- 1Guizhou University of Traditional Chinese Medicine, Guiyang, China
- 2Air Force Medical University Tangdu Hospital, Xi’an, China
Background: Emerging evidence indicates a correlation between imbalances in intestinal microbiota and changes in plasma metabolites in the progression of asthma. However, the causal link between these factors remains unclear.
Methods: A two-sample Mendelian randomization (MR) study was employed to evaluate the potential causal connection between gut microbiota, plasma metabolites, and asthma susceptibility. Gut microbiota data from expansive genome-wide genotype studies and 16S fecal microbiome datasets were examined by the MiBioGen Alliance. Asthma data were procured from the FinnGen biobank analysis, while comprehensive Genome-Wide Association Studies (GWAS) summary statistics for plasma metabolites were derived from the NHGRI-EBI GWAS Catalog. Fluctuations in intestinal flora and plasma metabolites in asthma patients were evaluated using the weighted mode method. Additionally, pleiotropic and heterogeneity analyses were performed to ascertain the reliability of the findings.
Results: Upon examining the gut microbiota through MR with the IVW method, alongside tests for heterogeneity and pleiotropy, findings reveal a negative association between the abundance of the Christensenellaceae R.7 group and asthma risk. In contrast, the Bifidobacterium and Prevotella 7 genera exhibit a positive association with asthma risk, indicating they may be potential risk factors (p < 0.05). Furthermore, MR analysis of 1,400 metabolites employing Weighted median, IVW, and Weighted mode methods resulted in p-values below 0.05. Subsequent tests for pleiotropy and heterogeneity showed that the levels of 3,5-dichloro-2,6-dihydroxybenzoic acid have a negative correlation with asthma, whereas the phenylalanine to phosphate ratio has a positive correlation, suggesting their potential as risk factors for asthma (p < 0.05).
Conclusion: The current Mendelian randomization study provides evidence supporting a potential causal link between specific gut microbiota taxa, plasma metabolites, and asthma. These findings offer novel perspectives for future research and the development of treatment and prevention strategies for asthma.
1 Introduction
Asthma, also known as bronchial asthma, is a chronic inflammatory condition that impacts the airways. It is marked by intermittent airflow blockage, severe symptoms, increased bronchial sensitivity, and inflammation (John, 2015; Gisell Mosnaim Asthma in Adults, 2023). Studies have revealed that over 300 million individuals worldwide have asthma, with prevalence increasing rapidly in recent years attributed to air pollution and climate change (Wang et al., 2023). As a major global health issue, asthma imposes significant economic costs on individuals and societies.
Host-microorganism interactions locally modulate cellular functions, affect immune responses, and significantly impact disease progression. Multiple studies have demonstrated that the host-microorganism interactions impact local and peripheral tissues (Marsland et al., 2015). In 1980, McDermott and Bienenstock introduced the concept of a “common mucosal immune system”, suggesting an interconnected network across the respiratory, gastrointestinal, oral, and urogenital tracts. This system, composed of tissues, cells, and effector molecules, aims to defend against pathogens. Further, they proposed that the immune cells within this network facilitate cross-communication between different mucosal tissues (McDermott et al., 1980). Growing evidence supports that stimulating the common mucosal immune system in one area can significantly affect another (Tulic and Piche, 2016). Additionally, research indicated that changes in the intestinal microenvironment, including shifts in microbiota composition, are associated with the progression of various lung diseases, suggesting substantial interaction between the intestinal and respiratory mucosal areas (Bruzzese et al., 2014).
Over the last decade, human microbiome studies have shown that our intestinal symbiote plays a crucial role in maintaining bodily balance by modulating immune responses in the gastrointestinal system and beyond (ckhed et al., 2005). Imbalances in intestinal flora can influence lung diseases by altering immune, hormonal, and metabolite balance (Budden et al., 2016). Numerous studies have established a close relationship between plasma metabolites and asthma (Lumia et al., 2011; Chiu et al., 2021; Ekstr ö m et al., 2022). However, the existence of an interactive axis involving gut microbiota, plasma metabolites, and asthma still requires further investigation.
Mendelian Randomization (MR) is a statistical method that combines data from Genome-Wide Association Studies (GWAS) and uses Single Nucleotide Polymorphisms (SNPs) as instrumental variables to draw causal inferences without confounders and biases (Greenland, 2018). Two-sample MR analysis synthesizes SNP exposure and outcome data from distinct GWAS to produce a comprehensive causal estimate (Liu et al., 2023). In this investigation, two-stage MR analysis was employed to explore the relationship between plasma metabolites in GM and 1400 and asthma. This method enhances our understanding of the relationship, providing a theoretical foundation for asthma research and identifying potential innovative treatment strategies.
2 Materials and methods
2.1 Study design
This study employed a two-way MR analysis to discern causal relationships among gut microbiota, plasma metabolites, and asthma, as depicted in Figure 1. This analysis treated gut microbiota and plasma metabolites as exposures, while asthma was considered the outcome. GWAS data for gut microbiota were obtained from the Mibiogen consortium, and plasma metabolite data were derived from the comprehensive metabolite statistics in the NHGRI-EBI GWAS Catalog (Chen et al., 2023). Data on asthma were acquired from the FinnGen research project. Genetic variants from these datasets were utilized as instrumental variables (IVs) in two-sample MR analyses conducted using the “TwoSampleMR” package in R software. The study adhered to three fundamental assumptions for MR analyses: (1) a significant correlation between IVs and exposure factors, (2) no correlation between IVs and any confounding factors associated with both exposure and outcome and (3) the exclusive influence of IVs on outcomes via exposures (Skrivankova et al., 2021). All datasets utilized in this study are publicly available, and ethical approval was obtained from the corresponding institutions for each GWAS involved.
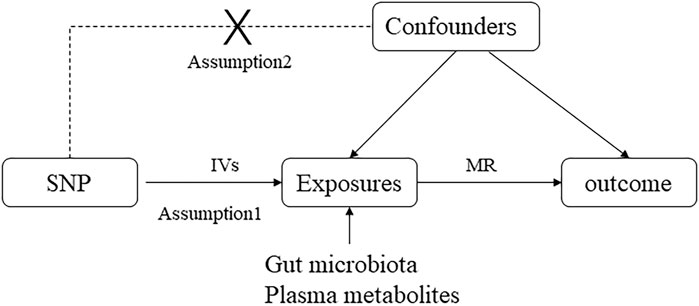
Figure 1. Directed acyclic graphs are employed in classical Mendelian randomization designs. Arrows represent causal relationships between variables, pointing from the cause to the effect. Placing “X” on the arrowed line blocks the causal pathway. MR denotes Mendelian randomization.
