- 1Department of Plastic and Reconstructive Surgery, Xijing Hospital, Fourth Military Medical University, Xi’an, China
- 2Department of Stomatology, Zhongnan Hospital of Wuhan University, Wuhan, China
- 3Department of Clinical Oncology, Xijing Hospital, Fourth Military Medical University, Xi’an, China
Elevated polyamine levels are required for tumor transformation and development; however, expression patterns of polyamines and their diagnostic potential have not been investigated in oral squamous cell carcinoma (OSCC), and its impact on prognosis has yet to be determined. A total of 440 OSCC samples and clinical data were obtained from The Cancer Genome Atlas (TCGA) and Gene Expression Omnibus (GEO). Consensus clustering was conducted to classify OSCC patients into two subgroups based on the expression of the 17 polyamine regulators. Polyamine-related differentially expressed genes (PARDEGs) among distinct polyamine clusters were determined. To create a prognostic model, PARDEGs were examined in the training cohorts using univariate-Lasso-multivariate Cox regression analyses. Six prognostic genes, namely, “CKS2,” “RIMS3,” “TRAC,” “FMOD,” CALML5,” and “SPINK7,” were identified and applied to develop a predictive model for OSCC. According to the median risk score, the patients were split into high-risk and low-risk groups. The predictive performance of the six gene models was proven by the ROC curve analysis of the training and validation cohorts. Kaplan–Meier curves revealed that the high-risk group had poorer prognosis. Furthermore, the low-risk group was more susceptible to four chemotherapy drugs according to the IC50 of the samples computed by the “pRRophetic” package. The correlation between the risk scores and the proportion of immune cells was calculated. Meanwhile, the tumor mutational burden (TMB) value of the high-risk group was higher. Real-time quantitative polymerase chain reaction was applied to verify the genes constructing the model. The possible connections of the six genes with various immune cell infiltration and therapeutic markers were anticipated. In conclusion, we identified a polyamine-related prognostic signature, and six novel biomarkers in OSCC, which may provide insights to identify new treatment targets for OSCC.
1 Introduction
The three primary polyamines, namely, putrescine, spermidine, and spermine (McNamara et al., 2021), are plentiful both inside the cell and outside it. Polyamines are multifunctional polycations which are necessary for practically all living things (Novita Sari et al., 2021). Polyamines, because of their cationic nature, could interact with macromolecules such as DNA, RNA, phospholipids, and proteins to increase gene regulation via epigenetic and chromatin structural change, mRNA structure stabilization, cell growth, proliferation and differentiation, and ion channel regulation (Pegg, 2009; Murray-Stewart et al., 2018). It is used by cells throughout the body to support growth, and microorganisms can interact with polyamines to reduce the expression of pro-inflammatory genes (Holbert et al., 2022). The downstream of numerous significant carcinogenic pathways is the polyamine metabolism (Casero et al., 2018). The polyamine pathway is a viable target for anti-cancer treatment since it is frequently dysregulated in cancer. Additionally, when polyamine production is downregulated by pharmacological and genetic techniques, it will cause cancer cells to senescence and undergo apoptosis (Minois et al., 2011). It may be argued that polyamine levels could serve as a cancer marker because they promote proliferation as well (McNamara et al., 2021), and the increased demand of tumor cells for polyamines, to some extent, shows that targeted polyamines are important strategies in cancer treatments. Polyamines and polyamine metabolites measured under humoral environment have shown potential as biomarkers for different cancers (Liu et al., 2018; Nakajima et al., 2018). At present, polyamine inhibitors and analogs are effective for cancer in experimental animal models and in clinic; for example, α-difluoromethylornithine (DFMO) was evaluated as a potential combined cancer therapy drug (Holbert et al., 2022). For another example, spermidine and spermine that have been reported could stimulate apoptosis of macrophages stimulated by Helicobacter pylori (Chaturvedi et al., 2004), and DFMO could inhibit the apoptosis (Gobert et al., 2002). In various malignancies, the involvement of polyamines in the development of cancer has been demonstrated. Colorectal cancer (Snezhkina et al., 2016) is regulated by the oncogenic transcription factors c-MYC and C/EBP β via a number of important enzymes in the polyamine metabolic pathway. Ornithine decarboxylase (ODC) has been identified as an effective carcinogenic transformation factor, which is the most effective transcriptional target in neuroblastoma (Lu et al., 2003). Polyamines are abundant in breast tissue and can promote cell proliferation by promoting growth factor receptors (Çelik et al., 2017). In prostate cancer, the concentration of spermine is related to the malignant grade of cancer (Tsoi et al., 2016), and it becomes a biomarker of the malignant degree of cancer.
At present, studies on polyamines and oral cancer focus on the detection of the saliva metabolism. Japanese scholars have characterized the metabolic changes in patients' saliva samples (Ohshima et al., 2017) among the potential 25 metabolites, and the polyamine metabolism showed significant changes. In the study of Ishikawa et al. (2019), salivary ornithine can be utilized to distinguish between oral epithelial dysplasia and oral squamous cell cancer as an intermediate metabolite in the urea cycle, which is a well-known metabolic marker of various tumors (Nijakowski et al., 2022). These recently discovered polyamine-related genes may aid in the development of an improved mechanistic comprehension of OSCC, which may provide a productive insight to the clinical application of the condition.
In our study, we combined genomic data from 420 OSCC samples from TCGA and GEO datasets. We discovered two distinct polyamine modification patterns and found that the immune cell infiltration is different in clusters, indicating that the modification played a vital role in shaping individual tumor microenvironment characterizations. Furthermore, we developed a scoring model to assess individual tumor polyamine alteration patterns and predict patients' clinical response to chemical therapy (Figure 1). These findings showed that polyamine alteration is critical in establishing various tumor immune microenvironment profiles and driving therapeutic intervention approaches for oral squamous cell carcinoma.
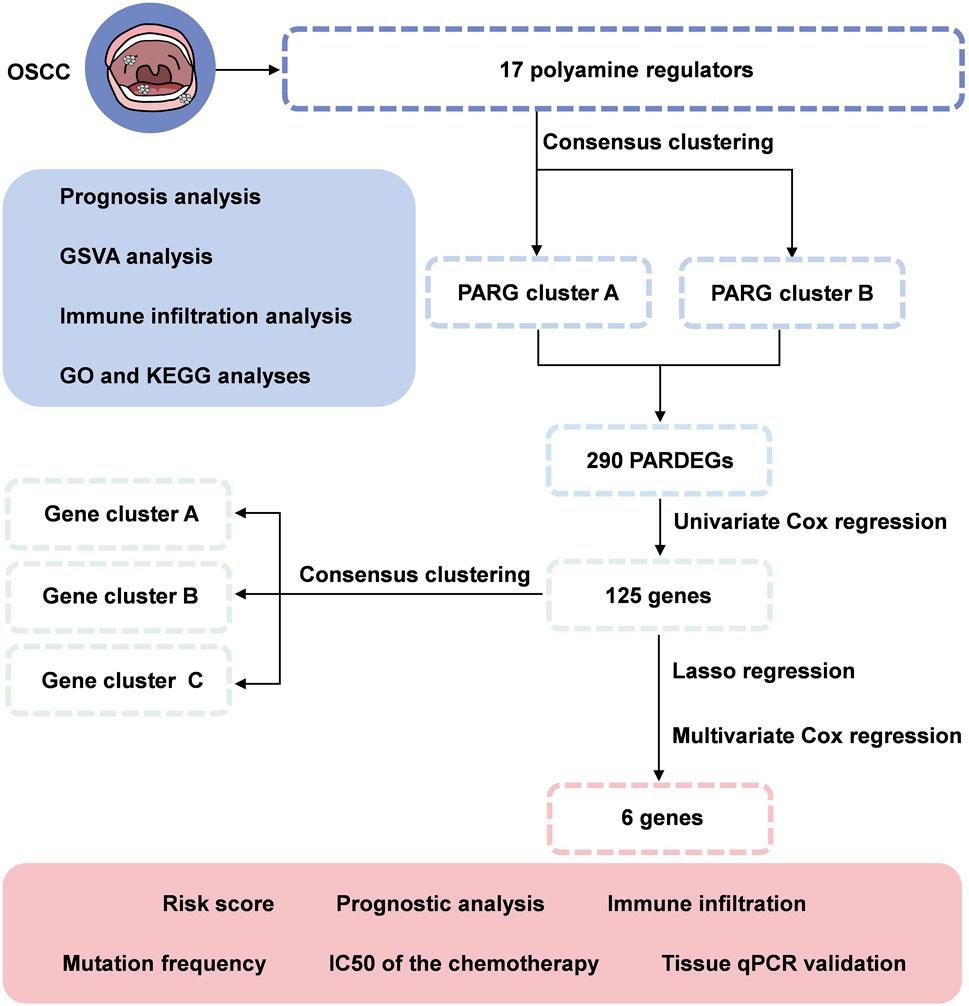
FIGURE 1. Flowchart of identifying a polyamine-related signature and six novel prognostic biomarkers in oral squamous cell carcinoma.
