- 1Department of Basic and Applied Sciences for Engineering (SBAI), Università“Sapienza”, Roma, Italy
- 2Department of Biochemical Sciences “Alessandro Rossi Fanelli”, Università“Sapienza”, Roma, Italy
- 3Department of Biology and Biotechnologies “Charles Darwin”, Università“Sapienza”, Roma, Italy
- 4Centre for Human Technologies (CHT), Istituto Italiano di Tecnologia (IIT), Genova, Italy
- 5Center for Life Nano and Neuro Science, Istituto Italiano di Tecnologia (IIT), Roma, Italy
- 6Department of Physics, Università“Sapienza”, Roma, Italy
The development of methods able to modulate the binding affinity between proteins and peptides is of paramount biotechnological interest in view of a vast range of applications that imply designed polypeptides capable to impair or favour Protein-Protein Interactions. Here, we applied a peptide design algorithm based on shape complementarity optimization and electrostatic compatibility and provided the first experimental in vitro proof of the efficacy of the design algorithm. Focusing on the interaction between the SARS-CoV-2 Spike Receptor-Binding Domain (RBD) and the human angiotensin-converting enzyme 2 (ACE2) receptor, we extracted a 23-residues long peptide that structurally mimics the major interacting portion of the ACE2 receptor and designed in silico five mutants of such a peptide with a modulated affinity. Remarkably, experimental KD measurements, conducted using biolayer interferometry, matched the in silico predictions. Moreover, we investigated the molecular determinants that govern the variation in binding affinity through molecular dynamics simulation, by identifying the mechanisms driving the different values of binding affinity at a single residue level. Finally, the peptide sequence with the highest affinity, in comparison with the wild type peptide, was expressed as a fusion protein with human H ferritin (HFt) 24-mer. Solution measurements performed on the latter constructs confirmed that peptides still exhibited the expected trend, thereby enhancing their efficacy in RBD binding. Altogether, these results indicate the high potentiality of this general method in developing potent high-affinity vectors for hindering/enhancing protein-protein associations.
1 Introduction
Protein-protein interactions (PPIs) play a pivotal role in numerous biological processes. Within these interactions, selected mutations at the interfaces can lead to significant changes in binding affinity, resulting in physiological or pathological phenotypes (Forbes et al., 2015; Landrum et al., 2016). Indeed, the stability of PPIs is the result of a complex fine-tuning of chemical-physical properties at the interfaces and entropic effects (Vangone and Bonvin, 2015; Desantis et al., 2022), making the substitution of even just one residue potentially disruptive. Therefore, in the last years, several computational methods have been developed to predict the effects of mutations on binding, based on a wide variety of techniques (Moretti et al., 2013; Brender and Zhang, 2015; Geng et al., 2019; Rodrigues et al., 2019). In addition, it has been recently presented a protocol for amino acid refinement through a computational method for the design of peptides and proteins interface (Ochoa et al., 2021).
A paramount example of such a PPI is the complex formation between the SARS-CoV-2 RBD and the human ACE2 receptor protein. This interaction is crucial for viral infection, as it triggers a cascade of events ultimately leading to viral entry into the host cell (Walls et al., 2020). Also in this case, mutations in the RBD or ACE2 interface have been shown to affect virus infectivity and disease conditions (Barton et al., 2021). Considering the significance of this interaction, considerable efforts have been devoted to the development of computational methods for predicting mutation effects in the RBD-ACE2 interface (Zou et al., 2020; Bai et al., 2021; Miotto et al., 2022; Miotto et al., 2023).
To date, several experimental structures of the complex between ACE2 and the receptor-binding domain of the SARS-CoV-2 Spike protein have been determined, providing the structural basis for the specific interaction mechanism and highlighting the critical residues involved in the complex formation (Shang et al., 2020). Interestingly, the peptide “IEEQAKTFLDKFNHEAEDLFYQSSLASWNYNTN” (residues 21–53) appears to mimic the major interacting portion of the ACE2 receptor to the SARS-CoV-2 Spike RBD (Kuznetsov et al., 2022). Therefore, it is not surprising that peptides of different lengths, including residues 24 to 53 of the ACE2 receptor, have commonly been reported to exhibit high affinity binding to various regions of the SARS-CoV-2 Spike protein (de Campos et al., 2021; Larue et al., 2021). Many of these peptides, derived from the N-terminal α-helix of ACE2, have been tested in response to the SARS-CoV-2 pandemic (Tzotzos, 2022). Since the SARS-CoV-2 virus enters cells through the interaction between the Spike glycoprotein and ACE2 ectodomain, disrupting the Spike/ACE2 interaction represents a major target for preventing cell infection (Gheblawi et al., 2020; Papageorgiou and Mohsin, 2020).
In the present investigation, our focus was devoted to the exploration of the α-helix peptide derived from the N-terminus of the ACE2 sequence, specifically encompassing residues 21–43, in its interactions with the RBD protein. Upon application of a protocol design discussed previously (Di Rienzo et al., 2021a), we were able to generate a set of peptide mutants with a modulated affinity with Spike protein. Such a protocol is based, beyond a coarse-grained evaluation of electrostatics compatibility, on the application of the 2D Zernike formalism, to obtain a compact representation of the local shape of molecular surfaces (Milanetti et al., 2021). In this framework, the geometry of a molecular region is described by an ordered set of numbers: this ensures an easy evaluation of the shape complementarity between two molecular regions by calculating the euclidean distance between the corresponding Zernike descriptors. Therefore, when a residue is substituted, it is possible to evaluate whether the shape of the mutated peptide is more complementary with the molecular partner. In the past years, this formalism has proven its efficacy in similar optimization protocols (Di Rienzo et al., 2020; De Lauro et al., 2022; Di Rienzo et al., 2023a), or more in general to evaluate the local similarity or complementarity (Venkatraman et al., 2009; Daberdaku and Ferrari, 2018; 2019; Miotto et al., 2021; Di Rienzo et al., 2022; Piacentini et al., 2022; Di Rienzo et al., 2023b). Hence, we generated a set of five ACE2-derived peptide mutants, four of them endowed with predicted higher affinity for the RBD protein with respect to the wildtype (WT) peptide and one with predicted lower affinity.
Initially, we confirmed, with extensive molecular dynamics simulations of the six peptides (WT + five mutants), that even when the peptides are extracted from the whole ACE2 structure, they maintain the elongated α-helix structure. The peptides were subsequently synthesized and subjected to rigorous in vitro testing using biolayer interferometry methods (BLI). These analyses enabled us to experimentally determine the binding affinity between the peptides and the RBD protein, strongly confirming the accuracy of our computational predictions. Thus, to gain insight into the molecular mechanisms driving the increase of the binding affinity we performed molecular dynamics simulations of the complexes between RBD and each of the six peptides.
Finally, as a proof of concept, we genetically fused either the wild-type ACE2 peptide or the optimized peptide with the highest affinity for RBD at the N-terminus of human H ferritin 24-mer. Ferritin is a naturally occurring human protein that thanks to its endogenous nature ensures excellent biocompatibility, biodegradability, and low toxicity, essential features for clinical applications. Its symmetrical spherical architecture, high thermal stability, self-assembly ability, and ease of production in recombinant form make it a promising platform for drug delivery and vaccine development. These structural features make it an ideal candidate for clinical nanocarrier applications. The fusion with ferritin aims to overcome the limitations associated with free peptides, such as rapid renal clearance and reduced bioavailability, by leveraging the multivalent effect of the ferritin’s 24-meric structure. This strategy has the potential to significantly enhance the therapeutic efficacy of peptide-based treatments against SARS-CoV-2 by improving their stability, bioavailability, and virus neutralization capacity. Prior research on ferritin-based drug delivery systems (N. Song, et al., 2021; Palombarini et al., 2020), and in the context of SARS-CoV-2 treatment and vaccine development (Kalathiya et al., 2021; Khoshnejad et al., 2018; Kim et al., 2017; Kim et al., 2022; Kanekiyo et al., 2013; Powell et al., 2021; Sliepen et al., 2015), provides a foundation for the investigation reported in the present paper.
2 Results and discussion
2.1 Computational analysis of the ACE2-derived peptides
In a previous paper, we presented a designed computational protocol aiming to generate soluble-ACE2 mutants with increased or decreased affinity with SARS-CoV-2 Spike (Di Rienzo et al., 2021b). Here, we applied the same protocol, restraining the application onto residues into the α-helix peptide range 21–43. The spatial arrangement of the peptide within the ACE2-RBD complex can be observed in Figure 1.