2.2 Data sources
The study examined 16S ribosomal RNA gene sequencing and genotyping data across 24 populations from nations including the United States, Canada, Israel, Korea, Germany, Denmark, the Netherlands, Belgium, Sweden, Finland, and the United Kingdom. Our team compiled data on 211 bacterial traits across 9 species, 131 genera, 16 classes, 20 orders, and 35 families, excluding undefined taxa (Kurilshikov et al., 2021). The resulting GWAS summary statistics for all 1,091 blood and 309 plasma metabolites are available for download at the NHGRI-EBI GWAS Catalog (https://www.ebi.ac.uk/gwas/). Additionally, GWAS summary data, including statistics for asthma, were obtained from the FinnGen study involving 53,598 asthma cases and 409,335 control subjects of European ancestry, identified in Dataset: ukb-b-18113.
2.3 Instrumental variables
This study analyzed the association between asthma as the outcome and gut microbiota and plasma metabolites as exposure variables. IVs were selected following specific guidelines. Initially, SNPs linked to the exposure taxa had to reach a genome-wide significance level (p < 1e-05). Subsequently, a linkage disequilibrium (LD) analysis (R2< 0.001, clustering distance = 10,000 kb), based on the European 1,000 Genome Project, was conducted. SNPs that did not adhere to these criteria were excluded. A statistical value F > 10 was the threshold for identifying an IV as strong. IVs not meeting this criterion were considered weakly associated with the exposure and subsequently excluded.
2.4 Mendelian randomization analysis
This study employed methods, including MR Egger, Weighted median, IVW, Simple mode, and Weighted mode, to identify the causal relationship between GM, metabolites, and asthma. IVW was the primary MR method used when horizontal pleiotropy was absent, and its results were considered the main indicator of causation. The correlation between GM, metabolites, and asthma was examined using the other four established MR methods. The MR-Egger intercept test was employed to identify any pleiotropy in the dataset, with a significance level of p < 0.05 indicating the presence of pleiotropy. Heterogeneity was assessed using IVW and MR-Egger tests, with p < 0.05 indicating significant heterogeneity. Additionally, a leave-one-out sensitivity analysis was conducted to determine the influence of specific genetic variants on the results, with scatter plots, forest plots, and funnel plots further illustrating the robustness and sensitivity of the findings.
2.5 Statistical analysis
All statistical analyses, encompassing MR and sensitivity checks, were conducted using R software. The MR analyses were performed with the “Two Sample MR” and “MR-PRESSO” software packages.
3 Results
3.1 Participants and genetic tool variables associated with gut microbiota and metabolites
We refined the gut microbiota exposure data by applying a cut-off at F > 10 and eliminating deficient instrumental variables, which yielded 1531 SNP records (Supplementary Table S1). When we integrated this data with asthma outcome information, we identified seven bacterial species significantly associated with asthma (p < 0.05), as illustrated in Table 1. Similarly, we analyzed metabolite exposure data using F > 10 and excluding weak instrument variables, resulting in 34,843 SNP entries (Supplementary Table S2). Combining these findings with asthma outcomes, we found 219 metabolites significantly associated with asthma (p < 0.05), as depicted in Figure 2.
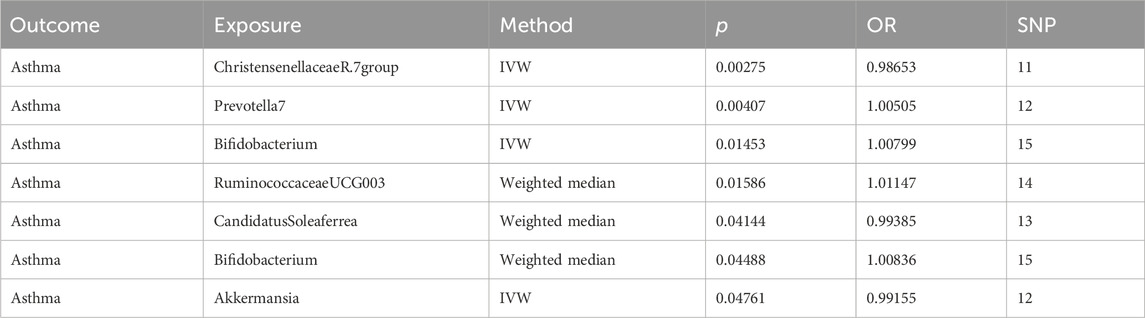
Table 1. MR results of causal relationships between the gut microbiota and asthma, and its phenotypes risk.
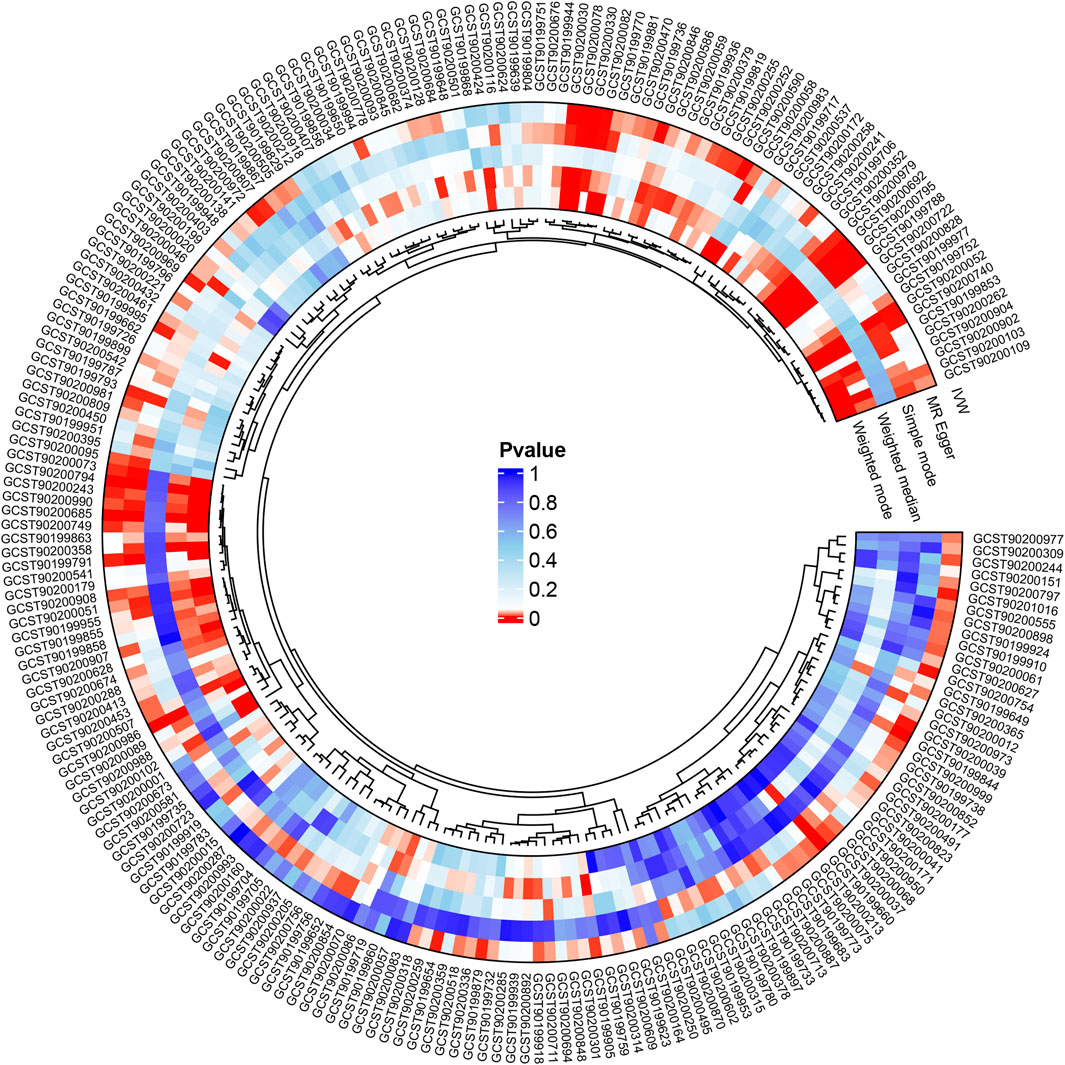
Figure 2. Following the integration of filtered plasma metabolites with asthma outcome data, Mendelian randomization analysis was performed using MR Egger, Weighted median, IVW, Simple mode, and Weighted mode methods, revealing 219 metabolites significantly linked to asthma (p < 0.05).