2 Materials and methods
2.1 Data collection
We obtained the sequence data for 323 samples with OSCC from TCGA and 97 samples from the NCBI GEO database (GSE41613). A total of 17 polyamine signatures were extracted from Holbert’s research (Holbert et al., 2022) and four gene sets in the Gene Set Enrichment Analysis database as follow: GOBP_REGULATION_OF_POLYAMINE_TRANSMEMBRANE_TRANSPORT, GOBP_PUTRESCINE_METABOLIC_PROCESS, GOBP_PUTRESCINE_BIOSYNTHETIC_PROCESS, and GOBP_POLYAMINE_TRANSMEMBRANE_TRANSPORT.
2.2 Consensus clustering analysis of 17 polyamine regulators
We chose to investigate potential molecular subgroups by using the “ConsensusClusterPlus” R package (Wilkerson and Hayes, 2010) to perform consensus clustering analysis to identify distinct polyamine modification patterns based on the expression of 17 polyamine regulators (AGMAT, AMD1, ATP13A2, AZIN1, AZIN2, OAZ1, OAZ2, OAZ3, ODC1, AOC1, PAOX, SAT1, SAT2, SMOX, ARG1, SRM, and SMS).
2.3 Functional analyses
To identify differently expressed genes in distinct groups, the “limma” package in R was used. The additional verification of the GO processes in BP, CC, MF, and KEGG pathways was associated with signature analysis for the differentially expressed genes in the cluster; the “clusterProfiler” package in R (Yu et al., 2012) was applied in each sample with a statistical threshold of p < 0.05.
2.4 Tumor mutational burden and immune cell infiltration
Patients' single-nucleotide variant data were used to estimate the tumor mutational burden (TMB). The single sample gene set enrichment analysis (ssGSEA) algorithms assessed the 28 immune cells infiltration in the OSCC microenvironment.
2.5 Differentially expressed genes identified between unique polyamine modification patterns
Our previous consensus clustering algorithm classified patients into two distinct polyamine modification patterns, and polyamine-related differentially expressed genes (PARDEGs) among distinct polyamine phenotypes were determined. R package was applied to assess PARDEGs in OSCC samples among different clusters. Gene expression data were normalized to calculate the differentially expressed statistics. The significance filtering criteria of PARDEGs were set as an adjusted value of p < 0.05.
2.6 Polyamine-related risk signature construction and validation
In order to create an optimal polyamine-related risk signature based on linear integration of the regression coefficient obtained and the expression level of the chosen genes, we generated a polyamine-related score pattern using LASSO regression with the “glmnet” R package using intersecting genes from the public datasets. The risk score was computed as follows:
2.7 Survival analysis
To compare the OS of different clusters of OSCC patients, the Kaplan–Meier analysis and the “survminer” programs were utilized. The “survivalROC” test was used to create ROC curves in order to assess the accuracy of the risk signatures in predicting the outcomes of OSCC patients. The bigger the AUC, the better the risk model’s prediction power is.
2.8 Quantitative real-time PCR
Total RNA extraction using TRIzol reagent (Servicebio, Wuhan, China) was performed as previously described (Wu et al., 2022). Total RNA was then converted to a first-strand cDNA by ReverTra Ace (Toyobo, Tokyo, Japan) and subjected to qRT-PCR (Bio-Rad CFX96, America) with ChamQ Universal SYBR qPCR Master Mix (Vazyme Biotech, Nanjing, China). In total, three duplicates of each sample were analyzed. Once the expression levels of GAPDH were analyzed, the results were calculated in 2^-ΔΔCt. The target genes' primer sequences are included in Supplementary Table S3.
2.9 Drug sensitivity and interactions
Furthermore, we used the R package “pRRophetic” (Geeleher et al., 2014) to assess each OSCC patient’s therapy response, which is based on the 50% inhibitory concentration (IC50) acquired from the Genomics of Drug Sensitivity in Cancer (GDSC) website.
2.10 Statistical analysis
The data were analyzed by R software (https://www.r-project.org/). R packages (ESTIMATE, glmnet, ggplot2, GSVA, limma, survminer, and survival) were applied for data analysis and graph plotting. The median value of tumor purity or risk scores was treated as the cutoff value for the two subgroups. The qPCR results were analyzed by GraphPad (version 9.0.0). In order to compare the statistical differences between the two groups, the Student’s t-test was employed. When there were more than two groups, Kruskal–Wallis and one-way ANOVA tests were used. The p-value was always two-sided; a value of p < 0.05 was considered statistically different (*, p < 0.05; **, p < 0.01; ***, p < 0.001).
3 Results
3.1 Outline of polyamine-related gene expression changes in OSCC and a PPI network
We first investigated the expression of 17 polyamine regulators (AGMAT, AMD1, ATP13A2, AZIN1, AZIN2, OAZ1, OAZ2, OAZ3, ODC1, AOC1, PAOX, SAT1, SAT2, SMOX, ARG1, SRM, and SMS) in OSCC patients. The results showed that most polyamine regulators were expressed higher in neoplastic tissues in OSCC patients than in their normal tissues. However, ARG1 had low expression in cancer tissues and high expression in normal tissues (Figure 2A). The mutation analysis of OSCC patients showed that only 4.74% of 506 samples had polyamine regulator gene alterations. The mutations frequency was concentrated in ODC1, AOC1, SAT1, and AMD1, most of which were missense mutations (Figure 2B).
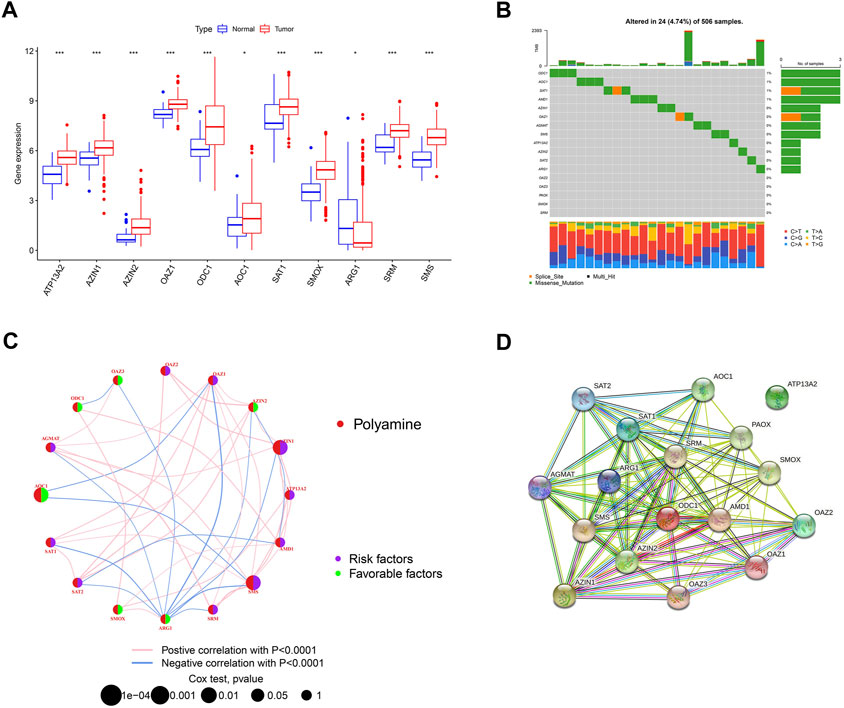
FIGURE 2. Overview of genetic and prognostic information of polyamine regulators in OSCC. (A) Boxplot of 17 polyamine regulators expression in OSCC and its adjacent normal tissue. (B) Waterfall plot of the polyamine regulators altered in OSCC samples. (C) Interaction of the polyamine regulators. Size of each cell represents the survival effect of each gene. Red represents a positive correlation, whereas blue indicates a negative correlation. (D) PPI network map showed the interaction of the 17 polyamine regulators.
We made a correlation analysis on these polyamine genes to see whether they interact with each other. The regulator network depicted the complete picture of the interactions of the 17 polyamine regulators, the regulator interconnections, and their prognostic value in OSCC patients. Results in Figure 1C show that 6/17 of the polyamine regulators are favorable factors, and among them, ARG1 has the most abundant blue lines (representing negative correlation), which shows its regulatory importance in tumors. In addition, AMD1, AZ1N1, ARG1, and OAZ1 have the most related lines with other genes, indicating that cross-talk among the regulators plays a role in regulation (Figure 2C). A PPI (protein–protein interaction) network shows the interaction between proteins encoded by polyamine genes. ATP13A2 has no interaction with other proteins but has connections with other genes in the correlation network, suggesting that there may still be proteins encoded by intermediate genes that have not been included in tumor development. For others, pink lines between SRM and ODC1, AMD1 and AZIN2, etc., indicated that there has been experimental verification of interaction (experimentally determined). Most of the gene connections of polyamines are still emerald green (text mining), which means they have research potential and need to be further explored (Figure 2D).