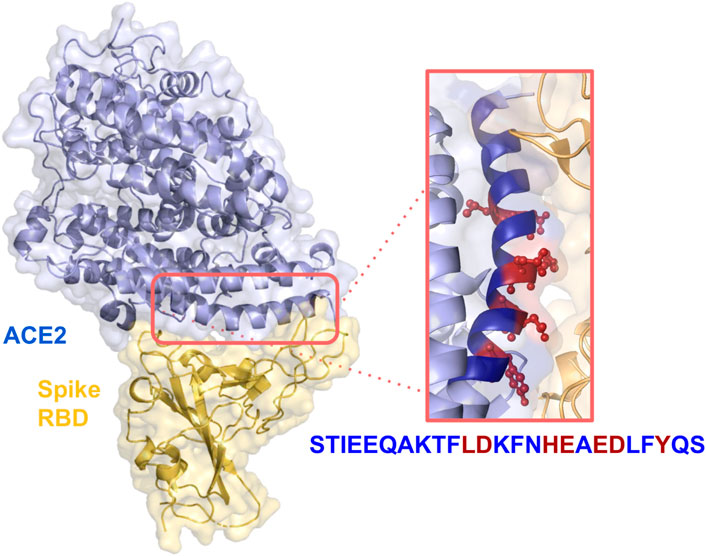
FIGURE 1. Crystal structure of SARS-CoV-2 spike receptor-binding domain bound with ACE2 (PDB id: 6VW1). Relevant side chains of the residues in the α-helix are shown in the orange box.
This protocol performs the substitution in each position of the WT molecule with the 19 other possible lateral chains, selecting, among the substitutions with compatible electrostatics, the ones driving the highest increase in shape complementarity. Using this approach, we can generate peptides with controlled compatibility with the partner and with a defined number of mutations with respect to the wild type. We decided to set the number of mutations to three to preserve the global features of the peptide. Hence, we selected the four mutated peptide sequences (HA1, HA2, HA3, and HA4) that exhibited the highest nominal affinities towards the RBD compared to the WT sequence. Moreover, inverting the function to optimize in the computational protocol, we selected the peptide sequence with the predicted lowest affinity (LA1) to work as a control. The sequences of each of the six peptides are detailed in Table 1, where the specific mutated amino acids are highlighted in red.
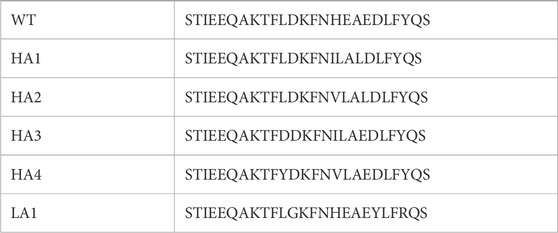
TABLE 1. Sequences of the wild type α-helix peptide and the list of mutated sequences HA1-4 and LA1. The mutated amino acids are highlighted in red.
To characterize the properties of these six peptide sequences, we performed molecular dynamics simulations. In particular, each of the 6 peptides has been extensively simulated in water as a monomer (1μs-long each). Indeed, it has to be noted that we performed the shape complementarity optimization using the elongated α-helix form of the peptide when inserted in the ACE2 structure. Therefore, the peptides’ behavior in water was assessed to ensure the applicability of our computational optimization protocol. Relevant results are summarized in Figure 2.
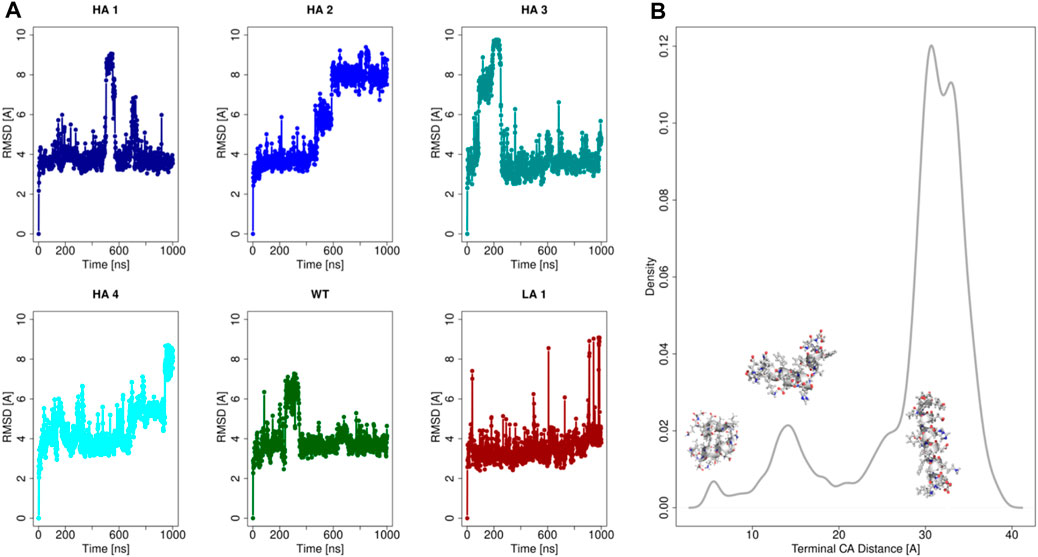
FIGURE 2. Analysis of the peptides molecular dynamics. (A) Root Mean Square Deviation (RMSD) as a function of time with respect to the initial configuration for each of the six peptides. (B) Density distribution of the spatial distance between the terminal α-carbon, for all of six peptides simulations.
In Panel (a) we reported the Root Mean Square Deviation (RMSD) of the simulations of all peptides. It emerges that all the mutants at the equilibrium can assume mainly three conformations in dynamics at room temperature, characterized by three equilibrium RMSD values. These three conformational states are characterized by three different levels of structure compactness, as demonstrated by the distribution reported in panel (b). Indeed, for each frame of all the molecular dynamics simulations, the distance between the peptides terminal C-alpha was calculated and its distribution is shown in Figure 2.
The simulations commence from the extended conformation, and the low RMSD values, approximately 4 Å, indicate frames where the peptide structure remains extended. Occasionally, albeit for brief periods, peptides may self-fold, reducing the distance between their terminal residues (refer to the distribution in Figure 2B). Consequently, high RMSD values, computed concerning the initial configuration of molecular dynamics, are observed in frames where the peptide undergoes further compaction. Three peaks are present in correspondence to the three main accessible configurations for the peptides. More importantly, the stretched configuration (the one with the highest value of terminal distance) is much more frequent, testifying its advantage in terms of free energy.
This result demonstrated that at equilibrium all peptides spend a significant amount of time in a stretched form, as previously observed (Baig et al., 2020). Therefore, the optimization performed using the elongated form of the peptide has in the ACE2 structure is meaningful and well-defined.
2.2 Spike-peptide complexes: experimental binding kinetics measurements and molecular dynamics simulation
Binding kinetics measurements were carried out by using the biolayer interferometry technique, in order to assess the affinity between the ACE2-derived peptides and RBD fragment in solution. This approach has been extensively employed in the investigation of antibody-antigen interactions (Concepcion et al., 2009; Kamat and Rafique, 2017; Petersen, 2017), with a specific emphasis on the interactions between SARS-CoV-2 variants and antibodies (Dzimianski et al., 2020; Ginex et al., 2022; Wang et al., 2022; Wang et al., 2023).
In the present study, we performed biotinylation of the WT, HA4, HA3, HA2, HA1, and LA1 peptides to enable their immobilization on the biosensor surface. Subsequently, we conducted kinetic measurements (as depicted in Figure 3) at various concentrations of RBD ranging from 100 μM to 0.16 µM. In Table 2 we presented the obtained KD values, representing the affinity between the peptides and RBD, as well as the shape complementarity balance predicted by the computational protocol.