3.2 Mendelian randomization analysis of intestinal microbiota and asthma
Analysis of intestinal flora via the IVW method revealed certain correlations with asthma risk: Akkermansia and Christensenellaceae R.7 group bacteria demonstrated a negative correlation with asthma risk, suggesting a potential protective role. Conversely, Bifidobacterium and Prevotella7 bacteria showed a positive correlation, indicating they might act as risk factors for asthma (p < 0.05). These findings are illustrated in Figure 3.
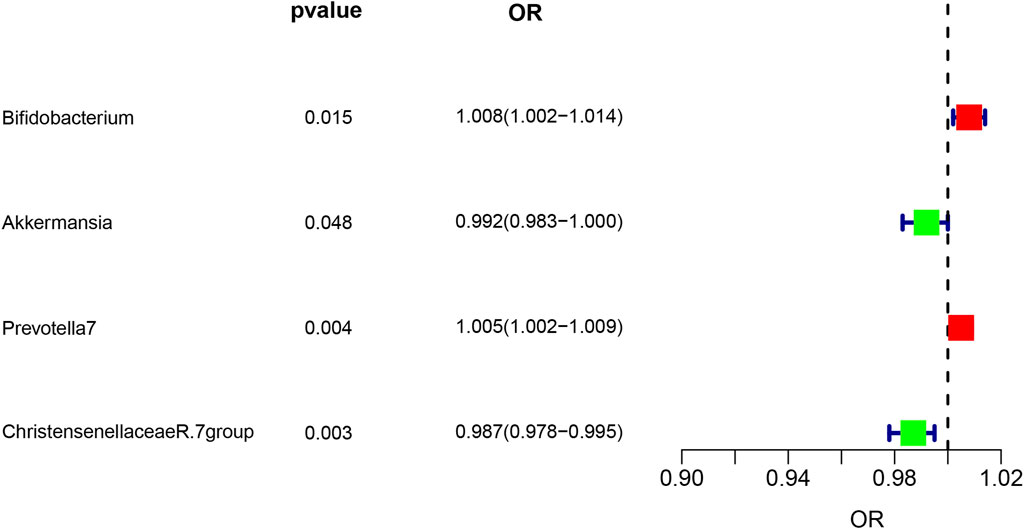
Figure 3. Forest plot illustrating the causal effect of Gut microbiota on asthma risk using the IVW method. Protective factors are depicted in green, while risk factors are shown in red.
3.3 Mendelian randomization analysis of 3 metabolites and asthma
The IVW analysis revealed that 14 were associated with asthma among the identified metabolites, with nine exhibiting negative correlations and five showing positive correlations (p < 0.05), as shown in Figure 4. The application of the Weighted median, IVW, and Weighted mode methods consistently produced significant p-values below 0.05. Particular metabolites such as 1,2-dilinoleoyl-GPC (18:2/18:2), Hydroxyalmitoyl sphingomyelin (d18:1/16:0(OH)), and 3,5-dichloro-2,6-dihydroxybenzoic acid displayed negative correlations with asthma. In contrast, metabolites Arachidronate (20: 4n6) to pyruvate ratio and Phenylalanine to phosphate ratio exhibited positive correlations with asthma, indicating potential risk factors (p < 0.05) (Figure 4), Reverse MR analysis did not reveal any correlation between asthma and metabolites.
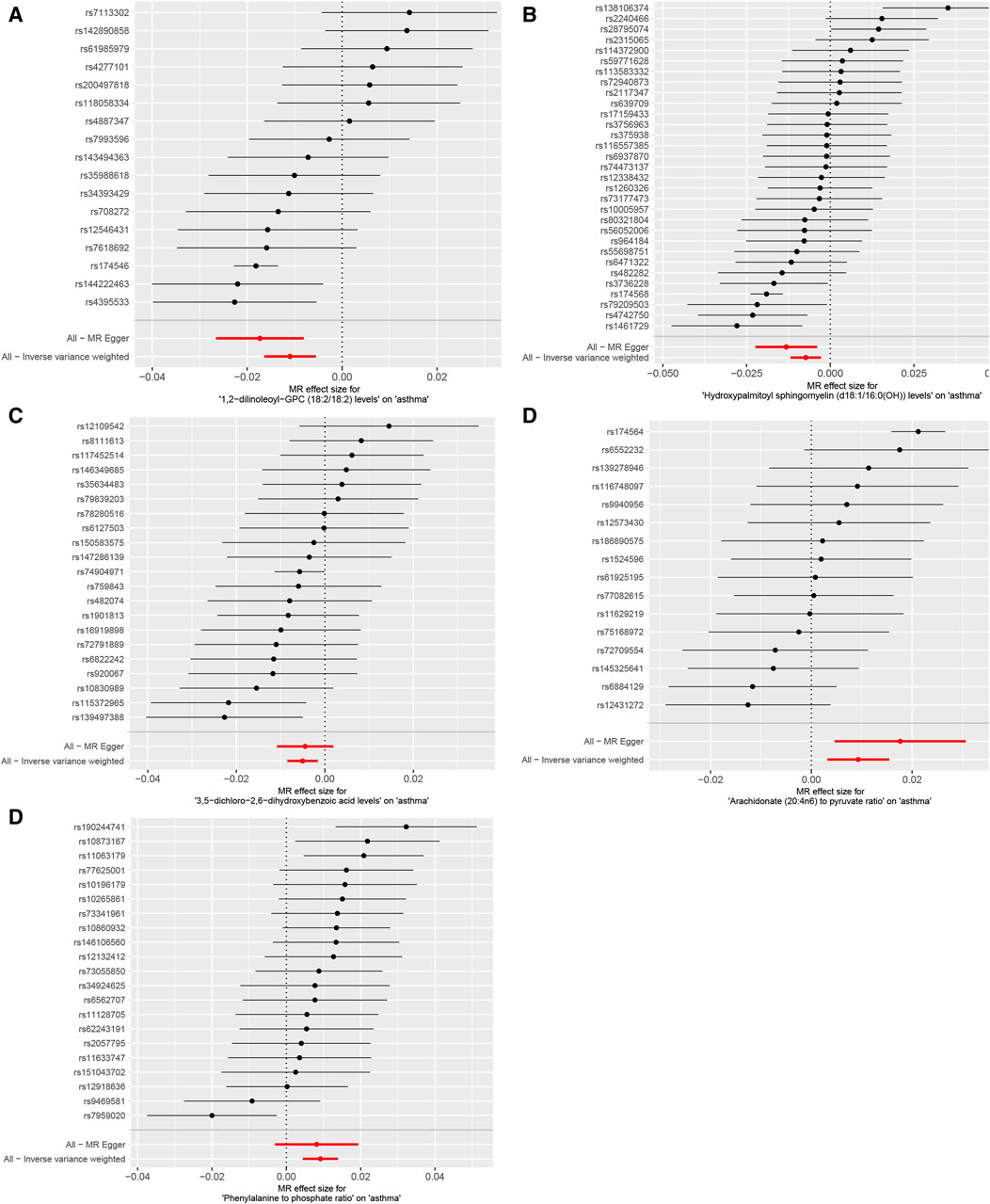
Figure 4. Mendelian randomization analysis visualizes the causal effects of plasma metabolites on asthma risk through forest plots. (A) The levels of 1,2-dilinoleoyl-GPC (18:2/18:2), (B) the levels of Hydroxypalmitoyl sphingomyelin [d18:1/16:0(OH)], (C) the levels of 3,5-dichloro-2,6-dihydroxybenzoic acid, (D) the ratio of Arachidonate (20:4n6) to pyruvate, (E) the ratio of Phenylalanine to phosphate. p-values less than 0.05 for all three methods: Weighted median, IVW, and Weighted mode methodologies.