3.2 Polyamine modification patterns characterized by gene expression
Based on the hypotheses of polyamine regulators with OSCC progression, we identified two distinct polyamine-related gene (PARG) clusters (Figure 3A; Supplementary Figures S1A–J); thus, we divided all patients into cluster PARG-A (n = 221) and PARG-B (n = 199) by consensus clustering. PARG-A exhibited the worst prognosis, whereas PARG-B had a prominent survival advantage (Figure 3B).
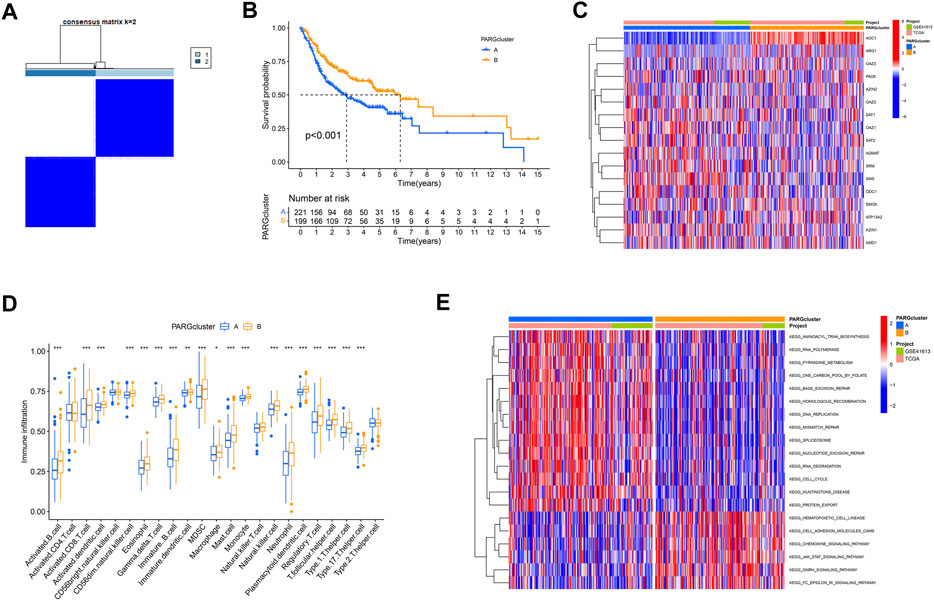
FIGURE 3. Construction of two polyamine-related gene (PARG) clusters in OSCC patients. (A) Consensus clustering of OSCC patients. (B) OS curves of OSCC patients in two PARG clusters. (C) Heatmap of 17 polyamine regulator expression in two clusters. (D) Quantity of immunological infiltration cells as determined by ssGSEA between PARG clusters. (E) Heatmap of GSVA in the KEGG pathway between PARG clusters.
After the differential analysis of all polyamine-related genes (PARGs), heatmap showed that AOC1 showed low expression in PARG-B with poor prognosis, and its high expression in PARG-A was helpful to the prognosis of OSCCs. Also, although the expression of an ARG1 gene is lower than that of other PARGs in OSCC patients (Figure 2A), it can still be seen that its high expression indicates a better prognosis (Figure 3C).
3.3 Immune cell infiltration and pathways in two PARG clusters
We also analyzed the differences between the two clusters of immune infiltrating cells. We used ssGSEA to create a boxplot to visualize and compare the 23 immune infiltrating cell subtypes among distinct clusters (Figure 3D). Compared to PARG-A, the expression of most lymphocytes and myeloid cells in PARG-B was higher, such as activated CD8+ T cells, T follicular helper cell, and Type 1/17 T helper cells. Natural killer cells (NK cells), which are considered to be the next leading role of cellular immunotherapy (Laskowski et al., 2022), have higher infiltration in PARG-B with better prognosis.
Moreover, we used GSVA to explore the biological molecular changes of PARG-A and B. As shown in the result in the heatmap (Figure 3E), PARG-A has a poor prognosis, concentrated in metabolic-related pathways, such as pyrimidine metabolism, one carbon pool by folate, aminoacyl-tRNA biosynthesis, and RNA polymerase. PARG-B is concentrated in a chemokine signaling pathway. Chemokines are small molecular weight cytokines, whose main role is to recruit leukocyte subsets under stable and pathological conditions, that transmit cell signals after binding with the chemokine receptor, which are expressed on the cell surface. Cell adhesion molecule cam has the function of maintaining the normal tissue structure, regulating immune response and inflammatory response, and is also highly expressed in PARG-B.
Based on our findings, the two polyamine modification patterns had distinct immune infiltration characteristics.
3.4 GO term and the KEGG pathway analyses
Despite the fact that the consensus clustering technique based on PARG expression categorized OSCC patients into two polyamine modification phenotypes, the underlying genetic modifications and expression perturbations within these phenotypes remained unknown. Based on these questions, we investigated the possible polyamine-related transcriptional expression changes in OSCC across two polyamine modification types. We identified 289 polyamine-related differentially expressed genes (PARDEGs) (Supplementary Table S1) between the two PARG clusters.
The Gene Ontology (GO) and Kyoto Encyclopedia of Genes and Genomes (KEGG) analyses of PARDEGs were carried out. The biological process (BP) (Figure 4A) of PARDEGs was concentrated in the occurrence and development of epithelial development. At the same time, it was concentrated in the human immune response, acute human response, and other immune-related pathways. The most abundant cellular component (CC) influenced the immune microenvironment, in which the external side of plasma is more expressed. The serine-type peptidase activity and serine hydrolase activity were the two most prevalent molecular function (MF) terms. Also, other pathways in the KEGG pathway analysis showed that the cytotoxic receptor and chemokine signaling pathway were the most abundant pathways (Figure 4B).
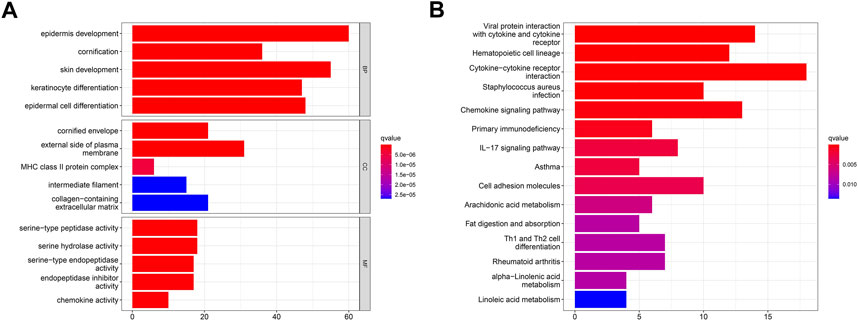
FIGURE 4. Functional annotation of 17 polyamine regulators using GO terms and the KEGG pathway. GO (A) and KEGG (B) analyses of the identified genes.
3.5 PARDEGs construct phenotypes in OSCC
We first conducted univariate Cox regression among the 289 PARDEGs, and we got 125 prognostic genes in total, as shown in Supplementary Table S2. Then, we performed consensus clustering analysis based on the 125 prognostic genes and obtained three stable transcriptomic phenotypes (Figure 5A; Supplementary Figures S2A–J).
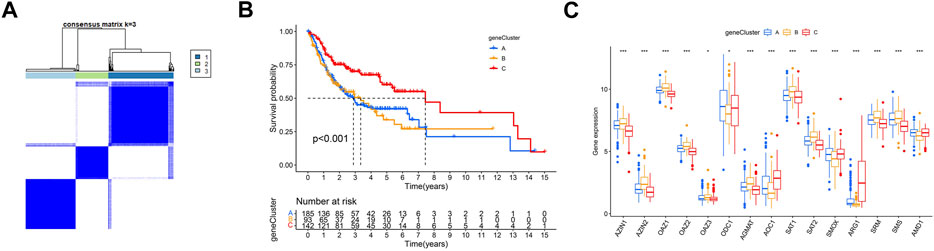
FIGURE 5. Identification of polyamine-related differentially expressed gene (PARDEG) clusters. (A) Consensus clustering of OSCC patients based on PARDEGs. (B) Comparison of the three cluster survival probability of OSCC patients. (C) Polyamine marker expression in three clusters.
Among them, the survival rate of group B (n = 93) is the worst, while that of group C (n = 142) is more advantageous in the long survival probability (Figure 5B). By analyzing the polyamine regulator expression in A, B, and C groups, we found that the previously mentioned favorable factors ARG1 and AOC1 were highly expressed in the C group; in other words, they were expressed low in A and B groups (Figure 5C).
In order to build a prognostic model related to the oral squamous cell carcinoma, the LASSO regression for these genes was carried out (Figures 6A, B), and biomarkers of the 12 genes were screened. Additionally, by the univariate Cox regression, we can identify whether these genes are risk or preventive factors (Figure 6C). Finally, multivariate Cox regression was used to select the final six penalty genes (Figure 6D). At this time, the risk of overfitting is minimizing.
