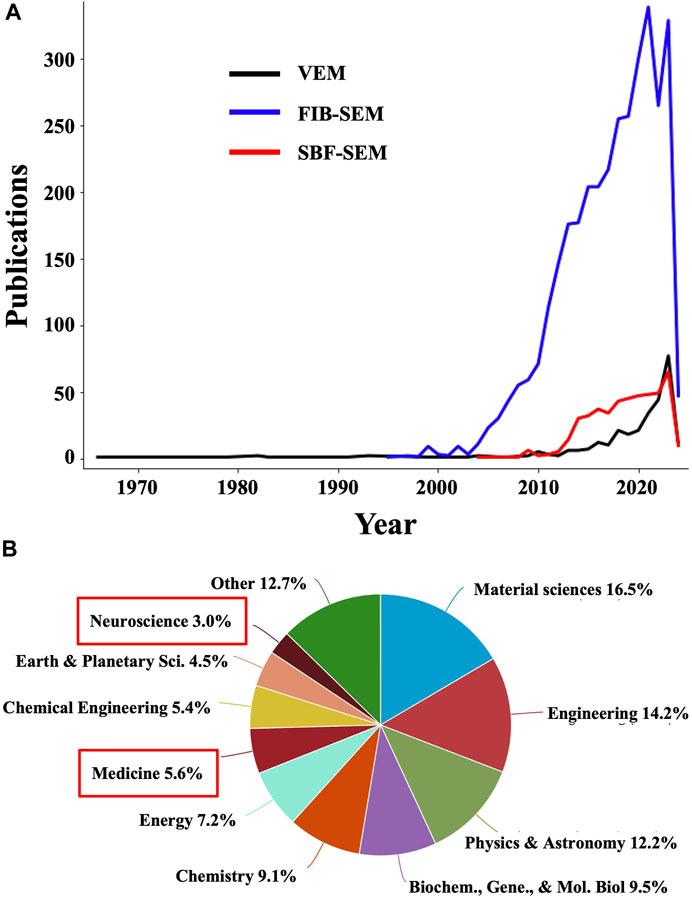
Figure 1. The number of publications using volume EM and related methodologies is undergoing exponential growth. (A) Plots showing the number of publications in Elsevier’s Scopus database containing “volume EM” OR “volume electron microscopy” OR “volumetric electron microscopy” (black line); “focused ion beam scanning electron microscopy” OR “FIB-SEM” OR “FIBSEM” OR “FIB SEM” OR “focused ion beam SEM” OR “FIB scanning electron microscopy” (blue line); and “serial block-face scanning electron microscopy” OR “serial block face scanning electron microscopy” OR “serial block face SEM” OR “serial block-face SEM” (red line). (B) Distribution of scientific disciplines represented in the combined publications in (A). Medicine and neuroscience, highlighted with red boxes, are underrepresented and thus constitute areas of opportunity for an increased application of VEM and related techniques.
In general, VEM generates serial sections from thick biological samples, which are imaged using TEM or SEM with datasets then being computationally aligned and reconstructed into 3D representations of the sample, allowing researchers to better visualize complex spatial relationships between cellular structures and thereby understand their organization within tissues with improved accuracy. While primarily utilized in specialized research settings and academic institutions for detailed investigations of tissue ultrastructure and molecular composition, VEM methods are rapidly becoming more efficient (Kornfeld and Denk, 2018) and are thus primed to enhance clinical histopathological examinations. They are particularly valuable for studying complex biological systems such as the brain, where figuring out the 3D arrangement of neurons and synapses across the entire “brain connectome” is critical for fully understanding brain function (Ohno et al., 2015). Another target that is well-suited for VEM approaches is the 3D tumor microenvironment (Jadav et al., 2023). Furthermore, recent applications of VEM have revealed structural defects in samples from patients with primary ciliary dyskinesia, autoimmune disorders, and neurodegenerative diseases that were not detectable with traditional EM on thin sections (Peddie et al., 2022). The growing use of ML to improve FIB-SEM analyses is increasingly allowing researchers to investigate whole-organelle morphologies (Heinrich et al., 2021), synaptic connectivity (Dorkenwald et al., 2017; Santuy et al., 2020), and other cellular features at an unprecedented scale and with unparalleled levels of detail, both necessary for advancing our understanding of the mechanisms underlying pathological processes. When translated into the clinic, these striking advances will enable further improvements in the specificity, sensitivity, and thoroughness of histopathological diagnoses.
1.3 Cryogenic EM/ET and FIB-SEM at the dawn of high-resolution cellular pathology
Cryogenic electron microscopy (cryoEM) (Frank, 2006; Bendory et al., 2020) and tomography (cryoET; Frank, 2008; Wagner et al., 2017; Turk and Baumeister, 2020) offer unique advantages for visualizing biological specimens in 3D at even higher resolution than traditional EM technologies, without artifacts from chemical fixation and with fewer mechanical manipulations. These techniques involve flash-freezing biological samples in fully hydrated, near-native states (Dubochet et al., 1981; McDowall et al., 1983; Dubochet et al., 1988). Single-particle analysis cryoEM (SPA) is a cutting-edge technique for in vitro macromolecular structure determination at atomic (Nakane et al., 2020; Yip et al., 2020) and near-atomic (Hryc and Baker, 2022; Li et al., 2023) resolutions. This method relies on extensive classification and averaging of hundreds to millions of 2D projections of individual instances of macromolecules, also referred to as “single particles”, typically floating in different orientations in solution before being vitrified by plunge freezing.
Technological advances ranging from better specimen support grids (Russo and Passmore, 2014; Yu et al., 2016; Noble et al., 2018; Doerr, 2019), cryopreservation devices (Jain et al., 2012; Darrow et al., 2019), detectors (Levin et al., 2023; Peng et al., 2023), microscopes (Fréchin et al., 2023) and associated hardware such as energy filters (Grimm et al., 1997), cold field emission guns (Hamaguchi et al., 2019; Kato et al., 2019), phase plates (Danev and Baumeister, 2017), and increasingly sophisticated and automated software for data collection (Thompson et al., 2019) and processing (Punjani et al., 2017) have reduced the cost of macromolecular structure determination by cryoEM SPA (Chua et al., 2022), turning it from a niche technique into a mainstream method (de la Cruz and Eng, 2023). Although SPA is unlikely to find immediate applications in histopathology, its technological developments spill over and benefit cryoET and related methodologies, the latter being predictably much more likely to revolutionize cellular pathology in the years to come.
CryoET visualizes specimens in 3D by computationally reconstructing tomograms from a series of multiple 2D images taken from the same target area at different angles (Kremer et al., 1996). By virtue of its three-dimensional nature, cryoET is uniquely capable of revealing the structures of pleomorphic systems, from cells (Kürner and Baumeister, 2006) to amyloid filaments (Shahmoradian et al., 2013; Darrow et al., 2015; Bäuerlein et al., 2017; Guo et al., 2018; Galaz-Montoya et al., 2021; Trinkaus et al., 2021) and enveloped viruses (Obr and Schur, 2019; Li, 2022), including those captured while infecting cells (Grünewald and Cyrklaff, 2006; Quemin et al., 2020), among many others. Indeed, cryoET is particularly well suited for studying spatial relationships between macromolecular complexes and cellular organelles, as well as dynamic processes such as viral infection (Murata et al., 2017; Graham and Zhang, 2023; Hernandez-Gonzalez et al., 2023; Liu et al., 2024) and replication (Dai et al., 2013; Jin et al., 2018) cycles, including for SARS-CoV-2 (Klein et al., 2020). Of note, directly visualizing multiple types of complexes at molecular resolution has not been demonstrated even with the most advanced room-temperature VEM techniques; therefore, whether preceded by cryoVEM or not, cryoET has an unparalleled capability to reveal the intricacies of cellular ultrastructure at the highest resolutions in near-native states.
Automation of tilt series acquisition (Mastronarde, 2005) and ensuing tomographic reconstruction (Noble and Stagg, 2015; Mastronarde and Held, 2017; Zheng et al., 2022; Chaillet et al., 2023; Khavnekar et al., 2023) has helped cryoET become a routine technique for many specimens. Downstream subtomogram averaging (STA) (Galaz-Montoya and Ludtke, 2017; Förster, 2022) can yield structures at subnanometer (Schur et al., 2013) and near-atomic (Schur et al., 2016) resolutions, even for complexes within cells (Tegunov et al., 2021). While STA was first demonstrated nearly 30 years ago (Walz et al., 1997) using ad hoc scripts (Nicastro et al., 2006), the first semi-automated pipelines for STA started emerging only about a decade ago (Castaño-Díez et al., 2012; Hrabe et al., 2012; Galaz-Montoya et al., 2015), and have become increasingly sophisticated and efficient (Bharat and Scheres, 2016; Galaz-Montoya et al., 2016; Castaño-Díez et al., 2017; Himes and Zhang, 2018; Fernandez and Li, 2021; Zivanov et al., 2022; Burt et al., 2024), with the field rapidly taking large strides towards full automation for some specimens (Chen et al., 2019; Ni et al., 2022; Balyschew et al., 2023). Clearly, these advancements have positioned STA at the forefront of high-resolution structural biology in situ.
In the context of potential histopathological examination by cryoEM/ET, while plunge-freezing suffices to vitrify biochemically isolated macromolecular complexes and small cells bacterial cells and platelets (Dobro et al., 2010) and is also adequate to visualize the thin periphery of larger eukaryotic cells (Martins et al., 2021), or thin neuronal processes (Shahmoradian et al., 2014), high-pressure freezing (HPF) is required to properly vitrify multicellular organisms and tissues up to ∼200 µm in thickness (Moor, 1987; Dahl and Staehelin, 1989; Studer et al., 2001). Various strategies have been developed to thin down cryopreserved, thick biological specimens to render them amenable to cryoEM/ET imaging. For example, cryo-sectioning (Watkins, 2001; Griffith et al., 2008) uses an ultramicrotome to generate thin sections (∼50–500 nm thick) from vitrified samples; however, it’s an extremely challenging and slow-throughput technique that suffers from myriad artifacts that distort the frozen samples (Al-Amoudi et al., 2005). On the other hand, cryogenic FIB-SEM (cryoFIB-SEM) can mill thick specimens into lamellae more efficiently and presumably with fewer artifacts than cryosectioning (Marko et al., 2006). These lamellae can be rendered optimally thin (∼60–350 nm in thickness) for subsequent cryoET (Villa et al., 2013), though thicker lamellae (∼400–500 + nm) have also been analyzed to preserve more of the 3D cellular context near targets of interest (Wu et al., 2020). Of note, challenging specimens that exhibit relatively thick peripheries (∼400–600 + nm thickness) and are not easy candidates for cryoFIB-SEM milling have also been characterized by cryoET (Dudek et al., 2023). Irrespective of what method is used to thin out tissue samples, montage tomography (Peck et al., 2022) will likely play a central role in generating reconstructions at the highest possible resolution over large continuous areas.
1.4 Correlative light and electron microscopy for targeted, high-resolution structural histopathology
Among the most prolific techniques in structural histopathology, IHC and ISH combining EM and LM/FM are inherently correlative owing to their use of fluorescent probes to localize specific molecules in tissues. All high-resolution EM techniques commonly used in molecular biosciences to examine targets in cells described in prior sections can also be combined with LM/FM through correlative light and electron microscopy (CLEM) methods, including VEM techniques (Bushby et al., 2012). For example, a recent study applied correlative light and VEM to visualize morphological changes in the developing brain (Hayashi et al., 2023). While these methods may be underrepresented in the clinic owing to technical limitations and cost, they are primed to revolutionize the field of structural pathology.
CryoCLEM approaches (Lucić et al., 2007; Sartori et al., 2007; Plitzko et al., 2009; Hampton et al., 2017), including those involving cryoVEM methods (Vidavsky et al., 2016) such as cryoSBF-SEM (Hoffman et al., 2020) and cryoFIB-SEM (Gorelick et al., 2019), are not yet routine but constitute an actively developing field given their capacity to highlight molecular identities during ultrastructural examination of cells and tissues without chemical fixation and staining artifacts (Bharat and Kukulski, 2019). Increasing efforts to automate these techniques will help to make them routine for many specimens in the near future (Klumpe et al., 2021; Yang et al., 2021; Weiner et al., 2022), including bacteria and other pathogens (Liedtke et al., 2022), particularly as ML strategies are incorporated into many steps of the workflows (Seifert et al., 2020). Recent implementations have demonstrated cryoCLEM of entire cells using cryoSBF-SEM followed by cryoFIB-SEM lamellae milling and cryoET of targeted areas of interest (Wu et al., 2020), as well as for tissues using the ‘lift-out’ technique (Schaffer et al., 2019; Kuba et al., 2021) and entire organisms using ‘serial lift-out’ (Schiøtz et al., 2023). Of particular relevance to histopathology evaluations at high resolution, correlative strategies have been demonstrated even for complex montage cryoET (Yang et al., 2023), which powerfully allows researchers to stitch multiple tomograms together, thereby delivering 3D views over large, continuous areas of the imaged specimen. Exciting developments that combine cryogenic super-resolution LM and FM with cryoEM methods (Chang et al., 2014; Wolff et al., 2016; Dahlberg and Moerner, 2021), for which novel supports (Last et al., 2023) are being proposed to reduce problems with specimen heating (Dahlberg et al., 2022), will usher a new era of correlative studies with unprecedented specificity and resolution. Given cryoCLEM’s unique ability to uncover rare events in cells and tissues (Kukulski et al., 2011; Ader and Kukulski, 2017), several of the techniques falling under this umbrella term will predictably find applications in the advancement of personalized medicine through high-resolution structural cellular pathology and histopathology.