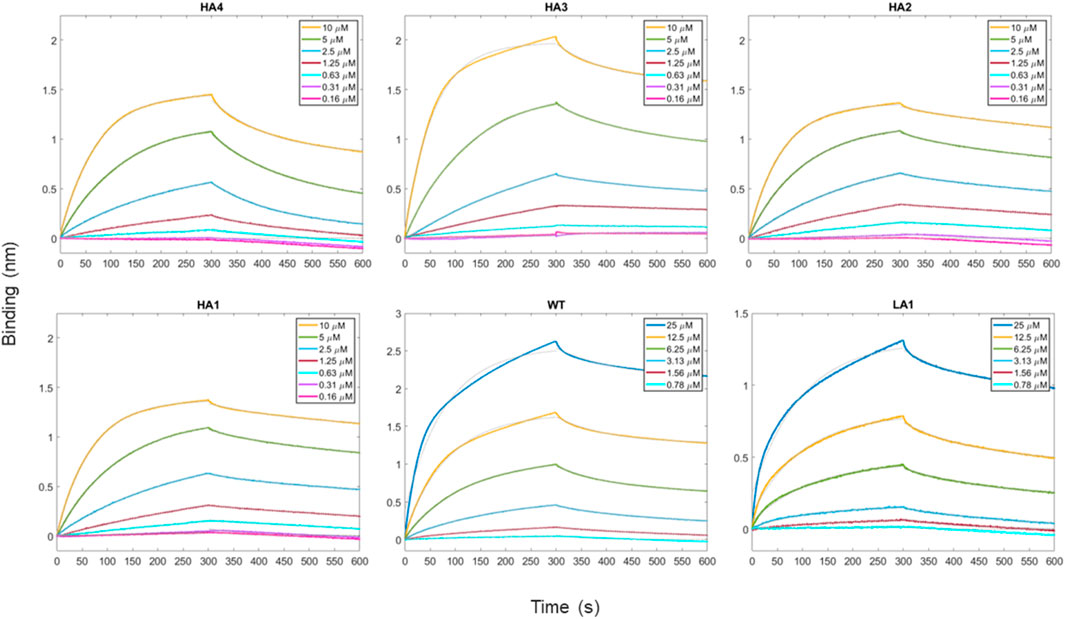
FIGURE 3. Time-course of the reaction between peptides and RBD as the analyte protein in solution at various concentrations. For all measurements, a 300 s time period was set for both association and dissociation steps. Peptides have been loaded first at fixed concentration (50 μg/mL) on biosensors initialized with streptavidin molecule.

TABLE 2. The values of KD with standard errors from data analysis of BLI assay traces are reported in the first row. KD were obtained directly within the Octet software. In the second row we report the complementarity balance, that is the increase in shape complementarity, in terms of distance between Zernike descriptors, with respect to the Wild Type.
The shape complementarity balance describes the increase in shape complementarity due to the residue substitutions with respect to the wild type, as calculated with the euclidean distance between the Zernike descriptors of the peptides and the Spike binding site. Notably, the affinity constants are in the micromolar range and exhibit the same trend as the one predicted via the computational approach.
Specifically, the HA4, HA3, HA2, and HA1 mutants displayed increased affinity towards RBD compared to the WT peptide. Conversely, LA1 exhibited significantly lower affinity.
In order to further understand the molecular mechanisms driving the peptide recognition process, we performed six 1μs-long molecular dynamics simulations of the SARS-CoV-2 Spike protein in complex with each of the peptides. The complexes were built by extracting the Spike-peptide complex from the experimental Spike-ACE2 complex and substituting the appropriate mutated residues within the peptide. The main results of the simulations are summarized in Figure 4 and can be discussed as follows.
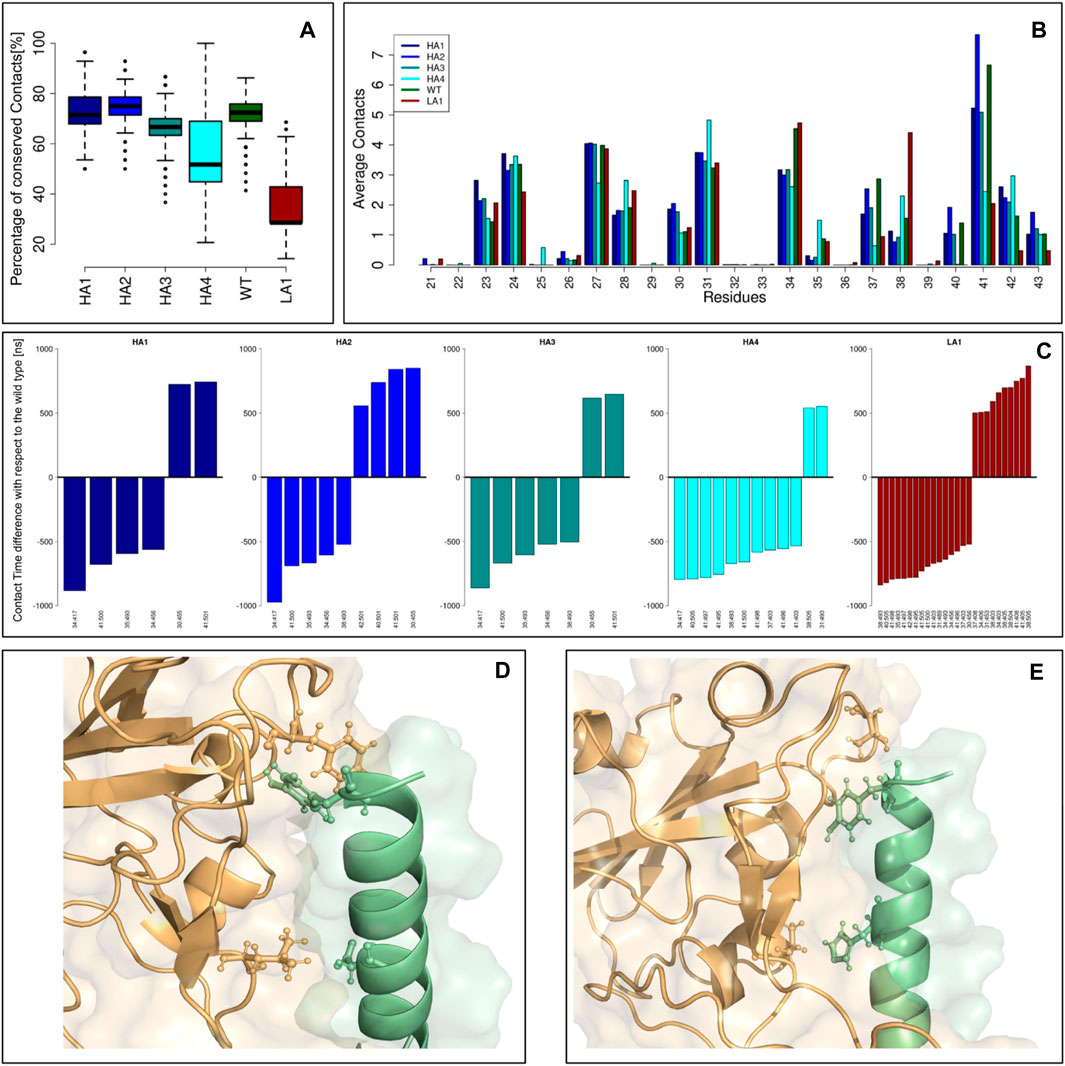
FIGURE 4. Peptide-spike complexes molecular dynamics. (A) Boxplots describing the percentage of conserved contacts at the equilibrium, with respect to those observed in the starting structure. (B) The average contacts each peptide residue made during the equilibrium simulation frames. Each color represents a specific mutant peptide-Spike complex. (C) Intermolecular links characterized by an occurrence difference higher than 50% with respect to the wild type. (D) Molecular representation of the Peptide-Spike complex with highlighted the 30:455 and 41:501 links, acquired by the high affinity mutants. (E) Molecular representation of the Peptide-Spike complex with highlighted the 34:417 and 41:500 links, lost by the high affinity mutants.
Defining a residue-residue intermolecular contact as a pair of Spike-Peptide residues with at least one of their atom-atom pairs closer than 4 A, we first studied the intermolecular contacts conserved along the dynamics, as an indicator of the stability of the molecular complex. In particular, in panel (a) we reported the boxplots describing the percentage of conserved contacts at the equilibrium, with respect to those observed in the starting structure. Notably, the low-affinity mutant displays a low number of conserved contacts as a consequence of its reduced complex stability, in full agreement with the results of the biochemical essays. Another proof of this behavior can be searched on the RMSD trend over time for each of the 6 peptides simulated in complex with the Spike protein (Supplementary Figure S1). All peptides with increased affinity and the wild-type peptide exhibit significant stability, evidenced by the consistent RMSD trend. Conversely, in the simulation of the peptide with reduced affinity, we observe a moment when the peptide undergoes a conformational change (RMSD peak) before readjusting to a new position. At that moment, the peptide shifts, losing many of its initial intermolecular contacts (as depicted in Figure 4A), confirming that the design has rendered it unsuitable for this situation.
We thus moved to the analysis of the peptide residues responsible for the interaction with Spike. The average number of intermolecular contacts of each residue, executed during the molecular dynamics, were calculated and shown in panel (b). Interestingly, some residues exhibited a different behavior depending on which mutant we were considering. A very high number of contacts are undertaken by residue 41 in both WT and HA mutants, whereas the LA mutant dramatically reduces the reactivity of such a residue. Conversely, residue 34 is characterized by a high number of contacts in WT and LA peptides, whereas in all the HA mutants this residue lessens the interaction with Spike.