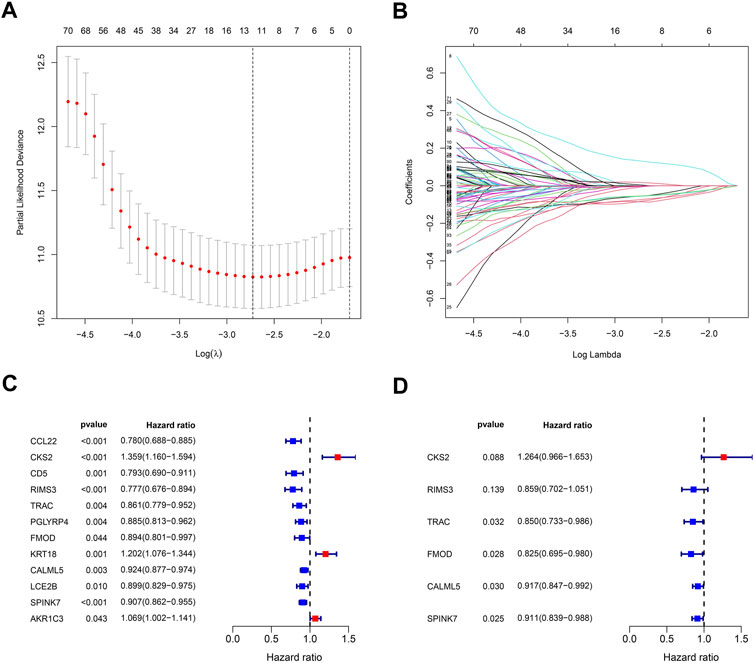
FIGURE 6. Stepwise identification of PARG risk signature of the model. (A) Cross-validation for tuning parameter selection in the proportional hazards model. (B) LASSO coefficient of the PARDEGs. (C) Forest plot of the univariate Cox regression analysis in DEGs. (D) Forest plot of the multivariate Cox regression analysis in DEGs.
3.6 The development of the risk score by PARDEGs and prognostic analysis
According to the six penalty genes, namely, “CKS2,” “RIMS3,” “TRAC,” “FMOD,” “CALML5,” and “SPINK7,” the model was established and its effectiveness was verified. The risk scores were calculated as the following formula:
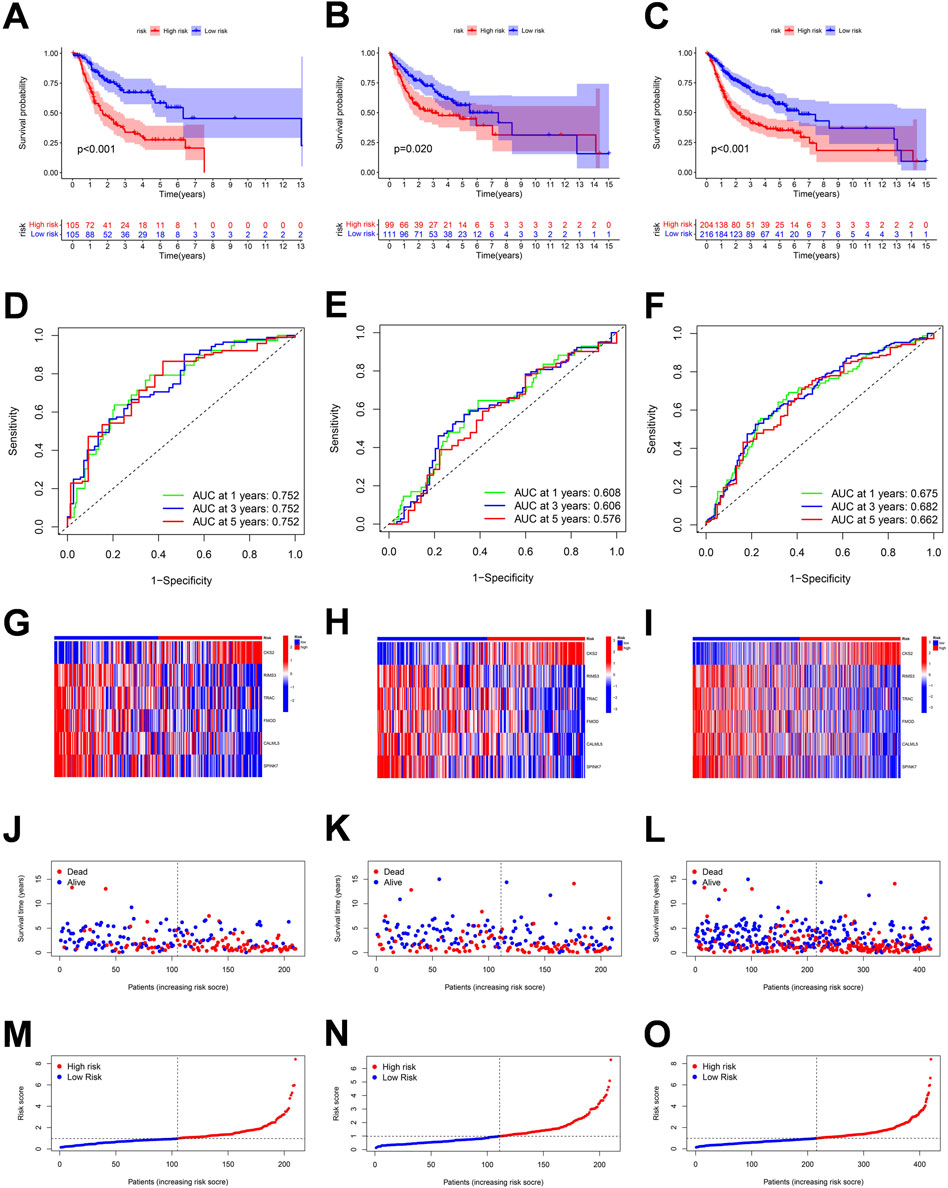
FIGURE 7. Correlation between the risk score and overall survival of OSCC patients in the training, validation, and the whole cohort. (A–C) Overall survival (OS) of the high-risk group was significantly shorter than that of the low-risk group. (D–F) ROC curve and the areas under the curve for predicting a 1-, 3-, and 5-year OS in OSCC patient. (G–I) Heatmap of six genes’ expression in the training, test, and total cohorts. (J–O) Based on the polyamine-related risk score, groups are distributed. Scatterplot showed the variations in OSCC patients' survival rates between high-risk and low-risk categories.
3.7 Correlation between PARGs and its related clusters with risk scores
The Sankey plot shows the flow of the OSCC patients’ survival and death status from the first clustering analysis to the second clustering analysis and then to the final risk-related group (Figure 8A). The risk scores of the two groups were consistent with the previous survival prognosis (Figures 8B, C). Again, we examined the expression of genes related to polyamines in the two groups determined by the risk score; as shown in Figure 8D, PAOX, AOC1, and ARG1 were expressed higher in the low-risk group, while AZIN1, OAZ1, SAT2, AGMAT, SMS, and SRM were the opposite.
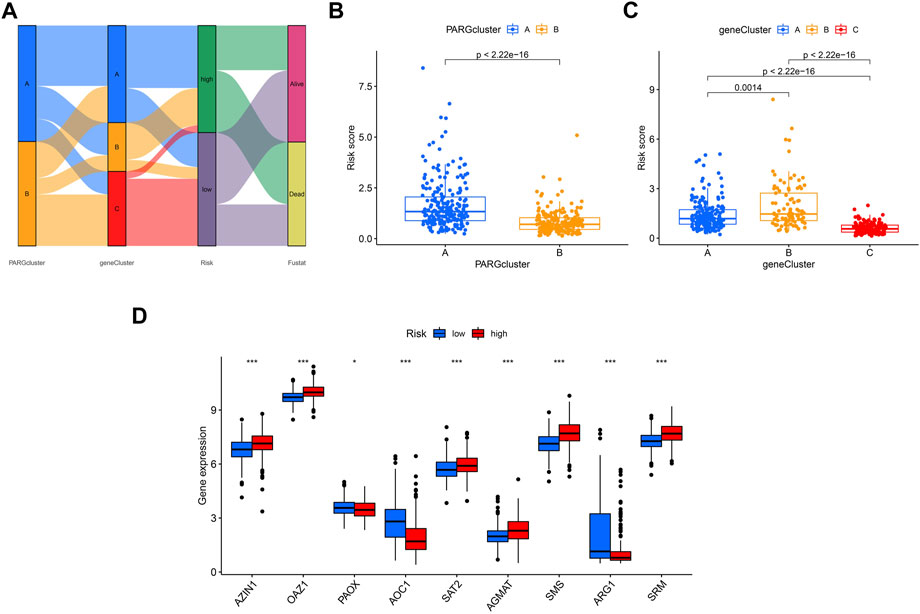
FIGURE 8. Comparison of the effectiveness of risk scores in different groups. (A) Sankey plot shows the OSCC patients’ distribution in different states of our analyses. (B) Risk score in A and B clusters related to PARGs. (C) Risk score in A, B, and C clusters divided by the PARDEG cluster-related genes. (D) High- and low-risk groups expressed PARGs significantly different.