2 Challenges and limitations in traditional and cryogenic microscopy techniques for structural studies of macromolecules, cells, and tissues
Despite being powerful techniques to examine cells and organelles, as well as the broad localization of macromolecules within them, the resolution of standard LM/FM setups is diffraction limited (Masters, 2020), providing spatial insights at up to ∼170–250 nm resolution laterally and at ∼2–3x lower resolution axially (Valli et al., 2021), which does not allow to discern molecular details. This may preclude detection of finer changes in the structure and distribution of subcellular components, as well as of conformational changes in macromolecular complexes, which may be reflective of early-stage or mild disease phenotypes. Furthermore, in histopathology applications, the use of fluorescent probes typically requires their conjugation with antibodies as well as cell fixation and permeabilization, which, depending on the system under investigation, can induce artifacts that alter the distribution and structure of subcellular components (Yoshida et al., 2023). In general, tagging endogenous molecules with fluorescent probes or overexpressing fusion constructs can be toxic to cells and/or change the localization, distribution, structure, and function of the labeled targets (Jensen, 2012). While tissue fixation with aldehydes such as formaldehyde or glutaraldehyde is a critical step in sample preparation in traditional EM to stabilize cellular structures and prevent their degradation, it can also add to a gamut of histopathological artifacts due to chemical cross-linking and tissue shrinkage (Taqi et al., 2018). Moreover, different fixatives and other specimen preparation steps like embedding, sectioning, and heavy metal staining may differentially preserve, alter, or obscure cellular components, leading to selective or anisotropic distortions that may preclude visualizing the native morphology and composition of tissues. In addition to these caveats during specimen preparation, the next frontier in microscopy of fixed specimens, namely, VEM techniques such as SBF-SEM and FIB-SEM, remain niche, slow-throughput, and costly (Guérin et al., 2019); however, increasing automation and the incorporation of AI/ML-based technologies are rapidly making them more efficient (Peddie et al., 2022; Collinson et al., 2023).
In the cryogenic domain, while SPA can yield functional insights at extremely high resolution and has found applications in drug discovery, development, and design (Subramaniam et al., 2016; Renaud et al., 2018), this technique only works in vitro, typically requiring harsh biochemical purification of the complexes of interest. Interestingly, SPA has recently been demonstrated for complexes in cell lysates, which is arguably closer to their native context than visualizing them in highly purified solutions (Yi et al., 2019). Nonetheless, by virtue of imaging thin layers of frozen specimens in solution or lysates, macromolecules are exposed to denaturing forces at the air-water interface during SPA experiments (Glaeser and Han, 2017). Because of these and other issues, SPA might prove less applicable to histopathological diagnostics in the future than cryoVEM methodologies, including cryoSBF-SEM and cryoFIB-SEM, with or without subsequent cryoET and STA.
Although methodologies and protocols are continuously improving, the technical difficulty and gross artifacts of cryo-sectioning have precluded it from becoming more widely applicable and routine even in basic science laboratories (Titze and Genoud, 2016). On the other hand, a big limitation of cryoFIB-SEM milling in producing lamella for imaging at high resolution with cryoET is that this hybrid method currently only allows for imaging relatively small, localized regions within cells and tissues, with most of the frozen material being burned off by the milling process. Furthermore, while cryoFIB-SEM artifacts are commonly regarded as relatively mild compared to overt cryo-sectioning crevasses, damage from the ion beam is not constrained to the surface of lamellae. On the contrary, ion beam damage to the specimen has been demonstrated to propagate through tens of nanometers into milled lamellae (Giannuzzi and Stevie, 1999; Volkert and Minor, 2007), from both surfaces, with the extent of damage depending on the sample, the nature of the ion beam (e.g., using gallium vs. argon ions) (Berger et al., 2023; Lucas and Grigorieff, 2023), and the accelerating voltage used to mill (Mayer et al., 2007).
Localizing regions of interest to mill lamellae has proven to be another significant challenge, particularly for multicellular and tissue specimens (Navarro, 2022). While correlative techniques can aid in such a task, particularly developments incorporating super-resolution techniques, the limited number of fluorescent probes amenable to imaging at cryogenic temperatures limits the wider applicability of these techniques (Dahlberg et al., 2018). In addition, the risks of contamination and devitrification are high due to multiple specimen transfers between microscopes and other pieces of equipment for most setups. Even when the targets of interest can be localized within frozen cells or tissues on cryoEM grids, the difficulty in adding high-contrast fiducial markers after cryoFIB-SEM lamella milling complicates the downstream task of cryoET reconstruction, depending on lamella thickness and the contrast of features in the sample (Harapin et al., 2015). Furthermore, cryoEM support grids and electron microscope specimen stages used in the life sciences are not designed to allow for full-range tilting. Even if full-range tilting were possible, the slab-geometry of frozen-hydrated specimens and milled lamellae increases the mean free path of electrons through the specimen as the tilt angle increases, yielding high-tilt images of limited value, and eventually occluding the electron beam completely at high tilt angles (Galaz-Montoya and Ludtke, 2017). Indeed, historically one of the greatest limitations in cryoET stems from the tilt range for productive data collection being limited to ± 60° for most specimens, which gives rise to the so-called “missing wedge” and its associated artifacts, such as anisotropic resolution in the reconstructed tomograms (Radermacher, 1988). These artifacts negatively affect downstream processes such as feature identification, segmentation, and quantitative analyses.
Although not a direct experimental limitation, the raw frame images and downstream files generated during data collection and processing by cryoEM/ET comprise many terabytes of data for each specimen examined. This is a consequence of modern direct electron detectors (Milazzo et al., 2005) enabling the acquisition of multiple images or “frames” of the same specimen area at a rapid rate, with low electron doses, which can then be aligned to derive averaged images with much higher contrast and signal to noise ratio (Veesler et al., 2013). Furthermore, these detectors are becoming faster and larger (McMullan et al., 2016), guaranteeing a more rapid rate of dataset growth for cryoEM and related techniques which, on top of everything, are becoming increasingly popular (Callaway, 2020). By now, it is widely recognized that cryoEM and cryoET are “big data” methods (Baldwin et al., 2018) that pose myriad associated challenges in data storage, transfer, processing, and management in general, requiring advanced and costly computational resources (Poger et al., 2023). A related challenge in the field is that many academic software applications are developed for ad hoc purposes by non-professional programmers and thus can often be suboptimally designed as well as poorly distributed, documented, and maintained, particularly in fields that are not computationally focused. While many impressive tools have been developed by the academic community for analysis of datasets generated by cryoEM/ET and related techniques, their practical use and wide adoption are often not without challenges due to limited dissemination, scant quality metrics and benchmarking studies, particularly for cryoET tomograms in the absence of STA, lacking standardization of data formats, as well as complexities in software installation, usage, maintenance, compatibility with heterogeneous hardware systems, and interoperability across operating systems and complementary software upstream or downstream in the data processing pipeline.
3 Current AI applications in cryoEM as a routine technique and cryoET at the frontier of high-resolution cellular biology
Recent ML and AI applications have been increasingly contributing to the rapid rise of cryoEM since the turn of the millennium but most prominently over the last 6 years (Figure 2), enabling efficient, unbiased workflows that can reconstruct SPA structures from cell extracts without knowing the identities or relative abundance of their biochemical components a priori (Skalidis et al., 2022). These types of feats have been possible and will become increasingly common thanks to AI-assisted acceleration and improvement in various workflow steps. These include specimen screening before data collection (Bouvette et al., 2022; Cheng et al., 2023), micrograph denoising (Tegunov and Cramer, 2019; Bepler et al., 2020), structure reconstruction (Giri et al., 2023), and postprocessing (Sanchez-Garcia et al., 2021), with recent efforts having been particularly concentrated on particle picking (Wang et al., 2016; Zhu et al., 2017; Sanchez-Garcia et al., 2018; George et al., 2021) and model building (He et al., 2022; DiIorio and Kulczyk, 2023; Giri et al., 2023). Machine learning approaches have sped up cryoEM SPA structure determination to the point that, for many specimens, including those exhibiting compositional and conformational heterogeneity (Zhong et al., 2021), multiple structures at near-atomic resolution can be derived in a few days, sometimes even from a single imaging session and processing the corresponding data using a single workstation equipped with GPU acceleration (Kimanius et al., 2016). Of note, the methodological similarities between cryoEM SPA and cryoET STA facilitate a mutually beneficial transfer of technologies across these methodologies.
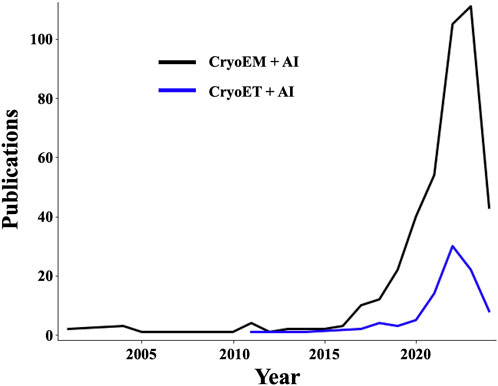
Figure 2. The number of publications showcasing the use of AI in cryoEM/ET applications is undergoing exponential growth. Plots showing a growing trend in the number of publications in Elsevier’s Scopus database containing (“cryoem” OR “cryo-em” OR “cryogenic electron microscopy” OR “cryo electron microscopy”) terms (black curve), or (“cryogenic electron tomography” OR “Subtomogram averaging” OR “cryo-et” OR “cryoet” OR “cryo electron tomography”) terms (blue curve), in conjunction with (“deep learning” OR “artificial intelligence” OR “machine learning” OR “neural networks”) terms.
For cryoET, the first AI applications emerged about a decade ago but have only taken off over the last 4 years (Figure 2) coming mostly from a few research groups (Zeng et al., 2021b). The main foci of attention have been the detection (Moebel et al., 2021; Lamm et al., 2022), classification (Moebel and Kervrann, 2022), and alignment (Zeng et al., 2021a) of macromolecular complexes in tomograms as well as feature annotation (Chen et al., 2017; Genthe et al., 2023). Automated and semi-automated solutions to the related problems posed by particle picking and feature annotation are of particular significance since thoroughly accomplishing these tasks manually for large cryoET datasets is outright impossible at realistic timescales, even with efficiency-driven strategies (Danita et al., 2022), and the process is subject to high levels of inconsistency and bias (Hecksel et al., 2016). Some of the most exciting AI-powered developments in cryoET are forefront methods that use CNNs to restore the missing wedge (Liu et al., 2022; Zhang et al., 2023). Another impressive application is the “deep iterative subtomogram clustering approach” (DISCA), a fully automated, label-free, and template-free pattern mining algorithm that uses CNNs to extract and cluster rotationally and translationally invariant features as subtomogram classes (Zeng et al., 2023), which can then be aligned and averaged as a post-preprocessing step.
4 Discussion: opportunities to further potentiate large-scale cryoET analyses of cells and tissues with AI towards enabling preventive, high-resolution structural histopathology diagnosis
From ancient observations to modern innovations, the trajectory of pathology reflects humanity’s enduring quest to unravel the mysteries of disease. Anatomical, cellular, molecular, and digital pathology represent complementary chapters in this ever-evolving quest, which is well poised to incorporate insights from the leading edge of high-resolution structural biology, namely, cryoEM/ET and related methodologies (Supplementary Figure S1). The synergistic integration of the flourishing vanguard technologies reviewed here will help propel humanity toward a future of improved diagnostics and targeted therapies at the dawn of personalized medicine.
Even though cryoEM SPA is regarded as less likely to directly apply to histopathological diagnostics any time soon compared to cryoET, cryoSBF-SEM, and cryoFIB-SEM, because it requires harsh biochemical purification or cell lysis, it’s conceivable that future technological breakthroughs may allow to cost-effectively determine structures of macromolecules purified from patient tissues to derive clinically relevant information that could complement clinical proteomics (Petricoin et al., 2004; Mani et al., 2022) in the delivery of personalized medicine. Indeed, several structures of polymorphic amyloidogenic tau filaments have been resolved to high resolution by cryoEM SPA, including from samples purified from patient tissues (Scheres et al., 2020). Interestingly, a recent and exciting study that reported a newly discovered amyloidogenic motif, Polymorphic Amyloid Motif of Repeat 4 (PAM4), replicated several of these structures in vitro by cryoEM SPA of a synthetic PAM4 peptide (Louros et al., 2024). However, in spite of the challenges comprehensively reviewed here, cryoET and STA downstream of cryoVEM and cryoFIB-SEM lamellae milling are likely to play a more central role in achieving the promise of high-resolution structural histopathology diagnostics directly from patient tissues and cells or patient-derived models in a near-native state and context, such as organoids and iPSCs.