Finally, in panel (c), we studied which intermolecular links differ the most between the WT and the mutant peptides. Indeed, for each residue-residue intermolecular pair, we calculated the occurrence in both WT and mutant simulation frames: we reported for each mutant peptide the barplots indicating the residue-residue links that highlight a difference with the Wild Type higher than 50%. In other words, each bar represents a contact with Spike that has been acquired or lost by a mutant with respect to the ones characterizing the Wild Type: these contacts can be responsible for the variation in binding affinity these mutants exhibit. We noted that some common features are present in the increased affinity mutants simulations. On one hand, the HA mutants acquired, with respect to the WT complex, the interactions 30:455 and 41:501 (represented in panel (d)), whereas they lost the interactions 34:417 and 41:500 (panel (e)). It has to be noted that among all the residues involved in these dramatic changes, only residue 34 undergoes a mutation (H34I or H34V): this result proves the capability of the design protocol to compactly summarize the overall interface features.
2.3 ACE2-derived peptides/ferritin chimeric nanoparticles
Based on the findings obtained from the individual peptides, we sought to demonstrate the feasibility of fusing these peptides with human ferritin H as a proof of concept. Indeed, the application of the peptides alone for future therapeutic purposes is limited due to challenges related to poor bioavailability and high clearance rates. To overcome these limitations, one strategy involves combining the peptides with nanoparticles of diverse compositions. Nanoparticles (NPs) have demonstrated their potential as conjugate scaffolds, enhancing peptide functionality and leveraging their intrinsic properties, often leading to synergistic effects (Jeong et al., 2018).
Protein-based nanoparticles are particularly suitable, given their biocompatibility, ease of producing monodisperse forms through recombinant techniques, and the ability to be modulated via genetic engineering approaches. Among these protein nanoparticles, ferritin serves as an ideal platform for diagnostic and therapeutic applications (Calisti et al., 2018; Palombarini et al., 2021; Affatigato et al., 2023). Comprised of 24 subunits, human ferritin forms a nanosphere with a diameter of 12 nm. Modifying a single subunit enables the functionalization of the entire nanoparticle (Incocciati et al., 2023).
In the case of ACE2-derived peptides, fusing the peptide sequence to the N-terminus of human H ferritin offers the advantage of generating a chimeric protein that presents 24 peptides on its surface. This arrangement leads to a multivalent effect while concurrently reducing peptide clearance.
In our specific case, we opted to genetically fuse both the WT sequence and the HA1 sequence, which exhibits the highest affinity for RBD, to the N-terminus of human H ferritin. To ensure structural integrity, a flexible spacer consisting of four glycine residues was included in the fusion construct. WT-HFt and HA1-HFt constructs were overexpressed in Escherichia coli. Interestingly, the WT-HFt was successfully expressed in a soluble form, while, HA1-HFt was obtained in inclusion bodies, necessitating additional steps for purification. Nevertheless, both proteins were purified to a high degree of purity (Supplementary Figure S3), enabling subsequent structural and functional characterization.
SDS-PAGE analysis demonstrates that the individual subunits of each chimeric ferritin exhibit the expected increase in molecular weight due to the addition of the peptide. Moreover, High-performance size exclusion chromatography (HP-SEC) analysis and native gel electrophoresis confirm the correct assembly of these ferritins into their 24mer form (Incocciati et al., 2022). The HPLC elution profile reveals a higher molecular weight for the chimeric ferritins compared to human H ferritin. As shown in Figure 5A, the WT-HFt variant displays a weight increase of 77,266 Da (retention time 3.53 min) while the HA1-HFt shows an increase of 75,924 Da (retention time 3.67 min).
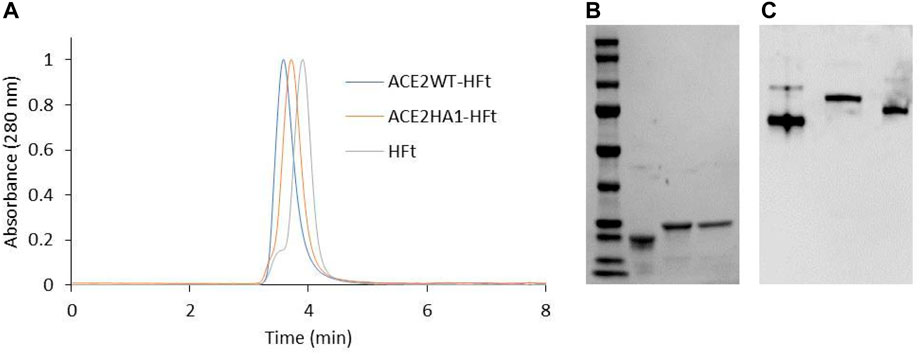
FIGURE 5. Biochemical characterization of ACE2WT-HFt and ACE2HA1-HFt compared to HFt. (A) HP-SEC analysis. (B) SDS-PAGE (lane 1: marker, lane 2: HFt, lane3: ACE2WT-HFt, lane 4: ACE2HA1-HFt). (C) native gel electrophoresis (lane1: HFt, lane2: ACE2WT-HFt, lane 3: ACE2HA1-HFt).
Similarly, the native gel electrophoresis demonstrates that the chimeric variants migrate slower than ferritin alone (Figures 5B, C), as expected. The disparities in electrophoretic mobility between the two mutants can be attributed to their differing masses and charges. After characterization, the binding capacity of the two chimeric ferritins to the Spike RBD was assessed. The analysis was performed using the BLI technique, wherein His-tagged RBD was immobilized on the Ni-NTA biosensor, and the concentrations of the chimeric ferritins were systematically varied. Subsequently, the binding kinetics were examined, and the results are illustrated in Figure 6. The analysis of the kinetic plots revealed a dissociation constant (KD) of 10.81 nM for WT-HFt and 8.32 nM for HA1-HFt, providing quantitative insights into the affinity of the respective constructs for RBD. A summary of these results can be find in Table 3.
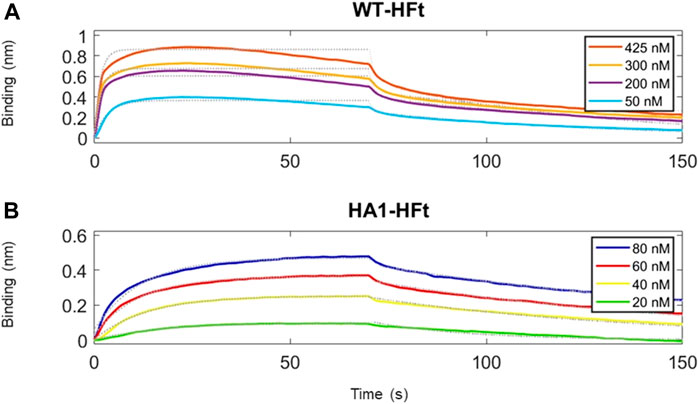
FIGURE 6. Time courses of the reaction between RBD and ferritin constructs. RBD protein has been loaded first at fixed concentration (50 μg/mL) on Ni-NTA biosensors. (A) ferritin with WT peptide construct is the analyte protein in solution at various concentrations. We set a 70 s time interval for the association step and 80 s for dissociation. (B) ferritin with HA1 peptide construct is the analyte protein in solution at various concentrations. Association and dissociation steps have duration respectively of 70 s and 80 s. Temperature was 25 °C.
3 Conclusion
The key output of the present article is related to the in silico testing and in vitro experimental validation of a method for the design of polypeptide mutants with optimized binding capabilities to their target protein. Such achievement paves the way for a wide range of possible applications in modulating the binding affinity of selected protein-protein interactions by rational prediction of site-selected mutants using a simple algorithm based on Zernike polynomials descriptors. Computational studies and in vitro experiments have been focused on the interaction between the SARS-CoV-2 Spike protein and its main cellular receptor, ACE2 as this interaction stands as a prime and extensively studied example of protein-protein binding and has been instrumental in understanding the mechanisms behind viral cell invasion at molecular level. Leveraging the compatibility of the Spike-ACE2 interface, we explored the use of soluble ACE2 fragments as potential inhibitors of such a paramount example of protein-protein interaction.
In this study, we selected a peptide representing ACE2’s major interaction region and aimed to enhance its affinity with the Spike receptor-binding domain (RDB) for more effective inhibition. Thus far, we used a computational mutagenesis protocol that assessed shape complementarity and electrostatic interactions at the peptide-protein interface.
In other preceding works, we have demonstrated the significant role that shapes complementarity and electrostatic compatibility play at the interface, highlighting how the Zernike formalism is refined enough to capture this aspect (Di Rienzo et al., 2021a; De Lauro et al., 2022; Desantis et al., 2022; Grassmann et al., 2023). Undoubtedly, other physicochemical features are crucial for a comprehensive characterization of the compatibility between two protein interfaces. In our plans, we intend to incorporate these aspects into the algorithm cost function to account for them. However, these two characteristics successfully describe at least a substantial portion of this phenomenon, as evidenced by the success of our design protocol. In fact, not only are we able to achieve peptides with improved binding affinity, but we also obtain a negative control with worsened binding affinity due to compromised electrostatic compatibility and deteriorated shape complementarity. Indeed, our results identified peptide mutants with predicted changes in binding affinity as compared to the wild type, and these findings were confirmed in a set of in vitro experiments. Most significantly, the observed results mark the first successful in vitro experimental validation of our protein design algorithm.