3.8 Correlation between immune infiltration cells and risk scores
Simultaneously, we examined the expression of 22 immune cell infiltration correlated with risk scores by ssGSEA. There was a significant inverse correlation between the risk score and the naïve B cells, resting mast cells, resting dendritic cells, activated memory CD 4 + T cells, CD8+ T cells, helper follicular T cells, gamma delta T cells, and regulatory T cells. The rest cell types were positively correlated with risk scores such as memory B cells, M0 macrophages, activated mast cells, and resting NK cells (Figures 9A–L).
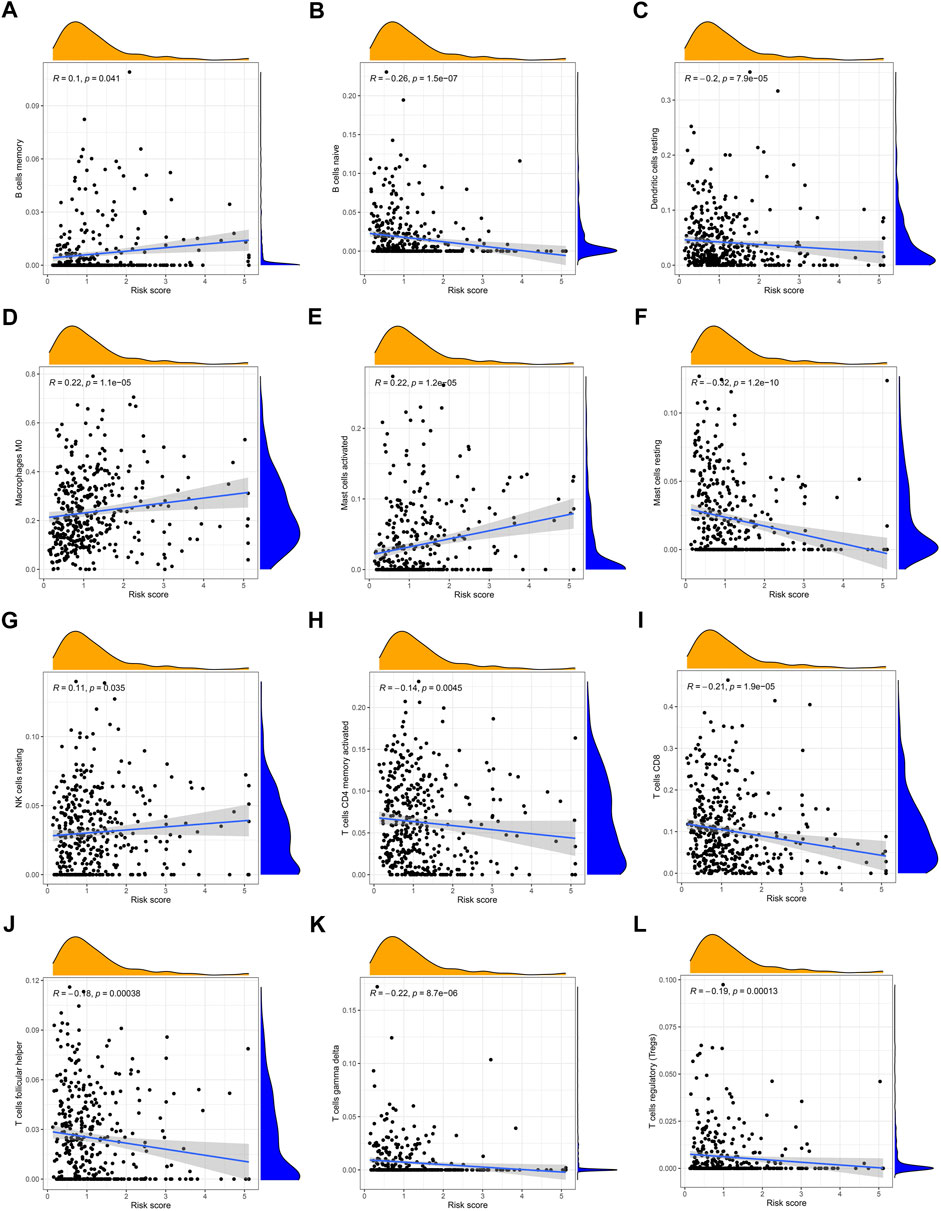
FIGURE 9. Correlation between the risk score and the immune cell infiltration by ssGSEA (A) memory B cells, (B) naive B cells, (C) resting dendritic cells, (D) M0 macrophages, (E) activated mast cells, (F) resting mast cells, (G) resting NK cells, (H) activated memory CD4+ T cells, (I) CD8+ T cells, (J) follicular helper T cells, (K) gamma delta T cells, and (L) regulatory T cells.
3.9 Differences in mutation frequency between high- and low-risk groups
Different risk scores are related to the TMB (tumor mutation burden) of OSCC patients. The higher the risk score of patients, the higher their TMB is (Figure 10A). Additionally, A, B, and C groups are shown to be correlated with risk scores and TMB; group C has the lowest risk score, and its TMB is lower than that of groups A and B (Figure 10B). Then, we analyzed the mutation load of different risk groups. Most of the mutations are concentrated in TP53, TTN, and FAT1; overall, the high-risk group had a greater mutation rate (97.44%) than the low-risk group (88.89%).
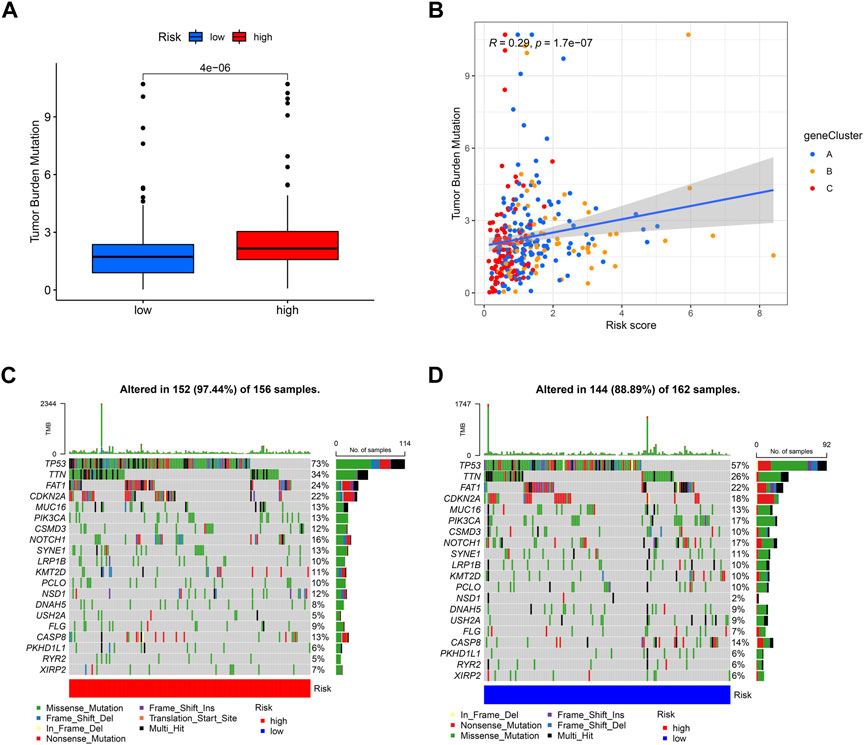
FIGURE 10. Exploration of risk scores and tumor mutation burden. (A) High-risk score tumors were markedly correlated with a higher TMB (p = 4e−06, Student’s t-test). (B) There was a positive correlation between risk scores and TMB (p = 1.7e−07). Tumor mutation landscape in high-risk (C) and low-risk (D) score groups of OSCC patients were presented in the waterfall plots.
3.10 The IC50 of the chemotherapy between high- and low-risk groups
In tumor treatment, chemotherapy still dominates in therapeutic drugs. Depending on the risk score, it might provide various chemotherapeutic effects; a cluster with a high risk score was more likely to respond to chemotherapy. By comparing drug sensitivity, we discovered four chemotherapeutic medications, such as cisplatin, docetaxel, doxorubicin, and pacitaxel, which showed better efficacy in high-risk groups (Figures 11A–D).