While reproducible specimen preparation and grid screening have become highly efficient and routine for many cryoEM SPA targets, these steps remain a more significant initial bottleneck in cryoET, particularly for multicellular organisms and tissues. Once specimens have been prepared, automatically identifying good areas for tilt series collection is one of the challenges to overcome in the near future that will resolve a significant bottleneck, as recently accomplished for single particle cryoEM with the help of AI algorithms (Yokoyama et al., 2020). After specimen preparation and grid screening, successful automated acquisition of tilt series is comparatively fast and routine, and the possibility of boosting this step has been recently demonstrated by emerging methods such as continuous and fast incremental tomography (Chreifi et al., 2019). Approaches leveraging AI/ML could conceivably help to automatically “prune” low-quality images during or right after data collection by learning to identify and exclude images with anomalous defocus, large stage drift, poor contrast, radiation damage, excessive contamination, reflections from non-vitreous “bad ice”, and/or specimen charging causing uncorrectable image blurriness. This would reduce the amount of parasitic data downstream, precluding corresponding storage and processing overhead burdens. Additionally, strategies that sample milled lamellae more efficiently—like parallel (Eisenstein et al., 2023) and montage (Peck et al., 2022) tomography—are already improving data collection for cryoFIB-SEM milled samples. Of note, the latter allows visualizing large continuous areas of the specimen by stitching together multiple sequential reconstructions from adjacent areas, constituting an important stepping stone towards building high-resolution molecular atlases over large regions in cells and tissues. Importantly, montage cryoET has recently been demonstrated in conjunction with correlative techniques (Yang et al., 2023). A relatively neglected albeit useful strategy is the addition of gold fiducial markers to lamellae after cryoFIB-SEM milling (Harapin et al., 2015). While many software packages can now successfully align tilt series of thin specimens without fiducials, quality is often compromised as most specimens yield low-contrast images, particularly ones near the thickness limits for cryoET (∼500–600 nm), precluding optimal tilt series alignment and tomographic reconstruction. Continued development of phase plates (Danev and Baumeister, 2017), and AI-driven denoising methods (Palovcak et al., 2020) to enhance image contrast will predictably increase the number of datasets that can be optimally reconstructed in the absence of gold fiducial markers.
CryoEM/ET and related imaging modalities have been recognized as “big data” methods that meet the definition criteria of rapidly producing large amounts of varied data (Baldwin et al., 2018; Poger et al., 2023). As such, data management, processing, and analysis have become larger bottlenecks than data collection. While the quest for efficient data representations in cryoEM/ET is not new, and has been addressed in a few studies (Fluty and Ludtke, 2022), increasing the efficiency of storage and transfer of high-dimensionality data is still an area of substantial opportunity. This challenge could benefit from the development of unsupervised ML techniques that integrate statistical methods like Principal Component Analysis and autoencoders to achieve effective data compression without significant loss of information. Furthermore, simulations and supervised machine learning approaches training on existing data could be leveraged to create predictive models that facilitate experimental parameter optimization, thereby saving time and computational resources. Recent algorithms have demonstrated rapid, automated, even on-the-fly tomographic reconstruction (Zheng et al., 2022), including accurate and detailed determination of the contrast transfer function and astigmatism for tilted specimens (Mastronarde, 2024), as well as missing wedge restoration by CTF deconvolution (Croxford et al., 2021) and other methods. Indeed, multiple increasingly automated pipelines have emerged to expedite cryoET workflows (Morado et al., 2016; Böhning and Bharat, 2021), including subtomogram averaging, tomographic annotation, and software interoperability (Jiménez de la Morena et al., 2022). Despite such exciting and fast-paced progress, the use of ML to automate tasks in cryoET and related techniques is in its infancy, begging for increased and closer collaboration between biological scientists and microscopists with computer scientists, as well as between academia and the healthcare, medical, pharmaceutical, and biotech industries (Garousi et al., 2016). Indeed, given how computationally and data-intensive cryoEM/ET analyses are, there is a great need for efficient, cross-platform, easy-to-install, well-maintained, well-documented, and user-friendly software for data processing and analysis. Moreover, the availability of AI-based tools for coding and software development (Wu T. et al., 2023; Moradi Dakhel et al., 2023) could help in the attainment of such a goal. Moving into the future, increased file format standardization, improved software documentation and maintenance, and adoption of best practices guided by extensive benchmarking (Turoňová et al., 2020) will contribute to reducing post-data collection bottlenecks.
In terms of alleviating missing wedge distortions, redesigned specimen supports, as well as holders and stages for electron microscopes to enable in-plane rotation of grids and/or full-range tilting as in might prove to be particularly rewarding. A proof of concept for this, namely, the development and usage of carbon nanopipettes as cylindrical specimen supports, was published a decade ago (Palmer and Löwe, 2014) but has failed to gain traction. Furthermore, the unique potential of using three orthogonal projections to minimize mathematical ambiguity in the orientation and conformation of single particles was qualitatively illustrated also a decade ago (Galaz-Montoya, 2014), a concept that could be extended to collecting tri-orthogonal images of cells and tissues, thereby experimentally precluding the missing wedge problem altogether. Alternatively, emerging AI algorithms in computer vision such as vision transformers (Khan et al., 2022), foundation models (Ma and Wang, 2023), and coordinate networks (Tancik et al., 2020), which outperform CNNs in many tasks, might help to produce the next-generation of AI-powered solutions for cryoEM/ET and related technologies, particularly in improving missing wedge restoration as well as feature segmentation, classification, and downstream quantitative analyses. Coordinate networks have indeed recently been demonstrated to outperform the leading CNNs implementation for missing wedge restoration (Liu et al., 2022) in terms of shape fidelity and reconstruction efficiency (Van Veen et al., 2024), albeit this was only through in silico experiments and replication of these results on cryoET data is pending.
The rise of cryoCLEM methods has alleviated the issue of specimen localization in cryoFIB-SEM milled specimens to some extent but these techniques still suffer from poor axial resolution. On the other hand, the development of super-resolution light microscopy techniques such as 4pi microscopy, stochastic optical reconstruction microscopy (STORM), stimulated emission depletion (STED) microscopy, and photoactivated localization microscopy (PALM) have bridged the gap between LM/FM and EM (Henriques et al., 2011), allowing microscopists to visualize biological systems at resolutions between ∼20 and 100 nm. Furthermore, these techniques can be correlated with EM to gain increased molecular details (Timmermans and Otto, 2015). For example, a recent seminal study used a combination of STED, SBF-SEM, TEM, and STA with biophysical techniques to discover unique structural features never described before in Lewy pathology, directly in Parkinson disease patient post-mortem brain tissues (Shahmoradian et al., 2019). Other promising studies have recently demonstrated the use of cryoCLEM and cryoET to examine the structures of amyloid plaques in situ directly in cryogenic ultramicrotomy slices from rodent brains (Leistner et al., 2023), as well as the use of xenon plasma-based cryoFIB milling followed by cryoET to examine cellular structures in post-mortem human brain tissues (Creekmore et al., 2024). An exciting preprint showcasing a tour-de-force combining extensive cryoCLEM, cryosectioning, cryoFIB-SEM lift-out, and cryoET examined the structures of fibrillar amyloid-β and tau inclusions directly in post-mortem brain tissues from Alzheimer disease patients and control donors (Gilbert et al., 2023).
On the specimen front, patient-derived organoids are emerging as extremely useful model systems (Ooft et al., 2019) that may guide the development of improved, targeted treatments (Chen et al., 2021). These and patient-derived induced pluripotent stem cells (iPSCs) (Malik and Rao, 2013) represent huge steps toward realizing the dream of minimally invasive diagnostics and will play a prominent role in structural histopathology given the demonstrated feasibility of imaging them with cryoVEM techniques and cryoET. For example, a recent cryoET study examining neurons differentiated from Huntington disease (HD) patient-derived iPSCs identified unique structural phenotypes in neurite organelles, likely corresponding to early disease stages, and validated their rescue upon knockdown of a candidate therapeutic target (Wu G.-H. et al., 2023). Further studies of these systems leveraging cryoFIB-SEM lamella milling could help confirm whether the observed phenotypes are also reflected in altered structures through the much thicker neuronal cell body or even in post-mortem, patient-derived brain tissues. Along those lines, automated fiducialless alignment strategies have remedied the problem of lacking gold fiducials in tilt series from cryoFIB-SEM lamellae for favorable cases in which the specimen has been thinned out enough and high-contrast features are present, well distributed, and trackable within the field of view throughout the tilt series, including at high-tilt angles. Ongoing denoising and alignment strategies powered by AI may facilitate the accurate reconstruction of tomograms from tilt series of lamellae on the thicker end of the spectrum lacking fiducials.
The emergence of super-resolution cryoCLEM methods offers the promise to yield a plethora of exciting observations from samples in fully hydrated, near-native conditions. There’s great diagnostic potential in such types of findings if efficient protocols and pipelines can be developed to reduce turnaround time and cost. The marked increase in interest in cryoEM/ET, cryoVEM, and related techniques, as well as their rapid democratization (Stuart et al., 2016; Serbynovskyi et al., 2024), as evidenced by the opening of national service centers for macromolecular structure determination (Eng et al., 2019), training (Eng et al., 2023), and cryoET specimen preparation (Larson et al., 2022) in the USA constitute foreshadowings of the promising potential of these technologies to advance biomedicine, particularly drug design and the nascent field of high-resolution, near-native-state cellular structural pathology and histopathology championed in this review.
5 Conclusion
Although cryoEM/ET and super-resolution light microscopy techniques typically suffer from lower throughput compared to conventional microscopy methods, as the medical field embraces what Peter Attia calls the “Medicine 3.0” paradigm (Attia, 2023), turnover time may become less critical to many clinical diagnoses given the preventive nature of this model that will usher humanity away from “sick-care” toward genuine “healthcare”. Medicine 3.0 focuses on proactive, early interventions, thereby reducing the urgency of speedy diagnostic assays, in contrast to the demands of currently prevalent sick-care systems (Friebe, 2022) that are primarily reactive to acute pathologies at advanced stages. Increased and improved diagnostic capabilities will enable healthcare to transition from disease detection based on gross distortions in the biochemical signatures and the structural architectures of organs, tissues, and cells, to disease prevention based on high-resolution visualization of early abnormal alterations. This review is somewhat of a cryogenic analog to a recent call to increasingly use 3D EM techniques to quantitatively examine organelles as a proxy for disease monitoring (Neikirk et al., 2023). The application of iteratively improved ML models to mine growing, multi-scale, “big data” cryoVEM and cryoET datasets of patient tissues and cells should enable increasingly advanced and accurate image restoration, pattern identification, and feature classification at nanometric resolution, thereby affording greater statistical confidence to the interpretation of subcellular and macromolecular structures corresponding to early disease phenotypes, essential to realizing the possibility of personalized, preventive structural pathology.
Author contributions
JGGM: Conceptualization, Investigation, Writing–original draft, Writing–review and editing.
Funding
The author(s) declare that no financial support was received for the research, authorship, and/or publication of this article.
Conflict of interest
The author declares that the research was conducted in the absence of any commercial or financial relationships that could be construed as a potential conflict of interest.
Publisher’s note
All claims expressed in this article are solely those of the authors and do not necessarily represent those of their affiliated organizations, or those of the publisher, the editors and the reviewers. Any product that may be evaluated in this article, or claim that may be made by its manufacturer, is not guaranteed or endorsed by the publisher.
Supplementary material
The Supplementary Material for this article can be found online at: https://www.frontiersin.org/articles/10.3389/fmolb.2024.1390858/full#supplementary-material
SUPPLEMENTARY FIGURE S1 | Schematic diagram showing a high-level overview of alternative coarse-grained workflows for imaging biological samples in microscopy studies involving cryogenic electron tomography (cryoET), focused ion beam (FIB) scanning electron microscopy (SEM), and/or correlative light and electron microscopy (CLEM). Three prominent fixation methods are outlined in purple boxes near the top, with colored lines representing the shortest experimental pathways for the four specific techniques highlighted in this review. These efficient paths are mostly applicable to thin specimens such as small unicellular organisms or the periphery of single cells while the dashed lines indicate more complex, alternative paths that can be followed depending on specific experimental requirements and objectives, typically suitable for thicker cells, multicellular organisms, and tissues. All paths lead down to orange boxes that highlight the reconstruction outcomes for final visualization of the imaged specimens (namely a tomographic reconstruction or a volume made of adjacent FIB-SEM slices). Note: The diagram and its connections may not be exhaustive and each pathway could be broken down into many more fine-grained experimental steps that are out of scope for this review.