In order to complete the picture, wild-type and optimized peptides were fused to the N-terminus of human H ferritin 24-mer, a most popular protein carrier that was recently demonstrated to be a preferred multivalent epitope displaying agent in the construction of protein-based nanoparticles for efficient vaccine constructs assembly. Further in vivo studies will be necessary in order to assess the reduced clearance of the present construct with respect to isolated peptides upon administration in appropriate experimental animal systems. In this context, the multivalency effect of peptide-fused ferritin 24-mer was also investigated in its binding to immobilized RBD protein using biolayer interferometry measurements. Experimental data demonstrated a 250-fold increase in the binding affinity of the peptide-HFt constructs with respect to the isolated peptides in biolayer interferometry experiments.
In conclusion, the body of experimental results provides the first strong experimental evidence for the effectiveness of the reported computational design protocol. The method thus proved to be highly adaptable and very efficient, thus setting the stage for a comprehensive approach to modulate a wide range of protein-protein interactions.
4 Materials and methods
4.1 Peptides and chimeric ferritin production
The wild-type RBD of SARS-CoV-2 spike protein was provided by GenScript. The protein is a C-term HIS-tagged recombinant protein with a predicted molecular weight of 30 kD. The storage buffer was phosphate-buffered saline at pH 7.2, and the calculated pI was 8.91; the stock concentration was 0.89 mg/mL according to the manufacturer.
ACE2-derived peptides WT, HA1, HA2, HA3, HA4, LA1 were synthesized by GenScript and provided in the lyophilized form. Before use, they were resuspended in PBS buffer containing either 3% NH4OH or 5% NH3 (depending on the total net charge of the peptides). The final stock concentration for each peptide was 5 mg/mL.
The synthetic genes encoding for HA1-HFt and WT-HFt chimeric proteins were synthesized by GenScript and optimized for expression in Escherichia coli cells. Peptide sequences were inserted at the N-terminal region of the ferritin through a 4 glycine-spacer. The genes were then subcloned into pET22 b vector e transformed in BL21 (DE3) competent cells.
HFt was expressed and purified as previously reported (Palombarini et al., 2019).
WT-HFt purification protocol: Bacterial paste from 1 L culture was resuspended in 50 mL of 20 mM sodium phosphate buffer, pH 7.4 containing 150 mM NaCl and protease inhibitors (Roche©) and disrupted by sonication. The soluble fraction was precipitated with 20% (NH4)2SO4 for 1 h at 4°C. The pellet was resuspended in 20 mM sodium phosphate buffer pH 7.4 containing 150 mM NaCl and extensively dialyzed versus the same buffer overnight at 4 °C. Finally, the protein was loaded onto a HiLoad 26/600 Superdex 200 pg column equilibrated with 20 mM sodium phosphate buffer pH 7.4 containing 150 mM NaCl, using an AKTA-Pure apparatus (Cytiva).
HA1-HFt purification protocol: Bacterial paste from 1 L culture was resuspended in 50 mL of 50 mM Tris/glycine buffer, pH 7.5 containing 150 mM NaCl, 0.5 mM TCEP and protease inhibitors (Roche©) and disrupted by sonication. The insoluble fraction was resuspended in 50 mL of 100 mM Tris/glycine buffer pH 8.5, containing 0.5 mM TCEP and 0.5 M urea. The solution was stirred for 30 min at room temperature. The resulting washed pellet was resuspended in 50 mL of 100 mM Tris/glycine buffer pH 8.5, containing 0.5 mM TCEP and 4 M urea, and stirred for 30 min at room temperature. After centrifugation, the soluble fraction was dialyzed overnight versus 50 mM Tris/glycine buffer pH 9 at 4°C. The protein sample was then dialyzed for 4 h versus sodium phosphate buffer pH 7.4, concentrated, and loaded onto a HiLoad 26/600 Superdex 200 pg as described above.
The protein fractions eluting at the retention time of ferritin were pooled, concentrated using AmiconUltra-15 centrifugal filter devices (100 kDa cut-off), sterile filtered, and stored at 4 °C. Protein concentration was calculated by measuring the UV absorption at 280 nm (ε280 = 19,000 M−1 cm−1 and 20,400 M−1 cm−1 for HFt and the chimeric proteins, respectively). Protein purity was checked by SDS-PAGE and the correct quaternary assembly was evaluated by high-performance size exclusion chromatography (HP-SEC).
High-performance size exclusion chromatography. The purity and aggregation state of protein samples were analyzed by HP-SEC. HP-SEC analyses were performed by means of an Agilent Infinity 1260 HPLC apparatus equipped with UV detectors using an Agilent AdvanceBio SEC 300 A 2.7 um 4.6 × 150 mm column. Isocratic analysis was carried out with 20 mM sodium phosphate buffer pH 7.4 as the mobile phase. The flow rate was 0.7 mL/min over an elution window of 10 min. Ferritin elution was followed using UV detection at 220 nm and 280 nm.
4.2 Biolayer interferometry
BLI assays were performed using the Octet N2 system (Sartorius). The interaction between ACE2-derived peptides (WT, HA1, HA2, HA3, HA4, LA1), and RBD was conducted by immobilizing the biotinylated peptides on High Precision Streptavidin 2.0 (SAX2) biosensors, with varying concentrations of RBD in the range of 0.16 µM–100 µM. The peptides were either subjected to a biotinylation reaction using the BTAG biotinylation kit (Sigma-Aldrich) or were provided pre-biotinylated by the manufacturer (GenScript). The interaction between the chimeric proteins WT-HFt (in the range of 425 nM–50 nM) or HA1-HFt (in the range of 80 nM–20 nM) and RBD was performed by immobilizing the His-tagged RBD on nickel nitriloacetic acid (Ni-NTA) biosensors.
The biosensors were first equilibrated for 10 min in 1X kinetic buffer (Sartorius), which consisted of PBS with 0.02% Tween20, 0.1% BSA, and 0.05% NaN3. Subsequently, depending on the assay, either ACE2-derived peptide or RBD was loaded onto the corresponding biosensor at a concentration of 50 μg/mL for an appropriate time interval, as indicated in the Sartorius biosensors’ datasheets. The duration of each experimental step was optimized in order to achieve maximum binding capacity in each experiment. When available, the concentration range for each associating protein was chosen based on KD values obtained from the literature or experimentally determined for different scenarios where KD values were unknown.
The recorded data were analyzed using the Octet software to extrapolate the kinetic parameters. All association and dissociation curves were fitted using a single exponential function. Pseudo-first order (PFO) conditions were met when the initial concentration of one of the two reagents was significantly higher (between 50- and 100-fold) than the other (Malatesta, 2005; Kumaraswamy and Tobias, 2015; Müller-Esparza et al., 2020).
4.3 Protein surface construction
As a starting point, we used the experimental RBD-Spike complex structure labeled with the PDB code 6vw1 (Shang et al., 2020): it was solved with x-ray crystallography with a resolution of 2.68 A. The structure report ACE2 residues from Serine 19 to Alanine 614 and RBD residues from Asparagine 334 to Proline 527. Starting from the wild type ACE2 sequence, we modeled all the possible point mutations in range 21–43 using Scwrl4 (Krivov et al., 2009). Atomic charges and radii were assigned using PDB2PQR (Dolinsky et al., 2004). Solvent Accessible Surface and are computed using dms software (Richards, 1977).
4.4 Computational protocol for peptide design
We applied here the protocol design we developed in a previous publication (Di Rienzo et al., 2021b), restraining the set of mutable residues to the range 21–43.
The main step of the protocol are:
- Starting from the experimental structure, we extracted the surface generated by residue 21–43 from the whole surface and we calculated its 2D Zernike descriptors (20th order, 121 invariant descriptors).
- We calculated the 2D Zernike descriptors regarding the Spike binding site, defined as the set of Spike residues closer than 5 A to any ACE2 atoms.
- We measured the shape complementarity as the Euclidean distance between these two sets of descriptors.
- To assess Coulomb contributions, we developed a Coarse Grained atomic representation. We assigned atomic partial charges and we represented each residue with a bead summarizing the main chain and a bead summarizing the side chain. For each residue they are located on the geometrical center and are charged with the sum of the partial charges of the corresponding atoms. The electrostatic compatibility is computed with a standard Coulomb potential.
The design algorithm works as follows:
- From the wild type ACE2 sequence, we modeled all the possible point mutations in residue range 21–43.
