- The Key Laboratory of Molecular Biology of Infectious Diseases Designated by the Chinese Ministry of Education, Chongqing Medical University, Chongqing, China
Introduction: Coronavirus disease 2019 (COVID-19) has become a global pandemic and poses a serious threat to human health. Many studies have shown that pre-existing nonalcoholic steatohepatitis (NASH) can worsen the clinical symptoms in patients suffering from COVID-19. However, the potential molecular mechanisms between NASH and COVID-19 remain unclear. To this end, key molecules and pathways between COVID-19 and NASH were herein explored by bioinformatic analysis.
Methods: The common differentially expressed genes (DEGs) between NASH and COVID-19 were obtained by differential gene analysis. Enrichment analysis and protein-protein interaction (PPI) network analysis were carried out using the obtained common DEGs. The key modules and hub genes in PPI network were obtained by using the plug-in of Cytoscape software. Subsequently, the hub genes were verified using datasets of NASH (GSE180882) and COVID-19 (GSE150316), and further evaluated by principal component analysis (PCA) and receiver operating characteristic (ROC). Finally, the verified hub genes were analyzed by single-sample gene set enrichment analysis (ssGSEA) and NetworkAnalyst was used for the analysis of transcription factor (TF)-gene interactions, TF-microRNAs (miRNA) coregulatory network, and Protein-chemical Interactions.
Results: A total of 120 DEGs between NASH and COVID-19 datasets were obtained, and the PPI network was constructed. Two key modules were obtained via the PPI network, and enrichment analysis of the key modules revealed the common association between NASH and COVID-19. In total, 16 hub genes were obtained by five algorithms, and six of them, namely, Kruppel-like factor 6 (KLF6), early growth response 1 (EGR1), growth arrest and DNA-damage-inducible 45 beta (GADD45B), JUNB, FOS, and FOS-like antigen 1 (FOSL1) were confirmed to be closely related to NASH and COVID-19. Finally, the relationship between hub genes and related pathways was analyzed, and the interaction network of six hub genes was constructed with TFs, miRNAs, and compounds.
Conclusion: This study identified six hub genes related to COVID-19 and NASH, providing a new perspective for disease diagnosis and drug development.
Introduction
COVID-19 is an acute respiratory disease caused by severe acute respiratory syndrome coronavirus 2 (SARS-CoV-2) infection. Given that the virus is highly contagious and can be transmitted through respiratory droplets and close contact, it has been prevalent all over the world and caused serious health problems. Novel coronavirus mainly affects respiratory organs, resulting in upper respiratory symptoms such as dry cough, fever, fatigue, and nasal congestion. Patients with severe COVID-19 rapidly develop acute respiratory distress syndrome, metabolic acidosis, coagulation dysfunction, and multiple organ failure (Price-Haywood et al., 2020). However, COVID-19 is also associated with extrapulmonary manifestations, including muscle and joint pain, loss of smell and taste, ocular conjunctival congestion, diarrhea, rash, and neurological symptoms (Gupta et al., 2020). It has been observed that approximately 14%–53% of COVID-19 patients without pre-existing liver disease suffer from mild to moderate liver injury (Ji et al., 2020; Wang et al., 2020), and that the increased proportion of liver injury in severe COVID-19 cases is significantly higher than that in mild cases (Cai et al., 2020; Huang et al., 2020). In addition, patients with previous liver disease present more severe symptoms and have higher mortality as a result of this viral disease (Su and Hsu, 2021).
Non-alcoholic fatty liver disease (NAFLD) is a common liver disease, which is the manifestation of metabolic syndrome in the liver, including simple steatosis, NASH, and liver cirrhosis. It has become the main cause of adult chronic liver disease (Ludwig et al., 1980). NASH is a severe type of NAFLD, which can lead to liver fibrosis and cirrhosis. The global prevalence of NAFLD is 25%, while that of NASH in NAFLD patients is 59.10% (Younossi et al., 2018), and the prevalence rate is increasing year by year. Patients with NAFLD/NASH have been shown to be exposed to a higher risk of severe COVID-19 disease (Boettler et al., 2020; Su and Kao, 2020). A number of studies have demonstrated the association between the odds of intensive care unit admission and mortality in patients with COVID-19 and pre-existing NAFLD or NASH. In addition, the ratio of medical device use during hospitalization has significantly increased, presenting statistical significance (Hashemi et al., 2020). In addition, a large number of database studies in the United States indicated a strong positive correlation between metabolic syndrome and the risk of COVID-19, and among all the hereby investigated metabolic risk factors, the incidence of NASH and COVID-19 was the strongest (Ghoneim et al., 2020). COVID-19 is still in the epidemic stage, and the number of infections is increasing. Understanding the interaction of the two diseases, NASH and COVID-19, is critical for treating COVID-19 patients with NASH comorbidities.
Herein, the interaction mechanism of NASH and COVID-19 was analyzed using bioinformatics. The expression profile data of NASH and COVID-19 were obtained from the Gene Expression Omnibus (GEO) database. After determining the DEGs shared by NASH and COVID-19, functional annotation, PPI network creation, and key module analysis, as well as identification and validation of hub genes, were performed. Finally, six important hub genes were obtained, ssGSEA was performed on the six hub genes, and protein–chemical, gene–TF, and gene–miRNA interaction networks were constructed. Overall, the hub genes obtained from the analysis provides new insights into the potential molecular mechanism of the coexistence of NASH and COVID-19.
Result
Identification of DEGs between COVID-19 and NASH
In order to study the interaction between NASH and COVID-19, differential analyses for transcriptome datasets for COVID-19 and microarray datasets for NASH were performed. Genes that met the criteria of the false discovery rate (FDR) < 0.05 and |log2FoldChange |≥1 were identified as DEGs, and there were 11,860 DEGs between SARS-CoV-2-infected patients and normal individuals (Figure 1A). Meanwhile, 637 DEGs were observed between NASH patients and normal individuals (Figure 1B). A total of 183 common DEGs were identified after taking the intersection (Figure 1C), and there were 120 DEGs that shared the same expression trend, including 53 upregulated genes and 67 downregulated genes.
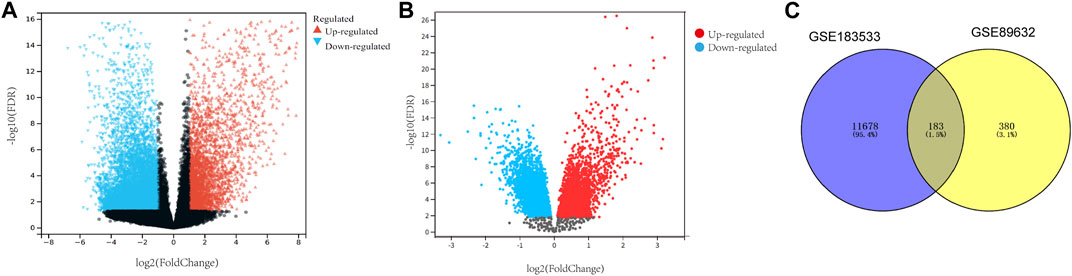
FIGURE 1. Identification of common DEGs in both NASH and COVID-19. (A) Volcano map of the COVID-19 dataset. (B) Volcano map of the NASH dataset. (C) Venn diagram displaying the shared DEGs among COVID-19 and NASH. DEGs, differentially expressed genes; NASH, non-alcoholic steatohepatitis; COVID-19, coronavirus disease 2019.
Gene Ontology and Kyoto Encyclopedia of Genes and Genomes analyses
Gene Ontology (GO) analysis focuses on describing the properties of genes or proteins, paying attention to the functions of genes in cells or organisms. Kyoto Encyclopedia of Genes and Genomes (KEGG) analysis focuses on describing the actions or interactions of genes or proteins inside and outside the cell, paying attention to the role of genes in metabolic pathways.
In order to further explore the functions and pathways of 120 common DEGs, GO and KEGG enrichment analyses were carried out. GO analysis showed that the genes were mainly enriched in the regulation of miRNA transcription, RNA polymerase II transcription regulator complex, DNA-binding transcription activator activity, and integrated stress response signaling. KEGG analysis showed that the gene was enriched in osteoclast differentiation, bile secretion, TNF signaling pathway, and glycerolipid metabolism pathways (Figures 2A, B).
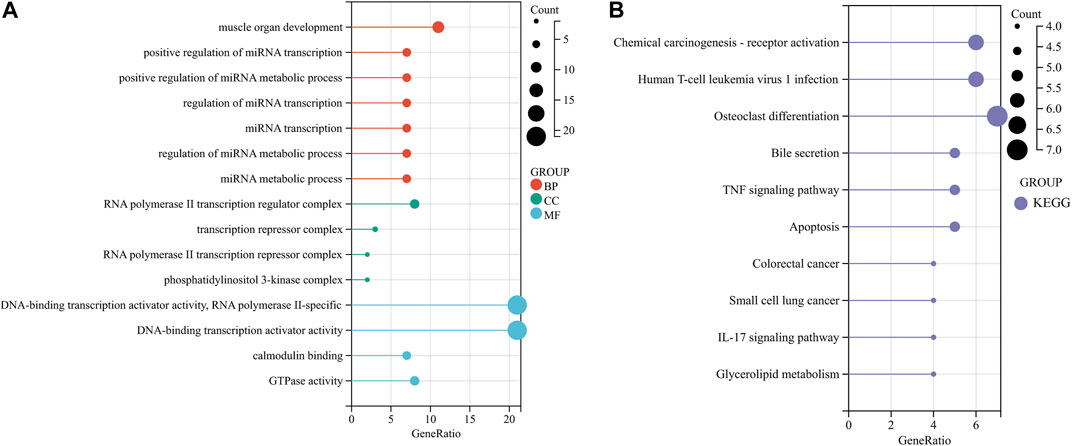
FIGURE 2. Functional enrichment analysis of common DEGs. (A, B) Enrichment analysis results of GO and KEGG pathways. BP, CC, and MF belong to GO analysis. GO, Gene Ontology; KEGG, Kyoto Encyclopedia of Genes and Genomes; BP, biological process; CC, cellular component; MF, molecular function.