References
Ader, N. R., and Kukulski, W. (2017). triCLEM: combining high-precision, room temperature CLEM with cryo-fluorescence microscopy to identify very rare events. Methods Cell Biol. 140, 303–320. doi:10.1016/bs.mcb.2017.03.009
Al-Amoudi, A., Studer, D., and Dubochet, J. (2005). Cutting artefacts and cutting process in vitreous sections for cryo-electron microscopy. J. Struct. Biol. 150, 109–121. doi:10.1016/j.jsb.2005.01.003
Attia, P. (2023). Outlive: the science and art of longevity harmony/rodale. Available at: https://play.google.com/store/books/details?id=CspvEAAAQBAJ.
Baldwin, P. R., Tan, Y. Z., Eng, E. T., Rice, W. J., Noble, A. J., Negro, C. J., et al. (2018). Big data in cryoEM: automated collection, processing and accessibility of EM data. Curr. Opin. Microbiol. 43, 1–8. doi:10.1016/j.mib.2017.10.005
Balyschew, N., Yushkevich, A., Mikirtumov, V., Sanchez, R. M., Sprink, T., and Kudryashev, M. (2023). Streamlined structure determination by cryo-electron tomography and subtomogram averaging using TomoBEAR. Nat. Commun. 14, 6543. doi:10.1038/s41467-023-42085-w
Banerji, S., and Mitra, S. (2022). Deep learning in histopathology: a review. Wiley Interdiscip. Rev. Data Min. Knowl. Discov. 12. doi:10.1002/widm.1439
Bäuerlein, F. J. B., Saha, I., Mishra, A., Kalemanov, M., Martínez-Sánchez, A., Klein, R., et al. (2017). In situ architecture and cellular interactions of PolyQ inclusions. Cell 171, 179–187. e10. doi:10.1016/j.cell.2017.08.009
Bendory, T., Bartesaghi, A., and Singer, A. (2020). Single-particle cryo-electron microscopy: mathematical theory, computational challenges, and opportunities. IEEE Signal Process. Mag. 37, 58–76. doi:10.1109/msp.2019.2957822
Bepler, T., Kelley, K., Noble, A. J., and Berger, B. (2020). Topaz-Denoise: general deep denoising models for cryoEM and cryoET. Nat. Commun. 11, 5208. doi:10.1038/s41467-020-18952-1
Berger, C., Dumoux, M., Glen, T., Yee, N. B.-Y., Mitchels, J. M., Patáková, Z., et al. (2023). Plasma FIB milling for the determination of structures in situ. Nat. Commun. 14, 629. doi:10.1038/s41467-023-36372-9
Bharat, T. A. M., and Kukulski, W. (2019). Cryo-correlative light and electron microscopy. Correl. Imaging, 137–153. doi:10.1002/9781119086420.ch8
Bharat, T. A. M., and Scheres, S. H. W. (2016). Resolving macromolecular structures from electron cryo-tomography data using subtomogram averaging in RELION. Nat. Protoc. 11, 2054–2065. doi:10.1038/nprot.2016.124
Böhning, J., and Bharat, T. A. M. (2021). Towards high-throughput in situ structural biology using electron cryotomography. Prog. Biophys. Mol. Biol. 160, 97–103. doi:10.1016/j.pbiomolbio.2020.05.010
Bouvette, J., Huang, Q., Riccio, A. A., Copeland, W. C., Bartesaghi, A., and Borgnia, M. J. (2022). Automated systematic evaluation of cryo-EM specimens with SmartScope. Elife 11, e80047. doi:10.7554/eLife.80047
Burt, A., Toader, B., Warshamanage, R., von Kügelgen, A., Pyle, E., Zivanov, J., et al. (2024). An image processing pipeline for electron cryo-tomography in RELION-5. Available at: https://www.biorxiv.org/content/10.1101/2024.04.26.591129v1.
Bushby, A. J., Mariggi, G., Armer, H. E. J., and Collinson, L. M. (2012). “Chapter 18 - correlative light and volume electron microscopy: using focused ion beam scanning electron microscopy to image transient events in model organisms,” in Methods in cell biology, Editors T. Müller-Reichert, and P. Verkade (Academic Press), 357–382. doi:10.1016/B978-0-12-416026-2.00018-2
Callaway, E. (2020). Revolutionary cryo-EM is taking over structural biology. Nature 578, 201. doi:10.1038/d41586-020-00341-9
Castaño-Díez, D., Kudryashev, M., Arheit, M., and Stahlberg, H. (2012). Dynamo: a flexible, user-friendly development tool for subtomogram averaging of cryo-EM data in high-performance computing environments. J. Struct. Biol. 178, 139–151. doi:10.1016/j.jsb.2011.12.017
Castaño-Díez, D., Kudryashev, M., and Stahlberg, H. (2017). Dynamo Catalogue: geometrical tools and data management for particle picking in subtomogram averaging of cryo-electron tomograms. J. Struct. Biol. 197, 135–144. doi:10.1016/j.jsb.2016.06.005
Chaillet, M. L., van der Schot, G., Gubins, I., Roet, S., Veltkamp, R. C., and Förster, F. (2023). Extensive angular sampling enables the sensitive localization of macromolecules in electron tomograms. Int. J. Mol. Sci. 24, 13375. doi:10.3390/ijms241713375
Chang, Y.-W., Chen, S., Tocheva, E. I., Treuner-Lange, A., Löbach, S., Søgaard-Andersen, L., et al. (2014). Correlated cryogenic photoactivated localization microscopy and cryo-electron tomography. Nat. Methods 11, 737–739. doi:10.1038/nmeth.2961
Chen, M., Bell, J. M., Shi, X., Sun, S. Y., Wang, Z., and Ludtke, S. J. (2019). A complete data processing workflow for cryo-ET and subtomogram averaging. Nat. Methods 16, 1161–1168. doi:10.1038/s41592-019-0591-8
Chen, M., Dai, W., Sun, S. Y., Jonasch, D., He, C. Y., Schmid, M. F., et al. (2017). Convolutional neural networks for automated annotation of cellular cryo-electron tomograms. Nat. Methods 14, 983–985. doi:10.1038/nmeth.4405
Chen, P., Zhang, X., Ding, R., Yang, L., Lyu, X., Zeng, J., et al. (2021). Patient-derived organoids can guide personalized-therapies for patients with advanced breast cancer. Adv. Sci. 8, e2101176. doi:10.1002/advs.202101176
Chen, X., Zheng, B., and Liu, H. (2011). Optical and digital microscopic imaging techniques and applications in pathology. Anal. Cell. Pathol. 34, 5–18. doi:10.3233/ACP-2011-0006
Cheng, A., Kim, P. T., Kuang, H., Mendez, J. H., Chua, E. Y. D., Maruthi, K., et al. (2023). Fully automated multi-grid cryoEM screening using Smart Leginon. IUCrJ 10, 77–89. doi:10.1107/S2052252522010624
Chreifi, G., Chen, S., Metskas, L. A., Kaplan, M., and Jensen, G. J. (2019). Rapid tilt-series acquisition for electron cryotomography. J. Struct. Biol. 205, 163–169. doi:10.1016/j.jsb.2018.12.008
Chua, E. Y. D., Mendez, J. H., Rapp, M., Ilca, S. L., Tan, Y. Z., Maruthi, K., et al. (2022). Better, faster, cheaper: recent advances in cryo–electron microscopy. Annu. Rev. Biochem. 91, 1–32. doi:10.1146/annurev-biochem-032620-110705
Collinson, L. M., Bosch, C., Bullen, A., Burden, J. J., Carzaniga, R., Cheng, C., et al. (2023). Volume EM: a quiet revolution takes shape. Nat. Methods 20, 777–782. doi:10.1038/s41592-023-01861-8
Creekmore, B. C., Kixmoeller, K., Black, B. E., Lee, E. B., and Chang, Y.-W. (2024). Ultrastructure of human brain tissue vitrified from autopsy revealed by cryo-ET with cryo-plasma FIB milling. Nat. Commun. 15, 2660. doi:10.1038/s41467-024-47066-1
Croxford, M., Elbaum, M., Arigovindan, M., Kam, Z., Agard, D., Villa, E., et al. (2021). Entropy-regularized deconvolution of cellular cryotransmission electron tomograms. Proc. Natl. Acad. Sci. U. S. A. 118, e2108738118. doi:10.1073/pnas.2108738118
Dahl, R., and Staehelin, L. A. (1989). High-pressure freezing for the preservation of biological structure: theory and practice. J. Electron Microsc. Tech. 13, 165–174. doi:10.1002/jemt.1060130305
Dahlberg, P. D., and Moerner, W. E. (2021). Cryogenic super-resolution fluorescence and electron microscopy correlated at the nanoscale. Annu. Rev. Phys. Chem. 72, 253–278. doi:10.1146/annurev-physchem-090319-051546
Dahlberg, P. D., Perez, D., Hecksel, C. W., Chiu, W., and Moerner, W. E. (2022). Metallic support films reduce optical heating in cryogenic correlative light and electron tomography. J. Struct. Biol. 214, 107901. doi:10.1016/j.jsb.2022.107901
Dahlberg, P. D., Sartor, A. M., Wang, J., Saurabh, S., Shapiro, L., and Moerner, W. E. (2018). Identification of PAmKate as a red photoactivatable fluorescent protein for cryogenic super-resolution imaging. J. Am. Chem. Soc. 140, 12310–12313. doi:10.1021/jacs.8b05960
Dai, W., Fu, C., Raytcheva, D., Flanagan, J., Khant, H. A., Liu, X., et al. (2013). Visualizing virus assembly intermediates inside marine cyanobacteria. Nature 502, 707–710. doi:10.1038/nature12604
Danev, R., and Baumeister, W. (2017). Expanding the boundaries of cryo-EM with phase plates. Curr. Opin. Struct. Biol. 46, 87–94. doi:10.1016/j.sbi.2017.06.006
Danita, C., Chiu, W., and Galaz-Montoya, J. G. (2022). Efficient manual annotation of cryogenic electron tomograms using IMOD. Star. Protoc. 3, 101658. doi:10.1016/j.xpro.2022.101658
Darrow, M. C., Moore, J. P., John Walker, R., Doering, K., and King, R. S. (2019). Chameleon: next generation sample preparation for CryoEM based on spotiton. Microsc. Microanal. 25, 994–995. doi:10.1017/S1431927619005701
Darrow, M. C., Sergeeva, O. A., Isas, J. M., Galaz-Montoya, J. G., King, J. A., Langen, R., et al. (2015). Structural mechanisms of mutant huntingtin aggregation suppression by the synthetic chaperonin-like CCT5 complex explained by cryoelectron tomography. J. Biol. Chem. 290, 17451–17461. doi:10.1074/jbc.M115.655373
de la Cruz, M. J., and Eng, E. T. (2023). Scaling up cryo-EM for biology and chemistry: the journey from niche technology to mainstream method. Structure 31, 1487–1498. doi:10.1016/j.str.2023.09.009
de Matos, J., Ataky, S. T. M., de Souza Britto, A., Soares de Oliveira, L. E., and Lameiras Koerich, A. (2021). Machine learning methods for histopathological image analysis: a review. Electronics 10, 562. doi:10.3390/electronics10050562
de Senneville, B. D., Khoubai, F. Z., Bevilacqua, M., Labedade, A., Flosseau, K., Chardot, C., et al. (2021). Deciphering tumour tissue organization by 3D electron microscopy and machine learning. Commun. Biol. 4, 1390. doi:10.1038/s42003-021-02919-z
DiIorio, M. C., and Kulczyk, A. W. (2023). Novel artificial intelligence-based approaches for ab initio structure determination and atomic model building for cryo-electron microscopy. Micromachines (Basel) 14, 1674. doi:10.3390/mi14091674
Dobro, M. J., Melanson, L. A., Jensen, G. J., and McDowall, A. W. (2010). “Chapter three - plunge freezing for electron cryomicroscopy,” in Methods in enzymology Editor G. J. Jensen (Academic Press), 63–82. doi:10.1016/S0076-6879(10)81003-1
Doerr, A. (2019). Graphene-on-gold grids for cryo-EM. Nat. Methods 16, 578. doi:10.1038/s41592-019-0488-6
Dorkenwald, S., Schubert, P. J., Killinger, M. F., Urban, G., Mikula, S., Svara, F., et al. (2017). Automated synaptic connectivity inference for volume electron microscopy. Nat. Methods 14, 435–442. doi:10.1038/nmeth.4206
Dubochet, J., Adrian, M., Chang, J. J., Homo, J. C., Lepault, J., McDowall, A. W., et al. (1988). Cryo-electron microscopy of vitrified specimens. Q. Rev. Biophys. 21, 129–228. doi:10.1017/s0033583500004297
Dubochet, J., Booy, F. P., Freeman, R., Jones, A. V., and Walter, C. A. (1981). LOW TEMPERATURE ELECTRON MICROSCOPY. Annu. Rev. Biophys. Bioeng. 10, 133–149. doi:10.1146/annurev.bb.10.060181.001025
Dudek, N. K., Galaz-Montoya, J. G., Shi, H., Mayer, M., Danita, C., Celis, A. I., et al. (2023). Previously uncharacterized rectangular bacterial structures in the dolphin mouth. Nat. Commun. 14, 2098. doi:10.1038/s41467-023-37638-y
Egerton, R. F., and Watanabe, M. (2022). Spatial resolution in transmission electron microscopy. Micron 160, 103304. doi:10.1016/j.micron.2022.103304
Eisenstein, F., Yanagisawa, H., Kashihara, H., Kikkawa, M., Tsukita, S., and Danev, R. (2023). Parallel cryo electron tomography on in situ lamellae. Nat. Methods 20, 131–138. doi:10.1038/s41592-022-01690-1
Eng, E. T., Rice, W. J., Cheng, A., Carragher, B., and Potter, C. S. (2019). What to expect from cryo-EM (national service centers). Acta Crystallogr. Sect. A Found. Adv. 75, a29. doi:10.1107/S0108767319099707
Eng, E. T., Zimanyi, C., Aragon, M., Chua, E. Y. D., Kopylov, E., Dubbeldam, C., et al. (2023). The national center for CryoEM access and training - establishing a cross-facility-honored training curriculum. Microsc. Microanal. 29, 1042–1043. doi:10.1093/micmic/ozad067.533
Fernandez, J.-J., and Li, S. (2021). TomoAlign: a novel approach to correcting sample motion and 3D CTF in CryoET. J. Struct. Biol. 213, 107778. doi:10.1016/j.jsb.2021.107778
Fluty, A. C., and Ludtke, S. J. (2022). Precision requirements and data compression in CryoEM/CryoET. J. Struct. Biol. 214, 107875. doi:10.1016/j.jsb.2022.107875
Förster, F. (2022). Subtomogram analysis: the sum of a tomogram’s particles reveals molecular structure in situ. J. Struct. Biol. X 6, 100063. doi:10.1016/j.yjsbx.2022.100063
Frank, J. (2006) Three-dimensional electron microscopy of macromolecular assemblies: visualization of biological molecules in their native state. Oxford University Press. Available at: https://play.google.com/store/books/details?id=kjGKy2LeWnUC.
