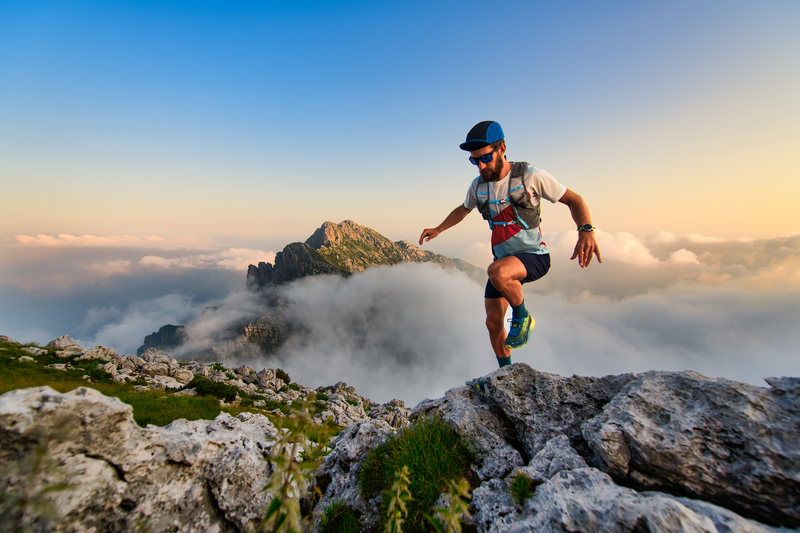
94% of researchers rate our articles as excellent or good
Learn more about the work of our research integrity team to safeguard the quality of each article we publish.
Find out more
MINI REVIEW article
Front. Mol. Biosci. , 06 October 2022
Sec. Biophysics
Volume 9 - 2022 | https://doi.org/10.3389/fmolb.2022.959688
This article is part of the Research Topic Highly Resolved Spatio-Temporal Dynamics of Genome Organization and its Link With Transcriptional Dynamics View all 5 articles
The three-dimensional (3D) structure of chromosomes influences essential biological processes such as gene expression, genome replication, and DNA damage repair and has been implicated in many developmental and degenerative diseases. In the past two centuries, two complementary genres of technology—microscopy, such as fluorescence in situ hybridization (FISH), and biochemistry, such as chromosome conformation capture (3C or Hi-C)—have revealed general principles of chromosome folding in the cell nucleus. However, the extraordinary complexity and cell-to-cell variability of the chromosome structure necessitate new tools with genome-wide coverage and single-cell precision. In the past decade, single-cell Hi-C emerges as a new approach that builds upon yet conceptually differs from bulk Hi-C assays. Instead of measuring population-averaged statistical properties of chromosome folding, single-cell Hi-C works as a proximity-based “biochemical microscope” that measures actual 3D structures of individual genomes, revealing features hidden in bulk Hi-C such as radial organization, multi-way interactions, and chromosome intermingling. Single-cell Hi-C has been used to study highly dynamic processes such as the cell cycle, cell-type-specific chromosome architecture (“structure types”), and structure–expression interplay, deepening our understanding of DNA organization and function.
How DNA folds in the nucleus is a fundamental question in biology. The spatial separation of the euchromatin and heterochromatin has been observed since the early age of microscopy. In the 1980s, the development of DNA fluorescence in situ hybridization (FISH) validated the hypothesis of chromosome territories and revived the study of nuclear architecture (Jerkovic’ and Cavalli, 2021). FISH and fluorescent protein imaging brought about fruitful research on the behavior of genomic loci; however, their genomic coverage and resolution remained a bottleneck for further exploration along the path (Ulianov et al., 2017).
The development of genome-wide chromatin architecture technologies has brought the field of 3D genomics into a new era (Figure 1). At the single-cell level, these technologies primarily fall into two categories: sequencing-based methods and imaging-based methods (Ulianov and Razin, 2021). Imaging-based methods directly measure the 3D coordinates of labeled genomic loci. These methods include chromatin tracing (Wang et al., 2016; Bintu et al., 2018; Nir et al., 2018), ORCA (Mateo et al., 2019), Hi-M (Cardozo Gizzi et al., 2019), DNA-MERFISH (Su et al., 2020), DNA seqFISH+ (Takei et al., 2021), OligoFISSEQ (Nguyen et al., 2020), and IGS (Payne et al., 2021).
FIGURE 1. Single-cell Hi-C provides a holistic high-resolution view into the 3D structure of our genetic blueprint. (Left) DNA fluorescence in situ hybridization (FISH) provided the first look into genome organization in the cell nucleus by directly measuring 3D coordinates (i.e., x, y, and z) of various genomic loci (or entire chromosomes, in the case of chromosome painting) but is limited by the optical resolution and spectrum (i.e., the number of loci). (Right) Chromosome conformation capture (3C or Hi-C) indirectly measures nuclear architecture through 3D proximity between genomic loci (i.e., “contact map”). Bulk Hi-C measures the average 3D proximity (probability (P) that two loci are within a certain 3D distance (d)) among a large population of cells and, therefore, cannot produce true 3D structures (dashed line). In particular, the “all-to-all” inter-chromosomal contacts in bulk Hi-C provide conflicting spatial constraints, while chromatin domains (three are depicted here) would be seemingly isolated from each other. In contrast, single-cell Hi-C offers a new concept of a “biochemical microscope.” 3D proximity of a single cell can be converted into actual 3D coordinates of the whole genome, yielding high-resolution structures without the need for specialized equipment.