- We accepted only substitutions with non-unfavourable electrostatics modifications. In other words, we accepted only mutations that do not cause an increase in electrostatic energy at the interface.
- We identified among them the best two mutations in terms of shape complementarity increase, as calculated in terms of Zernike descriptors.
- Settled these substitutions, we repeated the computational mutagenesis on all the remaining binding site residues, therefore defining four double site mutants.
- Repeating three times this procedure, we identified eight possible ACE2 mutants characterized by increased shape complementarity and compatible electrostatics.
Analogously, we perform the same procedure aiming in decreasing the shape complementarity. We thus obtained eight mutants with decreased shape complementarity. Finally we selected the best four mutants and the worst mutant in terms of increase and decrease of complementarity, respectively, to be tested experimentally.
4.5 Molecular dynamics simulation
We used Gromacs 2021 to run all the molecular dynamics simulations (Abraham et al., 2015). Topologies of the system were built using the CHARMM-27 force field (Brooks et al., 2009). Each molecular system was firstly minimized with the steepest descent algorithm. Thus, we performed the thermalization of the system running sequentially in a NVT and NPT environments 2 0.1ns-long simulations at 2fs time-step. The temperature is set at 300 K with v-rescale thermostat (Bussi et al., 2007) and the pressure (1 bar) is kept constant using Parrinello-Rahman barostat (Parrinello and Rahman, 1980). A cut-off of 1.2 nm was imposed for the evaluation of short-range non-bonded interactions and the Particle Mesh Ewald method (Cheatham et al., 1995) for the long-range electrostatic interactions.
Data availability statement
The raw data supporting the conclusion of this article will be made available by the authors, without undue reservation.
Author contributions
GP: Conceptualization, Data curation, Investigation, Methodology, Writing–original draft, Writing–review and editing. RP: Conceptualization, Data curation, Investigation, Methodology, Writing–original draft, Writing–review and editing. AI: Data curation, Methodology, Writing–original draft. ABon: Data curation, Methodology, Writing–original draft, Writing–review and editing. AM: Data curation, Methodology, Writing–original draft. JR: Methodology, Writing–original draft. EZ: Methodology, Writing–original draft. MM: Methodology, Software, Writing–original draft. EM: Methodology, Software, Writing–original draft. GT: Conceptualization, Methodology, Writing–original draft. GR: Conceptualization, Funding acquisition, Supervision, Writing–original draft. ABof: Conceptualization, Data curation, Methodology, Project administration, Supervision, Writing–original draft, Writing–review and editing. LD: Conceptualization, Investigation, Methodology, Project administration, Software, Supervision, Writing–original draft, Writing–review and editing.
Funding
The author(s) declare financial support was received for the research, authorship, and/or publication of this article. This research was partially funded by grants from ERC-2019-Synergy Grant (ASTRA, no. 855923); EIC-2022-PathfinderOpen (ivBM-4PAP, no. 101098989); Project “National Center for Gene Therapy and Drugs based on RNA Technology” (CN00000041) financed by Next-Generation EU PNRR MUR—M4C2—Action 1.4—Call “Potenziamento strutture di ricerca e creazione di “campioni nazionali di R&S” (CUP J33C22001130001). Moreover, this research was partially funded by PAN-HUB project no T4-AN-07, “Traiettoria 4 del Piano Sviluppo e Coesione Salute”—FSC 2014-2020, to ABof.
Conflict of interest
The authors declare that the research was conducted in the absence of any commercial or financial relationships that could be construed as a potential conflict of interest.
The author(s) declared that they were an editorial board member of Frontiers, at the time of submission. This had no impact on the peer review process and the final decision.
Publisher’s note
All claims expressed in this article are solely those of the authors and do not necessarily represent those of their affiliated organizations, or those of the publisher, the editors and the reviewers. Any product that may be evaluated in this article, or claim that may be made by its manufacturer, is not guaranteed or endorsed by the publisher.
Supplementary material
The Supplementary Material for this article can be found online at: https://www.frontiersin.org/articles/10.3389/fmolb.2023.1332359/full#supplementary-material
References
Abraham, M. J., Murtola, T., Schulz, R., Páll, S., Smith, J. C., Hess, B., et al. (2015). GROMACS: high performance molecular simulations through multi-level parallelism from laptops to supercomputers. SoftwareX 1–2, 19–25. doi:10.1016/j.softx.2015.06.001
Affatigato, L., Licciardi, M., Bonamore, A., Martorana, A., Incocciati, A., Boffi, A., et al. (2023). Ferritin-coated SPIONs as new cancer cell targeted magnetic nanocarrier. Molecules 28 (3), 1163. PMID: 36770830; PMCID: PMC9919024. doi:10.3390/molecules28031163
Bai, C., Wang, J., Chen, G., Zhang, H., An, K., Xu, P., et al. (2021). Predicting mutational effects on receptor binding of the spike protein of SARS-CoV-2 variants. J. Am. Chem. Soc. 143 (42), 17646–17654. doi:10.1021/jacs.1c07965
Baig, M. S., Alagumuthu, M., Rajpoot, S., and Saqib, U. (2020). Identification of a potential peptide inhibitor of SARS-CoV-2 targeting its entry into the host cells. Drugs R&D 20 (3), 161–169. doi:10.1007/s40268-020-00312-5
Barton, M. I., MacGowan, S. A., Kutuzov, M. A., Dushek, O., Barton, G. J., and van der Merwe, P. A. (2021). Effects of common mutations in the SARS-CoV-2 Spike RBD and its ligand, the human ACE2 receptor on binding affinity and kinetics. ELife 10, e70658. doi:10.7554/eLife.70658
Brender, J. R., and Zhang, Y. (2015). Predicting the effect of mutations on protein-protein binding interactions through structure-based interface profiles. PLOS Comput. Biol. 11 (10), e1004494. doi:10.1371/journal.pcbi.1004494
Brooks, B. R., Brooks, C. L., Mackerell, A. D., Nilsson, L., Petrella, R. J., Roux, B., et al. (2009). CHARMM: the biomolecular simulation program. J. Comput. Chem. 30 (10), 1545–1614. doi:10.1002/jcc.21287
Bussi, G., Donadio, D., and Parrinello, M. (2007). Canonical sampling through velocity rescaling. J. Chem. Phys. 126 (1), 014101. doi:10.1063/1.2408420
Calisti, L., Trabuco, M. C., Boffi, A., Testi, C., Montemiglio, L. C., des Georges, A., et al. (2018). Engineered ferritin for lanthanide binding. PLoS One 13 (8), e0201859. doi:10.1371/journal.pone.0201859
Cheatham, T. I., Miller, J. L., Fox, T., Darden, T. A., and Kollman, P. A. (1995). Molecular dynamics simulations on solvated biomolecular systems: the particle mesh Ewald method leads to stable trajectories of DNA, RNA, and proteins. J. Am. Chem. Soc. 117 (14), 4193–4194. doi:10.1021/ja00119a045
Concepcion, J., Witte, K., Wartchow, C., Choo, S., Yao, D., Persson, H., et al. (2009). Label-free detection of biomolecular interactions using BioLayer interferometry for kinetic characterization. Comb. Chem. High Throughput Screen. 12 (8), 791–800. doi:10.2174/138620709789104915
Daberdaku, S., and Ferrari, C. (2018). Exploring the potential of 3D Zernike descriptors and SVM for protein–protein interface prediction. BMC Bioinforma. 19 (1), 35. doi:10.1186/s12859-018-2043-3
Daberdaku, S., and Ferrari, C. (2019). Antibody interface prediction with 3D Zernike descriptors and SVM. Bioinformatics 35 (11), 1870–1876. doi:10.1093/bioinformatics/bty918