Frank, J. (2008) Electron tomography: methods for three-dimensional visualization of structures in the cell. Springer Science and Business Media. Available at: https://play.google.com/store/books/details?id=LWx6JKQy34AC.
Fréchin, L., Holvec, S., von Loeffelholz, O., Hazemann, I., and Klaholz, B. P. (2023). High-resolution cryo-EM performance comparison of two latest-generation cryo electron microscopes on the human ribosome. J. Struct. Biol. 215, 107905. doi:10.1016/j.jsb.2022.107905
Friebe, M. (2022). “From SICKCARE to HEALTHCARE to HEALTH,” in Novel innovation design for the future of health: entrepreneurial concepts for patient empowerment and health democratization Editor M. Friebe (Cham: Springer International Publishing), 23–32. doi:10.1007/978-3-031-08191-0_3
Galaz-Montoya, J. G. (2014) Implementation of single particle cryo electron tomography in eman2 and its application to study the tric chaperonin’s interactions with mutant huntingtin aggregates. doi:10.13140/RG.2.2.36630.52803
Galaz-Montoya, J. G., Flanagan, J., Schmid, M. F., and Ludtke, S. J. (2015). Single particle tomography in EMAN2. J. Struct. Biol. 190, 279–290. doi:10.1016/j.jsb.2015.04.016
Galaz-Montoya, J. G., Hecksel, C. W., Baldwin, P. R., Wang, E., Weaver, S. C., Schmid, M. F., et al. (2016). Alignment algorithms and per-particle CTF correction for single particle cryo-electron tomography. J. Struct. Biol. 194, 383–394. doi:10.1016/j.jsb.2016.03.018
Galaz-Montoya, J. G., and Ludtke, S. J. (2017). The advent of structural biology in situ by single particle cryo-electron tomography. Biophys. Rep. 3, 17–35. doi:10.1007/s41048-017-0040-0
Galaz-Montoya, J. G., Shahmoradian, S. H., Shen, K., Frydman, J., and Chiu, W. (2021). Cryo-electron tomography provides topological insights into mutant huntingtin exon 1 and polyQ aggregates. Commun. Biol. 4, 849. doi:10.1038/s42003-021-02360-2
Garousi, V., Petersen, K., and Ozkan, B. (2016). Challenges and best practices in industry-academia collaborations in software engineering: a systematic literature review. Inf. Softw. Technol. 79, 106–127. doi:10.1016/j.infsof.2016.07.006
Genthe, E., Miletic, S., Tekkali, I., Hennell James, R., Marlovits, T. C., and Heuser, P. (2023). PickYOLO: fast deep learning particle detector for annotation of cryo electron tomograms. J. Struct. Biol. 215, 107990. doi:10.1016/j.jsb.2023.107990
George, B., Assaiya, A., Roy, R. J., Kembhavi, A., Chauhan, R., Paul, G., et al. (2021). CASSPER is a semantic segmentation-based particle picking algorithm for single-particle cryo-electron microscopy. Commun. Biol. 4, 200. doi:10.1038/s42003-021-01721-1
Giannuzzi, L. A., and Stevie, F. A. (1999). A review of focused ion beam milling techniques for TEM specimen preparation. Micron 30, 197–204. doi:10.1016/S0968-4328(99)00005-0
Giepmans, B. N. G., Deerinck, T. J., Smarr, B. L., Jones, Y. Z., and Ellisman, M. H. (2005). Correlated light and electron microscopic imaging of multiple endogenous proteins using Quantum dots. Nat. Methods 2, 743–749. doi:10.1038/nmeth791
Gilbert, M. A. G., Fatima, N., Jenkins, J., O’Sullivan, T. J., Schertel, A., Halfon, Y., et al. (2023). In situ cryo-electron tomography of β-amyloid and tau in post-mortem Alzheimer’s disease brain. Available at: https://www.biorxiv.org/content/.
Giri, N., Roy, R. S., and Cheng, J. (2023). Deep learning for reconstructing protein structures from cryo-EM density maps: recent advances and future directions. Curr. Opin. Struct. Biol. 79, 102536. doi:10.1016/j.sbi.2023.102536
Glaeser, R. M., and Han, B.-G. (2017). Opinion: hazards faced by macromolecules when confined to thin aqueous films. Biophys. Rep. 3, 1–7. doi:10.1007/s41048-016-0026-3
Gorelick, S., Buckley, G., Gervinskas, G., Johnson, T. K., Handley, A., Caggiano, M. P., et al. (2019). PIE-scope, integrated cryo-correlative light and FIB/SEM microscopy. eLife 8, e45919. doi:10.7554/elife.45919
Graham, L., and Orenstein, J. M. (2007). Processing tissue and cells for transmission electron microscopy in diagnostic pathology and research. Nat. Protoc. 2, 2439–2450. doi:10.1038/nprot.2007.304
Graham, M., and Zhang, P. (2023). Cryo-electron tomography to study viral infection. Biochem. Soc. Trans. 51, 1701–1711. doi:10.1042/BST20230103
Griffith, J., Mari, M., De Mazière, A., and Reggiori, F. (2008). A cryosectioning procedure for the ultrastructural analysis and the immunogold labelling of yeast Saccharomyces cerevisiae. Traffic 9, 1060–1072. doi:10.1111/j.1600-0854.2008.00753.x
Grimm, R., Bärmann, M., Häckl, W., Typke, D., Sackmann, E., and Baumeister, W. (1997). Energy filtered electron tomography of ice-embedded actin and vesicles. Biophys. J. 72, 482–489. doi:10.1016/S0006-3495(97)78689-2
Grünewald, K., and Cyrklaff, M. (2006). Structure of complex viruses and virus-infected cells by electron cryo tomography. Curr. Opin. Microbiol. 9, 437–442. doi:10.1016/j.mib.2006.06.016
Guérin, C. J., Kremer, A., Borghgraef, P., Shih, A. Y., and Lippens, S. (2019). “Chapter 5 - combining serial block face and focused ion beam scanning electron microscopy for 3D studies of rare events,” in Methods in cell biology, Editors T. Müller-Reichert, and G. Pigino (Academic Press), 87–101. doi:10.1016/bs.mcb.2019.03.014
Guo, Q., Lehmer, C., Martínez-Sánchez, A., Rudack, T., Beck, F., Hartmann, H., et al. (2018). In situ structure of neuronal C9orf72 poly-GA aggregates reveals proteasome recruitment. Cell 172, 696–705. e12. doi:10.1016/j.cell.2017.12.030
Gurcan, M. N., Boucheron, L. E., Can, A., Madabhushi, A., Rajpoot, N. M., and Yener, B. (2009). Histopathological image analysis: a review. IEEE Rev. Biomed. Eng. 2, 147–171. doi:10.1109/RBME.2009.2034865
Hamaguchi, T., Maki-Yonekura, S., Naitow, H., Matsuura, Y., Ishikawa, T., and Yonekura, K. (2019). A new cryo-EM system for single particle analysis. J. Struct. Biol. 207, 40–48. doi:10.1016/j.jsb.2019.04.011
Hampton, C. M., Strauss, J. D., Ke, Z., Dillard, R. S., Hammonds, J. E., Alonas, E., et al. (2017). Correlated fluorescence microscopy and cryo-electron tomography of virus-infected or transfected mammalian cells. Nat. Protoc. 12, 150–167. doi:10.1038/nprot.2016.168
Hanna, M. G., and Pantanowitz, L. (2019). “Digital pathology,” in Encyclopedia of biomedical engineering Editor R. Narayan (Oxford: Elsevier), 524–532. doi:10.1016/B978-0-12-801238-3.99958-6
Harapin, J., Börmel, M., Sapra, K. T., Brunner, D., Kaech, A., and Medalia, O. (2015). Structural analysis of multicellular organisms with cryo-electron tomography. Nat. Methods 12, 634–636. doi:10.1038/nmeth.3401
Harms, P. W., Frankel, T. L., Moutafi, M., Rao, A., Rimm, D. L., Taube, J. M., et al. (2023). Multiplex immunohistochemistry and immunofluorescence: a practical update for pathologists. Mod. Pathol. 36, 100197. doi:10.1016/j.modpat.2023.100197
Hayashi, S., Ohno, N., Knott, G., and Molnár, Z. (2023). Correlative light and volume electron microscopy to study brain development. Microscopy 72, 279–286. doi:10.1093/jmicro/dfad002
He, J., Lin, P., Chen, J., Cao, H., and Huang, S.-Y. (2022). Model building of protein complexes from intermediate-resolution cryo-EM maps with deep learning-guided automatic assembly. Nat. Commun. 13, 4066. doi:10.1038/s41467-022-31748-9
Hecksel, C. W., Darrow, M. C., Dai, W., Galaz-Montoya, J. G., Chin, J. A., Mitchell, P. G., et al. (2016). Quantifying variability of manual annotation in cryo-electron tomograms. Microsc. Microanal. 22, 487–496. doi:10.1017/S1431927616000799
Heinrich, L., Bennett, D., Ackerman, D., Park, W., Bogovic, J., Eckstein, N., et al. (2021). Whole-cell organelle segmentation in volume electron microscopy. Nature 599, 141–146. doi:10.1038/s41586-021-03977-3
Henriques, R., Griffiths, C., Hesper Rego, E., and Mhlanga, M. M. (2011). PALM and STORM: unlocking live-cell super-resolution. Biopolymers 95, 322–331. doi:10.1002/bip.21586
Hernandez-Gonzalez, M., Calcraft, T., Nans, A., Rosenthal, P. B., and Way, M. (2023). A succession of two viral lattices drives vaccinia virus assembly. PLoS Biol. 21, e3002005. doi:10.1371/journal.pbio.3002005
Himes, B. A., and Zhang, P. (2018). emClarity: software for high-resolution cryo-electron tomography and subtomogram averaging. Nat. Methods 15, 955–961. doi:10.1038/s41592-018-0167-z
Hoffman, D. P., Shtengel, G., Xu, C. S., Campbell, K. R., Freeman, M., Wang, L., et al. (2020). Correlative three-dimensional super-resolution and block-face electron microscopy of whole vitreously frozen cells. Science 367, eaaz5357. doi:10.1126/science.aaz5357
Hofman, F. M., and Taylor, C. R. (2013). Immunohistochemistry. Curr. Protoc. Immunol. 103, 21.4.1–21. doi:10.1002/0471142735.im2104s103
Hrabe, T., Chen, Y., Pfeffer, S., Cuellar, L. K., Mangold, A.-V., and Förster, F. (2012). PyTom: a python-based toolbox for localization of macromolecules in cryo-electron tomograms and subtomogram analysis. J. Struct. Biol. 178, 177–188. doi:10.1016/j.jsb.2011.12.003
Hryc, C. F., and Baker, M. L. (2022). AlphaFold2 and CryoEM: revisiting CryoEM modeling in near-atomic resolution density maps. iScience 25, 104496. doi:10.1016/j.isci.2022.104496
Jadav, N., Velamoor, S., Huang, D., Cassin, L., Hazelton, N., Eruera, A.-R., et al. (2023). Beyond the surface: investigation of tumorsphere morphology using volume electron microscopy. J. Struct. Biol. 215, 108035. doi:10.1016/j.jsb.2023.108035
Jain, T., Sheehan, P., Crum, J., Carragher, B., and Potter, C. S. (2012). Spotiton: a prototype for an integrated inkjet dispense and vitrification system for cryo-TEM. J. Struct. Biol. 179, 68–75. doi:10.1016/j.jsb.2012.04.020
Jensen, E. (2014). Technical review: in situ hybridization. Anat. Rec. 297, 1349–1353. doi:10.1002/ar.22944
Jensen, E. C. (2012). Use of fluorescent probes: their effect on cell biology and limitations. Anat. Rec. 295, 2031–2036. doi:10.1002/ar.22602
Jiménez de la Morena, J., Conesa, P., Fonseca, Y. C., de Isidro-Gómez, F. P., Herreros, D., Fernández-Giménez, E., et al. (2022). ScipionTomo: towards cryo-electron tomography software integration, reproducibility, and validation. J. Struct. Biol. 214, 107872. doi:10.1016/j.jsb.2022.107872