PPI network construction and module analysis
In order to explore the interaction between proteins encoded by common DEGs, a PPI network of 120 common DEGs was created using the STRING online website. The network consisted of 116 nodes and 185 edges (Figure 3A). The two most closely related gene modules were obtained using Cytoscape’s MCODE plug-in. Module 1 had 10 nodes and 44 edges, and the score (density multiplied by the number of members) was 9.778 (Figure 3B). Module 2 had six nodes and eight edges, with a score of 3.2 (Figure 3C). In terms of biological function, the genes in the key modules were mainly involved in the regulation of miRNA transcription and metabolic pathways, RNA polymerase II transcription regulator complex, and DNA-binding transcription activator activity (Figure 3D). For the purpose of significant pathway analysis, WikiPathways and Reactome databases were also used alongside the KEGG pathway. The enrichment analysis showed that the genes in the key modules were mainly enriched in inflammatory signaling pathways, for example, IL-17 signaling pathway, TNF signaling pathway, human T-cell leukemia virus 1 infection, TGF-beta signaling pathway, and NGF-stimulated transcription (Figure 3E). Inflammation is a characteristic of NASH and is considered to be the driving force for the progression of the disease to fibrosis, cirrhosis, or HCC. In addition, novel coronavirus can induce systemic inflammation. The inflammatory reaction was closely related to COVID-19 and non-alcoholic hepatitis. Therefore, it was speculated that the inflammation-related pathways involved in the genes in the key modules may play an important role in the occurrence and development of these two diseases. The top enriched shared pathways are summarized in Supplementary Tables S1, S2.
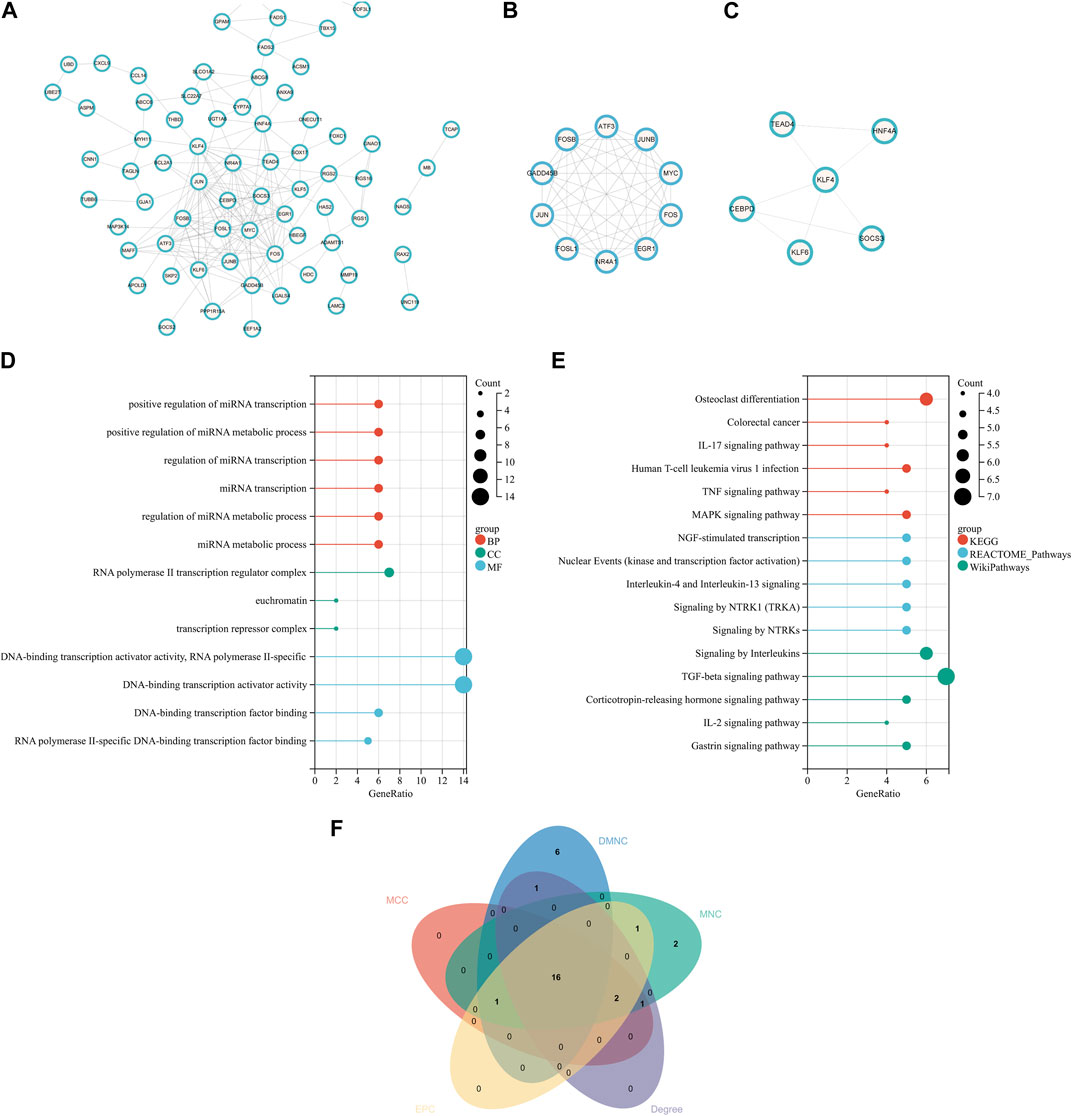
FIGURE 3. PPI network, analysis of key modules, and identification of hub genes. (A) PPI network of the common DEGs. (B, C) Key modules of the PPI network. (D) GO enrichment analysis results of the modular genes. (E) Results of KEGG, Reactome, and WikiPathways enrichment analyses of the modular genes. (F) Venn diagram showing that 16 hub genes were obtained by five algorithms. PPI, protein–protein interaction.
Hub gene identification
Key genes playing an important role in the coexistence of NASH and COVID-19 were identified using cytoHubba. The top 20 hub genes in the PPI network were obtained by MCC, MNC, DMNC, Degree, and EPC. After the intersection with the online Venn tool, 16 common hub genes were obtained (Figure 3F), which were all downregulated genes. Most of these genes existed in key modules 1 and 2. The top 20 hub genes in five algorithms are listed in Supplementary Table S3.
Validation of hub genes
The expression levels of 16 hub genes were verified by GSE150316 for COVID-19 and GSE180882 for NASH. The results showed that compared with normal tissues, six hub genes in COVID-19 and NASH datasets, namely, FOS, JUNB, EGR1, KLF6, FOSL1, and GADD45B, were significantly downregulated (Figures 4A, B). In addition, six hub genes were further identified by PCA, and the results showed that the dimension of these six genes was simplified to two principal components of PC1 and PC2. PC1 and PC2 of two experimental sets and two validation sets were accounted for more than 70% of the total variance. After dimensionality reduction, six genes could clearly distinguish between disease samples and normal samples in four datasets (Figures 4C–F). At the same time, the expression of six hub genes in related cancers was also analyzed. Based on TCGA and GTEx databases, the expression levels of FOS, GADD45B, and EGR1 in liver hepatocellular carcinoma (LIHC) and lung adenocarcinoma (LUAD) were lower than those in normal samples. KLF6 and JUNB were downregulated in LUAD and lung squamous cell carcinoma (LUSC) (p < 0.05). The results are shown in Supplementary Figures S1A–F.