In contrast, sequencing-based methods measure 3D spatial proximity between genomic loci, producing “chromatin contact maps” that indirectly reflect the relationship between 3D coordinates. The majority of these methods are based on the digestion of DNA followed by proximity ligation—originally pioneered by bulk chromosome conformation capture assays (3C (Dekker et al., 2002) or Hi-C (Lieberman-Aiden et al., 2009))—yielding artificial ligation junctions (“chromatin contacts”) between genomic loci that are far away along the linear sequence but nearby in 3D. Therefore, here, we refer to them as “single-cell Hi-C” (scHi-C) methods (Nagano et al., 2013, 2017; Flyamer et al., 2017; Ramani et al., 2017; Stevens et al., 2017; Tan et al., 2018; Lee et al., 2019; Li et al., 2019). Other sequencing-based methods are ligation-free—such as scSPRITE (Arrastia et al., 2021), GAM (Beagrie et al., 2017), and immunoGAM (Winick-Ng et al., 2021).
These technologies greatly improved our understanding of both higher-order and fine-scale chromosome structures (Fraser et al., 2015). Hi-C strongly supported the existence of chromosome territories because contacts are highly enriched within each chromosome (“intra-chromosome”). Spatial segregation of the euchromatin and heterochromatin manifests as a “plaid” (or “checkerboard”) pattern in the contact map—a phenomenon termed “chromatin A/B compartmentalization” (Lieberman-Aiden et al., 2009). Further studies have shown that sub-compartments exist within the primary A (euchromatin) and B (heterochromatin) compartments, corresponding to spatial segregation of additional epigenetic marks (Rao et al., 2014). The driving force behind compartmentalization might be the phase separation of the chromatin (Falk et al., 2019).
On finer scales, chromatin domains [also known as “topological-associated domains” (TADs)] were discovered as sub-megabase (Mb) chromatin structures (Dixon et al., 2012; Nora et al., 2012; Sexton et al., 2012). At this scale, chromatin loops (also known as “dots” in the contact map), “stripes,” and more complex patterns also emerged from high-resolution Hi-C (Rao et al., 2014) and Micro-C data (Hsieh et al., 2020; Krietenstein et al., 2020; Akgol Oksuz et al., 2021). Among them, loops manifest as pixels in the contact map with higher contact frequencies than neighboring pixels and are usually found at domain boundaries (i.e., at the corner of diagonal “squares” in the contact map). Some believed loops to be stable, but recent studies have preferred a more transient view (Mirny and Dekker, 2021).
In this mini review, we focus on proximity ligation-based DNA sequencing methods for measuring single-cell nuclear architecture. We first introduce current technologies and then review discoveries made by applying them to different biological systems—especially those achievable only with single-cell methods.
In 2013, Nagano et al. (2013) pioneered an incredible feat of bringing Hi-C—which normally required a large number of cells—to the single-cell level. Although key features of bulk Hi-C—such as the enrichment of intra-chromosomal contacts (i.e., chromosome territory)—are preserved, single-cell Hi-C (scHi-C) contact maps uncovered extraordinary cell-to-cell variability of the genome structure. In particular, the highly variable, “patchy” inter-chromosomal contacts are in sharp contrast to the much smoother “all-to-all” contacts in bulk Hi-C. Although the diploid nature of our genome prevented the 3D reconstruction of autosomes (because each contact map was a mixture of different chromosomal copies) at the time, Nagano et al. solved the structure of the (single-copy) male X chromosome. The spatial resolution of these structures was limited by the biochemistry of the time.
In 2017, multiple groups optimized scHi-C technologies. Flyamer et al. (2017) improved the sensitivity of scHi-C and applied it to the oocyte-to-zygote transition in mice. Nagano et al. (2017) redesigned their assay to yield more contacts and better throughput and applied it to the cell cycle in mouse embryonic stem cells (mESCs). Stevens et al. (2017) solved the first 3D genome structure of a single mammalian cell, taking advantage of a special haploid mESC line, thus circumventing the challenge of the normally diploid genome. In sci-Hi-C, Ramani et al. (2017) used combinatorial indexing to achieve high throughput. Single-cell Hi-C methods until 2017 have been reviewed in detail by Ulianov et al. (2017).
In 2018, Tan et al. (2018) solved the first 3D genome structure of the human genome [and the diploid mouse genome using a new method termed Dip-C—which combined improved biochemical sensitivity including a transposon-based whole-genome amplification (WGA) method META] and an algorithm to solve the challenge of the diploid genome by imputing haplotypes from sparse data—and applied Dip-C to the human blood.
scHi-C methods continued to grow in the past years. In 2019, methyl-Hi-C (Li et al., 2019) and sn-m3c-Seq (Lee et al., 2019) combined proximity ligation and whole-genome bisulfite sequencing (WGBS) to simultaneously profile DNA methylation and chromatin architecture.
Sensitivity and scalability are two major criteria to compare the performance of scHi-C methods (Galitsyna and Gelfand, 2021; Zhou et al., 2021). Scalability has been improved by automation (Nagano et al., 2017; Tan et al., 2021) and split-pool barcoding (Ramani et al., 2017). Sensitivity has been improved by better WGA methods (Flyamer et al., 2017; Tan et al., 2018) and one-step library preparation with transposase (Nagano et al., 2017; Stevens et al., 2017; Tan et al., 2018). However, a systematic, uniform comparison of scHi-C methods that takes into account the cell type, ploidy, sequencing depth, and data processing is still lacking (Lando et al., 2018).
Originally defined as contact-rich “squares” along the diagonal line in bulk Hi-C data, chromatin domains [also known as topologically associating domains (TADs)] are characterized by increased contacts within each domain (“intra-domain”) and decreased contacts between domains (“inter-domain”). This appealing feature seems to suggest a role in genome function—for example, to confer specificity of enhancer–promoter interactions by partitioning regulatory elements into different domains (van Arensbergen et al., 2014); however, evidence both for and against this proposed function has accumulated (Nora et al., 2017; Rao et al., 2017; Ghavi-Helm et al., 2019; Zuin et al., 2022). Therefore, the origin and function of chromatin domains are still under debate.