Jin, J., Galaz-Montoya, J. G., Sherman, M. B., Sun, S. Y., Goldsmith, C. S., O’Toole, E. T., et al. (2018). Neutralizing antibodies inhibit chikungunya virus budding at the plasma membrane. Cell Host Microbe 24, 417–428. e5. doi:10.1016/j.chom.2018.07.018
Kato, T., Makino, F., Nakane, T., Terahara, N., Kaneko, T., Shimizu, Y., et al. (2019). CryoTEM with a cold field emission gun that moves structural biology into a new stage. Microsc. Microanal. 25, 998–999. doi:10.1017/S1431927619005725
Khan, S., Naseer, M., Hayat, M., Zamir, S. W., Khan, F. S., and Shah, M. (2022). Transformers in vision: a survey. ACM Comput. Surv. 54, 1–41. doi:10.1145/3505244
Khavnekar, S., Erdmann, P., and Wan, W. (2023). TOMOMAN: streamlining cryo-electron tomography and subtomogram averaging workflows using TOMOgram MANager. Microsc. Microanal. 29, 1020. doi:10.1093/micmic/ozad067.516
Kievits, A. J., Lane, R., Carroll, E. C., and Hoogenboom, J. P. (2022). How innovations in methodology offer new prospects for volume electron microscopy. J. Microsc. 287, 114–137. doi:10.1111/jmi.13134
Kimanius, D., Forsberg, B. O., Scheres, S. H. W., and Lindahl, E. (2016). Accelerated cryo-EM structure determination with parallelisation using GPUs in RELION-2. Elife 5, e18722. doi:10.7554/eLife.18722
Klein, S., Cortese, M., Winter, S. L., Wachsmuth-Melm, M., Neufeldt, C. J., Cerikan, B., et al. (2020). SARS-CoV-2 structure and replication characterized by in situ cryo-electron tomography. Nat. Commun. 11, 5885. doi:10.1038/s41467-020-19619-7
Klumpe, S., Fung, H. K., Goetz, S. K., Zagoriy, I., Hampoelz, B., Zhang, X., et al. (2021). A modular platform for automated cryo-FIB workflows. Elife 10, e70506. doi:10.7554/eLife.70506
Kornfeld, J., and Denk, W. (2018). Progress and remaining challenges in high-throughput volume electron microscopy. Curr. Opin. Neurobiol. 50, 261–267. doi:10.1016/j.conb.2018.04.030
Kremer, J. R., Mastronarde, D. N., and McIntosh, J. R. (1996). Computer visualization of three-dimensional image data using IMOD. J. Struct. Biol. 116, 71–76. doi:10.1006/jsbi.1996.0013
Kuba, J., Mitchels, J., Hovorka, M., Erdmann, P., Berka, L., Kirmse, R., et al. (2021). Advanced cryo-tomography workflow developments - correlative microscopy, milling automation and cryo-lift-out. J. Microsc. 281, 112–124. doi:10.1111/jmi.12939
Kukulski, W., Schorb, M., Welsch, S., Picco, A., Kaksonen, M., and Briggs, J. A. G. (2011). Correlated fluorescence and 3D electron microscopy with high sensitivity and spatial precision. J. Cell Biol. 192, 111–119. doi:10.1083/jcb.201009037
Kürner, J., and Baumeister, W. (2006). “Cryo-electron tomography reveals the architecture of a bacterial cytoskeleton,” in Complex intracellular structures in prokaryotes Editor J. M. Shively (Berlin, Heidelberg: Springer Berlin Heidelberg), 313–318. doi:10.1007/7171_030
Lamm, L., Righetto, R. D., Wietrzynski, W., Pöge, M., Martinez-Sanchez, A., Peng, T., et al. (2022). MemBrain: a deep learning-aided pipeline for detection of membrane proteins in Cryo-electron tomograms. Comput. Methods Programs Biomed. 224, 106990. doi:10.1016/j.cmpb.2022.106990
Larson, M., Thompson, K., and Wright, E. (2022). National network for cryo-electron tomography application portal. Microsc. Microanal. 28, 3216–3217. doi:10.1017/S1431927622011965
Last, M. G. F., Tuijtel, M. W., Voortman, L. M., and Sharp, T. H. (2023). Selecting optimal support grids for super-resolution cryogenic correlated light and electron microscopy. Sci. Rep. 13, 8270. doi:10.1038/s41598-023-35590-x
Leistner, C., Wilkinson, M., Burgess, A., Lovatt, M., Goodbody, S., Xu, Y., et al. (2023). The in-tissue molecular architecture of β-amyloid pathology in the mammalian brain. Nat. Commun. 14, 2833. doi:10.1038/s41467-023-38495-5
Levin, B. D. A., Spilman, M., and Bammes, B. (2023). Event-based direct detectors and their applications in electron microscopy. Anal. wiley.Com. doi:10.1002/was.000700117
Li, S. (2022). Cryo-electron tomography of enveloped viruses. Trends biochem. Sci. 47, 173–186. doi:10.1016/j.tibs.2021.08.005
Li, S., Zhang, K., and Chiu, W. (2023). Near-atomic resolution cryo-EM image reconstruction of RNA. Methods Mol. Biol. 2568, 179–192. doi:10.1007/978-1-0716-2687-0_12
Liedtke, J., Depelteau, J. S., and Briegel, A. (2022). How advances in cryo-electron tomography have contributed to our current view of bacterial cell biology. J. Struct. Biol. X 6, 100065. doi:10.1016/j.yjsbx.2022.100065
Liu, J., Corroyer-Dulmont, S., Pražák, V., Khusainov, I., Bahrami, K., Welsch, S., et al. (2024). The palisade layer of the poxvirus core is composed of flexible A10 trimers. Nat. Struct. Mol. Biol. doi:10.1038/s41594-024-01218-5
Liu, Y.-T., Zhang, H., Wang, H., Tao, C.-L., Bi, G.-Q., and Zhou, Z. H. (2022). Isotropic reconstruction for electron tomography with deep learning. Nat. Commun. 13, 6482. doi:10.1038/s41467-022-33957-8
Louis, D. N., Gerber, G. K., Baron, J. M., Bry, L., Dighe, A. S., Getz, G., et al. (2014). Computational pathology: an emerging definition. Arch. Pathol. Lab. Med. 138, 1133–1138. doi:10.5858/arpa.2014-0034-ED
Louros, N., Wilkinson, M., Tsaka, G., Ramakers, M., Morelli, C., Garcia, T., et al. (2024). Local structural preferences in shaping tau amyloid polymorphism. Nat. Commun. 15, 1028. doi:10.1038/s41467-024-45429-2
Lucas, B. A., and Grigorieff, N. (2023). Quantification of gallium cryo-FIB milling damage in biological lamellae. Proc. Natl. Acad. Sci. U. S. A. 120, e2301852120. doi:10.1073/pnas.2301852120
Lucić, V., Kossel, A. H., Yang, T., Bonhoeffer, T., Baumeister, W., and Sartori, A. (2007). Multiscale imaging of neurons grown in culture: from light microscopy to cryo-electron tomography. J. Struct. Biol. 160, 146–156. doi:10.1016/j.jsb.2007.08.014
Luther, P. K. (2006). “Sample shrinkage and radiation damage of plastic sections,” in Electron tomography: methods for three-dimensional visualization of structures in the cell Editor J. Frank (New York, NY: Springer New York), 17–48. doi:10.1007/978-0-387-69008-7_2
Ma, J., and Wang, B. (2023). Towards foundation models of biological image segmentation. Nat. Methods 20, 953–955. doi:10.1038/s41592-023-01885-0
Madabhushi, A., and Lee, G. (2016). Image analysis and machine learning in digital pathology: challenges and opportunities. Med. Image Anal. 33, 170–175. doi:10.1016/j.media.2016.06.037
Malik, N., and Rao, M. S. (2013). A review of the methods for human iPSC derivation. Methods Mol. Biol. 997, 23–33. doi:10.1007/978-1-62703-348-0_3
Mani, D. R., Krug, K., Zhang, B., Satpathy, S., Clauser, K. R., Ding, L., et al. (2022). Cancer proteogenomics: current impact and future prospects. Nat. Rev. Cancer 22, 298–313. doi:10.1038/s41568-022-00446-5
Marko, M., Hsieh, C., Moberlychan, W., Mannella, C. A., and Frank, J. (2006). Focused ion beam milling of vitreous water: prospects for an alternative to cryo-ultramicrotomy of frozen-hydrated biological samples. J. Microsc. 222, 42–47. doi:10.1111/j.1365-2818.2006.01567.x
Martins, B., Sorrentino, S., Chung, W.-L., Tatli, M., Medalia, O., and Eibauer, M. (2021). Unveiling the polarity of actin filaments by cryo-electron tomography. Structure 29, 488–498.e4. doi:10.1016/j.str.2020.12.014
Masters, B. R. (2020). “Abbe’s theory of image formation in the microscope,” in Springer series in optical sciences SPringer series in optical sciences (Cham: Springer International Publishing), 65–108. doi:10.1007/978-3-030-21691-7_6
Mastronarde, D. N. (2005). Automated electron microscope tomography using robust prediction of specimen movements. J. Struct. Biol. 152, 36–51. doi:10.1016/j.jsb.2005.07.007
Mastronarde, D. N. (2024). Accurate, automatic determination of astigmatism and phase with Ctfplotter in IMOD. J. Struct. Biol. 216, 108057. doi:10.1016/j.jsb.2023.108057
Mastronarde, D. N., and Held, S. R. (2017). Automated tilt series alignment and tomographic reconstruction in IMOD. J. Struct. Biol. 197, 102–113. doi:10.1016/j.jsb.2016.07.011
Mayer, J., Giannuzzi, L. A., Kamino, T., and Michael, J. (2007). TEM sample preparation and FIB-induced damage. MRS Bull. 32, 400–407. doi:10.1557/mrs2007.63
McDowall, A. W., Chang, J. J., Freeman, R., Lepault, J., Walter, C. A., and Dubochet, J. (1983). Electron microscopy of frozen hydrated sections of vitreous ice and vitrified biological samples. J. Microsc. 131, 1–9. doi:10.1111/j.1365-2818.1983.tb04225.x
McMullan, G., Faruqi, A. R., and Henderson, R. (2016). “Chapter one - direct electron detectors,” in Methods in enzymology Editor R. A. Crowther (Academic Press), 1–17. doi:10.1016/bs.mie.2016.05.056
Milazzo, A.-C., Leblanc, P., Duttweiler, F., Jin, L., Bouwer, J. C., Peltier, S., et al. (2005). Active pixel sensor array as a detector for electron microscopy. Ultramicroscopy 104, 152–159. doi:10.1016/j.ultramic.2005.03.006
Moebel, E., and Kervrann, C. (2022). Towards unsupervised classification of macromolecular complexes in cryo electron tomography: challenges and opportunities. Comput. Methods Programs Biomed. 225, 107017. doi:10.1016/j.cmpb.2022.107017
Moebel, E., Martinez-Sanchez, A., Lamm, L., Righetto, R. D., Wietrzynski, W., Albert, S., et al. (2021). Deep learning improves macromolecule identification in 3D cellular cryo-electron tomograms. Nat. Methods 18, 1386–1394. doi:10.1038/s41592-021-01275-4
Moor, H. (1987). “Theory and practice of high pressure freezing,” in Cryotechniques in biological electron microscopy, Editors R. A. Steinbrecht, and K. Zierold (Berlin, Heidelberg: Springer Berlin Heidelberg), 175–191. doi:10.1007/978-3-642-72815-0_8
Moradi Dakhel, A., Majdinasab, V., Nikanjam, A., Khomh, F., Desmarais, M. C., and Jiang, Z. M. (2023). GitHub copilot AI pair programmer: asset or liability? J. Syst. Softw. 203, 111734. doi:10.1016/j.jss.2023.111734
Morado, D. R., Hu, B., and Liu, J. (2016). Using tomoauto: a protocol for high-throughput automated cryo-electron tomography. J. Vis. Exp., e53608. doi:10.3791/53608
Murata, K., Zhang, Q., Gerardo Galaz-Montoya, J., Fu, C., Coleman, M. L., Osburne, M. S., et al. (2017). Visualizing adsorption of cyanophage P-SSP7 onto marine prochlorococcus. Sci. Rep. 7, 44176. doi:10.1038/srep44176