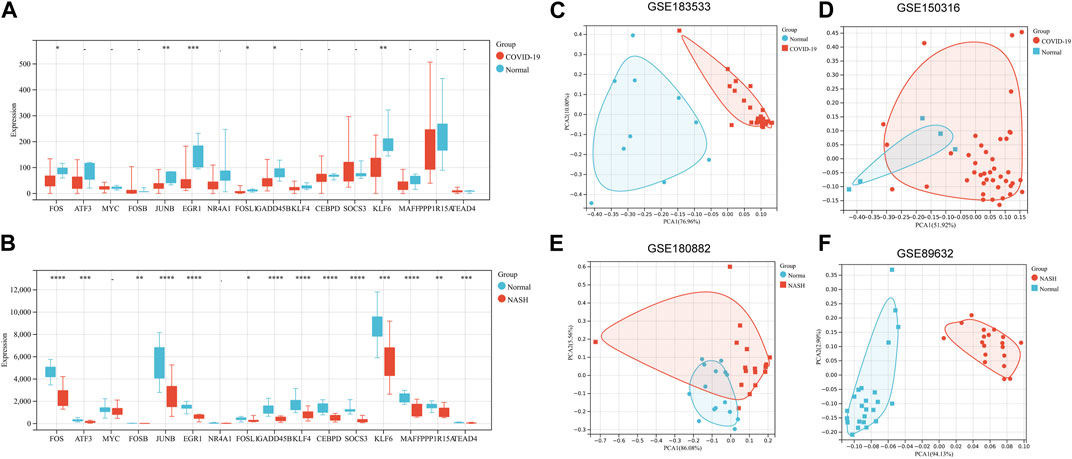
FIGURE 4. Validation of hub genes. (A) Validation of 16 hub genes’ expression in GSE150316 for COVID-19. (B) Validation of 16 hub genes’ expression in GSE180882 for NASH. (C–F) PCA for six key genes (FOS, FOSL1, JUNB, KLF6, EGR1, and GADD45B) in GSE89632, GSE180882, GSE183533, and GSE150316. *p < 0.05, **p < 0.01, ***p < 0.001. PCA, principal component analysis.
Evaluation of hub genes
The ROC of six hub genes in the datasets (GSE183553, GSE89632, GSE89632, and GSE180882) were drawn to evaluate the prediction accuracy of hub genes. In the four datasets, the area under the curve (AUC) values of five hub genes were more than 0.8 (Figures 5A–D). It was proved that FOS, JUNB, EGR1, KLF6, FOSL1, and GADD45B showed favorable diagnostic value. These results demonstrated the potential of the aforementioned hub genes as biomarkers for the diagnosis of NASH and COVID-19.
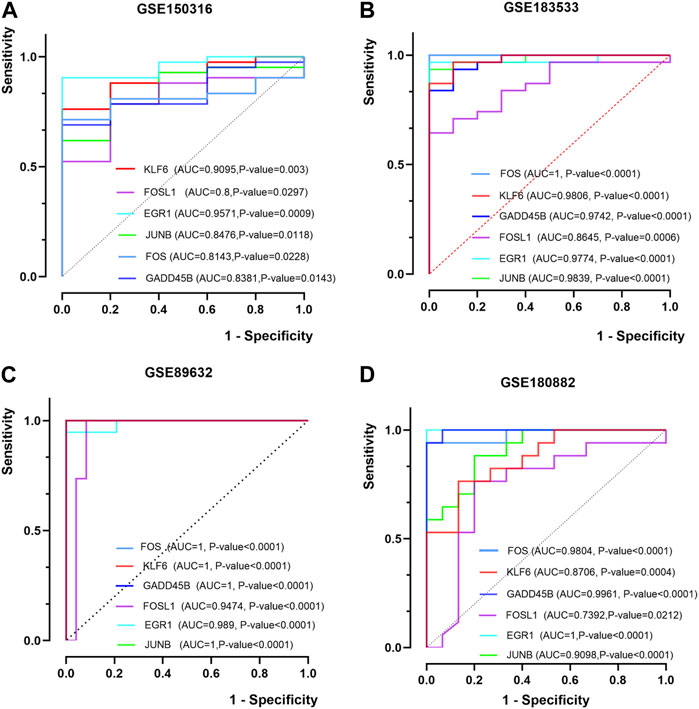
FIGURE 5. ROC curves of the hub genes. (A, B) ROC curves of six hub genes in GSE150316 and GSE183533 for COVID-19. (C, D) ROC curves of six hub genes in GSE89632 and GSE180882 for NASH. ROC, receiver operating characteristic; AUC, area under the curve.
Relationship between hub genes and related pathways
Based on the enrichment results of key modules, 30 pathways were selected from KEGG and WikiPathways gene sets, and Spearman’s correlation analysis was used to analyze their relationship with six hub genes (Figures 6A–D). As shown in the figure, EGR1, KLF6, FOSL1, and FOS were negatively correlated with fatty acid metabolism, oxidation by cytochrome P450, and eicosanoid metabolism via the cytochrome P450 monooxygenases pathway. GADD45B and KLF6 had a negative correlation with the VEGFA–VEGFR2 signaling pathway, TNF-alpha signaling pathway, and TGF-beta signaling pathway. JUNB had a negative correlation with the TNF signaling pathway, gastrin signaling pathway, and IL-17 signaling pathway. In total, 15 of the 30 passageways are shown in Supplementary Figures S2A–D.
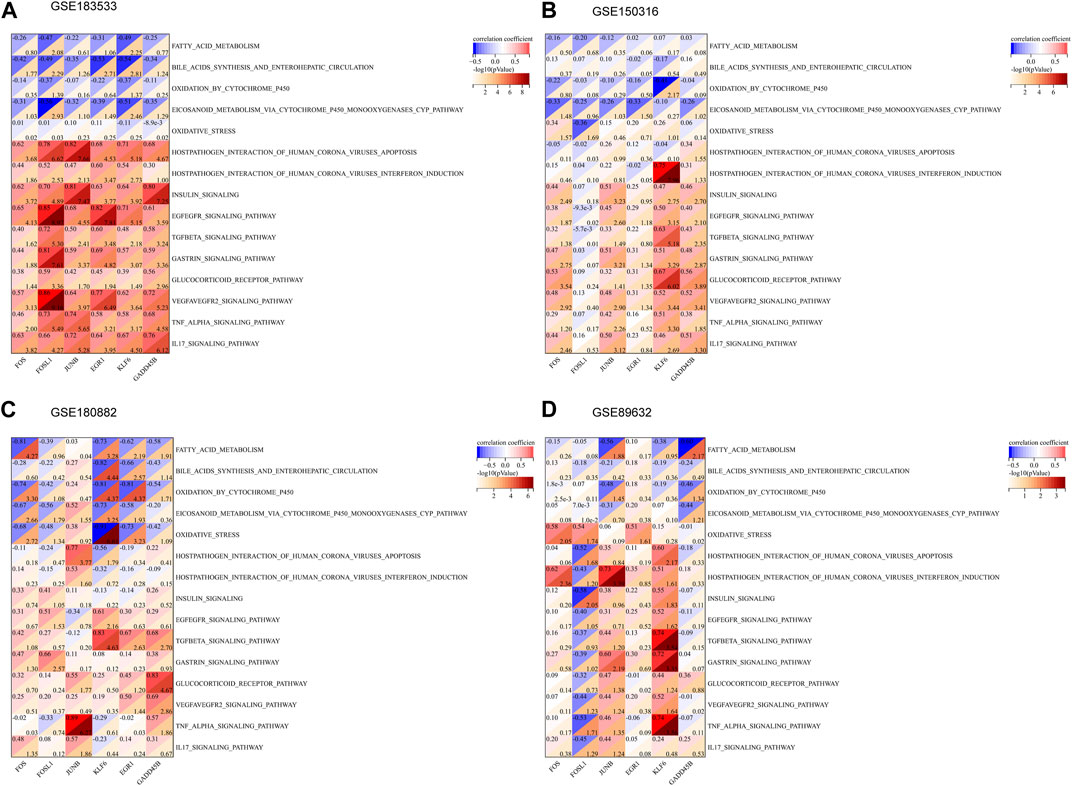
FIGURE 6. Association between the hub genes and related pathways. (A–D) Correlation results of hub genes and 15 pathways in GSE183533, GSE150316, GSE180882, and GSE89632.
Networks of gene–miRNA and TF–gene interactions
In order to deeply explore the regulatory molecules of hub genes, the gene–miRNA interaction network was constructed, which consisted of 37 nodes and 116 edges. mir-191-5p, mir-155-5p, and mir-1-3p interacted with six hub genes: FOS, JUNB, EGR1, KLF6, FOSL1, and GADD45B. Meanwhile, four hub genes (EGR1, FOS, KLF6, and FOSL1) had a higher degree of connection in the network (Figure 7A; Supplementary Table S4). In addition, the TF–gene interaction network comprising 41 nodes and 59 edges was also identified, where TFs interacted with five hub genes: JUNB, GADD45B, FOS, EGR1, and KLF6. CREB1, RELA, E2F6, and ESR1 were identified as important TFs (Figure 7B; Supplementary Table S5).