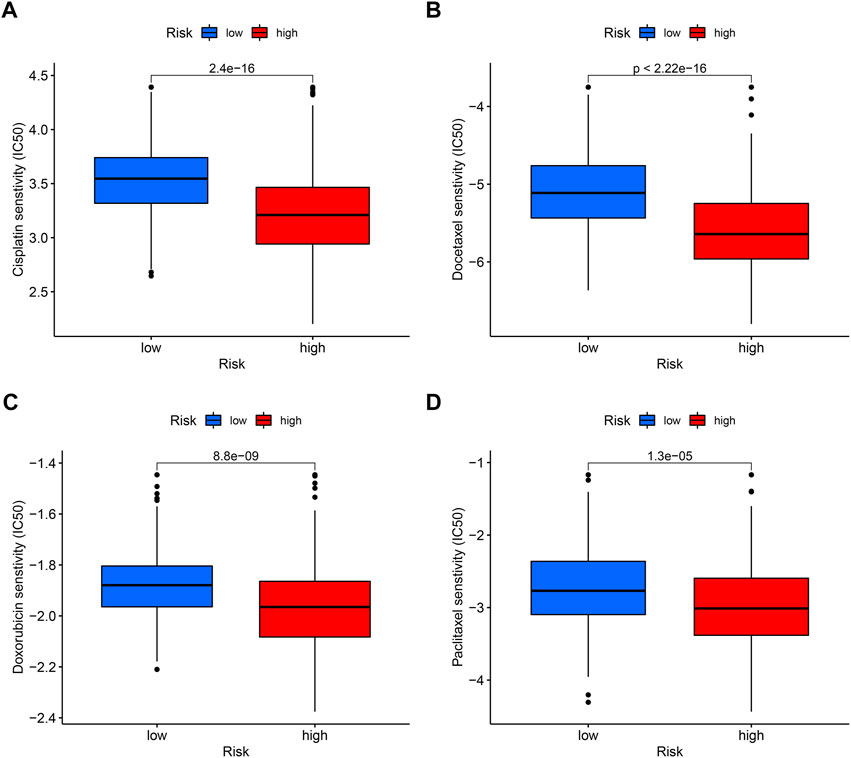
FIGURE 11. Sensitivity of low- and high-risk patients to four common chemotherapy agents. The y-axis represents 50% inhibitory concentration (IC50). (A) Cisplatin, (B) docetaxel, (C) doxorubicin, and (D) paclitaxel.
3.11 PARDEG expression levels and its correlation between immune cell infiltration and chemotherapy
We examined the expression levels of PARDEG expression in cancer and normal tissues. Three genes were differently expressed between normal and tumor tissue samples in boxplots (p < 0.05) (Figure 12A). Two of these, CKS2 and RIMS3, were shown to be substantially expressed in tumor tissues. Also, SPINK7 is highly expressed in normal tissues. When compared to paired adjacent normal tissues in clinically collected samples, q-PCR results showed that CKS2 was statistically substantially more expressed in the OSCC tissues (p < 0.001) (Figure 12B).
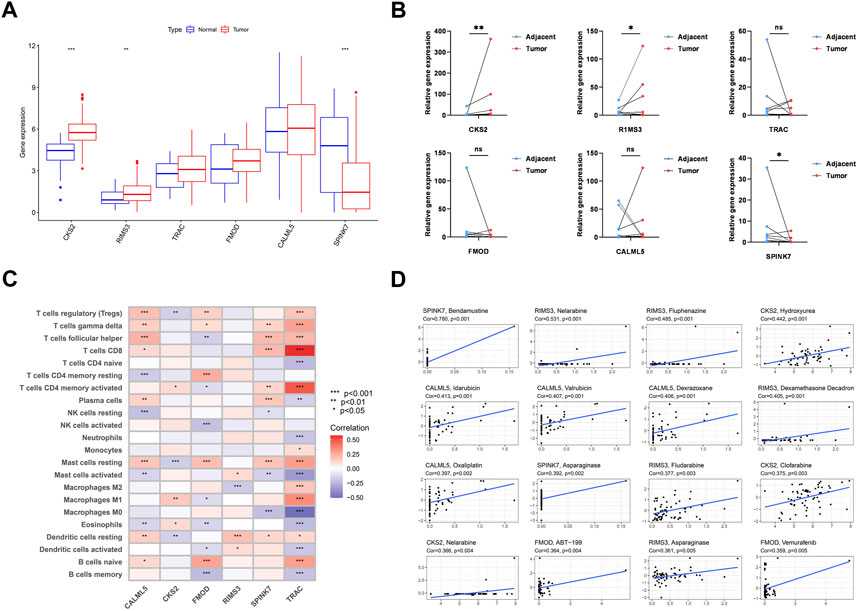
FIGURE 12. Six genes’ expression and its correlation with immune cells and chemotherapy. (A) Expression of six genes constructed the model. (B) RNA expression level of six genes in 12 pairs of tumor and normal adjacent tissues from OSCC patients. (C) Correlation between each TME infiltration cell 22 type and each regulator constructing the model using Spearman’s analysis. (D) Top 16 chemotherapy correlated to the six genes.
The heatmap shows the interaction of six genes and immune cell infiltration in OSCC. TRAC is significantly correlated with CD8+, CD4 memory-activated B cells, and macrophages (Figure 12C). Here, the association between PARDEGs and different chemotherapeutic drugs is carried out for six genes (Figure 12D), and the 16 drugs in different genes with the highest correlation are listed as follows: SPINK7-bendamustine, RIMS3-nelarabine, CKS2-hydroxyurea, CALML5-idarubicin, and FMOD-ABT-199.
4 Discussion
The role of the polyamine metabolism in tumors has been gradually reported, and some reviews have emerged and stated the current status of the polyamine metabolism in tumors (McNamara et al., 2021; Novita Sari et al., 2021). Lewis et al. assessed the 2-difluoromethylornithine (DFMO) as a treatment for OSCC in a cat, which is a polyamine inhibitor (Lewis et al., 2013). Polyamine-related genes might provide an exploitable treatment strategy, advance individual therapy, and enhance the prognosis of patients. Data from Chen (Hsu et al., 2019) demonstrated an overabundance of polyamine and its associated metabolites in OSCC. Ornithine decarboxylase 1 (ODC1), the mutations of which were concentrated in our research the most (Figure 2B), is now frequently increased in cancer cells (Choi et al., 2016) and necessary for cell proliferation, transforms ornithine into putrescine, and is associated with cellular mechanisms that lead to an increase in polyamine metabolites (Miller-Fleming et al., 2015). A promising approach for overcoming the limitations of systemic therapies and advancing the science of immunotherapy is the targeting of polyamines. Therefore, it is important to properly understand and evaluate the links and processes among polyamines, immunity, and OSCC.
Amine oxidase copper containing 1 (AOC1) catalyzes the breakdown of related molecules and oxidatively deaminates putrescine and histamine. According to recent research studies (Ding et al., 2022), in order to stop the growth of cancer cells, the activity of AOC1 on spermidine produces reactive oxygen species and results in ferroptosis. Proline and polyamides are produced by urea cycle enzyme arginase-1 (ARG1), which is essential for tumor cell growth. It is an important regulator of both innate and adaptive immune responses in the arginine metabolism. When the cell dies, it is released from the phagolysosome and depletes the microenvironment of arginine, which inhibits the proliferation of T cells, natural killer cells, and the release of cytokines (Munder et al., 2006). Consistent with this, the upregulation of AOC1 and ARG1 genes in OSCC was observed in our independent dataset from TCGA.
Then, to evaluate the pattern of polyamine alteration in OSCC patients, the risk score method was created by six genes as follows: “CKS2” (Gao et al., 2021), a subunit of the cyclin dependent kinases and is essential for their biological function. “RIMS3” (Deng et al., 2022) is essential for transmembrane transporter binding activity. “TRAC” (Long et al., 2021) is a protein coding gene related to immunodeficiency. “FMOD” (Silva et al., 2022) can affect the rate of fibrils formation as a biomarker of prostate cancer. “CALML5” (Kitazawa et al., 2021), which can bind calcium, may be involved in terminal differentiation of keratinocytes. The expression changes of “SPINK7” (Pennacchiotti et al., 2021) can be relevant in predicting OSCC at a molecular level. Patients with high-risk scores have the worse OS related to poor survival prognosis and high TMB than those with low risk scores and inhibitory TME status. These findings suggested that the risk score can offer an innovative approach to assessing the TME status and prognosis of oral squamous cell carcinoma.
The adaptive immune system’s lymphogenesis and T-cell and B-cell activation are both influenced by polyamines. One of the many ways that a B-cell activity can stop the formation of cancers is through stimulating CD4+ T cells and CD8+ T cells, as well as by producing antibodies that are reactive to tumors. Previous research has demonstrated (Zeng et al., 2021) that many subtypes have varying immunological and immune cell infiltration, which affect their prognoses and responsiveness to immunotherapy. Therefore, based on the expression of genes associated to polyamine clusters, we classified patients into three clusters. The expression of polyamine synthesis enzymes is higher in malignant tumors than in normal tissues, and this is associated with an immunosuppressive phenotype. The exchange of materials and energy between tumor cells occurs in the TME, which is crucial to the biology of tumors (Yoshihara et al., 2013). Immunosuppressive environments enable the use of polyamine-reduction methods to boost the antitumor immune responses.