Chromatin domains are usually illustrated based on bulk Hi-C as “globules” of DNA with the two boundary loci stably contacting (Figure 1 middle); however, an alternative explanation is that domains are just population-averaged contact preferences with no discernable structures in single cells. The true extent of cell-to-cell variability is masked in bulk Hi-C data.
Single-cell Hi-C (scHi-C) shed light on those questions. As expected, the ensemble average of scHi-C contact maps reproduced all bulk Hi-C features—including the intensity and position of each chromatin domain “square” (Nagano et al., 2013). In addition, within each cell, the average contact profile (“pile-up”) of bulk Hi-C domains and bulk Hi-C loops showed expected enrichment of contacts within domains and at loop anchors (Flyamer et al., 2017; Gassler et al., 2017).
Cluster of contacts were prominently detected in scHi-C contact maps; however, boundaries of these “single-cell chromatin domains” [sometimes referred to as “TAD-like structures” (TDLs)] were highly variable across cells and did not always coincide with bulk Hi-C domains (Flyamer et al., 2017). Since then, additional algorithms have been developed to systematically annotate single-cell domains, and these domains were found to prefer common boundaries and correlate with epigenetic marks and transcription (Zhou et al., 2019; Li et al., 2021; Zhang et al., 2021). Cell-to-cell variability of chromatin domains has been confirmed with imaging-based methods, which provided additional mechanistic insights (Bintu et al., 2018; Finn et al., 2019; Luppino et al., 2020; Szabo et al., 2020)—for example, cohesin seemed dispensable for single-cell domains (Bintu et al., 2018) but is necessary for bulk Hi-C domains (Rao et al., 2017; Schwarzer et al., 2017).
What is the nature of single-cell chromatin domains? Do they have the same origin as bulk Hi-C domains? Modeling suggested that domain structures called “proto-TADs” naturally emerged from random chromatin polymers confined to a volume (Gürsoy et al., 2014) and that entropy might play a role (Vasquez et al., 2016). The theory of loop extrusion was also used to explain the heterogeneity of single-cell domains, where many chromatin loops are dynamically created and extruded by loop-extruding factors (LEFs) translocating along the chromatin fiber (Flyamer et al., 2017; Liang and Perez-Rathke, 2021; Yu et al., 2021; Dequeker et al., 2022; Li et al., 2022). Questions about single-cell domains have not been settled yet. Care must be taken when studying this question because chromatin domains have developed into a complex concept with different meanings and origins (Beagan and Phillips-Cremins, 2020).
Unlike the “globular” domains, chromatin compartmentalization does not imply a specific physical structure. Chromatin compartments were originally defined from a statistical perspective: genomic loci were divided based on their chromosome-wide contact profiles into two mutually anti-correlated types—“compartment A” and “compartment B.”
Single-cell Hi-C (scHi-C) allowed bulk Hi-C A/B compartments to be visualized in 3D. When scHi-C 3D structures were colored by bulk-defined compartments, the A and B compartments were relatively segregated (Stevens et al., 2017; Tan et al., 2018). This segregation was consistent with imaging-based studies, where single chromosomes showed polarized configuration of compartments (Wang et al., 2016); later, results with higher resolution found more variable spatial arrangements like “sandwiches” and more overlaps between A and B (Su et al., 2020).
Coloring 3D structures with bulk-defined compartments has limitations because single cells—especially cells of different types—can have different epigenomic profiles and, therefore, different chromatin compartments (Su et al., 2020; Ulianov and Razin, 2021). This is even more necessary, considering exceptions to the traditional A/B classification [e.g., the intermediate (“I-type”) compartment, splicing factories, and polycomb-repressed H3K27me3 regions] (Mirny and Dekker, 2021).
The de novo compartment calling directly from single cells is conceptually challenging because bulk Hi-C algorithms rely on cross-correlations between population-averaged long-range contact profiles. Long-range contacts are sparse in single cells because of the sensitivity of contact detection and, more fundamentally, because each genomic locus can only have a limited number of 3D neighbors in each cell.
To accomplish de novo compartment annotation in single cells, a practical solution is to define each genomic locus’s “single-cell compartment score” as the average value of some property of its 3D neighbors, instead of binary A/B categorization. For example, in defining the “A-association score,” the property of choice was the bulk Hi-C A/B compartment (Nagano et al., 2017), whereas in defining “scA/B” values, the property of choice was the frequency of CpG dinucleotides (which is derived from the genome sequence itself rather than from bulk Hi-C) (Tan et al., 2018).
These single-cell compartment scores are essentially the result of 3D “diffusion”/“smoothing” of certain genomic properties. Globally, these metrics were highly correlated with bulk Hi-C annotation (Nagano et al., 2017); however, their cell-to-cell differences were found to convey critical cell identity information, which can be extracted by the dimension-reduction algorithms to tell apart cell types without additional information (i.e., “3D genome structure typing”) (Tan et al., 2018). In addition, developmental switching of single-cell compartment scores correlates with changes in transcription (Tan et al., 2021).
More recent algorithms include imputation based on hypergraph representation learning, which enabled direct identification of single-cell compartments (Zhang et al., 2021). Non-backtracking walks were also reported to find compartments in sparse single-cell data (Polovnikov et al., 2020). It would be worth comparing these methods, especially on large datasets with complex cell types.
By the time this mini review was written, no more than 20 single-cell Hi-C (scHi-C) studies have been published in peer-reviewed journals. A fair proportion of them studied cell lines or their synthetic mixture (Nagano et al., 2013, 2017; Ramani et al., 2017; Stevens et al., 2017; Li et al., 2019; Kim et al., 2020; Ulianov et al., 2021; Pang et al., 2022), while the application of scHi-C to primary tissues has been growing recently. Researchers have exploited different technical features of scHi-C according to the biological systems they studied.
