- 1Department of Integrated Traditional Chinese and Western Medicine, Zigong First People’s Hospital, Zigong, China
- 2Department of Integrated Traditional Chinese and Western Medicine, West China Hospital of Sichuan University, Chengdu, China
- 3Department of Pharmacy, The Second Affiliated Hospital of Kunming Medical University, Kunming, China
- 4Division of Pulmonary Diseases, Department of Respiratory and Critical Care Medicine, West China Hospital of Sichuan University, Chengdu, China
- 5School of Automation and Information Engineering, Sichuan University of Science and Engineering, Zigong, China
Background: An imbalance in the redox homeostasis has been reported in multiple cancers and is associated with a poor prognosis of disease. However, the prognostic value of redox-related genes in non-small-cell lung cancer (NSCLC) remains unclear.
Methods: RNA sequencing data, DNA methylation data, mutation, and clinical data of NSCLC patients were downloaded from The Cancer Genome Atlas and Gene Expression Omnibus databases. Redox-related differentially expressed genes (DEGs) were used to construct the prognostic signature using least absolute shrinkage and selection operator (LASSO) regression analysis. Kaplan–Meier survival curve and receiver operator characteristic (ROC) curve analyses were applied to validate the accuracy of the gene signature. Nomogram and calibration plots of the nomogram were constructed to predict prognosis. Pathway analysis was performed using gene set enrichment analysis. The correlations of risk score with tumor stage, immune infiltration, DNA methylation, tumor mutation burden (TMB), and chemotherapy sensitivity were evaluated. The prognostic signature was validated using GSE31210, GSE26939, and GSE68465 datasets. Real-time polymerase chain reaction (PCR) was used to validate dysregulated genes in NSCLC.
Results: A prognostic signature was constructed using the LASSO regression analysis and was represented as a risk score. The high-risk group was significantly correlated with worse overall survival (OS) (p < 0.001). The area under the ROC curve (AUC) at the 5-year stage was 0.657. The risk score was precisely correlated with the tumor stage and was an independent prognostic factor for NSCLC. The constructed nomogram accurately predicted the OS of patients after 1-, 3-, and 5-year periods. DNA replication, cell cycle, and ECM receptor interaction were the main pathways enriched in the high-risk group. In addition, the high-risk score was correlated with higher TMB, lower methylation levels, increased infiltrating macrophages, activated memory CD4+ T cells, and a higher sensitivity to chemotherapy. The signature was validated in GSE31210, GSE26939, and GSE68465 datasets. Real-time PCR validated dysregulated mRNA expression levels in NSCLC.
Conclusions: A prognostic redox-related gene signature was successfully established in NSCLC, with potential applications in the clinical setting.
Introduction
Lung cancer is the leading cause of cancer-related deaths worldwide, with an estimated 1.8 million deaths (18% of the total number of cancer deaths) (Sung et al., 2021). Non-small cell lung cancer (NSCLC) is a major type of lung cancer, accounting for approximately 85% of all lung cancers (Travis et al., 2015a; Travis et al., 2015b). Only 25% of patients with NSCLC are diagnosed during the early stages (Ni et al., 2018). Furthermore, cancer in approximately 80% of the diagnosed patients is already in the metastatic stage (Goldstraw et al., 2016). Despite recent progress in novel medications, such as targeted therapies and immunotherapies for NSCLC (Facchinetti et al., 2016), the 5 years survival rate remains below 15% (Goldstraw et al., 2016). Therefore, an effective biomarker is urgently required to predict the overall survival (OS) of patients diagnosed with NSCLC.
Advancements in the next-generation sequencing technology have identified multiple biomarkers correlated with prognosis, immune infiltration, and therapy in lung cancer, such as adhesion molecule interacting with CXADR antigen 1 (AMICA1) (Feng et al., 2022), chromobox protein homolog 3 (CBX3) (Niu et al., 2022), and lysine acetyltransferase 2B (KAT2B) (Zhou et al., 2022). However, the researchers have demonstrated that the construction of gene signature could predict better prognosis and therapy response. For instance, hypoxia-related gene signature (Chen et al., 2022), pyroptosis-related gene signature (Zhang and Yan, 2021; Li et al., 2022), autophagy-related gene signature (Wu et al., 2021a), and inflammatory response-related signature (Zou et al., 2021) may better predict prognosis, influence immunological state and guide individualized therapy due to its multidimensional parameters.