3.4 Sensitivity analysis
Our Mendelian randomization study on the gut microbiome employed the IVW and MR-Egger methods to test heterogeneity. The results indicated no heterogeneity between Bifidobacterium, Christensenellaceae R.7 group, Prevotella 7, 3,5-dichloro-2,6-dihydroxybenzoic acid levels, and Phenylalanine to phosphate ratio SNP about the outcomes. However, heterogeneity was detected in the relationships involving Akkermansia, 1,2-dilinoleoyl-GPC (18:2/18:2) levels, Hydroxypalmitoyl sphingomyelin [d18:1/16:0(OH)] levels, and Arachidonate (20:4n6) to pyruvate ratio SNP with the outcomes (p > 0.05) (Table 2). The MR-Egger regression intercept did not show a significant deviation from zero, indicating no pleiotropy (p > 0.05) (Table 3). Furthermore, the leave-one-out sensitivity analysis demonstrated that the associations observed between the exposure factors and outcomes were not influenced by any single SNP (Figure 5).
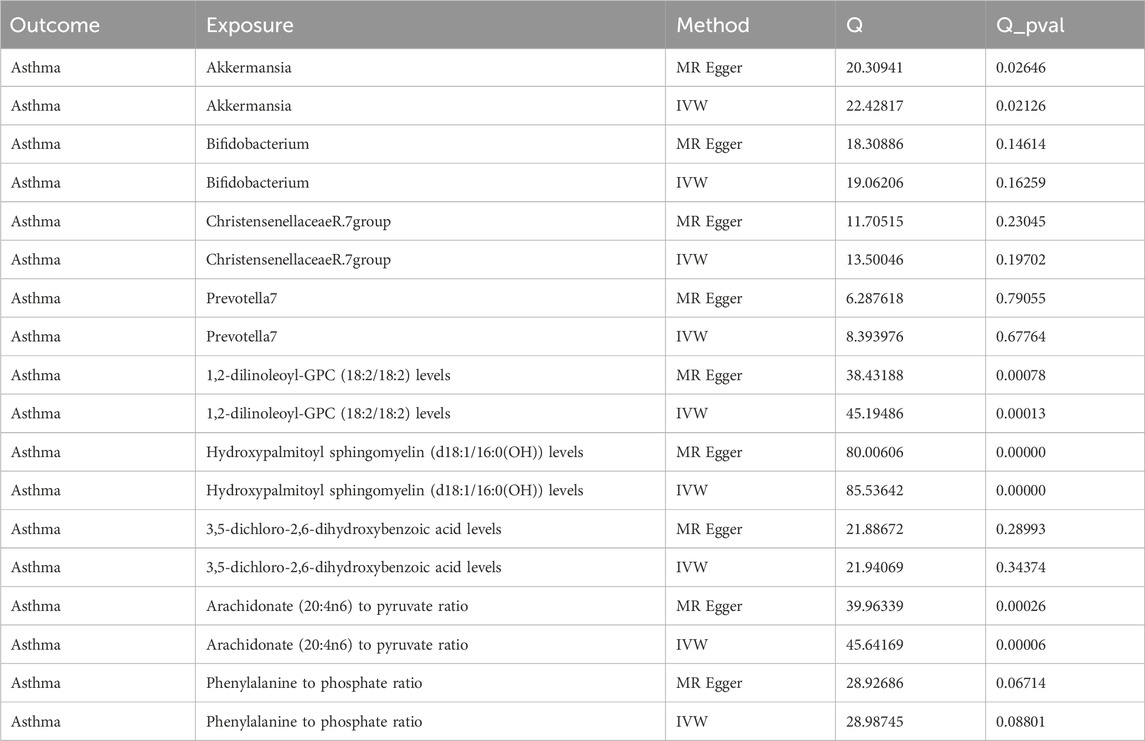
Table 2. Analysis of the heterogeneity in genetic causal effects of gut microbiota and plasma metabolites.
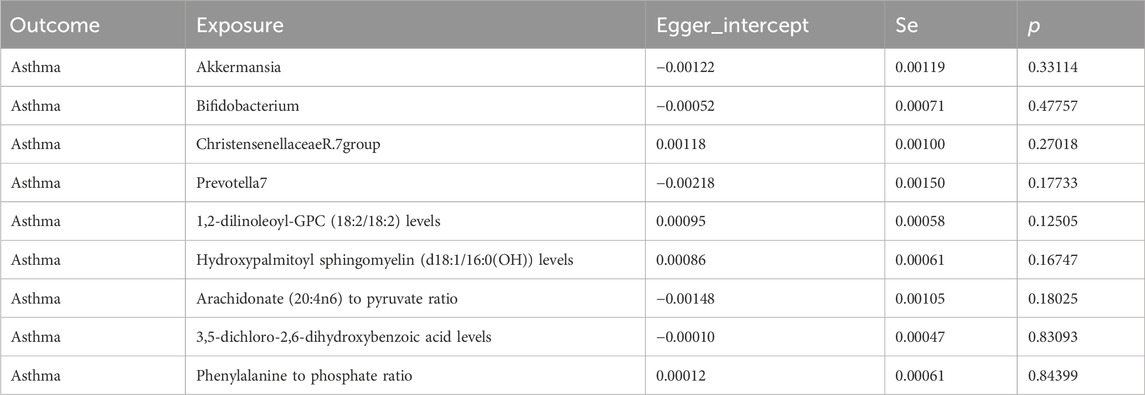
Table 3. Multivariate analysis of the pleiotropic effects of gut microbiota and plasma metabolites on genetic causation.