First, scHi-C has the unique advantage of straightforward reconstruction of 3D models (Figure 1 right) and can, therefore, reveal structural features unattainable from bulk Hi-C contact maps. This makes scHi-C a new approach to uncover, visualize, and quantify a variety of nuclear structures. Interesting examples include the Rabl configuration in many species (Stevens et al., 2017; Tan et al., 2018; Zhou et al., 2019), “inside-out” configuration of retinal rods in nocturnal animals (Tan et al., 2019), inter-chromosomal gene and enhancer hubs (also known as “Greek Islands”) in olfactory sensory neurons (Tan et al., 2019), and the “compact silent center” (CSC) in rice (Zhou et al., 2019).
Second, dynamic changes can be reconstructed despite the snapshot nature of Hi-C when enough cells in different states of a process are covered. A pioneering example is in silico cell cycle phasing of mouse embryonic stem cells (mESCs) based on scHi-C contact maps (Nagano et al., 2017). Intensities of compartments, domains, and loops were found to change continually along the cell cycle. Another example is early embryonic development, where bulk Hi-C observed early relaxed chromatin, followed by a prolonged process of higher-order structure establishment in succession (Du et al., 2017; Ke et al., 2017). scHi-C identified domains and loops as early as G1-phase zygotes (Flyamer et al., 2017; Gassler et al., 2017) but agreed that these structures were much weaker than in later stages (Zheng and Xie, 2019). A more recent study with a larger sample size found that domains could be divided into several clusters according to their temporal patterns, such as early parental-specific domains and de novo domains arising at later stages (Collombet et al., 2020). Later in the developmental process, the trajectory of mESC differentiation was analyzed using “contact decay profiles” (CDPs) of sci-Hi-C contact maps (Bonora et al., 2021).
Third, similar to other single-cell technologies that have been used to create a human cell atlas, scHi-C can capture many cell types at once from complex tissues. This is particularly useful when direct cell sorting is impossible because of a lack of prior knowledge (Schwartzman and Tanay, 2015). Applying scHi-C to the human and mouse brain, researchers demonstrated this “atlasing” capability by showing that major cell types can be delineated from chromosome conformation alone (e.g., clustering based on scA/B)—albeit less sensitive than transcriptome- or methylome-based clustering (Lee et al., 2019; Tan et al., 2021). This implies that cell identity is at least partially encoded in the chromatin structure (Winick-Ng et al., 2021). The chromatin structure was also found to correlate with gene expression (Tan et al., 2021) and the epigenome at single-cell resolution (Lee et al., 2019), which is expected because “omic” data were usually correlated with each other (Boix et al., 2021). More interestingly, scHi-C uncovered a new mode of neuron maturation—the radial reconfiguration of certain genes (Tan et al., 2019, 2021). This discovery again demonstrates that new epigenome technologies provide a new understanding of the cell activity from a different angle—in the case of scHi-C, the new angle is from “space.”
Single-cell Hi-C (scHi-C) is, in theory, a distance geometrical measurement of the chromatin, while microscopy is mostly Cartesian. scHi-C and microscopy tend to corroborate each other (Bintu et al., 2018) but also have important differences. The capture radius of the crosslinking-ligation chemistry in Hi-C is relatively short (presumably 10–100 nm) compared to the diffraction limit of conventional optical microscopy (Maslova and Krasikova, 2021). In addition, Hi-C does not measure chirality and, therefore, cannot differentiate enantiomorphs (i.e., mirror images) (Acemel et al., 2016; Xie et al., 2017). Combining scHi-C and microscopy will generate more accurate 3D structures using chirality and long-distance information. Microscopy followed by Hi-C has been used in some studies (Stevens et al., 2017; Lando et al., 2018).
Recent advances in bulk Hi-C chemistry—including new digestion enzymes (Hsieh et al., 2015, 2016) and crosslinking reagents (You et al., 2021)—are worth adopting for single cells. The use of micrococcal nuclease (MNase) will, in principle, increase the number of possible contacts and, therefore, alleviate the data sparsity problem (Galitsyna and Gelfand, 2021). In addition, scHi-C chemistry will also benefit from advances in whole-genome amplification (WGA). For example, the phi29 DNA polymerase in multiple displacement amplification (MDA) was reported to generate artifactual contacts in scHi-C (Ulianov et al., 2021); less biased WGA methods (Chen et al., 2017) and filtering algorithms may increase the accuracy of scHi-C.
One unanswered question in the field of 3D genomics is the function of genome folding: how do genome folding and gene expression influence each other? Functional perturbations—such as the depletion of a few key structural proteins—gave us a limited overview of this structure–function relationship (Rao et al., 2017). Genome editing studies (Zuin et al., 2022) and simultaneous multi-omic measurements may give us a more quantitative rulebook of genome organization. Development of new 3D multi-omic methods (Cardozo Gizzi et al., 2019; Nguyen et al., 2020; Payne et al., 2021; Takei et al., 2021) is, therefore, an exciting next step.
YC, JS, DX, and LT wrote the manuscript. JS illustrated the figures.
YC and DX were supported by the Major Research Plan of the National Natural Science Foundation of China (Grant No. 92049110). LT was supported by a Walter V. and Idun Berry Postdoctoral Fellowship from Stanford University. JS was supported by the Undergraduate Summer Research Fellowship in Chemistry from Stanford University.
DX and LT are inventors on a patent application (US16/615,872) filed by Harvard that covers Dip-C.
The remaining authors declare that the research was conducted in the absence of any commercial or financial relationships that could be construed as a potential conflict of interest.
All claims expressed in this article are solely those of the authors and do not necessarily represent those of their affiliated organizations, or those of the publisher, the editors, and the reviewers. Any product that may be evaluated in this article, or claim that may be made by its manufacturer, is not guaranteed or endorsed by the publisher.