Oxidative stress is typically caused by the accumulation of reactive oxygen species (ROS) (Li et al., 2021a). ROS production in cells can cause oxidative damage resulting from DNA point mutations (Lee et al., 2002; Ogrunc et al., 2014), disrupted lipid membranes (Halliwell and Chirico, 1993), and altered protein function (LeDoux et al., 1999; Kwon et al., 2004; Gao et al., 2007). Such oxidative damages are countered by antioxidant systems (Handy and Loscalzo, 2012), which scavenge harmful ROS. Normal ROS and antioxidant levels maintain redox homeostasis in healthy cells, while increased ROS levels are observed in cancer cells. These increased levels lead to genomic instability, facilitate cell proliferation, increase motility, and activate oncogenic signaling (Moloney and Cotter, 2018; Forman and Zhang, 2021). ROS can participate in several cancer signaling pathways implicated in tumor growth, metabolism, differentiation, and metastasis (Aggarwal et al., 2019). Importantly, redox imbalance has also been observed in NSCLC (Weng et al., 2018; Zhao et al., 2021a). For example, antioxidant enzymes including superoxide dismutase (SOD), catalase (CAT), and glutathione peroxidase (GPX) are all reduced in NSCLC in comparison with corresponding controls (Zalewska-Ziob et al., 2019), and glutathione (GSH) and its related enzymes that detoxify ROS, are increased in lung cancer (Luengo et al., 2019). Cellular ROS level is usually regulated by nuclear factor-erythroid 2-related factor 2 (NRF2) and its repressor Kelch-like ECH-associated protein 1 (KEAP1) (Zhang et al., 2015). Large-scale genomic studies have revealed that the KEAP1/NRF2 pathway is altered in 23% of lung adenocarcinoma (LUAD) and 34% of lung squamous cell carcinoma (LUSC), approximately (The Cancer Genome Atlas Research Network, 2014). Moreover, the upregulated activity and expression of the NADPH oxidase (NOX) family correlate with tumorigenesis of lung cancer through ROS production (Han et al., 2016). Additionally, cigarette smoking is the most important risk factor for lung cancer, which accounts for nearly two-thirds of all lung cancer cases (Hedyed et al., 2021), and cigarette smoking could induce oxidative stress and mitochondrial damage leading to metabolic reprogramming associated with increased glycolytic flux, contributing to epithelial-mesenchymal transition processes, and cell migration, therefore, promoting tumor progression (Di Vincenzo et al., 2021). However, there are no systematic studies on the role of redox-related genes in NSCLC. Thus, a better understanding of the molecular composition of these genes and their roles in NSCLC is necessary for its accurate prognosis and treatment.
In our study, a redox-related gene signature was established using the least absolute shrinkage and selection operator (LASSO) regression analysis to predict the OS, immune infiltration, and evaluate therapy response in patients diagnosed with NSCLC.
Materials and methods
Data preprocessing and identification of DEGs
RNA sequencing data, DNA methylation data, mutation data, and clinical information of patients with NSCLC were downloaded from the cancer genome atlas (TCGA) (https://www.cancer.gov/about-nci/organization/ccg/research/structural-genomics/tcga). A total of 1,037 cancer and 108 normal samples with sequencing data, 807 cancer and 71 normal samples with DNA methylation data, 1,059 tumor samples with mutation data, and 1,027 tumor samples with clinical data were obtained. The GSE31210, GSE68465, and GSE26939 datasets were downloaded from gene expression omnibus (GEO) database (https://www.ncbi.nlm.nih.gov/geo/), which included 226, 442, 116 patients diagnosed with NSCLC, respectively. GSE31210 was from GPL570 [HG-U133_Plus_2] Affymetrix Human Genome U133 Plus 2.0 Array, GSE68465 from GPL96 [HG-U133A] Affymetrix Human Genome U133A Array, and GSE26939 from GPL9053 Agilent-UNC-custom-4X44K. Patients with incomplete follow-up were excluded from the analysis. Therefore, TCGA cohort, GSE31210, GSE68465, and GSE26939 datasets included 978, 226, 442, and 114 patients with complete follow-up information, respectively. Baseline characteristics of patients with NSCLC are listed in Table 1. In TCGA database, 767 and 754 patients with complete follow-up data have mutation data, and methylation data, respectively. The sequencing data from TCGA database and GEO database were analyzed as the training cohort and test cohort, respectively. The redox-related gene sets were obtained from GSEA-MSigDB database (https://www.gsea-msigdb.org/gsea/msigdb), and two redox-related gene sets, namely “GO_CELL_REDOX_HOMEOSTASIS” and “GO_RESPONSE_TO_REDOX_STATE”, were downloaded for further analysis. A total of 4,087 redox-related genes were included in the current study (Supplementary Table S1) (Cook et al., 2011; Gerstberger et al., 2014). The redox-related differentially expressed genes (DEGs) were identified in TCGA cohort using the “limma package” in R programming language (Version 4.0.2) based on |log2 fold change (FC)| ≥ 2 and adjusted p-value < 0.05.