Musumeci, G. (2014). Past, present and future: overview on histology and histopathology. J. Histol. Histopathol. 1, 5. doi:10.7243/2055-091x-1-5
Nakane, T., Kotecha, A., Sente, A., McMullan, G., Masiulis, S., Brown, P. M. G. E., et al. (2020). Single-particle cryo-EM at atomic resolution. Nature 587, 152–156. doi:10.1038/s41586-020-2829-0
Navarro, P. P. (2022). Quantitative cryo-electron tomography. Front. Mol. Biosci. 9, 934465. doi:10.3389/fmolb.2022.934465
Neikirk, K., Lopez, E.-G., Marshall, A. G., Alghanem, A., Krystofiak, E., Kula, B., et al. (2023). Call to action to properly utilize electron microscopy to measure organelles to monitor disease. Eur. J. Cell Biol. 102, 151365. doi:10.1016/j.ejcb.2023.151365
Ni, T., Frosio, T., Mendonça, L., Sheng, Y., Clare, D., Himes, B. A., et al. (2022). High-resolution in situ structure determination by cryo-electron tomography and subtomogram averaging using emClarity. Nat. Protoc. 17, 421–444. doi:10.1038/s41596-021-00648-5
Niazi, M. K. K., Parwani, A. V., and Gurcan, M. N. (2019). Digital pathology and artificial intelligence. Lancet Oncol. 20, e253–e261. doi:10.1016/S1470-2045(19)30154-8
Nicastro, D., Schwartz, C., Pierson, J., Gaudette, R., Porter, M. E., and McIntosh, J. R. (2006). The molecular architecture of axonemes revealed by cryoelectron tomography. Science 313, 944–948. doi:10.1126/science.1128618
Noble, A. J., and Stagg, S. M. (2015). Automated batch fiducial-less tilt-series alignment in Appion using Protomo. J. Struct. Biol. 192, 270–278. doi:10.1016/j.jsb.2015.10.003
Noble, A. J., Wei, H., Dandey, V. P., Zhang, Z., Tan, Y. Z., Potter, C. S., et al. (2018). Reducing effects of particle adsorption to the air-water interface in cryo-EM. Nat. Methods 15, 793–795. doi:10.1038/s41592-018-0139-3
Obr, M., and Schur, F. K. M. (2019). “Chapter Five - structural analysis of pleomorphic and asymmetric viruses using cryo-electron tomography and subtomogram averaging,” in Advances in virus research Editor F. A. Rey (Academic Press), 117–159. doi:10.1016/bs.aivir.2019.07.008
Ohno, N., Katoh, M., Saitoh, Y., Saitoh, S., and Ohno, S. (2015). Three-dimensional volume imaging with electron microscopy toward connectome. Microscopy 64, 17–26. doi:10.1093/jmicro/dfu112
Ooft, S. N., Weeber, F., Dijkstra, K. K., McLean, C. M., Kaing, S., van Werkhoven, E., et al. (2019). Patient-derived organoids can predict response to chemotherapy in metastatic colorectal cancer patients. Sci. Transl. Med. 11, eaay2574. doi:10.1126/scitranslmed.aay2574
Pallua, J. D., Brunner, A., Zelger, B., Schirmer, M., and Haybaeck, J. (2020). The future of pathology is digital. Pathol. Res. Pract. 216, 153040. doi:10.1016/j.prp.2020.153040
Palmer, C. M., and Löwe, J. (2014). A cylindrical specimen holder for electron cryo-tomography. Ultramicroscopy 137, 20–29. doi:10.1016/j.ultramic.2013.10.016
Palovcak, E., Asarnow, D., Campbell, M. G., Yu, Z., and Cheng, Y. (2020). Enhancing the signal-to-noise ratio and generating contrast for cryo-EM images with convolutional neural networks. IUCrJ 7, 1142–1150. doi:10.1107/S2052252520013184
Peck, A., Carter, S. D., Mai, H., Chen, S., Burt, A., and Jensen, G. J. (2022). Montage electron tomography of vitrified specimens. J. Struct. Biol. 214, 107860. doi:10.1016/j.jsb.2022.107860
Peddie, C. J., and Collinson, L. M. (2014). Exploring the third dimension: volume electron microscopy comes of age. Micron 61, 9–19. doi:10.1016/j.micron.2014.01.009
Peddie, C. J., Genoud, C., Kreshuk, A., Meechan, K., Micheva, K. D., Narayan, K., et al. (2022). Volume electron microscopy. Nat. Rev. Methods Prim. 2, 51. doi:10.1038/s43586-022-00131-9
Peng, R., Fu, X., Mendez, J. H., Randolph, P. S., Bammes, B. E., and Stagg, S. M. (2023). Characterizing the resolution and throughput of the Apollo direct electron detector. J. Struct. Biol. X 7, 100080. doi:10.1016/j.yjsbx.2022.100080
Petricoin, E., Wulfkuhle, J., Espina, V., and Liotta, L. A. (2004). Clinical proteomics: revolutionizing disease detection and patient tailoring therapy. J. Proteome Res. 3, 209–217. doi:10.1021/pr049972m
Plitzko, J. M., Rigort, A., and Leis, A. (2009). Correlative cryo-light microscopy and cryo-electron tomography: from cellular territories to molecular landscapes. Curr. Opin. Biotechnol. 20, 83–89. doi:10.1016/j.copbio.2009.03.008
Poger, D., Yen, L., and Braet, F. (2023). Big data in contemporary electron microscopy: challenges and opportunities in data transfer, compute and management. Cell Biol. 160, 169–192. doi:10.1007/s00418-023-02191-8
Punjani, A., Rubinstein, J. L., Fleet, D. J., and Brubaker, M. A. (2017). cryoSPARC: algorithms for rapid unsupervised cryo-EM structure determination. Nat. Methods 14, 290–296. doi:10.1038/nmeth.4169
Quemin, E. R. J., Machala, E. A., Vollmer, B., Pražák, V., Vasishtan, D., Rosch, R., et al. (2020). Cellular electron cryo-tomography to study virus-host interactions. Annu. Rev. Virol. 7, 239–262. doi:10.1146/annurev-virology-021920-115935
Radermacher, M. (1988). Three-Dimensional reconstruction of single particles from random and nonrandom tilt series. J. Electron Microsc. Tech. 9, 359–394. doi:10.1002/jemt.1060090405
Renaud, J.-P., Chari, A., Ciferri, C., Liu, W.-T., Rémigy, H.-W., Stark, H., et al. (2018). Cryo-EM in drug discovery: achievements, limitations and prospects. Nat. Rev. Drug Discov. 17, 471–492. doi:10.1038/nrd.2018.77
Ribatti, D. (2018). An historical note on the cell theory. Exp. Cell Res. 364, 1–4. doi:10.1016/j.yexcr.2018.01.038
Russo, C. J., and Passmore, L. A. (2014). Electron microscopy: ultrastable gold substrates for electron cryomicroscopy. Science 346, 1377–1380. doi:10.1126/science.1259530
Sanchez-Garcia, R., Gomez-Blanco, J., Cuervo, A., Carazo, J. M., Sorzano, C. O. S., and Vargas, J. (2021). DeepEMhancer: a deep learning solution for cryo-EM volume post-processing. Commun. Biol. 4, 874. doi:10.1038/s42003-021-02399-1
Sanchez-Garcia, R., Segura, J., Maluenda, D., Carazo, J. M., and Sorzano, C. O. S. (2018). Deep Consensus, a deep learning-based approach for particle pruning in cryo-electron microscopy. IUCrJ 5, 854–865. doi:10.1107/S2052252518014392
Santuy, A., Tomás-Roca, L., Rodríguez, J.-R., González-Soriano, J., Zhu, F., Qiu, Z., et al. (2020). Estimation of the number of synapses in the hippocampus and brain-wide by volume electron microscopy and genetic labeling. Sci. Rep. 10, 14014. doi:10.1038/s41598-020-70859-5
Sartori, A., Gatz, R., Beck, F., Rigort, A., Baumeister, W., and Plitzko, J. M. (2007). Correlative microscopy: bridging the gap between fluorescence light microscopy and cryo-electron tomography. J. Struct. Biol. 160, 135–145. doi:10.1016/j.jsb.2007.07.011
Schaffer, M., Pfeffer, S., Mahamid, J., Kleindiek, S., Laugks, T., Albert, S., et al. (2019). A cryo-FIB lift-out technique enables molecular-resolution cryo-ET within native Caenorhabditis elegans tissue. Nat. Methods 16, 757–762. doi:10.1038/s41592-019-0497-5
Scheres, S. H., Zhang, W., Falcon, B., and Goedert, M. (2020). Cryo-EM structures of tau filaments. Curr. Opin. Struct. Biol. 64, 17–25. doi:10.1016/j.sbi.2020.05.011
Schiøtz, O. H., Kaiser, C. J. O., Klumpe, S., Morado, D. R., Poege, M., Schneider, J., et al. (2023). Serial Lift-Out: sampling the molecular anatomy of whole organisms. Nat. Methods. doi:10.1038/s41592-023-02113-5
Schur, F. K. M., Hagen, W. J. H., de Marco, A., and Briggs, J. A. G. (2013). Determination of protein structure at 8.5Å resolution using cryo-electron tomography and sub-tomogram averaging. J. Struct. Biol. 184, 394–400. doi:10.1016/j.jsb.2013.10.015
Schur, F. K. M., Obr, M., Hagen, W. J. H., Wan, W., Jakobi, A. J., Kirkpatrick, J. M., et al. (2016). An atomic model of HIV-1 capsid-SP1 reveals structures regulating assembly and maturation. Science 353, 506–508. doi:10.1126/science.aaf9620
Seifert, R., Markert, S. M., Britz, S., Perschin, V., Erbacher, C., Stigloher, C., et al. (2020). DeepCLEM: automated registration for correlative light and electron microscopy using deep learning. F1000Res 9, 1275. doi:10.12688/f1000research.27158.2
Serbynovskyi, V., Wang, J., Chua, E. Y., Ishemgulova, A., Alink, L. M., Budell, W. C., et al. (2024). CryoCycle your grids: plunge vitrifying and reusing clipped grids to advance cryoEM democratization. Available at: https://www.biorxiv.org/content/10.1101/2024.01.23.576763v1.
Shahmoradian, S. H., Galaz-Montoya, J. G., Schmid, M. F., Cong, Y., Ma, B., Spiess, C., et al. (2013). TRiC’s tricks inhibit huntingtin aggregation. Elife 2, e00710. doi:10.7554/eLife.00710
Shahmoradian, S. H., Galiano, M. R., Wu, C., Chen, S., Rasband, M. N., Mobley, W. C., et al. (2014). Preparation of primary neurons for visualizing neurites in a frozen-hydrated state using cryo-electron tomography. J. Vis. Exp., e50783. doi:10.3791/50783
Shahmoradian, S. H., Lewis, A. J., Genoud, C., Hench, J., Moors, T. E., Navarro, P. P., et al. (2019). Lewy pathology in Parkinson’s disease consists of crowded organelles and lipid membranes. Nat. Neurosci. 22, 1099–1109. doi:10.1038/s41593-019-0423-2
Skalidis, I., Kyrilis, F. L., Tüting, C., Hamdi, F., Chojnowski, G., and Kastritis, P. L. (2022). Cryo-EM and artificial intelligence visualize endogenous protein community members. Structure 30, 575–589.e6. e6. doi:10.1016/j.str.2022.01.001
Spence, J. C. H. (1999). The future of atomic resolution electron microscopy for materials science. Mat. Sci. Eng. R. Rep. 26, 1–49. doi:10.1016/S0927-796X(99)00005-4
Stuart, D. I., Subramaniam, S., and Abrescia, N. G. A. (2016). The democratization of cryo-EM. Nat. Methods 13, 607–608. doi:10.1038/nmeth.3946
Studer, D., Graber, W., Al-Amoudi, A., and Eggli, P. (2001). A new approach for cryofixation by high-pressure freezing. J. Microsc. 203, 285–294. doi:10.1046/j.1365-2818.2001.00919.x
Subramaniam, S., Earl, L. A., Falconieri, V., Milne, J. L., and Egelman, E. H. (2016). Resolution advances in cryo-EM enable application to drug discovery. Curr. Opin. Struct. Biol. 41, 194–202. doi:10.1016/j.sbi.2016.07.009
Tancik, M., Mildenhall, B., Wang, T., Schmidt, D., Srinivasan, P. P., Barron, J. T., et al. (2020). Learned initializations for optimizing coordinate-based neural representations. Available at: http://arxiv.org/abs/2012.02189.