Acemel, R. D., Tena, J. J., Irastorza-Azcarate, I., Marlétaz, F., Gómez-Marín, C., de la Calle-Mustienes, E., et al. (2016). A single three-dimensional chromatin compartment in amphioxus indicates a stepwise evolution of vertebrate Hox bimodal regulation. Nat. Genet. 48, 336–341. doi:10.1038/ng.3497
Akgol Oksuz, B., Yang, L., Abraham, S., Venev, S. V., Krietenstein, N., Parsi, K. M., et al. (2021). Systematic evaluation of chromosome conformation capture assays. Nat. Methods 18, 1046–1055. doi:10.1038/s41592-021-01248-7
Arrastia, M. V., Jachowicz, J. W., Ollikainen, N., Curtis, M. S., Lai, C., Quinodoz, S. A., et al. (2021). Single-cell measurement of higher-order 3D genome organization with scSPRITE. Nat. Biotechnol. 40, 64–73. doi:10.1038/s41587-021-00998-1
Beagan, J. A., and Phillips-Cremins, J. E. (2020). On the existence and functionality of topologically associating domains. Nat. Genet. 52, 8–16. doi:10.1038/s41588-019-0561-1
Beagrie, R., Scialdone, A., Schueler, M., Kraemer, D. C. A., Chotalia, M., Xie, S. Q., et al. (2017). Complex multi-enhancer contacts captured by genome architecture mapping. Nature 543, 519–524. doi:10.1038/nature21411
Bintu, B., Mateo, L. J., Su, J.-H., Sinnott-Armstrong, N. A., Parker, M., Kinrot, S., et al. (2018). Super-resolution chromatin tracing reveals domains and cooperative interactions in single cells. Science 362, eaau1783. doi:10.1126/science.aau1783
Boix, C. A., James, B. T., Park, Y. P., Meuleman, W., and Kellis, M. (2021). Regulatory genomic circuitry of human disease loci by integrative epigenomics. Nature 590, 300–307. doi:10.1038/s41586-020-03145-z
Bonora, G., Ramani, V., Singh, R., Fang, H., Jackson, D. L., Srivatsan, S., et al. (2021). Single-cell landscape of nuclear configuration and gene expression during stem cell differentiation and X inactivation. Genome Biol. 22, 279. doi:10.1186/s13059-021-02432-w
Cardozo Gizzi, A. M., Cattoni, D. I., Fiche, J.-B., Espinola, S. M., Gurgo, J., Messina, O., et al. (2019). Microscopy-based chromosome conformation capture enables simultaneous visualization of genome organization and transcription in intact organisms. Mol. Cell 74, 212–222. e5. doi:10.1016/j.molcel.2019.01.011
Chen, C., Xing, D., Tan, L., Li, H., Zhou, G., Huang, L., et al. (2017). Single-cell whole-genome analyses by linear amplification via transposon insertion (LIANTI). Science 356, 189–194. doi:10.1126/science.aak9787
Collombet, S., Ranisavljevic, N., Nagano, T., Varnai, C., Shisode, T., Leung, W., et al. (2020). Parental-to-embryo switch of chromosome organization in early embryogenesis. Nature 580, 142–146. doi:10.1038/s41586-020-2125-z
Dequeker, B. J. H., Scherr, M. J., Brandão, H. B., Gassler, J., Powell, S., Gaspar, I., et al. (2022). MCM complexes are barriers that restrict cohesin-mediated loop extrusion. Nature 606, 197–203. doi:10.1038/s41586-022-04730-0
Dekker, J., Rippe, K., Dekker, M., and Kleckner, N. (2002). Capturing chromosome conformation. Science 295, 1306–1311. doi:10.1126/science.1067799
Dixon, J. R., Selvaraj, S., Yue, F., Kim, A., Li, Y., Shen, Y., et al. (2012). Topological domains in mammalian genomes identified by analysis of chromatin interactions. Nature 485, 376–380. doi:10.1038/nature11082
Du, Z., Zheng, H., Huang, B., Ma, R., Wu, J., Zhang, X., et al. (2017). Allelic reprogramming of 3D chromatin architecture during early mammalian development. Nature 547, 232–235. doi:10.1038/nature23263
Falk, M., Feodorova, Y., Naumova, N., Imakaev, M., Lajoie, B. R., Leonhardt, H., et al. (2019). Heterochromatin drives compartmentalization of inverted and conventional nuclei. Nature 570, 395–399. doi:10.1038/s41586-019-1275-3
Finn, E. H., Pegoraro, G., Brandão, H. B., Valton, A.-L., Oomen, M. E., Dekker, J., et al. (2019). Extensive heterogeneity and intrinsic variation in spatial genome organization. Cell 176, 1502–1515. e10. doi:10.1016/j.cell.2019.01.020
Flyamer, I. M., Gassler, J., Imakaev, M., Brandão, H. B., Ulianov, S. V., Abdennur, N., et al. (2017). Single-nucleus Hi-C reveals unique chromatin reorganization at oocyte-to-zygote transition. Nature 544, 110–114. doi:10.1038/nature21711
Fraser, J., Williamson, I., Bickmore, W. A., and Dostie, J. (2015). An overview of genome organization and How we got there: From FISH to Hi-C. Microbiol. Mol. Biol. Rev. 79, 347–372. doi:10.1128/MMBR.00006-15
Galitsyna, A. A., and Gelfand, M. S. (2021). Single-cell Hi-C data analysis: Safety in numbers. Brief. Bioinform. 22, bbab316. doi:10.1093/bib/bbab316
Gassler, J., Brandão, H. B., Imakaev, M., Flyamer, I. M., Ladstätter, S., Bickmore, W. A., et al. (2017). A mechanism of cohesin‐dependent loop extrusion organizes zygotic genome architecture. EMBO J. 36, 3600–3618. doi:10.15252/embj.201798083