Construction and validation of the prognostic signature
Univariate Cox regression analysis was performed to identify prognostic genes using redox-related DEGs with p < 0.05. Subsequently, a prognostic signature was constructed using LASSO regression analysis following a linear combination of gene expression values multiplied by a regression coefficient (β). A formula was as follows: risk score = ∑ (coefficient i × expression of gene i). All patients could obtain a risk score using the formula and be divided into the low-risk or high-risk groups according to the median risk score. Kaplan–Meier survival curve was calculated between the two risk groups and was compared using the log-rank test. Receiver operator characteristic (ROC) curve analysis was used to assess the predictive accuracy of the prognostic signature, and area under the ROC curve (AUC) values with 95% confidence intervals (CIs) were calculated. The prognostic signature was validated using GSE31210, GSE68465, and GSE26939 datasets.
Correlation of risk score with clinical characteristics
The risk score in different age groups (≥65 and <65 years), biological sexes (female and male), Union for International Cancer Control (UICC) stages (stages I, II, III, and IV), T stages (T1, T2, T3, and T4), N stages (N0, N1, N2, and N3), and M stages (M0 and M1) were compared using R programming language in TCGA cohort. The clinical characteristics including age, gender, smoking history, UICC stage, and risk score were applied to identify independent prognostic factors contributing to NSCLC using univariate and multivariate Cox regression analyses.
Nomogram prediction model
A Nomogram prediction model was constructed to predict the 1-, 3-, and 5-years OS rates using independent prognostic factors with the “rms package” in R programming language, and calibration plots of the nomogram were applied to compare the nomogram-predicted probability of OS and the actual OS.
Gene set enrichment analysis
Gene set enrichment analysis (GSEA) is a computational method that determines whether a priori defined set of genes shows statistically significant and concordant differences between two biological states (Subramanian et al., 2005). The high- and low-risk groups were defined according to the median risk score in NSCLC. GSEA (version 4.0.3) was applied to perform Kyoto Encyclopedia of Genes and Genomes (KEGG) pathway analysis between the high- and low-risk expression groups.
Analysis of DNA methylation and mutation
The DNA methylation data were normalized as the beta value in TCGA database and the values of DNA methylation expression were excluded when their value was missing or zero. The analysis of DNA methylation was performed using the patients with DNA methylation data and complete follow-up data. DNA methylation levels of prognostic genes were compared between the high- and low-risk groups. The mutation data of LUAD and LUSC were allocated in two different files in TCGA database, thus gene mutation frequency of the two risk groups was calculated in LUAD, and LUSC, respectively, using the “maftools package” in R programming language. The correlation of risk score with TMB was evaluated in all NSCLC using the “ggpubr package” and “reshape2 package” in R programming language, and statistical analysis was performed using the Mann–Whitney test and Spearman rank correlation analysis. Kaplan–Meier survival curve was calculated between the high-risk score + high TMB group and the low-risk score + low TMB group and was compared using the log-rank test.
Analysis of immune infiltration
The gene expression matrix of NSCLC firstly converted tumor micro-environment score matrix using “commonGenes.gct,” “estimateScore.gct,” and “uniq.symbol.txt” in R programming language, and then immune-infiltration profiles were evaluated between the two risk groups and displayed in a violin plot by using the “vioplot” package. The correlation of the risk score with immune infiltration was calculated and displayed using Spearman rank correlation analysis with “limma package,” “reshape2 package,” “tidyverse package,” “ggplot2 package,” “ggpubr package,” and “ggExtra package” in R programming language.