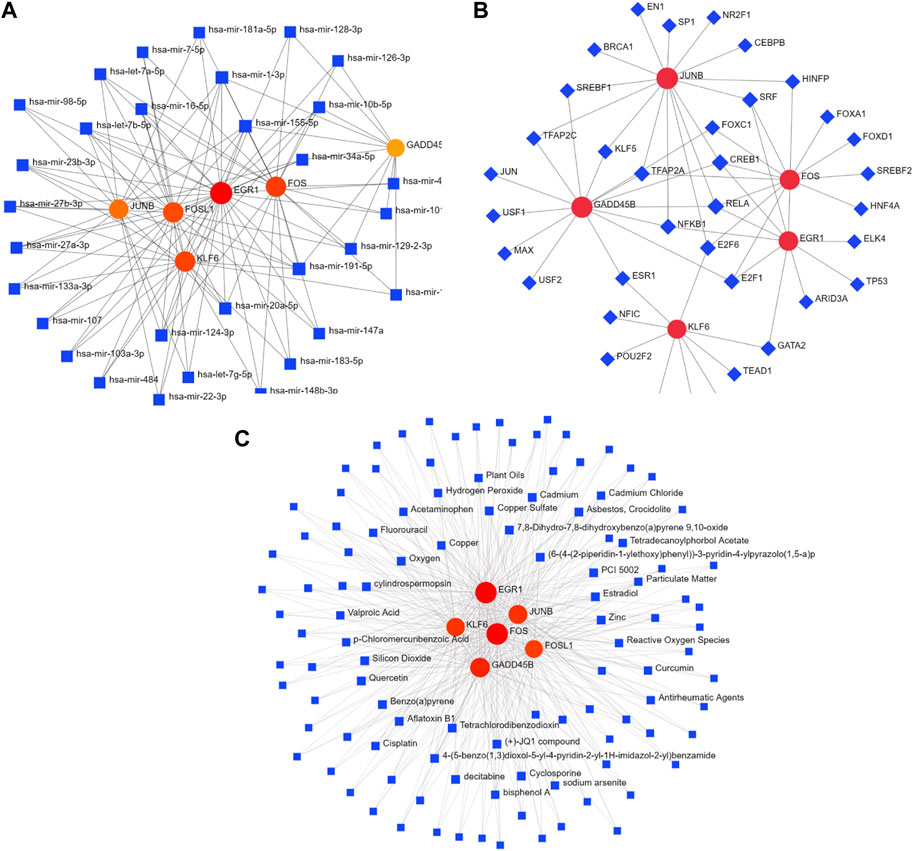
FIGURE 7. Interaction network of DEGs with TFs, miRNAs, and chemicals. (A) Interaction network of TF–DEG. Blue square nodes represent TFs, and gene symbols interacting with TFs are denoted as red circle nodes. (B) Interaction network of DEG–miRNA. Blue square nodes are miRNAs, and gene symbols interacting with TFs are denoted as circle nodes. (C) Interaction network of protein–chemical. Blue square nodes represent chemicals, and proteins interacting with chemicals are denoted as circle nodes. TFs, transcription factors.
Networks of protein–chemical interactions
Protein–chemical interaction networks help understand the mechanisms of disease occurrence and provide assistance in drug development. Herein, the networks of protein–chemical interactions for hub genes were established, which comprised 114 nodes and 434 edges (Figure 7C; Supplementary Table S6). Aflatoxin B1, oxygen, quercetin, and cyclosporine were the compounds that interacted with all six hub genes.
Discussion
As SARS-CoV-2 continues to spread worldwide, many studies have shown that patients with NASH are more likely to develop severe COVID-19 after being infected with SARS-CoV-2. In addition, patients with NASH disease will experience increased liver symptoms after being infected with COVID-19 (Bangash et al., 2020; Li and Fan, 2020; Mendez-Sanchez et al., 2020; Rismanbaf and Zarei, 2020). The common symptoms of NASH are obesity, type 2 diabetes, and hyperlipidemia, which are closely related to metabolic syndrome (Eslam et al., 2020). In metabolic abnormalities, expansion of metabolically active fat (high body mass index) can exacerbate chronic inflammatory changes, development of insulin resistance, and fibrosis (Guan et al., 2020; Guo et al., 2020). The detrimental interactions of the complex inflammatory pathways that have long been present in NASH may be dramatically enhanced after infection with novel coronavirus (Portincasa et al., 2020). To improve public understanding of the relationship between COVID-19 and NASH and provide new treatment ideas for patients with COVID-19 and NASH, the possible molecular biological functions and pathways between COVID-19 and NASH were hereby analyzed using bioinformatics.
Herein, 120 common DEGs between COVID-19 and NASH were obtained. The results of GO enrichment analysis showed that DEGs were mainly enriched in the regulation of miRNA transcription, RNA polymerase II transcription regulator complex, and DNA-binding transcription activator activity. Many sources of evidence have suggested the important role of Homo sapiens (hsa)-miRNAs in targeting the viral genome, regulating inflammatory signaling pathways, and enhancing the production/signaling of IFNs-I (Xu et al., 2014; Mortazavi-Jahromi et al., 2020a; Mortazavi-Jahromi et al., 2020b; El-Nabi et al., 2020; Guterres et al., 2020; Khan et al., 2020; Nersisyan et al., 2020). It is hypothesized that the immune inflammatory response triggered by COVID-19 affects the development of the disease by influencing the expression level and function of hsa-miRNAs (O'Connell et al., 2007; Ghorpade et al., 2013; Du et al., 2015; Chung et al., 2017). The results of KEGG enrichment analysis showed that DEGs were enriched in bile secretion, TNF signaling pathway, glycerolipid metabolism, and osteoclast differentiation. Generally, the TNF signaling pathway plays an important role in immune-involved inflammatory response and apoptosis (Bradham et al., 1998; Ding and Yin, 2004). The inhibition of cell death pathways mediated by TNF-α and IFN-γ to reduce tissue damage and inflammation is an adjuvant therapy for COVID-19 and other inflammatory diseases (Karki et al., 2021). Bile acids matter considerably in insulin sensitivity and metabolic homeostasis. Studies have reported the correlation between bile acid levels and the severity of NASH, as well as the dysregulation of free fatty acid-induced bile acid signaling in NASH (Bechmann et al., 2013). Bile acid derivatives and compounds that affect bile acid-related signaling pathways are considered potentially useful therapeutic agents for NASH (Arab et al., 2017; Gottlieb and Canbay, 2019). Enrichment analysis was performed on genes in key modules with scores greater than 3 in the PPI network. The enrichment results of genes in key modules and DEGs were similar to those in GO analysis. The pathways enriched in KEGG analysis included IL-17 signaling pathway and human T-cell leukemia virus 1 infection. HTLV-1 infection is a systemic inflammatory disease characterized by chronic evolution. Some studies have shown that both SARS-CoV-2 infection and HTLV-1 infection somehow shared similar immunologic properties (Sajjadi et al., 2022). WikiPathways showed that genes were mainly involved in the TGF-beta signaling pathway and network map of the SARS-CoV-2 signaling pathway. The signaling pathway map showed protein–protein interactions and downstream molecular events regulated by SARS-CoV-2, which was expected to contribute to the development of novel targeted therapeutics for COVID-19. Reactome pathways indicated that genes were mainly enriched in NGF-stimulated transcription. The expression of NGF was upregulated in response to liver inflammation or injury. It has been proved that NGF can improve liver fibrosis by regulating HSC cell apoptosis (Oakley et al., 2003). In addition, NGF has also been considered capable of providing a protective mechanism against oxidative damage (Tsai et al., 2014).
Bioinformatic analysis was used to obtain six hub genes associated with NASH and COVID-19 from PPI networks: FOS, JUNB, EGR1, KLF6, FOSL1, and GADD45B. These genes might play an important role in the development of the disease. GADD45B is a member of the GADD45 protein family and plays an important role in regulating a variety of cellular functions. GADD45B has paradoxical effects: positively, it promotes proliferation, growth and cell survival, playing a dominant role in hepatocytes, while negatively, it inhibits proliferation and stimulates apoptosis, especially in hepatocellular carcinoma. These effects depend on the cellular environment (Tianand and Locker, 2013). Studies have shown that GADD45B plays a regulatory role in liver-related metabolic diseases (Fuhrmeister et al., 2016; Cai et al., 2021). KLF6 is a transcription factor with tumor-suppressive effects. The overexpression of KLF6 has negative effects on tumor growth and progression; the silence of KLF6 leads to the increase of tumorigenicity (Ito et al., 2004; Sangodkar et al., 2009; Ahronian et al., 2016; Hsu et al., 2017). Studies have shown that KLF6 exhibits a cell growth inhibition function by regulating the expression of genes involved in cell cycle, apoptosis, and aging (Narla et al., 2001; Huang et al., 2008; Gao et al., 2017; Sabatino et al., 2019).
EGR1 is a transcription factor that plays a key role in inflammation and tissue repair (Hoffmann et al., 2008; Trizzino et al., 2021; Woodson and Kehn-Hall, 2022). Studies have shown the importance of EGR1 in maintaining the hepatic insulin response and claimed that the loss of EGR1 in hepatocytes leads to hepatic steatosis, which exacerbates the progression of non-alcoholic liver disease. This study revealed a previously unrecognized role of EGR1 in regulating lipid metabolism (Magee and Zhang, 2018; Li et al., 2019). In addition, the expression of EGR1 was positively correlated with the expression of Fos gene family, including FOS, FOSB, FOSL1, and FOSL2, suggesting that the two immediately expressed gene families might be cross-activated and mutually regulated (Yuan et al., 2022). FOS, FOSL1, and JUNB proteins are subunits of transcription factor activator protein-1 (AP-1). JUNB and FOS can combine with Jun to form transcription factor complex AP-1. The functions and pathways of these genes in inflammation development and metabolic responses are diverse. For example, studies have shown that FOSL1-deficient animals have less lung injury and a higher survival rate than the control group in experimental models of acute lung injury (Takada et al., 2011; Vaz et al., 2012). Another study has shown that FOSL1 expression in liver cells protects liver cells from secondary liver injury and inflammation (Hasenfuss et al., 2014). The function and pathway of these genes depend on the stage of the disease and the cellular environment. Herein, KEGG enrichment analysis showed that FOSL1, JUN, FOSB, and FOS were enriched in the IL-17 signaling pathway. Reactome pathway enrichment analysis showed that FOSL1, JUNB, FOS, and EGR1 were enriched in NGF-stimulated transcription and nuclear events (kinase and transcription factor activation). Additionally, the WikiPathways enrichment analysis showed that JUNB, JUN, FOS, and EGR1 were enriched in the network map of the SARS-CoV-2 signaling pathway and that FOSL1, JUNB, FOSB, and FOS were enriched in the corticotropin-releasing hormone signaling pathway.