Ghavi-Helm, Y., Jankowski, A., Meiers, S., Viales, R. R., Korbel, J. O., and Furlong, E. E. M. (2019). Highly rearranged chromosomes reveal uncoupling between genome topology and gene expression. Nat. Genet. 51, 1272–1282. doi:10.1038/s41588-019-0462-3
Gürsoy, G., Xu, Y., Kenter, A. L., and Liang, J. (2014). Spatial confinement is a major determinant of the folding landscape of human chromosomes. Nucleic Acids Res. 42, 8223–8230. doi:10.1093/nar/gku462
Hsieh, T.-H. S., Cattoglio, C., Slobodyanyuk, E., Hansen, A. S., Rando, O. J., Tjian, R., et al. (2020). Resolving the 3D landscape of transcription-linked mammalian chromatin folding. Mol. Cell 78, 539–553. e8. doi:10.1016/j.molcel.2020.03.002
Hsieh, T.-H. S., Fudenberg, G., Goloborodko, A., and Rando, O. J. (2016). Micro-C XL: Assaying chromosome conformation from the nucleosome to the entire genome. Nat. Methods 13, 1009–1011. doi:10.1038/nmeth.4025
Hsieh, T.-H. S., Weiner, A., Lajoie, B., Dekker, J., Friedman, N., and Rando, O. J. (2015). Mapping nucleosome resolution chromosome folding in yeast by Micro-C. Cell 162, 108–119. doi:10.1016/j.cell.2015.05.048
Jerkovic´, I., and Cavalli, G. (2021). Understanding 3D genome organization by multidisciplinary methods. Nat. Rev. Mol. Cell Biol. 22, 511–528. doi:10.1038/s41580-021-00362-w
Ke, Y., Xu, Y., Chen, X., Feng, S., Liu, Z., Sun, Y., et al. (2017). 3D chromatin structures of mature gametes and structural reprogramming during mammalian embryogenesis. Cell 170, 367–381. e20. doi:10.1016/j.cell.2017.06.029
Kim, H. J., Yardimci, G. G., Bonora, G., Ramani, V., Liu, J., Qiu, R., et al. (2020). Capturing cell type-specific chromatin compartment patterns by applying topic modeling to single-cell Hi-C data. PLoS Comput. Biol. 16 (9), e1008173. doi:10.1371/journal.pcbi.1008173
Krietenstein, N., Abraham, S., Venev, S. V., Abdennur, N., Gibcus, J., Hsieh, T.-H. S., et al. (2020). Ultrastructural details of mammalian chromosome architecture. Mol. Cell 78, 554–565. e7. doi:10.1016/j.molcel.2020.03.003
Lando, D., Stevens, T. J., Basu, S., and Laue, E. D. (2018). Calculation of 3D genome structures for comparison of chromosome conformation capture experiments with microscopy: An evaluation of single-cell Hi-C protocols. Nucleus 9, 190–201. doi:10.1080/19491034.2018.1438799
Lee, D.-S., Luo, C., Zhou, J., Chandran, S., Rivkin, A., Bartlett, A., et al. (2019). Simultaneous profiling of 3D genome structure and DNA methylation in single human cells. Nat. Methods 16, 999–1006. doi:10.1038/s41592-019-0547-z
Li, G., Liu, Y., Zhang, Y., Kubo, N., Yu, M., Fang, R., et al. (2019). Joint profiling of DNA methylation and chromatin architecture in single cells. Nat. Methods 16, 991–993. doi:10.1038/s41592-019-0502-z
Li, X., Zeng, G., Li, A., and Zhang, Z. (2021). DeTOKI identifies and characterizes the dynamics of chromatin TAD-like domains in a single cell. Genome Biol. 22, 217. doi:10.1186/s13059-021-02435-7
Li, X., Lee, L., Abnousi, A., Yu, M., Liu, W., Huang, L., et al. (2022). SnapHiC2: A computationally efficient loop caller for single cell Hi-C data. Comput. Struct. Biotechnol. J. 20, 2778–2783. doi:10.1016/j.csbj.2022.05.046
Liang, J., and Perez-Rathke, A. (2021). Minimalistic 3D chromatin models: Sparse interactions in single cells drive the chromatin fold and form many-body units. Curr. Opin. Struct. Biol. 71, 200–214. doi:10.1016/j.sbi.2021.06.017
Lieberman-Aiden, E., van Berkum, N. L., Williams, L., Imakaev, M., Ragoczy, T., Telling, A., et al. (2009). Comprehensive mapping of long-range interactions reveals folding principles of the human genome. Science 326, 289–293. doi:10.1126/science.1181369
Luppino, J. M., Park, D. S., Nguyen, S. C., Lan, Y., Xu, Z., Yunker, R., et al. (2020). Cohesin promotes stochastic domain intermingling to ensure proper regulation of boundary-proximal genes. Nat. Genet. 52, 840–848. doi:10.1038/s41588-020-0647-9
Maslova, A., and Krasikova, A. (2021). FISH going meso-scale: A microscopic search for chromatin domains. Front. Cell Dev. Biol. 9, 753097. doi:10.3389/fcell.2021.753097
Mateo, L. J., Murphy, S. E., Hafner, A., Cinquini, I. S., Walker, C. A., and Boettiger, A. N. (2019). Visualizing DNA folding and RNA in embryos at single-cell resolution. Nature 568, 49–54. doi:10.1038/s41586-019-1035-4
Mirny, L., and Dekker, J. (2021a04014). Mechanisms of chromosome folding and nuclear organization: Their interplay and open questions. Cold Spring Harb. Perspect. Biol. 14, a040147. doi:10.1101/cshperspect.a040147
Nagano, T., Lubling, Y., Stevens, T. J., Schoenfelder, S., Yaffe, E., Dean, W., et al. (2013). Single-cell Hi-C reveals cell-to-cell variability in chromosome structure. Nature 502, 59–64. doi:10.1038/nature12593