de Campos, L. J., Palermo, N. Y., and Conda-Sheridan, M. (2021). Targeting SARS-CoV-2 receptor binding domain with stapled peptides: an in silico study. J. Phys. Chem. B 125 (24), 6572–6586. doi:10.1021/acs.jpcb.1c02398
De Lauro, A., Di Rienzo, L., Miotto, M., Olimpieri, P. P., Milanetti, E., and Ruocco, G. (2022). Shape complementarity optimization of antibody–antigen interfaces: the application to SARS-CoV-2 spike protein. Front. Mol. Biosci. 9, 874296. doi:10.3389/fmolb.2022.874296
Desantis, F., Miotto, M., Di Rienzo, L., Milanetti, E., and Ruocco, G. (2022). Spatial organization of hydrophobic and charged residues affects protein thermal stability and binding affinity. Sci. Rep. 12 (1), 12087. doi:10.1038/s41598-022-16338-5
Di Rienzo, L., De Flaviis, L., Ruocco, G., Folli, V., and Milanetti, E. (2022). Binding site identification of G protein-coupled receptors through a 3D Zernike polynomials-based method: application to C. elegans olfactory receptors. J. Computer-Aided Mol. Des. 36 (1), 11–24. doi:10.1007/s10822-021-00434-1
Di Rienzo, L., Milanetti, E., Ruocco, G., and Lepore, R. (2021b). Quantitative description of surface complementarity of antibody-antigen interfaces. Front. Mol. Biosci. 8, 749784. doi:10.3389/fmolb.2021.749784
Di Rienzo, L., Milanetti, E., Testi, C., Montemiglio, L. C., Baiocco, P., Boffi, A., et al. (2020). A novel strategy for molecular interfaces optimization: the case of Ferritin-Transferrin receptor interaction. Comput. Struct. Biotechnol. J. 18, 2678–2686. doi:10.1016/j.csbj.2020.09.020
Di Rienzo, L., Miotto, M., Desantis, F., Grassmann, G., Ruocco, G., and Milanetti, E. (2023a). Dynamical changes of SARS-CoV-2 spike variants in the highly immunogenic regions impact the viral antibodies escaping. Proteins Struct. Funct. Bioinforma. 91, 1116–1129. doi:10.1002/prot.26497
Di Rienzo, L., Miotto, M., Milanetti, E., and Ruocco, G. (2023b). Computational structural-based GPCR optimization for user-defined ligand: implications for the development of biosensors. Comput. Struct. Biotechnol. J. 21, 3002–3009. doi:10.1016/j.csbj.2023.05.004
Di Rienzo, L., Monti, M., Milanetti, E., Miotto, M., Boffi, A., Tartaglia, G. G., et al. (2021a). Computational optimization of angiotensin-converting enzyme 2 for SARS-CoV-2 Spike molecular recognition. Comput. Struct. Biotechnol. J. 19, 3006–3014. doi:10.1016/j.csbj.2021.05.016
Dolinsky, T. J., Nielsen, J. E., McCammon, J. A., and Baker, N. A. (2004). PDB2PQR: an automated pipeline for the setup of Poisson-Boltzmann electrostatics calculations. Nucleic acids Res. 32, W665–W667. doi:10.1093/nar/gkh381
Dzimianski, J. V., Lorig-Roach, N., O’Rourke, S. M., Alexander, D. L., Kimmey, J. M., and DuBois, R. M. (2020). Rapid and sensitive detection of SARS-CoV-2 antibodies by biolayer interferometry. Sci. Rep. 10 (1), 21738. doi:10.1038/s41598-020-78895-x
Forbes, S. A., Beare, D., Gunasekaran, P., Leung, K., Bindal, N., Boutselakis, H., et al. (2015). COSMIC: exploring the world’s knowledge of somatic mutations in human cancer. Nucleic Acids Res. 43 (D1), D805–D811. doi:10.1093/nar/gku1075
Geng, C., Xue, L. C., Roel-Touris, J., and Bonvin, A. M. J. J. (2019). Finding the ΔΔ G spot: are predictors of binding affinity changes upon mutations in protein–protein interactions ready for it? WIREs Comput. Mol. Sci. 9 (5). doi:10.1002/wcms.1410
Gheblawi, M., Wang, K., Viveiros, A., Nguyen, Q., Zhong, J.-C., Turner, A. J., et al. (2020). Angiotensin-converting enzyme 2: SARS-CoV-2 receptor and regulator of the renin-angiotensin system: celebrating the 20th anniversary of the discovery of ACE2. Circulation Res. 126 (10), 1456–1474. doi:10.1161/CIRCRESAHA.120.317015
Ginex, T., Marco-Marín, C., Wieczór, M., Mata, C. P., Krieger, J., Ruiz-Rodriguez, P., et al. (2022). The structural role of SARS-CoV-2 genetic background in the emergence and success of spike mutations: the case of the spike A222V mutation. PLOS Pathog. 18 (7), e1010631. doi:10.1371/journal.ppat.1010631
Grassmann, G., Di Rienzo, L., Gosti, G., Leonetti, M., Ruocco, G., Miotto, M., et al. (2023). Electrostatic complementarity at the interface drives transient protein-protein interactions. Sci. Rep. 13 (1), 10207. doi:10.1038/s41598-023-37130-z
Incocciati, A., Bertuccini, L., Boffi, A., Macone, A., and Bonamore, A. (2022). Unlocking the treasure box: the role of HEPES buffer in disassembling an uncommon ferritin nanoparticle. Separations 9, 222. doi:10.3390/separations9080222
Incocciati, A., Kubeš, J., Piacentini, R., Cappelletti, C., Botta, S., Bertuccini, L., et al. (2023). Hydrophobicity-Enhanced ferritin nanoparticles for efficient encapsulation and targeted delivery of hydrophobic drugs to tumor cells. Protein Sci. 32, e4819. doi:10.1002/pro.4819
Jeong, W., Bu, J., Kubiatowicz, L. J., Chen, S. S., Kim, Y., and Hong, S. (2018). Peptide–nanoparticle conjugates: a next generation of diagnostic and therapeutic platforms? Nano Converg. 5 (1), 38. doi:10.1186/s40580-018-0170-1
Kalathiya, U., Padariya, M., Fahraeus, R., Chakraborti, S., and Hupp, T. R. (2021). Multivalent display of SARS-CoV-2 spike (RBD domain) of COVID-19 to nanomaterial, protein ferritin nanocages. Biomolecules 11 (2), 297. doi:10.3390/biom11020297
Kamat, V., and Rafique, A. (2017). Designing binding kinetic assay on the bio-layer interferometry (BLI) biosensor to characterize antibody-antigen interactions. Anal. Biochem. 536, 16–31. doi:10.1016/j.ab.2017.08.002
Kanekiyo, M., Wei, C. J., Yassine, H. M., McTamney, P. M., Boyington, J. C., Whittle, J. R., et al. (2013). Self-assembling influenza nanoparticle vaccines elicit broadly neutralizing H1N1 antibodies. Nature 499 (7456), 102–106. doi:10.1038/nature12202
Khoshnejad, M., Parhiz, H., Shuvaev, V. V., Dmochowski, I. J., and Muzykantov, V. R. (2018). Ferritin-based drug delivery systems: hybrid nanocarriers for vascular immunotargeting. J. Control. Release 282, 13–24. doi:10.1016/j.jconrel.2018.02.042
Kim, J., Heu, W., Jeong, S., and Kim, H.-S. (2017). Genetically functionalized ferritin nanoparticles with a high-affinity protein binder for immunoassay and imaging. Anal. Chim. Acta 988, 81–88. doi:10.1016/j.aca.2017.07.060
Kim, S. A., Kim, S., Kim, G. B., Goo, J., Kim, N., Lee, Y., et al. (2022). A multivalent vaccine based on ferritin nanocage elicits potent protective immune responses against SARS-CoV-2 mutations. Int. J. Mol. Sci. 23 (11), 6123. doi:10.3390/ijms23116123
Krivov, G. G., Shapovalov, M. V., and Dunbrack, R. L. (2009). Improved prediction of protein side-chain conformations with SCWRL4. Proteins 77 (4), 778–795. doi:10.1002/prot.22488
Kumaraswamy, S., and Tobias, R. (2015). Label-free kinetic analysis of an antibody–antigen interaction using biolayer interferometry. Methods Mol. Biol. 1278, 165–182. doi:10.1007/978-1-4939-2425-7_10
Kuznetsov, A., Arukuusk, P., Härk, H., Juronen, E., Ustav, M., Langel, Ü., et al. (2022). ACE2 peptide fragment interaction with different S1 protein sites. Int. J. peptide Res. Ther. 28, 7. doi:10.1007/s10989-021-10324-7
Landrum, M. J., Lee, J. M., Benson, M., Brown, G., Chao, C., Chitipiralla, S., et al. (2016). ClinVar: public archive of interpretations of clinically relevant variants. Nucleic Acids Res. 44 (D1), D862–D868. doi:10.1093/nar/gkv1222
Larue, R. C., Xing, E., Kenney, A. D., Zhang, Y., Tuazon, J. A., Li, J., et al. (2021). Rationally designed ACE2-derived peptides inhibit SARS-CoV-2. Bioconjugate Chem. 32 (1), 215–223. doi:10.1021/acs.bioconjchem.0c00664