The correlation of hub genes with some pathways was analyzed by ssGSEA. The results showed that EGR1, KLF6, FOSL1, and FOS were negatively correlated with the cytochrome P450 functional pathway, consistent with the increase in cytochrome P450 metabolism in NASH liver-like organs (McCarron et al., 2021). GADD45B and KLF6 were positively correlated with the TGF-β signaling pathway. TGF-β is a multifunctional cytokine with pro-fibrosis, anti-inflammatory, and immunosuppressive effects. In COVID-19 patients, the increased levels of TGF-β may occur to counteract the high inflammatory response (Russell et al., 2020), but it can also slow the recovery of disease in vivo by inhibiting the activity of the immune system in vivo (Sheng et al., 2015; Ferreira-Gomes et al., 2021). In addition, JUNB was hereby found positively correlated with the IL-17 signaling pathway and gastrin signaling pathway. IL-17 is a member of the multifunctional cytokine family. Studies have shown that IL-17 can produce protective and pathological effects in vivo (Amatya et al., 2017). The analysis of coronavirus-induced lung diseases has shown that the severity of the disease is positively correlated with the level of IL-17. IL-17 can be used not only as a biomarker of disease severity but also as a potential therapeutic target to mitigate the damage of SARS-CoV-2. However, studies have shown that mice lacking functional IL-17 receptor signaling are more likely to develop secondary pneumonia caused by bacteria after viral infection than wild-type mice (Kudva et al., 2011). The role of IL-17 in the immune system is complex and subtle. Extract-mixed solution can increase the expression of gastrin and motilin to reduce liver fat deposition, protect liver function, and slow down the development of NAFLD (Chen et al., 2013).
In order to further understand the regulatory mechanisms associated with hub genes, TFs and miRNAs related to six hub genes were hereby analyzed. TFs in the network such as CREB1, RELA, E2F1, NFKB1, and TFAP2A play important roles in the regulation of immunity, inflammation, cell proliferation, and apoptosis. The miRNAs that interact more with hub genes are hsa-mir-191-5p, hsa-mir-155-5p, hsa-mir-1-3p, hsa-mir-20a-5p, and hsa-mir-124-3p. Studies have shown that hsa-mir-191-5p regulates mitochondrial function by regulating the level of gene expression (Quiñones-Lombrañan and Blanco, 2015). Mitochondrial dysfunction leads to the formation of reactive oxygen and reactive nitrogen, increased steatosis, and lipid accumulation in hepatocytes (Grattagliano et al., 2012). In addition, hsa-mir-155-5p is involved in the regulation of immune response to SARS-CoV-2, cytokine storm, and antiviral processes (Qi et al., 2021). hsa-mir-124-3p is considered a potential candidate for treating COVID-19 and regulating ACE2 networks (Wicik et al., 2020; Prasad et al., 2021). Sardar et al. (2020) showed that the antiviral miRNAs, such as hsa-mir-1-3p and hsa-mir-20a-5p, were downregulated in the blood of patients with viral respiratory diseases.
Finally, several compounds that interacted with hub genes were identified through NetworkAnalyst. Quercetin is a well-known natural polyphenol with strong antioxidant, anti-inflammatory, immunomodulatory, and antiviral properties. In vitro and in vivo studies have shown that quercetin can be used as a candidate drug for the treatment of COVID-19 (Di Pierro et al., 2021; Saeedi-Boroujeni and Mahmoudian-Sani, 2021). Decitabine belongs to nucleoside analogs and is known as a broad-spectrum antiviral drug that inhibits viral transcription or replication by inhibiting DNA methylation (Ianevski et al., 2018). Several studies on cell lines have found that decitabine reduces SARS-CoV-2 replication (Zhang et al., 2020; Jang et al., 2021; Zheng et al., 2021). In addition, several clinical trials have been conducted to evaluate the therapeutic efficacy of decitabine in critically ill COVID-19 patients. Meanwhile, zinc is endowed with great significance in enhancing immune function and reducing inflammation (Bonaventura et al., 2015; Maares and Haase, 2016). In elderly subjects, the decrease in circulating zinc concentration is associated with increased levels of cytokines such as IL-6, IL-8, and TNF-α (Mariani et al., 2006; Barnett et al., 2010), making it a qualified candidate as a prophylaxis and adjuvant treatment for COVID-19 in high-risk groups.
In the post-epidemic era, how to provide better medical advice for patients with complications is an essential problem to be solved. In this study, to increase the understanding of the relationship between COVID-19 and NASH, bioinformatic analysis was used to identify key genes in COVID-19 and NASH, analyze gene-related pathways, and identify TFs, miRNAs, and compounds that interact with the genes. It is expected that the study results can provide new ideas for drug development in patients with complications, but the research also has some limitations: (i) the study fails to judge whether the downregulation of hub genes can lead to the deterioration of the disease or has a protective effect on the body. Further study will be carried out if more clinical information can be collected in the future; and (ii) the hub genes identified in the study are calculated and analyzed by bioinformatics and have not been verified in clinical trials or in vitro studies.
Method
Data source
The datasets used in the study were downloaded from the GEO database (http://www.ncbi. nlm. nih.gov/geo). “NASH” and “COVID-19” were used as keywords for searching the related gene expression dataset. The GSE183533 dataset contains the RNA sequencing results of lung samples from 31 patients with COVID-19 and 10 healthy individuals. Meanwhile, the GSE89632 dataset contains liver biopsy samples from 19 patients with NASH and 24 healthy individuals. The validation dataset includes GSE180882 (NASH) and GSE150316 (COVID-19).
Identification of DEGs in NASH and COVID-19
By using the transcriptome count data difference analysis tool of the Sangerbox online website (http://vip.sangerbox.com/home.html), the GSE183533 dataset was analyzed using the DESeq2 (version 1.32.0) package in R software. The GEO2R (www.ncbi.nlm.nih.gov/geo/ge2r) online analysis tool was used to perform differential analysis on the GSE89632 dataset. The differential genes between the experimental group and the control group were obtained, and genes that met the criteria of FDR <0.05 and |log2FoldChange |≥1 were identified as DEGs. Common DEGs between NASH and COVID-19 datasets were obtained using the online Venn diagram tool (https://bioinfogp.cnb. csic.es/tools/venny/). An adjusted p-value was corrected by the Benjamini–Hochberg procedure.
Enrichment analysis of DEGs
By using the GO/KEGG analysis tool of the online HIPLOT website (https://hiplot-academic.com/), GO and KEGG analyses were performed using the “clusterProfiler” package in R software to investigate possible functions and pathways in DEGs. GO items included biological process (BP), molecular function (MF), and cellular component (CC).
Construction of the PPI network and analysis of key modules
The PPI network of DEGs with a score (medium confidence) larger than 0.4 was constructed by the interaction gene search database search tool (STRING) (https://cn.string-db.org/) and visualized using Cytoscape 3.9.0. The plug-in MCODE of Cytoscape was used to identify important modules in the PPI network. The criteria were set as follows: Degree Cutoff Module = 2, Node Score Cutoff = 0.2, K-Core = 2, and Max Depth = 100. In addition, to analyze the functional pathways of key modules, GO, KEGG, Reactome and WikiPathways enrichment analyses of genes in key modules (clusters with scores >3) were performed on the online HIPLOT website. Enriched terms with adjusted p < 0.05 were considered significant.
Identification of hub genes
Hub genes of the PPI network were identified by five algorithms of Cytoscape plug-in cytoHubba: MCC, DMNC, MNC, Degree, and EPC. Intersection genes ranked in the top 20 among the five algorithms were identified as the hub genes.
Verification of hub genes by external datasets
The expression levels of the identified hub genes were verified in GSE150316 and GSE180882. The samples of the NASH dataset (GEO accession ID: GSE180882) were live liver tissue, containing 18 samples of NASH and 15 healthy samples. A total of 42 lung tissue samples infected with SARS-CoV-2 and five healthy lung tissue samples were selected from the GSE150316 dataset. The non-parametric test was conducted to compare the two groups of data. p < 0.05 was considered to be significant.
Verification of hub genes by PCA
The PCA visualization tool of the Sangerbox online website was used to test the ability of hub genes to distinguish different samples using the prcomp function for dimensionality reduction analysis.
Evaluation of hub genes by the ROC curve
The ROC curves were plotted using GraphPad Prism 8.0 software to evaluate the prediction accuracy of hub genes.
Single-sample gene set enrichment analysis
The enrichment scores of samples in the c2.cp. kegg.v7.4. and c2.cp. wikipathways.v7.4 gene sets were calculated by the gene set variation analysis (GSVA) tool of the Sangerbox online website (https://vip. Sangerbox. com/home.html). The correlations between six hub genes and pathways were determined by Spearman’s correlation analysis.