Nagano, T., Lubling, Y., Várnai, C., Dudley, C., Leung, W., Baran, Y., et al. (2017). Cell-cycle dynamics of chromosomal organization at single-cell resolution. Nature 547, 61–67. doi:10.1038/nature23001
Nguyen, H. Q., Chattoraj, S., Castillo, D., Nguyen, S. C., Nir, G., Lioutas, A., et al. (2020). 3D mapping and accelerated super-resolution imaging of the human genome using in situ sequencing. Nat. Methods 17, 822–832. doi:10.1038/s41592-020-0890-0
Nir, G., Farabella, I., Pérez Estrada, C., Ebeling, C. G., Beliveau, B. J., Sasaki, H. M., et al. (2018). Walking along chromosomes with super-resolution imaging, contact maps, and integrative modeling. PLoS Genet. 14, e1007872. doi:10.1371/journal.pgen.1007872
Nora, E. P., Goloborodko, A., Valton, A.-L., Gibcus, J. H., Uebersohn, A., Abdennur, N., et al. (2017). Targeted degradation of CTCF decouples local insulation of chromosome domains from genomic compartmentalization. Cell 169, 930–944. e22. doi:10.1016/j.cell.2017.05.004
Nora, E. P., Lajoie, B. R., Schulz, E. G., Giorgetti, L., Okamoto, I., Servant, N., et al. (2012). Spatial partitioning of the regulatory landscape of the X-inactivation centre. Nature 485, 381–385. doi:10.1038/nature11049
Pang, Q. Y., Tan, T. Z., Sundararajan, V., Chiu, Y.-C., Chee, E. Y. W., Chung, V. Y., et al. (2022). 3D genome organization in the epithelial-mesenchymal transition spectrum. Genome Biol. 23, 121. doi:10.1186/s13059-022-02687-x
Payne, A. C., Chiang, Z. D., Reginato, P. L., Mangiameli, S. M., Murray, E. M., Yao, C.-C., et al. (2021). In situ genome sequencing resolves DNA sequence and structure in intact biological samples. Science 371, eaay3446. doi:10.1126/science.aay3446
Polovnikov, K., Gorsky, A., Nechaev, S., Razin, S. V., and Ulianov, S. V. (2020). Non-backtracking walks reveal compartments in sparse chromatin interaction networks. Sci. Rep. 10, 11398. doi:10.1038/s41598-020-68182-0
Ramani, V., Deng, X., Qiu, R., Gunderson, K. L., Steemers, F. J., Disteche, C. M., et al. (2017). Massively multiplex single-cell Hi-C. Nat. Methods 14, 263–266. doi:10.1038/nmeth.4155
Rao, S. S. P., Huang, S.-C., Glenn St Hilaire, B., Engreitz, J. M., Perez, E. M., Kieffer-Kwon, K.-R., et al. (2017). Cohesin loss eliminates all loop domains. Cell 171, 305–320. e24. doi:10.1016/j.cell.2017.09.026
Rao, S. S. P., Huntley, M. H., Durand, N. C., Stamenova, E. K., Bochkov, I. D., Robinson, J. T., et al. (2014). A 3D map of the human genome at kilobase resolution reveals principles of chromatin looping. Cell 159, 1665–1680. doi:10.1016/j.cell.2014.11.021
Schwartzman, O., and Tanay, A. (2015). Single-cell epigenomics: Techniques and emerging applications. Nat. Rev. Genet. 16, 716–726. doi:10.1038/nrg3980
Schwarzer, W., Abdennur, N., Goloborodko, A., Pekowska, A., Fudenberg, G., Loe-Mie, Y., et al. (2017). Two independent modes of chromatin organization revealed by cohesin removal. Nature 551, 51–56. doi:10.1038/nature24281
Sexton, T., Yaffe, E., Kenigsberg, E., Bantignies, F., Leblanc, B., Hoichman, M., et al. (2012). Three-dimensional folding and functional organization principles of the Drosophila genome. Cell 148, 458–472. doi:10.1016/j.cell.2012.01.010
Stevens, T. J., Lando, D., Basu, S., Atkinson, L. P., Cao, Y., Lee, S. F., et al. (2017). 3D structures of individual mammalian genomes studied by single-cell Hi-C. Nature 544, 59–64. doi:10.1038/nature21429
Su, J.-H., Zheng, P., Kinrot, S. S., Bintu, B., and Zhuang, X. (2020). Genome-scale imaging of the 3D organization and transcriptional activity of chromatin. Cell 182, 1641–1659. e26. doi:10.1016/j.cell.2020.07.032
Szabo, Q., Donjon, A., Jerković, I., Papadopoulos, G. L., Cheutin, T., Bonev, B., et al. (2020). Regulation of single-cell genome organization into TADs and chromatin nanodomains. Nat. Genet. 52, 1151–1157. doi:10.1038/s41588-020-00716-8
Takei, Y., Yun, J., Zheng, S., Ollikainen, N., Pierson, N., White, J., et al. (2021). Integrated spatial genomics reveals global architecture of single nuclei. Nature 590, 344–350. doi:10.1038/s41586-020-03126-2
Tan, L., Ma, W., Wu, H., Zheng, Y., Xing, D., Chen, R., et al. (2021). Changes in genome architecture and transcriptional dynamics progress independently of sensory experience during post-natal brain development. Cell 184, 741–758.e17. e17. doi:10.1016/j.cell.2020.12.032
Tan, L., Xing, D., Chang, C.-H., Li, H., and Xie, X. S. (2018). Three-dimensional genome structures of single diploid human cells. Science 361, 924–928. doi:10.1126/science.aat5641
Tan, L., Xing, D., Daley, N., and Xie, X. S. (2019). Three-dimensional genome structures of single sensory neurons in mouse visual and olfactory systems. Nat. Struct. Mol. Biol. 26, 297–307. doi:10.1038/s41594-019-0205-2
Ulianov, S. V., and Razin, S. V. (2021). The two waves in single-cell 3D genomics. Amsterdam, Netherlands: Elsevier.