In addition (Cerqueira et al., 2020), tumors evade the immune system by lowering the activity of CD8+ T cells while boosting the activity of CD4+ T cells. Our ssGSEA (Figure 5D) revealed that the expression level of CD8+ T cells was substantially lower in cluster A, which has a high CD4/CD8 ratio. Also, immunosuppressive myeloid-derived suppressor cells (MDSCs) are often found in abundance in the immunosuppressive microenvironment of tumors (Latour et al., 2020). When the findings of our investigation were merged with the findings of the OS analysis, they agreed with previous conclusions. As a result, we expected that polyamine-related modification patterns would alter the TME of OSCC, hence influencing survival rates. In the experimental autoimmune mouse model, it showed that the coordinated blocking of polyamine uptake and synthesis prevented T-cell-mediated inflammation (Wu et al., 2020; Chia et al., 2022). Figure 12C shows that in the foundation of the foregoing prognostic model, the TRAC gene mostly promotes tumor growth in OSCC patients by regulating T cells in several directions (upregulating CD8, driving naive CD4 T cells to convert to activated CD4 T cells, and inducing macrophages M0 to convert to macrophages M1 and M2).
Thus, T-cell-targeting immunotherapies and other immunotherapeutic medications can be used to treat patients. By releasing PRF1, GNLY, or GZM and rupturing previous immunological tolerance, CD8 + T cells can destroy cancer cells and improve immunotherapy by activating the PD-1/PD-L1 immune inhibitory axis. This will advance not only immunotherapy but also the study of cancer.
In addition, patients with OSCC in the high-risk group, which mainly come from cluster A (with higher polyamine-related genes expression), were more sensitive to chemotherapy drugs (Figure 11). Patients with high polyamine-related genes expressed in liquid biopsies could be distinguished by imaging mass cytometry or other experiments, which can guide through the application of chemotherapy medications. Patients with OSCC currently undergo cisplatin, paclitaxel, and docetaxel as their first-line treatments (Meng et al., 2021). Doxorubicin was usually used in combination with radiotherapy as 2B chemotherapy, to treat head and neck adenomas and metastatic tumors, according to the NCCN guidelines.
Although we had used various techniques to strengthen our model, there were still some flaws and weaknesses. Because it was a retrospective study, it was subjected to the biases that were built by this research paradigm. It was challenging to conduct external validation for prediction though we had completed internal validation in the model. The immune cell infiltration and the fitting of chemotherapy methods displayed the results from several platforms, and we also used collected tissues to validate the RNA expression level, which could be viewed as external validation in a certain sense. We intend to gather more clinical datasets to reaffirm the importance of these polyamine-related genes.
5 Conclusion
In this study, two distinct polyamine modification patterns were discovered, and the immune cell infiltration was different in these clusters, indicating that the modification played a vital role in shaping individual tumor microenvironment characterizations. We built a six PARDEG model and attempted to identify the prognosis and immune infiltration of OSCCs. Moreover, the model could assess individual tumor polyamine alteration patterns and predict the clinical response of patients to chemical therapy. These findings are conducive to understanding the mechanism of polyamine in OSCC and provide novel targets for treating OSCC patients.
Data availability statement
Publicly available datasets were analyzed in this study. These data can be found online in the following: the datasets involved during the current study are available in TCGA (https://portal.gdc.cancer.gov/) and GEO (https://www.ncbi.nlm.nih.gov/gds/), and the protein–protein interaction (PPI) database was from STRING (https://string-db.org/).
Ethics statement
The studies involving human participants were reviewed and approved by the Medical Ethics Committee of the Zhongnan Hospital of Wuhan University. The patients/participants provided their written informed consent to participate in this study.
Author contributions
Study concepts: YL and BC; study design: YL and BC; data acquisition: XW and JT; quality control of data and algorithms: JT; data analysis and interpretation: XW; statistical analysis: JT; manuscript preparation: XW and JT; manuscript editing: YL and BC; manuscript review: YL and BC.
Funding
This work was financially supported by the National Natural Science Foundation of China (No. 32000960).
Acknowledgments
We thank the contributors for their significant public datasets as well as the GEO and TCGA databases for offering their platforms.
Conflict of interest
The authors declare that the research was conducted in the absence of any commercial or financial relationships that could be construed as a potential conflict of interest.
Publisher’s note
All claims expressed in this article are solely those of the authors and do not necessarily represent those of their affiliated organizations, or those of the publisher, the editors, and the reviewers. Any product that may be evaluated in this article, or claim that may be made by its manufacturer, is not guaranteed or endorsed by the publisher.
Supplementary material
The Supplementary Material for this article can be found online at: https://www.frontiersin.org/articles/10.3389/fmolb.2023.1073770/full#supplementary-material
References
Casero, R. A., Murray Stewart, T., and Pegg, A. E. (2018). Polyamine metabolism and cancer: Treatments, challenges and opportunities. Nat. Rev. Cancer 18 (11), 681–695. doi:10.1038/s41568-018-0050-3
Çelik, V. K., Kapancık, S., Kaçan, T., Kaçan, S. B., Kapancık, S., and Kılıçgün, H. (2017). Serum levels of polyamine synthesis enzymes increase in diabetic patients with breast cancer%. J. Endocr. Connect. 6 (8), 574–579. doi:10.1530/ec-17-0137
Cerqueira, M. A., Ferrari, K. L., de Mattos, A. C., Monti, C. R., and Reis, L. O. (2020). T cells CD4+/CD8+ local immune modulation by prostate cancer hemi-cryoablation. World J. Urol. 38 (3), 673–680. doi:10.1007/s00345-019-02861-0
Chaturvedi, R., Cheng, Y., Asim, M., Bussière, F. I., Xu, H., Gobert, A. P., et al. (2004). Induction of polyamine oxidase 1 by Helicobacter pylori causes macrophage apoptosis by hydrogen peroxide release and mitochondrial membrane depolarization. J. Biol. Chem. 279 (38), 40161–40173. doi:10.1074/jbc.M401370200
Chia, T. Y., Zolp, A., and Miska, J. (2022). Polyamine immunometabolism: Central regulators of inflammation, cancer and autoimmunity. Cells 11 (5), 896. doi:10.3390/cells11050896
Choi, Y., Oh, S. T., Won, M. A., Choi, K. M., Ko, M. J., Seo, D., et al. (2016). Targeting ODC1 inhibits tumor growth through reduction of lipid metabolism in human hepatocellular carcinoma. Biochem. Biophys. Res. Commun. 478 (4), 1674–1681. doi:10.1016/j.bbrc.2016.09.002
Deng, H., Chen, S., Yuan, X., and Zhang, J. (2022). Transcriptome sequencing analysis of the effect of β-elemene on colorectal cancer from the lncRNA-miRNA-mRNA perspective. Genet. Res. (Camb) 2022, 5896296. doi:10.1155/2022/5896296
Ding, Y., Feng, Y., Huang, Z., Zhang, Y., Li, X., Liu, R., et al. (2022). SOX15 transcriptionally increases the function of AOC1 to modulate ferroptosis and progression in prostate cancer. Cell Death Dis. 13 (8), 673. doi:10.1038/s41419-022-05108-w
Gao, F., Li, C., Zhao, X., Xie, J., Fang, G., and Li, Y. (2021). CKS2 modulates cell-cycle progression of tongue squamous cell carcinoma cells partly via modulating the cellular distribution of DUTPase. J. Oral Pathol. Med. 50 (2), 175–182. doi:10.1111/jop.13116
Geeleher, P., Cox, N., and Huang, R. S. (2014). pRRophetic: an R package for prediction of clinical chemotherapeutic response from tumor gene expression levels. PLoS One 9 (9), e107468. doi:10.1371/journal.pone.0107468
Gobert, A. P., Cheng, Y., Wang, J. Y., Boucher, J. L., Iyer, R. K., Cederbaum, S. D., et al. (2002). Helicobacter pylori induces macrophage apoptosis by activation of arginase II. J. Immunol. 168 (9), 4692–4700. doi:10.4049/jimmunol.168.9.4692