TF–DEG and DEG–miRNA interactions
The NetworkAnalyst platform (https://www.networkanalyst.ca/NetworkAnalyst/) was used to find the TFs interacting with the hub genes from the JASPAR database. The miRNAs interacting with the hub genes were found from TarBase v8.0. The TF–DEG interaction network and DEG–miRNA interaction network were determined.
Protein–chemical interactions
Compounds interacting with the hub genes were identified from The Comparative Toxicogenomics Database (CTD) using the NetworkAnalyst platform, and the protein–chemical interaction network was correspondingly constructed. The degree cutoff was set to 2.
Data availability statement
The datasets presented in this study can be found in online repositories. The names of the repository/repositories and accession number(s) can be found in the article/Supplementary Material.
Author contributions
HL and XC designed the research; HL analyzed the data obtained from the databases and wrote the manuscript. All authors contributed to the article and approved the submitted version.
Funding
This study was supported by the National Key R&D Program of China (2022YFA1303600).
Conflict of interest
The authors declare that the research was conducted in the absence of any commercial or financial relationships that could be construed as a potential conflict of interest.
Publisher’s note
All claims expressed in this article are solely those of the authors and do not necessarily represent those of their affiliated organizations, or those of the publisher, the editors, and the reviewers. Any product that may be evaluated in this article, or claim that may be made by its manufacturer, is not guaranteed or endorsed by the publisher.
Supplementary material
The Supplementary Material for this article can be found online at: https://www.frontiersin.org/articles/10.3389/fmolb.2023.1164220/full#supplementary-material
References
Ahronian, L. G., Zhu, L. J., Chen, Y.-W., Chu, H.-C., Klimstra, D. S., and Lewis, B. C. (2016). A novel KLF6-Rho GTPase axis regulates hepatocellular carcinoma cell migration and dissemination. Oncogene 35 (35), 4653–4662. doi:10.1038/onc.2016.2
Amatya, N., Garg, A. V., and Gaffen, S. L. (2017). IL-17 signaling: The Yin and the yang. Trends Immunol. 38 (5), 310–322. doi:10.1016/j.it.2017.01.006
Arab, J. P., Karpen, S. J., Dawson, P. A., Arrese, M., and Trauner, M. (2017). Bile acids and nonalcoholic fatty liver disease: Molecular insights and therapeutic perspectives. Hepatology 65 (1), 350–362. doi:10.1002/hep.28709
Bangash, M. N., Patel, J., and Parekh, D. (2020). COVID-19 and the liver: Little cause for concern. lancet Gastroenterology hepatology 5 (6), 529–530. doi:10.1016/S2468-1253(20)30084-4
Barnett, J. B., Hamer, D. H., and Meydani, S. N. (2010). Low zinc status: A new risk factor for pneumonia in the elderly? Nutr. Rev. 68 (1), 30–37. doi:10.1111/j.1753-4887.2009.00253.x
Bechmann, L. P., Kocabayoglu, P., Sowa, J. P., Sydor, S., Best, J., Schlattjan, M., et al. (2013). Free fatty acids repress small heterodimer partner (SHP) activation and adiponectin counteracts bile acid-induced liver injury in superobese patients with nonalcoholic steatohepatitis. Hepatology 57 (4), 1394–1406. doi:10.1002/hep.26225
Boettler, T., Newsome, P. N., Mondelli, M. U., Maticic, M., Cordero, E., Cornberg, M., et al. (2020). Care of patients with liver disease during the COVID-19 pandemic: EASL-ESCMID position paper. JHEP Rep. 2 (3), 100113. doi:10.1016/j.jhepr.2020.100113
Bonaventura, P., Benedetti, G., Albarède, F., and Miossec, P. (2015). Zinc and its role in immunity and inflammation. Autoimmun. Rev. 14 (4), 277–285. doi:10.1016/j.autrev.2014.11.008
Bradham, C. A., Plümpe, J. r., Manns, M. P., Brenner, D. A., and Trautwein, C. (1998). Mechanisms of hepatic toxicity. I. TNF-induced liver injury. Am. J. Physiology-Gastrointestinal Liver Physiology 275 (3), G387–G392. doi:10.1152/ajpgi.1998.275.3.G387
Cai, Q., Huang, D., Ou, P., Yu, H., Zhu, Z., Xia, Z., et al. (2020). COVID-19 in a designated infectious diseases hospital outside Hubei Province, China. Allergy 75 (7), 1742–1752. doi:10.1111/all.14309
Cai, X., Feng, Y., Xu, M., Yu, C., and Xie, W. (2021). Gadd45b is required in part for the anti-obesity effect of constitutive androstane receptor (CAR). Acta Pharm. Sin. B 11 (2), 434–441. doi:10.1016/j.apsb.2020.08.015
Chen, S., Zhou, H., Lin, M., Mi, R., and Li, L. (2013). Decoction vs extracts-mixed solution: Effect of yiqihuoxue formula on non-alcoholic fatty liver disease in rats. J. Tradit. Chin. Med. 33 (4), 513–517. doi:10.1016/s0254-6272(13)60157-0
Chung, J.-W., Jeong, S. H., Lee, S. M., Pak, J. H., Lee, G. H., Jeong, J.-Y., et al. (2017). Expression of microRNA in host cells infected with Helicobacter pylori. Gut liver 11 (3), 392–400. doi:10.5009/gnl16265
Di Pierro, F., Derosa, G., Maffioli, P., Bertuccioli, A., Togni, S., Riva, A., et al. (2021). Possible therapeutic effects of adjuvant quercetin supplementation against early-stage COVID-19 infection: A prospective, randomized, controlled, and open-label study. Int. J. Gen. Med. 14, 2359–2366. doi:10.2147/ijgm.S318720
Ding, W. X., and Yin, X. M. (2004). Dissection of the multiple mechanisms of TNF-alpha-induced apoptosis in liver injury. J. Cell. Mol. Med. 8 (4), 445–454. doi:10.1111/j.1582-4934.2004.tb00469.x
Du, J., Niu, X., Wang, Y., Kong, L., Wang, R., Zhang, Y., et al. (2015). MiR-146a-5p suppresses activation and proliferation of hepatic stellate cells in nonalcoholic fibrosing steatohepatitis through directly targeting Wnt1 and Wnt5a. Sci. Rep. 5 (1), 16163. doi:10.1038/srep16163
El-Nabi, S. H., Elhiti, M., and El-Sheekh, M. (2020). A new approach for COVID-19 treatment by micro-RNA. Med. hypotheses 143, 110203. doi:10.1016/j.mehy.2020.110203
Eslam, M., Sanyal, A. J., George, J., Sanyal, A., Neuschwander-Tetri, B., Tiribelli, C., et al. (2020). Mafld: A consensus-driven proposed nomenclature for metabolic associated fatty liver disease. Gastroenterology 158 (7), 1999–2014.e1. doi:10.1053/j.gastro.2019.11.312
Ferreira-Gomes, M., Kruglov, A., Durek, P., Heinrich, F., Tizian, C., Heinz, G. A., et al. (2021). SARS-CoV-2 in severe COVID-19 induces a TGF-β-dominated chronic immune response that does not target itself. Nat. Commun. 12 (1), 1961. doi:10.1038/s41467-021-22210-3
Fuhrmeister, J., Zota, A., Sijmonsma, T. P., Seibert, O., Cıngır, Ş., Schmidt, K., et al. (2016). Fasting-induced liver GADD 45β restrains hepatic fatty acid uptake and improves metabolic health. EMBO Mol. Med. 8 (6), 654–669. doi:10.15252/emmm.201505801
Gao, Y., Li, H., Ma, X., Fan, Y., Ni, D., Zhang, Y., et al. (2017). KLF6 suppresses metastasis of clear cell renal cell carcinoma via transcriptional repression of E2F1. Cancer Res. 77 (2), 330–342. doi:10.1158/0008-5472.CAN-16-0348
Ghoneim, S., Butt, M. U., Hamid, O., Shah, A., and Asaad, I. (2020). The incidence of COVID-19 in patients with metabolic syndrome and non-alcoholic steatohepatitis: A population-based study. Metab. Open 8, 100057. doi:10.1016/j.metop.2020.100057
Ghorpade, D. S., Sinha, A. Y., Holla, S., Singh, V., and Balaji, K. N. (2013). NOD2-nitric oxide-responsive microRNA-146a activates Sonic hedgehog signaling to orchestrate inflammatory responses in murine model of inflammatory bowel disease. J. Biol. Chem. 288 (46), 33037–33048. doi:10.1074/jbc.M113.492496
Gottlieb, A., and Canbay, A. (2019). Why bile acids are so important in non-alcoholic fatty liver disease (NAFLD) progression. Cells 8 (11), 1358. doi:10.3390/cells8111358
Grattagliano, I., de Bari, O., Bernardo, T. C., Oliveira, P. J., Wang, D. Q., and Portincasa, P. (2012). Role of mitochondria in nonalcoholic fatty liver disease--from origin to propagation. Clin. Biochem. 45 (9), 610–618. doi:10.1016/j.clinbiochem.2012.03.024
Guan, W. J., Ni, Z. Y., Hu, Y., Liang, W. H., Ou, C. Q., He, J. X., et al. (2020). Clinical characteristics of coronavirus disease 2019 in China. N. Engl. J. Med. 382 (18), 1708–1720. doi:10.1056/NEJMoa2002032
Guo, W., Li, M., Dong, Y., Zhou, H., Zhang, Z., Tian, C., et al. (2020). Diabetes is a risk factor for the progression and prognosis of COVID-19. Diabetes Metab. Res. Rev. 36 (7), e3319. doi:10.1002/dmrr.3319
Gupta, A., Madhavan, M. V., Sehgal, K., Nair, N., Mahajan, S., Sehrawat, T. S., et al. (2020). Extrapulmonary manifestations of COVID-19. Nat. Med. 26 (7), 1017–1032. doi:10.1038/s41591-020-0968-3