Ulianov, S. V., Tachibana-Konwalski, K., and Razin, S. V. (2017). Single-cell Hi-C bridges microscopy and genome-wide sequencing approaches to study 3D chromatin organization. BioEssays 39, 1700104. doi:10.1002/bies.201700104
Ulianov, S. V., Zakharova, V. V., Galitsyna, A. A., Kos, P. I., Polovnikov, K. E., Flyamer, I. M., et al. (2021). Order and stochasticity in the folding of individual Drosophila genomes. Nat. Commun. 12, 41. doi:10.1038/s41467-020-20292-z
van Arensbergen, J., van Steensel, B., and Bussemaker, H. J. (2014). In search of the determinants of enhancer–promoter interaction specificity. Trends Cell Biol. 24, 695–702. doi:10.1016/j.tcb.2014.07.004
Vasquez, P. A., Hult, C., Adalsteinsson, D., Lawrimore, J., Forest, M. G., and Bloom, K. (2016). Entropy gives rise to topologically associating domains. Nucleic Acids Res. 44, 5540–5549. doi:10.1093/nar/gkw510
Wang, S., Su, J.-H., Beliveau, B. J., Bintu, B., Moffitt, J. R., Wu, C., et al. (2016). Spatial organization of chromatin domains and compartments in single chromosomes. Science 353, 598–602. doi:10.1126/science.aaf8084
Winick-Ng, W., Kukalev, A., Harabula, I., Zea-Redondo, L., Szabo, D., Meijer, M., et al. (2021). Cell-type specialization is encoded by specific chromatin topologies. Nature 599, 684–691. doi:10.1038/s41586-021-04081-2
Xie, W. J., Meng, L., Liu, S., Zhang, L., Cai, X., and Gao, Y. Q. (2017). Structural modeling of chromatin integrates genome features and reveals chromosome folding principle. Sci. Rep. 7, 2818. doi:10.1038/s41598-017-02923-6
You, Q., Cheng, A. Y., Gu, X., Harada, B. T., Yu, M., Wu, T., et al. (2021). Direct DNA crosslinking with CAP-C uncovers transcription-dependent chromatin organization at high resolution. Nat. Biotechnol. 39, 225–235. doi:10.1038/s41587-020-0643-8
Yu, M., Abnousi, A., Zhang, Y., Li, G., Lee, L., Chen, Z., et al. (2021). SnapHiC: A computational pipeline to identify chromatin loops from single-cell Hi-C data. Nat. Methods 18, 1056–1059. doi:10.1038/s41592-021-01231-2
Zhang, R., Zhou, T., and Ma, J. (2021). Multiscale and integrative single-cell Hi-C analysis with Higashi. Nat. Biotechnol. 40, 254–261. doi:10.1038/s41587-021-01034-y
Zheng, H., and Xie, W. (2019). The role of 3D genome organization in development and cell differentiation. Nat. Rev. Mol. Cell Biol. 20, 535–550. doi:10.1038/s41580-019-0132-4
Zhou, J., Ma, J., Chen, Y., Cheng, C., Bao, B., Peng, J., et al. (2019a). Robust single-cell Hi-C clustering by convolution- and random-walk–based imputation. Proc. Natl. Acad. Sci. U. S. A. 116, 14011–14018. doi:10.1073/pnas.1901423116
Zhou, S., Jiang, W., Zhao, Y., and Zhou, D.-X. (2019b). Single-cell three-dimensional genome structures of rice gametes and unicellular zygotes. Nat. Plants 5, 795–800. doi:10.1038/s41477-019-0471-3
Zhou, T., Zhang, R., and Ma, J. (2021). The 3D genome structure of single cells. Annu. Rev. Biomed. Data Sci. 4, 21–41. doi:10.1146/annurev-biodatasci-020121-084709
Keywords: genome architecture, epigenetic regulation, spatial omics, DNA folding, chromatin structure
Citation: Chi Y, Shi J, Xing D and Tan L (2022) Every gene everywhere all at once: High-precision measurement of 3D chromosome architecture with single-cell Hi-C. Front. Mol. Biosci. 9:959688. doi: 10.3389/fmolb.2022.959688
Received: 06 June 2022; Accepted: 06 September 2022;
Published: 06 October 2022.
Edited by:
Andrzej Stasiak, Université de Lausanne, SwitzerlandReviewed by:
Ilya M. Flyamer, Friedrich Miescher Institute for Biomedical Research (FMI), SwitzerlandCopyright © 2022 Chi, Shi, Xing and Tan. This is an open-access article distributed under the terms of the Creative Commons Attribution License (CC BY). The use, distribution or reproduction in other forums is permitted, provided the original author(s) and the copyright owner(s) are credited and that the original publication in this journal is cited, in accordance with accepted academic practice. No use, distribution or reproduction is permitted which does not comply with these terms.
*Correspondence: Longzhi Tan, dHR0dEBzdGFuZm9yZC5lZHU=; Dong Xing, ZHhpbmdAcGt1LmVkdS5jbg==
Disclaimer: All claims expressed in this article are solely those of the authors and do not necessarily represent those of their affiliated organizations, or those of the publisher, the editors and the reviewers. Any product that may be evaluated in this article or claim that may be made by its manufacturer is not guaranteed or endorsed by the publisher.
Research integrity at Frontiers
Learn more about the work of our research integrity team to safeguard the quality of each article we publish.