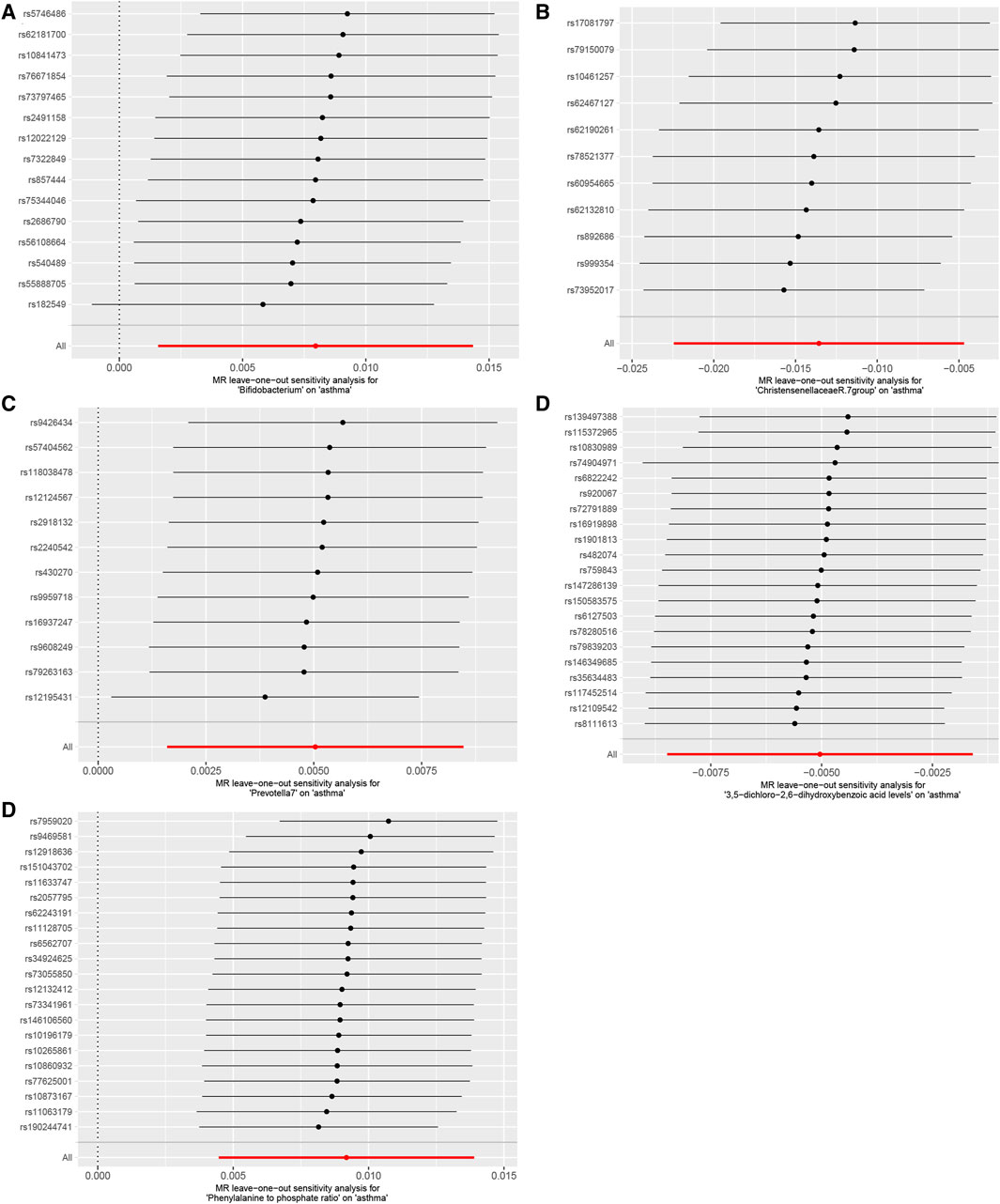
Figure 5. Using the leave-one-out method, the study analyzed the influence of the gut microbiome and blood plasma metabolites on asthma sensitivity, (A). Bifidobacterium; (B). Christensenellaceae R.7 group; (C). Prevotella 7; (D). 3,5-dichloro-2,6-dihydroxybenzoic acid levels; (E). Phenylalanine to phosphate ratio. The results indicated that no individual SNP significantly impacted the overall effect.
4 Discussion
Asthma is a common chronic inflammatory airway disease with complex aetiology, involving multiple factors such as genetics, environment, and immunity (Melissa, 2019; Hammad and Bart, 2021; Stevens et al., 2022). In recent years, the gut microbiome, as a key regulator of host health, has increasingly attracted attention for its relationship with asthma (Logo ń et al., 2023). This study investigated the causal relationship between gut microbiota, plasma metabolites, and asthma using a two-sample Mendelian randomization (MR) approach, combining extensive GWAS meta-analysis data from the MiBioGen consortium and GWAS summary statistics for 1,400 plasma metabolites from the NHGRI-EBI GWAS Catalog database. The results suggest that specific gut microbiota and plasma metabolites may have potential causal relationships with asthma, providing new research directions for asthma prevention and treatment.
Our research found that the abundance of Akkermansia and Christensenellaceae R.7 group were negatively associated with asthma risk, while Bifidobacterium and Prevotella 7 were positively associated with asthma risk. This finding is partially consistent with previous studies, but some discrepancies remain and require further investigation.
Akkermansia muciniphila, the major member of the Akkermansia genus, is a probiotic with significant benefits for host health (Derrien et al., 2004; Derrien et al., 2007; Zhang et al., 2019). Studies have shown that Akkermansia muciniphila can positively impact asthma by regulating gut barrier function, inhibiting inflammatory responses, and other mechanisms. Our research findings indicate that reduced levels of Akkermansia muciniphila may be associated with increased asthma risk, consistent with previous studies. For example, Esraah et al. found that resveratrol supplementation in asthma model mice significantly increased Akkermansia abundance and ameliorated asthma symptoms (Alharris et al., 2022). Additionally, David et al. demonstrated a negative correlation between asthma severity and faecal Akkermansia muciniphila levels (Michalovich et al., 2019). Although heterogeneity tests revealed high variability in Akkermansia muciniphila, it exhibited no pleiotropy. This variability could stem from differences in sequencing platforms, equipment, and operator procedures. Nonetheless, a literature review suggests a significant association between Akkermansia muciniphila and asthma. Our findings imply a potential genetic causal relationship between these gut microbes and asthma risk.