Taqi, S. A., Sami, S. A., Sami, L. B., and Zaki, S. A. (2018). A review of artifacts in histopathology. J. Oral Maxillofac. Pathol. 22, 279. doi:10.4103/jomfp.JOMFP_125_15
Tegunov, D., and Cramer, P. (2019). Real-time cryo-electron microscopy data preprocessing with Warp. Nat. Methods 16, 1146–1152. doi:10.1038/s41592-019-0580-y
Tegunov, D., Xue, L., Dienemann, C., Cramer, P., and Mahamid, J. (2021). Multi-particle cryo-EM refinement with M visualizes ribosome-antibiotic complex at 3.5 Å in cells. Nat. Methods 18, 186–193. doi:10.1038/s41592-020-01054-7
Thompson, R. F., Iadanza, M. G., Hesketh, E. L., Rawson, S., and Ranson, N. A. (2019). Collection, pre-processing and on-the-fly analysis of data for high-resolution, single-particle cryo-electron microscopy. Nat. Protoc. 14, 100–118. doi:10.1038/s41596-018-0084-8
Timmermans, F. J., and Otto, C. (2015). Contributed review: review of integrated correlative light and electron microscopy. Rev. Sci. Instrum. 86, 011501. doi:10.1063/1.4905434
Titze, B., and Genoud, C. (2016). Volume scanning electron microscopy for imaging biological ultrastructure. Biol. Cell 108, 307–323. doi:10.1111/boc.201600024
Trinkaus, V. A., Riera-Tur, I., Martínez-Sánchez, A., Bäuerlein, F. J. B., Guo, Q., Arzberger, T., et al. (2021). In situ architecture of neuronal α-Synuclein inclusions. Nat. Commun. 12, 2110. doi:10.1038/s41467-021-22108-0
Turk, M., and Baumeister, W. (2020). The promise and the challenges of cryo-electron tomography. FEBS Lett. 594, 3243–3261. doi:10.1002/1873-3468.13948
Turoňová, B., Hagen, W. J. H., Obr, M., Mosalaganti, S., Beugelink, J. W., Zimmerli, C. E., et al. (2020). Benchmarking tomographic acquisition schemes for high-resolution structural biology. Nat. Commun. 11, 876. doi:10.1038/s41467-020-14535-2
Valli, J., Garcia-Burgos, A., Rooney, L. M., Vale de Melo E Oliveira, B., Duncan, R. R., and Rickman, C. (2021). Seeing beyond the limit: a guide to choosing the right super-resolution microscopy technique. J. Biol. Chem. 297, 100791. doi:10.1016/j.jbc.2021.100791
van den Tweel, J. G., and Taylor, C. R. (2010). A brief history of pathology: preface to a forthcoming series that highlights milestones in the evolution of pathology as a discipline. Virchows Arch. 457, 3–10. doi:10.1007/s00428-010-0934-4
van der Laak, J., Litjens, G., and Ciompi, F. (2021). Deep learning in histopathology: the path to the clinic. Nat. Med. 27, 775–784. doi:10.1038/s41591-021-01343-4
Van Veen, D., Galaz-Montoya, J. G., Shen, L., Baldwin, P., Chaudhari, A. S., Lyumkis, D., et al. (2024). Missing wedge completion via unsupervised learning with coordinate networks. Available at: https://www.biorxiv.org/content/.
Veesler, D., Campbell, M. G., Cheng, A., Fu, C.-Y., Murez, Z., Johnson, J. E., et al. (2013). Maximizing the potential of electron cryomicroscopy data collected using direct detectors. J. Struct. Biol. 184, 193–202. doi:10.1016/j.jsb.2013.09.003
Vidavsky, N., Akiva, A., Kaplan-Ashiri, I., Rechav, K., Addadi, L., Weiner, S., et al. (2016). Cryo-FIB-SEM serial milling and block face imaging: large volume structural analysis of biological tissues preserved close to their native state. J. Struct. Biol. 196, 487–495. doi:10.1016/j.jsb.2016.09.016
Villa, E., Schaffer, M., Plitzko, J. M., and Baumeister, W. (2013). Opening windows into the cell: focused-ion-beam milling for cryo-electron tomography. Curr. Opin. Struct. Biol. 23, 771–777. doi:10.1016/j.sbi.2013.08.006
Volkert, C. A., and Minor, A. M. (2007). Focused ion beam microscopy and micromachining. MRS Bull. 32, 389–399. doi:10.1557/mrs2007.62
Wagner, J., Schaffer, M., and Fernández-Busnadiego, R. (2017). Cryo-electron tomography-the cell biology that came in from the cold. FEBS Lett. 591, 2520–2533. doi:10.1002/1873-3468.12757
Walz, J., Typke, D., Nitsch, M., Koster, A. J., Hegerl, R., and Baumeister, W. (1997). Electron tomography of single ice-embedded macromolecules: three-dimensional alignment and classification. J. Struct. Biol. 120, 387–395. doi:10.1006/jsbi.1997.3934
Wang, F., Gong, H., Liu, G., Li, M., Yan, C., Xia, T., et al. (2016). DeepPicker: a deep learning approach for fully automated particle picking in cryo-EM. J. Struct. Biol. 195, 325–336. doi:10.1016/j.jsb.2016.07.006
Watkins, S. (2001). Cryosectioning. Curr. Protoc. Mol. Biol. Chapter 14, Unit 14.2. doi:10.1002/0471142727.mb1402s07
Weiner, E., Pinskey, J. M., Nicastro, D., and Otegui, M. S. (2022). Electron microscopy for imaging organelles in plants and algae. Plant Physiol. 188, 713–725. doi:10.1093/plphys/kiab449
Wolff, G., Hagen, C., Grünewald, K., and Kaufmann, R. (2016). Towards correlative super-resolution fluorescence and electron cryo-microscopy. Biol. Cell 108, 245–258. doi:10.1111/boc.201600008
Wu, G.-H., Mitchell, P. G., Galaz-Montoya, J. G., Hecksel, C. W., Sontag, E. M., Gangadharan, V., et al. (2020). Multi-scale 3D cryo-correlative microscopy for vitrified cells. Structure 28, 1231–1237. doi:10.1016/j.str.2020.07.017
Wu, G.-H., Smith-Geater, C., Galaz-Montoya, J. G., Gu, Y., Gupte, S. R., Aviner, R., et al. (2023a). CryoET reveals organelle phenotypes in huntington disease patient iPSC-derived and mouse primary neurons. Nat. Commun. 14, 692. doi:10.1038/s41467-023-36096-w
Wu, T., He, S., Liu, J., Sun, S., Liu, K., Han, Q.-L., et al. (2023b). A brief overview of ChatGPT: the history, status quo and potential future development. IEEE/CAA J. Automatica Sinica 10, 1122–1136. doi:10.1109/JAS.2023.123618
Yang, J. E., Larson, M. R., Sibert, B. S., Kim, J. Y., Parrell, D., Sanchez, J. C., et al. (2023). Correlative montage parallel array cryo-tomography for in situ structural cell biology. Nat. Methods 20, 1537–1543. doi:10.1038/s41592-023-01999-5
Yang, J. E., Larson, M. R., Sibert, B. S., Shrum, S., and Wright, E. R. (2021). CorRelator: interactive software for real-time high precision cryo-correlative light and electron microscopy. J. Struct. Biol. 213, 107709. doi:10.1016/j.jsb.2021.107709
Yi, X., Verbeke, E. J., Chang, Y., Dickinson, D. J., and Taylor, D. W. (2019). Electron microscopy snapshots of single particles from single cells. J. Biol. Chem. 294, 1602–1608. doi:10.1074/jbc.RA118.006686
Yip, K. M., Fischer, N., Paknia, E., Chari, A., and Stark, H. (2020). Atomic-resolution protein structure determination by cryo-EM. Nature 587, 157–161. doi:10.1038/s41586-020-2833-4
Yokoyama, Y., Terada, T., Shimizu, K., Nishikawa, K., Kozai, D., Shimada, A., et al. (2020). Development of a deep learning-based method to identify “good” regions of a cryo-electron microscopy grid. Biophys. Rev. 12, 349–354. doi:10.1007/s12551-020-00669-6
Yoshida, S. R., Maity, B. K., and Chong, S. (2023). Visualizing protein localizations in fixed cells: caveats and the underlying mechanisms. J. Phys. Chem. B 127, 4165–4173. doi:10.1021/acs.jpcb.3c01658
Yu, G., Li, K., and Jiang, W. (2016). Antibody-based affinity cryo-EM grid. Methods 100, 16–24. doi:10.1016/j.ymeth.2016.01.010
Zeng, X., Howe, G., and Xu, M. (2021a). End-to-end robust joint unsupervised image alignment and clustering. Proc. IEEE Int. Conf. Comput. Vis. 2021, 3834–3846. doi:10.1109/iccv48922.2021.00383
Zeng, X., Kahng, A., Xue, L., Mahamid, J., Chang, Y.-W., and Xu, M. (2023). High-throughput cryo-ET structural pattern mining by unsupervised deep iterative subtomogram clustering. Proc. Natl. Acad. Sci. U. S. A. 120, e2213149120. doi:10.1073/pnas.2213149120
Zeng, X., Yang, X., Wang, Z., and Xu, M. (2021b). “Chapter 3 - a survey of deep learning-based methods for cryo-electron tomography data analysis,” in State of the art in neural networks and their applications Editors, A. S. El-Baz, and J. S. Suri (Academic Press), 63–72. doi:10.1016/B978-0-12-819740-0.00003-6
Zhang, H., Li, Y., Liu, Y., Li, D., Wang, L., Song, K., et al. (2023). A method for restoring signals and revealing individual macromolecule states in cryo-ET, REST. Rest. Nat. Commun. 14, 2937. doi:10.1038/s41467-023-38539-w
Zheng, S., Wolff, G., Greenan, G., Chen, Z., Faas, F. G. A., Bárcena, M., et al. (2022). AreTomo: an integrated software package for automated marker-free, motion-corrected cryo-electron tomographic alignment and reconstruction. J. Struct. Biol. X 6, 100068. doi:10.1016/j.yjsbx.2022.100068
Zhong, E. D., Bepler, T., Berger, B., and Davis, J. H. (2021). CryoDRGN: reconstruction of heterogeneous cryo-EM structures using neural networks. Nat. Methods 18, 176–185. doi:10.1038/s41592-020-01049-4
Zhu, Y., Ouyang, Q., and Mao, Y. (2017). A deep convolutional neural network approach to single-particle recognition in cryo-electron microscopy. BMC Bioinforma. 18, 348. doi:10.1186/s12859-017-1757-y
Keywords: cryogenic electron microscopy (cryoEM), cryogenic electron tomography (cryoET), cryogenic volume electron microscopy (cryoVEM), cryogenic focused ion beam milling scanning electron microscopy (cryoFIB-SEM), cryogenic correlative light and electron microscopy (cryoCLEM), artificial intelligence (AI), machine learning (ML), structural digital and computational cellular pathology
Citation: Galaz-Montoya JG (2024) The advent of preventive high-resolution structural histopathology by artificial-intelligence-powered cryogenic electron tomography. Front. Mol. Biosci. 11:1390858. doi: 10.3389/fmolb.2024.1390858
Received: 24 February 2024; Accepted: 08 May 2024;
Published: 29 May 2024.
Edited by:
Edward T. Eng, New York Structural Biology Center, United StatesReviewed by:
Qiangjun Zhou, Vanderbilt University, United StatesChang Liu, Biogen Idec, United States
Copyright © 2024 Galaz-Montoya. This is an open-access article distributed under the terms of the Creative Commons Attribution License (CC BY). The use, distribution or reproduction in other forums is permitted, provided the original author(s) and the copyright owner(s) are credited and that the original publication in this journal is cited, in accordance with accepted academic practice. No use, distribution or reproduction is permitted which does not comply with these terms.
*Correspondence: Jesús G. Galaz-Montoya, amdhbGF6QHN0YW5mb3JkLmVkdQ==