Holbert, C. E., Cullen, M. T., Casero, R. A., and Stewart, T. M. (2022). Polyamines in cancer: Integrating organismal metabolism and antitumour immunity. Nat. Rev. Cancer 22 (8), 467–480. doi:10.1038/s41568-022-00473-2
Hsu, C. W., Chen, Y. T., Hsieh, Y. J., Chang, K. P., Hsueh, P. C., Chen, T. W., et al. (2019). Integrated analyses utilizing metabolomics and transcriptomics reveal perturbation of the polyamine pathway in oral cavity squamous cell carcinoma. Anal. Chim. Acta 1050, 113–122. doi:10.1016/j.aca.2018.10.070
Ishikawa, S., Wong, D. T. W., Sugimoto, M., Gleber-Netto, F. O., Li, F., Tu, M., et al. (2019). Identification of salivary metabolites for oral squamous cell carcinoma and oral epithelial dysplasia screening from persistent suspicious oral mucosal lesions. Clin. Oral Investig. 23 (9), 3557–3563. doi:10.1007/s00784-018-2777-3
Kitazawa, S., Takaoka, Y., Ueda, Y., and Kitazawa, R. (2021). Identification of calmodulin-like protein 5 as tumor-suppressor gene silenced during early stage of carcinogenesis in squamous cell carcinoma of uterine cervix. Int. J. Cancer 149 (6), 1358–1368. doi:10.1002/ijc.33687
Laskowski, T. J., Biederstädt, A., and Rezvani, K. (2022). Natural killer cells in antitumour adoptive cell immunotherapy. Nat. Rev. Cancer 22, 557–575. doi:10.1038/s41568-022-00491-0
Latour, Y. L., Gobert, A. P., and Wilson, K. T. (2020). The role of polyamines in the regulation of macrophage polarization and function. Amino Acids 52 (2), 151–160. doi:10.1007/s00726-019-02719-0
Lewis, J. R., O'Brien, T. G., Skorupski, K. A., Krick, E. L., Reiter, A. M., Jennings, M. W., et al. (2013). Polyamine inhibitors for treatment of feline oral squamous cell carcinoma: A proof-of-concept study. J. Veterinary Dent. 30 (3), 140–145. doi:10.1177/089875641303000301
Liu, R., Lin, X., Li, Z., Li, Q., and Bi, K. (2018). Quantitative metabolomics for investigating the value of polyamines in the early diagnosis and therapy of colorectal cancer. Oncotarget 9 (4), 4583–4592. doi:10.18632/oncotarget.22885
Long, M. D., Jacobi, J. J., Singh, P. K., Llimos, G., Wani, S. A., Rowsam, A. M., et al. (2021). Reduced NCOR2 expression accelerates androgen deprivation therapy failure in prostate cancer. Cell Rep. 37 (11), 110109. doi:10.1016/j.celrep.2021.110109
Lu, X., Pearson, A., and Lunec, J. (2003). The MYCN oncoprotein as a drug development target. Cancer Lett. 197 (1), 125–130. doi:10.1016/S0304-3835(03)00096-X
McNamara, K. M., Gobert, A. P., and Wilson, K. T. (2021). The role of polyamines in gastric cancer. Oncogene 40 (26), 4399–4412. doi:10.1038/s41388-021-01862-x
Meng, X., Lou, Q. Y., Yang, W. Y., Wang, Y. R., Chen, R., Wang, L., et al. (2021). The role of non-coding RNAs in drug resistance of oral squamous cell carcinoma and therapeutic potential. Cancer Commun. (Lond) 41 (10), 981–1006. doi:10.1002/cac2.12194
Miller-Fleming, L., Olin-Sandoval, V., Campbell, K., and Ralser, M. (2015). Remaining mysteries of molecular biology: The role of polyamines in the cell. J. Mol. Biol. 427 (21), 3389–3406. doi:10.1016/j.jmb.2015.06.020
Minois, N., Carmona-Gutierrez, D., and Madeo, F. (2011). Polyamines in aging and disease. Aging (Albany NY) 3 (8), 716–732. doi:10.18632/aging.100361
Munder, M., Schneider, H., Luckner, C., Giese, T., Langhans, C. D., Fuentes, J. M., et al. (2006). Suppression of T-cell functions by human granulocyte arginase. Blood 108 (5), 1627–1634. doi:10.1182/blood-2006-11-010389
Murray-Stewart, T., Dunworth, M., Lui, Y., Giardiello, F. M., Woster, P. M., and Casero, R. A. (2018). Curcumin mediates polyamine metabolism and sensitizes gastrointestinal cancer cells to antitumor polyamine-targeted therapies. PLoS One 13 (8), e0202677. doi:10.1371/journal.pone.0202677
Nakajima, T., Katsumata, K., Kuwabara, H., Soya, R., Enomoto, M., Ishizaki, T., et al. (2018). Urinary polyamine biomarker panels with machine-learning differentiated colorectal cancers, benign disease, and healthy controls. Int. J. Mol. Sci. 19 (3), 756. doi:10.3390/ijms19030756
Nijakowski, K., Gruszczynski, D., Kopala, D., and Surdacka, A. (2022). Salivary metabolomics for oral squamous cell carcinoma diagnosis: A systematic review. Metabolites 12 (4), 294. doi:10.3390/metabo12040294
Novita Sari, I., Setiawan, T., Seock Kim, K., Toni Wijaya, Y., Won Cho, K., and Young Kwon, H. (2021). Metabolism and function of polyamines in cancer progression. Cancer Lett. 519, 91–104. doi:10.1016/j.canlet.2021.06.020
Ohshima, M., Sugahara, K., Kasahara, K., and Katakura, A. (2017). Metabolomic analysis of the saliva of Japanese patients with oral squamous cell carcinoma. Oncol. Rep. 37 (5), 2727–2734. doi:10.3892/or.2017.5561
Pegg, A. E. (2009). Mammalian polyamine metabolism and function. IUBMB Life 61 (9), 880–894. doi:10.1002/iub.230
Pennacchiotti, G., Valdés-Gutiérrez, F., González-Arriagada, W. A., Montes, H. F., Parra, J. M. R., Guida, V. A., et al. (2021). SPINK7 expression changes accompanied by HER2, P53 and RB1 can be relevant in predicting oral squamous cell carcinoma at a molecular level. Sci. Rep. 11 (1), 6939. doi:10.1038/s41598-021-86208-z
Silva, T., Gomes, C. P., Voigt, D. D., de Souza, R. B., Medeiros, K., Cosentino, N. L., et al. (2022). Fibromodulin gene variants (FMOD) as potential biomarkers for prostate cancer and benign prostatic hyperplasia. Dis. Markers 2022, 5215247. doi:10.1155/2022/5215247
Snezhkina, A. V., Krasnov, G. S., Lipatova, A. V., Sadritdinova, A. F., Kardymon, O. L., Fedorova, M. S., et al. (2016). The dysregulation of polyamine metabolism in colorectal cancer is associated with overexpression of c-myc and C/EBPβ rather than enterotoxigenic Bacteroides fragilis infection. Oxid. Med. Cell Longev. 2016, 2353560. doi:10.1155/2016/2353560
Tsoi, T. H., Chan, C. F., Chan, W. L., Chiu, K. F., Wong, W. T., Ng, C. F., et al. (2016). Urinary polyamines: A pilot study on their roles as prostate cancer detection biomarkers. PLoS One 11 (9), e0162217. doi:10.1371/journal.pone.0162217
Wilkerson, M. D., and Hayes, D. N. (2010). ConsensusClusterPlus: A class discovery tool with confidence assessments and item tracking. Bioinformatics 26 (12), 1572–1573. doi:10.1093/bioinformatics/btq170
Wu, R., Chen, X., Kang, S., Wang, T., Gnanaprakasam, J. R., Yao, Y., et al. (2020). De novo synthesis and salvage pathway coordinately regulate polyamine homeostasis and determine T cell proliferation and function. Sci. Adv. 6 (51), eabc4275. doi:10.1126/sciadv.abc4275
Wu, X., Tang, J., and Cheng, B. (2022). Oral squamous cell carcinoma gene patterns connected with RNA methylation for prognostic prediction. Oral Dis. doi:10.1111/odi.14341
Yoshihara, K., Shahmoradgoli, M., Martínez, E., Vegesna, R., Kim, H., Torres-Garcia, W., et al. (2013). Inferring tumour purity and stromal and immune cell admixture from expression data. Nat. Commun. 4, 2612. doi:10.1038/ncomms3612
Yu, G., Wang, L. G., Han, Y., and He, Q. Y. (2012). clusterProfiler: an R package for comparing biological themes among gene clusters. Omics 16 (5), 284–287. doi:10.1089/omi.2011.0118
Keywords: oral squamous cell carcinoma, bioinformatics analyses, The Cancer Genome Atlas, polyamines, prognostic model, immune infiltration
Citation: Tang J, Wu X, Cheng B and Lu Y (2023) Identification of a polyamine-related signature and six novel prognostic biomarkers in oral squamous cell carcinoma. Front. Mol. Biosci. 10:1073770. doi: 10.3389/fmolb.2023.1073770
Received: 18 October 2022; Accepted: 03 January 2023;
Published: 17 January 2023.
Edited by:
Dinesh Kumar, Centre of Bio-Medical Research (CBMR), IndiaReviewed by:
Tae Jin Lee, Augusta University, United StatesMaheshwor Thapa, Jackson Laboratory for Genomic Medicine, United States
Copyright © 2023 Tang, Wu, Cheng and Lu. This is an open-access article distributed under the terms of the Creative Commons Attribution License (CC BY). The use, distribution or reproduction in other forums is permitted, provided the original author(s) and the copyright owner(s) are credited and that the original publication in this journal is cited, in accordance with accepted academic practice. No use, distribution or reproduction is permitted which does not comply with these terms.
*Correspondence: Bo Cheng, chengbo@znhospital.cn; Yajie Lu, yajiel_fmmu@foxmail.com
†These authors have contributed equally to this work and share first authorship