Guterres, A., de Azeredo Lima, C. H., Miranda, R. L., and Gadelha, M. R. (2020). What is the potential function of microRNAs as biomarkers and therapeutic targets in COVID-19? Infect. Genet. Evol. 85, 104417. doi:10.1016/j.meegid.2020.104417
Hasenfuss, S. C., Bakiri, L., Thomsen, M. K., Williams, E. G., Auwerx, J., and Wagner, E. F. (2014). Regulation of steatohepatitis and PPARγ signaling by distinct AP-1 dimers. Cell Metab. 19 (1), 84–95. doi:10.1016/j.cmet.2013.11.018
Hashemi, N., Viveiros, K., Redd, W. D., Zhou, J. C., McCarty, T. R., Bazarbashi, A. N., et al. (2020). Impact of chronic liver disease on outcomes of hospitalized patients with COVID-19: A multicentre United States experience. Liver Int. 40 (10), 2515–2521. doi:10.1111/liv.14583
Hoffmann, E., Ashouri, J., Wolter, S., Doerrie, A., Dittrich-Breiholz, O., Schneider, H., et al. (2008). Transcriptional regulation of EGR-1 by the interleukin-1-JNK-MKK7-c-Jun pathway. J. Biol. Chem. 283 (18), 12120–12128. doi:10.1074/jbc.M800583200
Hsu, L.-S., Huang, R.-H., Lai, H.-W., Hsu, H.-T., Sung, W.-W., Hsieh, M.-J., et al. (2017). KLF6 inhibited oral cancer migration and invasion via downregulation of mesenchymal markers and inhibition of MMP-9 activities. Int. J. Med. Sci. 14 (6), 530–535. doi:10.7150/ijms.19024
Huang, C., Wang, Y., Li, X., Ren, L., Zhao, J., Hu, Y., et al. (2020). Clinical features of patients infected with 2019 novel coronavirus in Wuhan, China. lancet 395 (10223), 497–506. doi:10.1016/S0140-6736(20)30183-5
Huang, X., Li, X., and Guo, B. (2008). KLF6 induces apoptosis in prostate cancer cells through up-regulation of ATF3. J. Biol. Chem. 283 (44), 29795–29801. doi:10.1074/jbc.M802515200
Ianevski, A., Zusinaite, E., Kuivanen, S., Strand, M., Lysvand, H., Teppor, M., et al. (2018). Novel activities of safe-in-human broad-spectrum antiviral agents. Antivir. Res. 154, 174–182. doi:10.1016/j.antiviral.2018.04.016
Ito, G., Uchiyama, M., Kondo, M., Mori, S., Usami, N., Maeda, O., et al. (2004). Kruppel-like factor 6 is frequently down-regulated and induces apoptosis in non-small cell lung cancer cells. Cancer Res. 64 (11), 3838–3843. doi:10.1158/0008-5472.CAN-04-0185
Jang, Y., Shin, J. S., Lee, M. K., Jung, E., An, T., Kim, U. I., et al. (2021). Comparison of antiviral activity of gemcitabine with 2'-fluoro-2'-deoxycytidine and combination therapy with remdesivir against SARS-CoV-2. Int. J. Mol. Sci. 22 (4), 1581. doi:10.3390/ijms22041581
Ji, D., Qin, E., Xu, J., Zhang, D., Cheng, G., Wang, Y., et al. (2020). Non-alcoholic fatty liver diseases in patients with COVID-19: A retrospective study. J. hepatology 73 (2), 451–453. doi:10.1016/j.jhep.2020.03.044
Karki, R., Sharma, B. R., Tuladhar, S., Williams, E. P., Zalduondo, L., Samir, P., et al. (2021). Synergism of TNF-α and IFN-γ triggers inflammatory cell death, tissue damage, and mortality in SARS-CoV-2 infection and cytokine shock syndromes. Cell 184 (1), 149–168.e17. doi:10.1016/j.cell.2020.11.025
Khan, M. A.-A.-K., Sany, M. R. U., Islam, M. S., and Islam, A. B. M. M. K. (2020). Epigenetic regulator miRNA pattern differences among SARS-CoV, SARS-CoV-2, and SARS-CoV-2 world-wide isolates delineated the mystery behind the epic pathogenicity and distinct clinical characteristics of pandemic COVID-19. Front. Genet. 11, 765. doi:10.3389/fgene.2020.00765
Kudva, A., Scheller, E. V., Robinson, K. M., Crowe, C. R., Choi, S. M., Slight, S. R., et al. (2011). Influenza A inhibits Th17-mediated host defense against bacterial pneumonia in mice. J. Immunol. 186 (3), 1666–1674. doi:10.4049/jimmunol.1002194
Li, J., and Fan, J.-G. (2020). Characteristics and mechanism of liver injury in 2019 coronavirus disease. J. Clin. Transl. hepatology 8 (1), 13–17. doi:10.14218/JCTH.2020.00019
Li, Z., Yu, P., Wu, J., Tao, F., and Zhou, J. (2019). Transcriptional regulation of early growth response gene-1 (EGR1) is associated with progression of nonalcoholic fatty liver disease (NAFLD) in patients with insulin resistance. Med. Sci. Monit. 25, 2293–3004. doi:10.12659/msm.914044
Ludwig, J., Viggiano, T. R., Mcgill, D. B., and Oh, B. (1980). Nonalcoholic steatohepatitis: Mayo Clinic experiences with a hitherto unnamed disease. Mayo Clin. Proc. 8, 434–438.
Maares, M., and Haase, H. (2016). Zinc and immunity: An essential interrelation. Arch. Biochem. Biophys. 611, 58–65. doi:10.1016/j.abb.2016.03.022
Magee, N. S., and Zhang, Y. (2018). Hepatocyte early growth response 1 (EGR1) regulates lipid metabolism in nonalcoholic fatty liver disease. FASEB J. 32, 670–656. doi:10.1096/fasebj.2018.32.1_supplement.670.56
Mariani, E., Cattini, L., Neri, S., Malavolta, M., Mocchegiani, E., Ravaglia, G., et al. (2006). Simultaneous evaluation of circulating chemokine and cytokine profiles in elderly subjects by multiplex technology: Relationship with zinc status. Biogerontology 7 (5-6), 449–459. doi:10.1007/s10522-006-9060-8
McCarron, S., Bathon, B., Conlon, D. M., Abbey, D., Rader, D. J., Gawronski, K., et al. (2021). Functional characterization of organoids derived from irreversibly damaged liver of patients with NASH. Hepatology 74 (4), 1825–1844. doi:10.1002/hep.31857
Méndez-Sánchez, N., Valencia-Rodríguez, A., Qi, X., Yoshida, E. M., Romero-Gómez, M., George, J., et al. (2020). What has the COVID-19 pandemic taught us so far? Addressing the problem from a hepatologist’s perspective. J. Clin. Transl. hepatology 8 (2), 0024. doi:10.14218/JCTH.2020.00024
Mortazavi-Jahromi, S. S., Ahmadzadeh, A., Rezaieyazdi, Z., Aslani, M., Omidian, S., and Mirshafiey, A. (2020b). The role of β-d-mannuronic acid, as a new non-steroidal anti-inflammatory drug on expression of miR-146a, IRAK1, TRAF6, NF-κB and pro-inflammatory cytokines following a clinical trial in rheumatoid arthritis patients. Immunopharmacol. Immunotoxicol. 42 (3), 228–236. doi:10.1080/08923973.2020.1742734
Mortazavi-Jahromi, S. S., Aslani, M., and Mirshafiey, A. (2020a). A comprehensive review on miR-146a molecular mechanisms in a wide spectrum of immune and non-immune inflammatory diseases. Immunol. Lett. 227, 8–27. doi:10.1016/j.imlet.2020.07.008
Narla, G., Heath, K. E., Reeves, H. L., Li, D., Giono, L. E., Kimmelman, A. C., et al. (2001). KLF6, a candidate tumor suppressor gene mutated in prostate cancer. Science 294 (5551), 2563–2566. doi:10.1126/science.1066326
Nersisyan, S., Engibaryan, N., Gorbonos, A., Kirdey, K., Makhonin, A., and Tonevitsky, A. (2020). Potential role of cellular miRNAs in coronavirus-host interplay. PeerJ 8, e9994. doi:10.7717/peerj.9994
O'Connell, R. M., Taganov, K. D., Boldin, M. P., Cheng, G., and Baltimore, D. (2007). MicroRNA-155 is induced during the macrophage inflammatory response. Proc. Natl. Acad. Sci. 104 (5), 1604–1609. doi:10.1073/pnas.0610731104
Oakley, F., Trim, N., Constandinou, C. M., Ye, W., Gray, A. M., Frantz, G., et al. (2003). Hepatocytes express nerve growth factor during liver injury: Evidence for paracrine regulation of hepatic stellate cell apoptosis. Am. J. Pathol. 163 (5), 1849–1858. doi:10.1016/s0002-9440(10)63544-4
Portincasa, P., Krawczyk, M., Smyk, W., Lammert, F., and Di Ciaula, A. (2020). COVID-19 and non-alcoholic fatty liver disease: Two intersecting pandemics. Eur. J. Clin. Invest. 50 (10), e13338. doi:10.1111/eci.13338
Prasad, K., Alasmari, A. F., Ali, N., Khan, R., Alghamdi, A., and Kumar, V. (2021). Insights into the SARS-CoV-2-mediated alteration in the stress granule protein regulatory networks in humans. Pathogens 10 (11), 1459. doi:10.3390/pathogens10111459
Price-Haywood, E. G., Burton, J., Fort, D., and Seoane, L. (2020). Hospitalization and mortality among black patients and white patients with Covid-19. N. Engl. J. Med. 382 (26), 2534–2543. doi:10.1056/NEJMsa2011686
Qi, M., Liu, B., Li, S., Ni, Z., and Li, F. (2021). Construction and investigation of competing endogenous RNA networks and candidate genes involved in SARS-CoV-2 infection. Int. J. Gen. Med. 14, 6647–6659. doi:10.2147/ijgm.S335162
Quiñones-Lombraña, A., and Blanco, J. G. (2015). Chromosome 21-derived hsa-miR-155-5p regulates mitochondrial biogenesis by targeting Mitochondrial Transcription Factor A (TFAM). Biochim. Biophys. Acta 1852 (7), 1420–1427. doi:10.1016/j.bbadis.2015.04.004
Rismanbaf, A., and Zarei, S. (2020). Liver and kidney injuries in COVID-19 and their effects on drug therapy; a letter to editor. Archives Acad. Emerg. Med. 8 (1), e17.