Malatesta, F. (2005). The study of bimolecular reactions under non-pseudo-first order conditions. Biophys. Chem. 116 (3), 251–256. doi:10.1016/j.bpc.2005.04.006
Milanetti, E., Miotto, M., Di Rienzo, L., Monti, M., Gosti, G., and Ruocco, G. (2021). 2D Zernike polynomial expansion: finding the protein-protein binding regions. Comput. Struct. Biotechnol. J. 19, 29–36. doi:10.1016/j.csbj.2020.11.051
Miotto, M., Di Rienzo, L., Bò, L., Boffi, A., Ruocco, G., and Milanetti, E. (2021). Molecular mechanisms behind anti SARS-CoV-2 action of lactoferrin. Front. Mol. Biosci. 8, 607443. doi:10.3389/fmolb.2021.607443
Miotto, M., Di Rienzo, L., Gosti, G., Bo’, L., Parisi, G., Piacentini, R., et al. (2022). Inferring the stabilization effects of SARS-CoV-2 variants on the binding with ACE2 receptor. Commun. Biol. 5 (1), 20221. doi:10.1038/s42003-021-02946-w
Miotto, M., Di Rienzo, L., Grassmann, G., Desantis, F., Cidonio, G., Gosti, G., et al. (2023). Differences in the organization of interface residues tunes the stability of the SARS-CoV-2 spike-ACE2 complex. Front. Mol. Biosci. 10, 1205919. doi:10.3389/fmolb.2023.1205919
Moretti, R., Fleishman, S. J., Agius, R., Torchala, M., Bates, P. A., Kastritis, P. L., et al. (2013). Community-wide evaluation of methods for predicting the effect of mutations on protein-protein interactions. Proteins Struct. Funct. Bioinforma. 81 (11), 1980–1987. doi:10.1002/prot.24356
Müller-Esparza, H., Osorio-Valeriano, M., Steube, N., Thanbichler, M., and Randau, L. (2020). Bio-Layer interferometry analysis of the target binding activity of CRISPR-cas effector complexes. Front. Mol. Biosci. 7, 98. doi:10.3389/fmolb.2020.00098
Ochoa, R., Soler, M. A., Laio, A., and Cossio, P. (2021). PARCE: protocol for amino acid refinement through computational evolution. Comput. Phys. Commun. 260, 107716. doi:10.1016/j.cpc.2020.107716
Palombarini, F., Di Fabio, E., Boffi, A., Macone, A., and Bonamore, A. (2020). Ferritin nanocages for protein delivery to tumor cells. Molecules 25 (4), 825. PMID: 32070033; PMCID: PMC7070480. doi:10.3390/molecules25040825
Palombarini, F., Ghirga, F., Boffi, A., Macone, A., and Bonamore, A. (2019). Application of crossflow ultrafiltration for scaling up the purification of a recombinant ferritin. Protein Expr. Purif. 163, 105451. Epub 2019 Jul 10. PMID: 31301427. doi:10.1016/j.pep.2019.105451
Palombarini, F., Masciarelli, S., Incocciati, A., Liccardo, F., Di Fabio, E., Iazzetti, A., et al. (2021). Self-assembling ferritin-dendrimer nanoparticles for targeted delivery of nucleic acids to myeloid leukemia cells. J. Nanobiotechnol 19, 172. doi:10.1186/s12951-021-00921-5
Papageorgiou, A. C., and Mohsin, I. (2020). The SARS-CoV-2 spike glycoprotein as a drug and vaccine target: structural insights into its complexes with ACE2 and antibodies. Cells 9 (11), 2343. doi:10.3390/cells9112343
Parrinello, M., and Rahman, A. (1980). Crystal structure and pair potentials: a molecular-dynamics study. Phys. Rev. Lett. 45 (14), 1196–1199. doi:10.1103/PhysRevLett.45.1196
Petersen, R. (2017). Strategies using bio-layer interferometry biosensor Technology for vaccine research and development. Biosensors 7 (4), 49. doi:10.3390/bios7040049
Piacentini, R., Centi, L., Miotto, M., Milanetti, E., Di Rienzo, L., Pitea, M., et al. (2022). Lactoferrin inhibition of the complex formation between ACE2 receptor and SARS CoV-2 recognition binding domain. Int. J. Mol. Sci. 23 (10), 5436. doi:10.3390/ijms23105436
Powell, A. E., Zhang, K., Sanyal, M., Tang, S., Weidenbacher, P. A., Li, S., et al. (2021). A single immunization with spike-functionalized ferritin vaccines elicits neutralizing antibody responses against SARS-CoV-2 in mice. ACS central Sci. 7 (1), 183–199. doi:10.1021/acscentsci.0c01405
Richards, F. M. (1977). Areas, volumes, packing and protein structure. Annu. Rev. biophysics Bioeng. 6, 151–176. doi:10.1146/annurev.bb.06.060177.001055
Rodrigues, C. H. M., Myung, Y., Pires, D. E. V., and Ascher, D. B. (2019). mCSM-PPI2: predicting the effects of mutations on protein–protein interactions. Nucleic Acids Res. 47 (W1), W338–W344. doi:10.1093/nar/gkz383
Shang, J., Ye, G., Shi, K., Wan, Y., Luo, C., Aihara, H., et al. (2020). Structural basis of receptor recognition by SARS-CoV-2. Nature 581 (7807), 221–224. doi:10.1038/s41586-020-2179-y
Sliepen, K., Ozorowski, G., Burger, J. A., van Montfort, T., Stunnenberg, M., LaBranche, C., et al. (2015). Presenting native-like HIV-1 envelope trimers on ferritin nanoparticles improves their immunogenicity. Retrovirology 12 (1), 82–85. doi:10.1186/s12977-015-0210-4
Song, N., Zhang, J., Zhai, J., Hong, J., Yuan, C., and Liang, M. (2021). Ferritin: a multifunctional nanoplatform for biological detection, imaging diagnosis, and drug delivery. Drug Deliv. Chem. Res. 54 (17), 3313–3325. doi:10.1021/acs.accounts.1c00267
Tzotzos, S. (2022). Stapled peptides as potential inhibitors of SARS-CoV-2 binding to the hACE2 receptor. J. Peptide Sci. 28 (9), e3409. doi:10.1002/psc.3409
Vangone, A., and Bonvin, A. M. (2015). Contacts-based prediction of binding affinity in protein–protein complexes. ELife 4, e07454. doi:10.7554/eLife.07454
Venkatraman, V., Yang, Y. D., Sael, L., and Kihara, D. (2009). Protein-protein docking using region-based 3D Zernike descriptors. BMC Bioinforma. 10 (1), 407. doi:10.1186/1471-2105-10-407
Walls, A. C., Park, Y.-J., Tortorici, M. A., Wall, A., McGuire, A. T., and Veesler, D. (2020). Structure, function, and antigenicity of the SARS-CoV-2 spike glycoprotein. Cell 181 (2), 281–292. doi:10.1016/j.cell.2020.02.058
Wang, R., Huang, H., Yu, C., Sun, C., Ma, J., Kong, D., et al. (2022). A spike-trimer protein-based tetravalent COVID-19 vaccine elicits enhanced breadth of neutralization against SARS-CoV-2 Omicron subvariants and other variants. Sci. China Life Sci. 66, 1818–1830. doi:10.1007/s11427-022-2207-7
Wang, Y., Yan, A., Song, D., Dong, C., Rao, M., Gao, Y., et al. (2023). Biparatopic antibody BA7208/7125 effectively neutralizes SARS-CoV-2 variants including Omicron BA.1-BA.5. Cell Discov. 9 (1), 3. doi:10.1038/s41421-022-00509-9
Keywords: peptide design, molecular dynamics simulation, biolayer interferometry (BLI), shape complementarity, ferritin-based nanoparticle
Citation: Parisi G, Piacentini R, Incocciati A, Bonamore A, Macone A, Rupert J, Zacco E, Miotto M, Milanetti E, Tartaglia GG, Ruocco G, Boffi A and Di Rienzo L (2024) Design of protein-binding peptides with controlled binding affinity: the case of SARS-CoV-2 receptor binding domain and angiotensin-converting enzyme 2 derived peptides. Front. Mol. Biosci. 10:1332359. doi: 10.3389/fmolb.2023.1332359
Received: 02 November 2023; Accepted: 14 December 2023;
Published: 05 January 2024.
Edited by:
Didier Devaurs, University of Edinburgh, United KingdomReviewed by:
Emilia A. Lubecka, Gdansk University of Technology, PolandPilar Cossio, Flatiron Institute, United States
Copyright © 2024 Parisi, Piacentini, Incocciati, Bonamore, Macone, Rupert, Zacco, Miotto, Milanetti, Tartaglia, Ruocco, Boffi and Di Rienzo. This is an open-access article distributed under the terms of the Creative Commons Attribution License (CC BY). The use, distribution or reproduction in other forums is permitted, provided the original author(s) and the copyright owner(s) are credited and that the original publication in this journal is cited, in accordance with accepted academic practice. No use, distribution or reproduction is permitted which does not comply with these terms.
*Correspondence: Lorenzo Di Rienzo, bG9yZW56by5kaXJpZW56b0BpaXQuaXQ=
†These authors have contributed equally to this work