Christensenellaceae R.7 group is a gut microbiome associated with host health, involved in carbohydrate and protein metabolism, producing beneficial short-chain fatty acids, etc. (Morotomi et al., 2011; Demirci et al., 2019; Waters and Ruth, 2019). Chen et al. found that Christensenellaceae_R-7_group was associated with a lower risk of eczema, inhalant allergen sensitization, and physician-diagnosed inhalant allergy (Hu et al., 2021). Our findings suggest that Christensenellaceae R.7 group may positively impact asthma risk by modulating gut immunity and metabolism.
On the other hand, Bifidobacterium and Prevotella 7 were positively associated with asthma risk, suggesting a potential involvement in asthma pathogenesis. Previous studies have shown a protective role of Bifidobacterium in airway inflammation, but our findings contradict this. This may be attributed to the complex pathophysiology of asthma, where Bifidobacterium may play different roles in various asthma subtypes.
Prevotella is a gut microbiome associated with chronic inflammatory diseases, which can activate Toll-like receptor 2, induce Th17-mediated immune responses, and ultimately promote asthma development (Myxedema and conservative heart failure, 1970; Arumugam et al., 2011; Wu et al., 2011; Yadava et al., 2016).
Current research indicates that plasma metabolites may be involved in the development of asthma (Jung et al., 2013). Our study found that plasma metabolite levels of 1,2-dilinoleoyl-GPC (18:2/18:2), Hydroxypalmitoyl sphingomyelin [d18:1/16:0(OH)], and 3,5-dichloro-2,6-dihydroxybenzoic acid were negatively correlated with asthma, while the Arachidonate (20:4n6) to pyruvate ratio and Phenylalanine to phosphate ratio were positively correlated with asthma. These findings provide new insights into the metabolic mechanisms underlying asthma.
3,5-dichloro-2,6-dihydroxybenzoic acid, a potential participant in anti-inflammatory responses, may be produced by certain gut microbiota, which have been shown to generate anti-inflammatory metabolites such as short-chain fatty acids. Mirka et al. found that the intake of Arachidonate (20:4n6) could increase the risk of asthma in offspring (Lumia et al., 2011), and Sandra et al. also found its potential association with asthma development (Ekstr ö m et al., 2022). Our findings suggest that an elevated Arachidonate (20:4n6) to pyruvate ratio may be linked to an increased asthma risk.
Chih et al. revealed that highly sensitised asthma-related metabolites are primarily enriched in pyruvate and acetyl-CoA metabolism (Chiu et al., 2021). Cheryl et al. discovered that pyruvate kinase M2, which converts phosphoenolpyruvate to pyruvate, acts as a coactivator, enhancing the transcription of several pro-inflammatory cytokines (Van De Wettering et al., 2020). Numerous studies have also found that GM can regulate pyruvate metabolism (Cheng et al., 2022; Chen Qu et al., 2023; Xu et al., 2023). Our results suggest that an elevated Arachidonate (20:4n6) to pyruvate ratio might reflect abnormal pyruvate metabolism in asthma patients, thus exacerbating inflammatory responses.
Wei et al. found a significant increase in Phenylalanine levels when inducing allergic asthma in mice (Hsu et al., 2021), a finding supported by other researchers (Liu et al., 2018). It has also been established that GM can disrupt phenylalanine metabolism (Altered metabolome and microbiome features, 2023; Prognostic Value of Gut Microbe, 2023). After conducting heterogeneity tests, we noted that the levels of 1,2-dilinoleoyl-GPC (18:2/18:2), hydroxypalmitoyl sphingomyelin [d18:1/16:0 (OH)], and the arachidonate (20:4n6) to pulmonary ratio exhibit considerable heterogeneity, yet no pleiotropy was detected. A thorough literature review further confirms a strong link between these elements and asthma. Collectively, these findings suggest that GM may influence asthma by altering metabolomic pathways. Presently, knowledge about research on these metabolites remains limited, and studies are comparatively infrequent. More randomized clinical trials and functional experiments are necessary to confirm these findings and clarify the underlying mechanisms.
These findings further support the intricate interplay between gut microbiota, plasma metabolites, and asthma. Gut microbiota may influence host immune systems by modulating the production of metabolic products, contributing to the development of asthma. For instance, specific gut microbiota may suppress asthma-related inflammation by producing anti-inflammatory factors, while others may promote the production of inflammatory factors, exacerbating inflammation.
Our findings offer a novel perspective on understanding the pathophysiology of asthma and provide new avenues for future prevention and treatment strategies. For instance, modulating the composition and function of gut microbiota, through interventions like probiotic supplementation or faecal microbiota transplantation, could potentially improve asthma symptoms. Additionally, modifying dietary patterns to adjust metabolite levels could be explored as a preventive or therapeutic approach for asthma.
However, this study has several limitations. First, it is only an association study and cannot fully demonstrate causation. Future randomised controlled trials are needed to validate these findings. Second, the study only considered the relationship between gut microbiota, plasma metabolites, and asthma. However, changes in gut microbiota can be influenced by various factors such as dietary structure, environmental pollution, and medication use. These factors could affect the composition of gut microbiota and, consequently, the risk of developing asthma. Multi-factor analysis is required to comprehensively uncover the mechanisms underlying asthma development. Finally, the study sample was drawn from a European population, and the pathogenesis of asthma may vary across racial and geographic groups. The genetic background, living environment, and dietary habits of European populations may differ from those of other races, potentially limiting the generalisability of the findings. Future studies need to consider expanding the sample source, incorporating individuals from different racial and geographic groups, to validate the generalizability of the findings.
In conclusion, this study provides new evidence for understanding the causal relationship between gut microbiota, plasma metabolites, and asthma. Further research is needed to investigate the clinical significance of these findings and develop interventions targeting gut microbiota and plasma metabolites to enhance asthma prevention and treatment outcomes.
5 Conclusion
Our findings emphasize the potential role of gut microbiota and its metabolites in asthma pathogenesis, revealing new research directions and insights for innovative diagnostic, therapeutic, and prognostic approaches for this condition.
6 Data inclusion and exclusion criteria
SNPs associated with exposure factor categories reached genome-wide significance threshold (p < 1e-05). SNPs that did not meet the requirements were excluded based on LD analysis (R2< 0.001, clustering distance = 10,000 kb), using the 1,000 Genomes Project data for Europeans; a statistic F > 10 was set as the threshold for strong IVs, otherwise, IVs were considered to have a weak association with exposure and therefore excluded. IVs can only influence the outcome variable through the exposure factor.
Data availability statement
The datasets presented in this study can be found in online repositories. The names of the repository/repositories and accession number(s) can be found in the article/Supplementary Material.
Author contributions
LW: Investigation, Software, Visualization, Writing–original draft. ZL: Writing–original draft, Writing–review and editing, Data curation, Funding acquisition, Methodology, Resources, Supervision.
Funding
The author(s) declare that no financial support was received for the research, authorship, and/or publication of this article.
Acknowledgments
The authors thank all participants and researchers who contributed to this MR study. They also appreciate MiBioGen, NHGRI-EBI GWAS Catalog and FinnGen for the publication of the GWAS summary statistics.