Russell, B., Moss, C., George, G., Santaolalla, A., Cope, A., Papa, S., et al. (2020). Associations between immune-suppressive and stimulating drugs and novel COVID-19-a systematic review of current evidence. Ecancermedicalscience 14, 1022. doi:10.3332/ecancer.2020.1022
Sabatino, M. E., Castellaro, A., Racca, A. C., Carbajosa González, S., Pansa, M. F., Soria, G., et al. (2019). Krüppel-like factor 6 is required for oxidative and oncogene-induced cellular senescence. Front. Cell Dev. Biol. 7, 297. doi:10.3389/fcell.2019.00297
Saeedi-Boroujeni, A., and Mahmoudian-Sani, M. R. (2021). Anti-inflammatory potential of Quercetin in COVID-19 treatment. J. Inflamm. (Lond) 18 (1), 3. doi:10.1186/s12950-021-00268-6
Sajjadi, S., Hejazi, S., Ravanshad, S., and Jafarzadeh Esfehani, R. (2022). Human T-lymphotropic virus type 1 and novel coronavirus disease 2019; More complex than just a simple coinfection. Gene 834, 146550. doi:10.1016/j.gene.2022.146550
Sangodkar, J., Shi, J., DiFeo, A., Schwartz, R., Bromberg, R., Choudhri, A., et al. (2009). Functional role of the KLF6 tumour suppressor gene in gastric cancer. Eur. J. Cancer 45 (4), 666–676. doi:10.1016/j.ejca.2008.11.009
Sardar, R., Satish, D., and Gupta, D. (2020). Identification of novel SARS-CoV-2 drug targets by host MicroRNAs and transcription factors Co-regulatory interaction network analysis. Front. Genet. 11, 571274. doi:10.3389/fgene.2020.571274
Sheng, J., Chen, W., and Zhu, H. J. (2015). The immune suppressive function of transforming growth factor-β (TGF-β) in human diseases. Growth factors. 33 (2), 92–101. doi:10.3109/08977194.2015.1010645
Su, H.-Y., and Hsu, Y.-C. (2021). Patients with cirrhosis during the COVID-19 pandemic: Current evidence and future perspectives. World J. Clin. Cases 9 (13), 2951–2968. doi:10.12998/wjcc.v9.i13.2951
Su, T.-H., and Kao, J.-H. (2020). The clinical manifestations and management of COVID-19-related liver injury. J. Formos. Med. Assoc. 119 (6), 1016–1018. doi:10.1016/j.jfma.2020.04.020
Takada, Y., Gresh, L., Bozec, A., Ikeda, E., Kamiya, K., Watanabe, M., et al. (2011). Interstitial lung disease induced by gefitinib and toll-like receptor ligands is mediated by Fra-1. Oncogene 30 (36), 3821–3832. doi:10.1038/onc.2011.101
Tian, J., and Locker, J. (2013). Gadd45 in the liver: Signal transduction and transcriptional mechanisms. Gadd45 Stress Sens. Genes 793, 69–80. doi:10.1007/978-1-4614-8289-5_5
Trizzino, M., Zucco, A., Deliard, S., Wang, F., Barbieri, E., Veglia, F., et al. (2021). EGR1 is a gatekeeper of inflammatory enhancers in human macrophages. Sci. Adv. 7 (3), eaaz8836. doi:10.1126/sciadv.aaz8836
Tsai, M. S., Lin, Y. C., Sun, C. K., Huang, S. C., Lee, P. H., and Kao, Y. H. (2014). Up-regulation of nerve growth factor in cholestatic livers and its hepatoprotective role against oxidative stress. PLoS One 9 (11), e112113. doi:10.1371/journal.pone.0112113
Vaz, M., Reddy, N. M., Rajasekaran, S., and Reddy, S. P. (2012). Genetic disruption of Fra-1 decreases susceptibility to endotoxin-induced acute lung injury and mortality in mice. Am. J. Respir. Cell Mol. Biol. 46 (1), 55–62. doi:10.1165/rcmb.2011-0169OC
Wang, D., Hu, B., Hu, C., Zhu, F., Liu, X., Zhang, J., et al. (2020). Clinical characteristics of 138 hospitalized patients with 2019 novel coronavirus–infected pneumonia in Wuhan, China. jama 323 (11), 1061–1069. doi:10.1001/jama.2020.1585
Wicik, Z., Eyileten, C., Jakubik, D., Simões, S. N., Martins, D. C., Pavão, R., et al. (2020). ACE2 interaction networks in COVID-19: A physiological framework for prediction of outcome in patients with cardiovascular risk factors. J. Clin. Med. 9 (11), 3743. doi:10.3390/jcm9113743
Woodson, C. M., and Kehn-Hall, K. (2022). Examining the role of EGR1 during viral infections. Front. Microbiol. 13, 1020220. doi:10.3389/fmicb.2022.1020220
Xu, C., He, X., Zheng, Z., Zhang, Z., Wei, C., Guan, K., et al. (2014). Downregulation of microRNA miR-526a by enterovirus inhibits RIG-I-dependent innate immune response. J. virology 88 (19), 11356–11368. doi:10.1128/JVI.01400-14
Younossi, Z., Anstee, Q. M., Marietti, M., Hardy, T., Henry, L., Eslam, M., et al. (2018). Global burden of NAFLD and NASH: Trends, predictions, risk factors and prevention. Nat. Rev. Gastroenterology hepatology 15 (1), 11–20. doi:10.1038/nrgastro.2017.109
Yuan, L., Fung, T. S., He, J., Chen, R. A., and Liu, D. X. (2022). Modulation of viral replication, apoptosis and antiviral response by induction and mutual regulation of EGR and AP-1 family genes during coronavirus infection. Emerg. Microbes Infect. 11 (1), 1717–1729. doi:10.1080/22221751.2022.2093133
Zhang, Y. N., Zhang, Q. Y., Li, X. D., Xiong, J., Xiao, S. Q., Wang, Z., et al. (2020). Gemcitabine, lycorine and oxysophoridine inhibit novel coronavirus (SARS-CoV-2) in cell culture. Emerg. Microbes Infect. 9 (1), 1170–1173. doi:10.1080/22221751.2020.1772676
Keywords: coronavirus disease 2019, non-alcoholic steatohepatitis, hub genes, network analysis, bioinformatics
Citation: Lu H, Ma J, Li Y, Zhang J, An Y, Du W and Cai X (2023) Bioinformatic and systems biology approach revealing the shared genes and molecular mechanisms between COVID-19 and non-alcoholic hepatitis. Front. Mol. Biosci. 10:1164220. doi: 10.3389/fmolb.2023.1164220
Received: 12 February 2023; Accepted: 01 June 2023;
Published: 19 June 2023.
Edited by:
Ornella Cominetti, Nestlé Research Center, SwitzerlandReviewed by:
Alireza Tabibzadeh, Iran University of Medical Sciences, IranPartha Chattopadhyay, Council of Scientific and Industrial Research (CSIR), India
Copyright © 2023 Lu, Ma, Li, Zhang, An, Du and Cai. This is an open-access article distributed under the terms of the Creative Commons Attribution License (CC BY). The use, distribution or reproduction in other forums is permitted, provided the original author(s) and the copyright owner(s) are credited and that the original publication in this journal is cited, in accordance with accepted academic practice. No use, distribution or reproduction is permitted which does not comply with these terms.
*Correspondence: Xuefei Cai, caixuefei@cqmu.edu.cn