Conflict of interest
The authors declare that the research was conducted in the absence of any commercial or financial relationships that could be construed as a potential conflict of interest.
Publisher’s note
All claims expressed in this article are solely those of the authors and do not necessarily represent those of their affiliated organizations, or those of the publisher, the editors and the reviewers. Any product that may be evaluated in this article, or claim that may be made by its manufacturer, is not guaranteed or endorsed by the publisher.
Supplementary material
The Supplementary Material for this article can be found online at: https://www.frontiersin.org/articles/10.3389/fmolb.2024.1370919/full#supplementary-material
References
Alharris, E., Mohammed, A., Alghetaa, H., Zhou, J., Nagarkatti, M., and Nagarkatti, P. (2022). The ability of resveratrol to attenuate ovalbumin-mediated allergic asthma is associated with changes in microbiota involving the gut-lung Axis, enhanced barrier function and decreased inflammation in the lungs. Front. Immunol. 13, 805770. doi:10.3389/fimmu.2022.805770
Altered metabolome and microbiome features provide clubs in predicting recurs of valuable collectis J. Pharm. Biomed. Analysis, 2023, 239–115864. doi:10.1016/j.jpba.2023.115864
Arumugam, M., Raes, J., Pelletier, É., Le Paslier, D., Yamada, T., Mende, D. R., et al. (2011). Enterotypes of the human gut microbiome. Nature 473 (7346), 174–180. doi:10.1038/nature09944
Bruzzese, E., Callegari, M. L., Raia, V., Viscovo, S., Scotto, R., Ferrari, S., et al. (2014). Disrupted intestinal microbiota and intestinal inflammation in children with cystic fibrosis and its restoration with Lactobacillus GG: a randomised clinical trial. Plos one 9 (2), e87796. doi:10.1371/journal.pone.0087796
Budden, K. F., Gellatly, S. L., Wood, D. L. A., Cooper, M. A., Morrison, M., Hugenholtz, P., et al. (2016). Emerging pathogenic links between microbiota and the gut-lung axis. Nat. Rev. Microbiol. 15 (1), 55–63. doi:10.1038/nrmicro.2016.142
Chen, Q., Ren, R., Yue, S., Xu, J., Yang, H., Li, X., et al. (2023b). The combination of metagenome and metabolome to compare the differential effects and mechanisms of fructose and sucrose on the metabolic disorders and gut microbiota in vitro and in vivo. Food&Function 14 (15), 7284–7298. doi:10.1039/d3fo02246c
Chen, Y., Lu, T., Pettersson kymmer, U., Stewart, I. D., Butler-Laporte, G., Nakanishi, T., et al. (2023a). Genomic atlas of the plasma metabolome prioritizes metabolites implicated in human diseases. Nat. Genet. 55 (1), 44–53. doi:10.1038/s41588-022-01270-1
Cheng, L., Xu, L., Peng, L., Wang, Y., and Wei, X. (2022). Gut microbiota differentially mediated by qingmao tea and qingzhuan tea alleviated high-fat-induced obesity and associated metabolic disorders: the impact of microbial fermentation. Foods 11 (20), 3210. doi:10.3390/foods11203210
Chiu, C., Cheng, M., Chiang, M., Wang, C. J., and Tsai, M. H. (2021). Integrated metabolic and microbial analysis reveals host-microbial interactions in IgE-mediated childhood asthma. Sci. Rep. 11 (1), 23407. doi:10.1038/s41598-021-02925-5
ckhed, F. B., Ley, R. E., Sonnenburg, J. L., Peterson, D. A., and Gordon, J. I. (2005). Host-bacterial mutualism in the human intestine. Science 307 (5717), 1915–1920. doi:10.1126/science.1104816
Demirci, M., Tokman, H. B., Uysal, H. K., Demiryas, S., Karakullukcu, A., Saribas, S., et al. (2019). Reduced Akkermansia muciniphila and Faecalibacterium prausnitzii levels in the gut microbiota of children with allergic asthma. Allegologia Immunopathol. 47 (4), 365–371. doi:10.1016/j.aller2018.12.009
Derrien, M., Carmen Collado, M., Ben amor, K., Salminen, S., and de Vos, W. M. (2007). The Mucin degrader Akkermansia muciniphila is an abundant resident of the human intestinal tract. Appl. Environ. Microbiol. 74 (5), 1646–1648. doi:10.1128/AEM.01226-07
Derrien, M., Vaughan, E. E., Plug, C. M., and de Vos, W. M. (2004). Akkermansia muciniphila gen. nov., sp. nov., a human intestinal mucin-degrading bacterium. Int. J. Syst. Evol. Microbiol. 54 (Pt 5), 1469–1476. doi:10.1099/ijs.0.02873-0
Ekstr ö m, S., Sdona, E., Klevebro, S., Hallberg, J., Georgelis, A., Kull, I., et al. (2022). Dietary intake and plasma concentrations of PUFAs in childhood and adolescence in relation to asthma and lung function up to adulthood. Am. J. Clin. Nutr. 115 (3), 886–896. doi:10.1093/ajcn/nqab427
Gisell Mosnaim Asthma in Adults (2023). Asthma in Adults. new Engl. J. Med. 389 (11), 1023–1031. doi:10.1056/NEJMcp2304871
Greenland, S. (2018). An introduction to instrumental variables for epidemiologists. Int. J. Epidemiol. 47 (1), 358. doi:10.1093/ije/dyx275
Hammad, H., and Bart, N. (2021). The basic immunology of asthma. Cell 184 (6), 1469–1485. doi:10.1016/j.cell.2021.02-016
Hsu, W., Lin, L., Lu, C., Kao, S. T., and Lin, Y. L. (2021). Effect of you gui wan on house dust mite induced mouse allergic asthma via regulating amino acid metabolic disorder and gut dysbiosis. Biomolocules 11 (6), 812. doi:10.3390/biom11060812
Hu, C., Van Meel, E. R., Medina gomez, C., Kraaij, R., Barroso, M., Kiefte-de Jong, J., et al. (2021). A population-based study on associations of stool microbiota with atopic diseases in school-age children. J. Allergy Clin. Immunol. 148 (2), 612–620. doi:10.1016/j.jaci.2021.04.001
John, V. (2015). Fahy Type 2 inflation in asthma - present in most. absent many Nat. Rev. Immunol. 15 (1), 57–65. doi:10.1038/nri3786
Jung, J., Kim, S.-h., Lee, H.-s., Choi, G. S., Jung, Y. S., Ryu, D. H., et al. (2013). Serum metabolomics reveals pathways and biomarkers associated with asthma pathogenesis. Clin. Exp. energy 43 (4), 425–433. doi:10.1111/cea.12089
Kurilshikov, A., gomez, C. M., Bacigalupe, R., Radjabzadeh, D., Wang, J., Demirkan, A., et al. (2021). Large scale association analyses identify host factors influencing human gut microbiome composition. Nat. Genet. 53 (2), 156–165. doi:10.1038/s41588-020-00763-1
Liu, Y., Zheng, J., Zhang, H. P., Zhang, X., Wang, L., Wood, L., et al. (2018). Obesity-associated metabolic signatures correlate to clinical and inflammatory profiles of asthma: a pilot study. Allergy Asthma&Immunology Res. 10 (6), 628–647. doi:10.4168/aair.2018.10.6.628
Liu, Z., Chen, P., Luo, L., Liu, Q., Shi, H., and Yang, X. (2023). Causal effects of gut microbiome on endometriosis: a two-sample mendelian randomization study. BMC women's health 23 (1), 637. doi:10.1186/s12905-023-02742-0
Logo ń, K., Wirkosz, G. Ś., Nowak, M., Wrześniewska, M., Szczygieł, A., and Gomułka, K. (2023). The role of the microbiome in the pathogenesis and treatment of asthma. Biomedicines 11 (6), 1618. doi:10.3390/biomedicines11061618
Lumia, M., ivi Luukkainen, P., Tapanainen, H., Kaila, M., Erkkola, M., Uusitalo, L., et al. (2011). Dietary fatty acid composition during pregnancy and the risk of asthma in the offspring. Pediatr. Allergy Immunol. 22 (8), 827–835. doi:10.1111/j.1399-3038.2011.01202.x
Marsland, B. J., Trompette, A., and Eva, S. (2015). Gollwitzer the gut lung Axis in respiratory disease. Ann. Am. Therm. Soc. 12, 2 S150–S156. doi:10.1513/AnnalsATS.201503-133AW
McDermott, M. R., Clark, D. A., and Bienenstock, J. (1980). Evidence for a common mucosal immunologic system. II. Influence of the estrous cycle on B immunoblast migration into genital and intestinal tissues. J. Immunol. 124 (6), 2536–2539. doi:10.4049/jimmunol.124.6.2536
Melissa, D. G. (2019). Tatyana Gavrilova Understanding the immunology of astrology: pathophysiology, biomarkers, and treatments for astrotypes. Pediatr. Respir. Rev. 36, 118–127. doi:10.1016/j.prrv.2019.08.002
Michalovich, D., Rodriguez-Perez, N., Smolinska, S., Pirozynski, M., Mayhew, D., Uddin, S., et al. (2019). Obesity and disease severity magnify disturbed microbiome-immune interactions in asthma patients. Nat. Commun. 10 (1), 5711. doi:10.1038/s41467-019-13751-9
Morotomi, M., Nagai, F., and Watanabe, Y. (2011). Description of Christensenella minuta gen. nov., sp. nov., isolated from human faeces, which forms a distinct branch in the order Clostridiales, and proposal of Christensenellaceae fam. nov. Nov Int. J. Syst. Evol. Microbiol. 62 (Pt 1), 144–149. doi:10.1099/ijs.0.026989-0
Myxedema and conservative heart failure (1970). Myxedema and congestive heart failure. Ned. Tijdschr. Geneeskd. 114 (42), 1738–1741.
Prognostic value of gut microbe generated metabolite phenolic glutamine in patients with heart failure Eur. J. Heart Fail., 2023, doi:10.1002/ejhf.3111
Skrivankova, V. W., Richmond, R. C., Woolf, B. A. R., Davies, N. M., Swanson, S. A., VanderWeele, T. J., et al. (2021). Strengthening the reporting of observational studies in epidemiology using mendelian randomisation (STROBE-MR): explanation and elaboration. BMJ 375, N2233. doi:10.1136/bmj.n2233
Stevens, J., Steinmeyer, S., Bonfield, M., Peterson, L., Wang, T., Gray, J., et al. (2022). The balance between protective and pathogenic immune responses to pneumonia in the neonatal lung is enforced by gut microbiota. Sci. Transl. Med. 14 (649), eabl3981. doi:10.1126/scitranslmed.abl3981
Tulic, M. K., and Piche, T. (2016). Lung-gut cross-talk: evidence, mechanisms and implications for the mucosal inflammatory diseases. Clin. Exp. energy 46 (4), 519–528. doi:10.1111/cea.12723
Van De Wettering, C., Aboushusha, R., Manuel, A. M., Chia, S. B., Erickson, C., MacPherson, M. B., et al. (2020). Pyruvate kinase M2 promotes expression of proinflammatory mediators in house dust mite-induced allergic airways disease. J. Immunol. 204 (4), 763–774. doi:10.4049/jimmunol.1901086
Wang, Y., Kui Mou, Y., Wang, H. rui, Song, X. Y., Wei, S. Z., Ren, C., et al. (2023). Brain response in asthma: the role of "lung-brain" axis mediated by neuroimmune crosstalk. Front. Immunol. 14, 1240248. doi:10.3389/fimmu.2023.1240248
Waters, J. L., and Ruth, E. (2019). The human gut bacteria Christensenellaceae are widespread, heritable, and associated with health. BMC Biol. 17 (1), 83. doi:10.1186/s12915-019-0699-4
Wu, G. D., Chen, J., Hoffmann, C., Bittinger, K., Chen, Y. Y., Keilbaugh, S. A., et al. (2011). Linking long-term dietary patterns with gut microbial enterotypes. Science 334 (6052), 105–108. doi:10.1126/science.1208344
Xu, H., Yao, Y., Li, D., Zhu, W., Xiao, H., Xie, M., et al. (2023). Metagenome and metabolome insights into the energy compensation and exogenous toxin degradation of gut microbiota in high-altitude rhesus macaques (Macaca mulatta). NPJ biofilms microbiomes 9 (1), 20. doi:10.1038/s41522-023-00387-3
Yadava, K., é line Pattaroni, C., Sichelstiel, A. K., Trompette, A., Gollwitzer, E. S., Salami, O., et al. (2016). Microbiota promotes chronic pulmonary inflammation by enhancing IL-17A and autoantibodies. Am. J. Respir. Crit. Care Med. 193 (9), 975–987. doi:10.1164/rccm.201504-0779OC
Keywords: Mendelian randomization, intestinal flora, asthma, plasma metabolites, causality
Citation: Wang L and Lv Z (2024) Causal associations among gut microbiota, 1400 plasma metabolites, and asthma: a two-sample Mendelian randomization study. Front. Mol. Biosci. 11:1370919. doi: 10.3389/fmolb.2024.1370919
Received: 15 January 2024; Accepted: 21 June 2024;
Published: 22 July 2024.
Edited by:
Nilesh Sudhakar Ambhore, North Dakota State University, United StatesReviewed by:
Premanand Balraj, North Dakota State University, United StatesSangeeta Bhallamudi, New York University, United States
Copyright © 2024 Wang and Lv. This is an open-access article distributed under the terms of the Creative Commons Attribution License (CC BY). The use, distribution or reproduction in other forums is permitted, provided the original author(s) and the copyright owner(s) are credited and that the original publication in this journal is cited, in accordance with accepted academic practice. No use, distribution or reproduction is permitted which does not comply with these terms.
*Correspondence: Zhe Lv, bHZ6aGUyMDMxQDE2My5jb20=