Analysis of chemotherapy sensitivity
To assess the clinical therapeutic value of prognostic signature, the half inhibitory concentration (IC50) of different chemotherapy drugs was evaluated using all patients in prognostic signature with “pRRophetic packages” in R programming language. Nine chemotherapy drugs, namely bortezomib (Wang et al., 2022a), dasatinib (Kim et al., 2021; Redin et al., 2021), docetaxel (Xiao et al., 2022a; Matsumoto et al., 2022), midostaurin (Ctortecka et al., 2018), paclitaxel (Duan et al., 2022), parthenolide (Li et al., 2020a), pazopanib (Tanimoto et al., 2018), shikonin (Pan et al., 2021), and thapsigargin (Jaskulska et al., 2020) were collected from the previous literature, which has proven to have the potential of anti-tumor in lung cancer.
Real-time polymerase chain reaction validation
Sixteen paired lung tissues were obtained from patients with NSCLC in Zigong First People’s Hospital, and the clinical information was summarized in Table 2. Total RNA was extracted by using the E. Z.N.A. HP Total RNA Kit (Omega, GA, United States ). Complementary DNA (cDNA) was synthesized by using the PrimeScript™ RT reagent kit (Takara, Japan). Real-time PCR was performed by using iQ ™ SYBR Green Supermix (Bio-Rad). Levels of gene relative expression were normalized to β-actin Ct value, using a 2−ΔΔCt relative quantification method. The primer sequences used were listed in Table 3.
Statistical analysis
All statistical analyses were performed using R software (version 4.0.2) or GraphPad Prism (version 7.00). Comparisons between the two groups were quantified using Student’s t-test for parametric data or the Mann–Whitney test for nonparametric data. Statistical significance among multiple groups was tested using the Kruskal–Wallis test or analysis of variance (ANOVA). Statistical significance was set at values of p < 0.05.
Results
Identification of DEGs
The flowchart depicting the procedure followed in the study is shown in Figure 1. A total of 388 DEGs (279 up-regulated and 109 down-regulated genes) were identified in TCGA cohort when cancer tissues compared with control tissues.
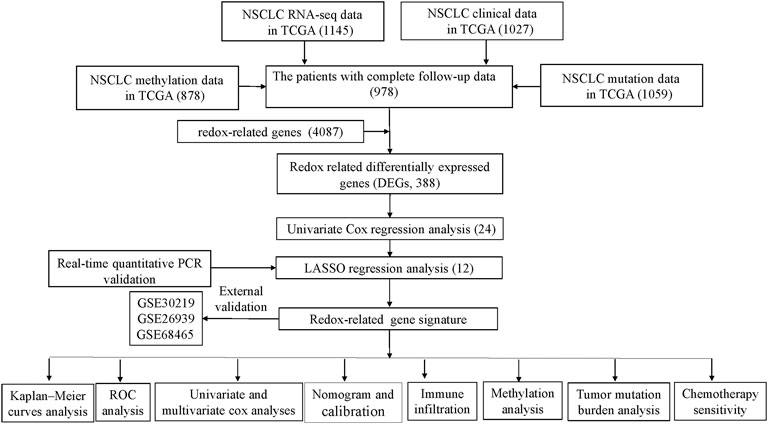
FIGURE 1. Schematic flowchart representing the procedure followed for establishing the gene signature, and the main findings of the study. Numbers within parenthesis indicate the size of the sample obtained. NSCLC, Non-small cell lung cancer; PCR, polymerase chain reaction; LASSO, least absolute shrinkage, and selection operator; ROC: receiver operator characteristic; TCGA, The Cancer Genome Atlas.
Construction of a prognostic signature in the TCGA cohort
Among the 388 DEGs, a total of 24 genes were correlated with prognosis using univariate Cox regression analysis (Figure 2A). Furthermore, a prognostic signature comprising 12 genes (CDC25C, GRIA1, CHEK2, KLK6, S100P, SPP1, COL1A1, CAV1, SCN1A, CYP24A1, GPR37, and SLC7A5) was constructed using the LASSO regression analysis (Figures 2B–D). The risk score for each patient was calculated as follows:
Then, based on the median risk score of 0.9403, 978 patients were divided into the high- and low-risk subgroups (Figure 2G). Kaplan–Meier survival curve showed that the high-risk group had a worse OS than the low-risk group (p < 0.001; Figure 2E), and the AUC values for OS at the 1-, 3-, 5-year periods were 0.674, 0.671, and 0.657, respectively (Figure 2F). Patients in the high-risk group had higher mortality rates than those in the low-risk group (Figure 2H).
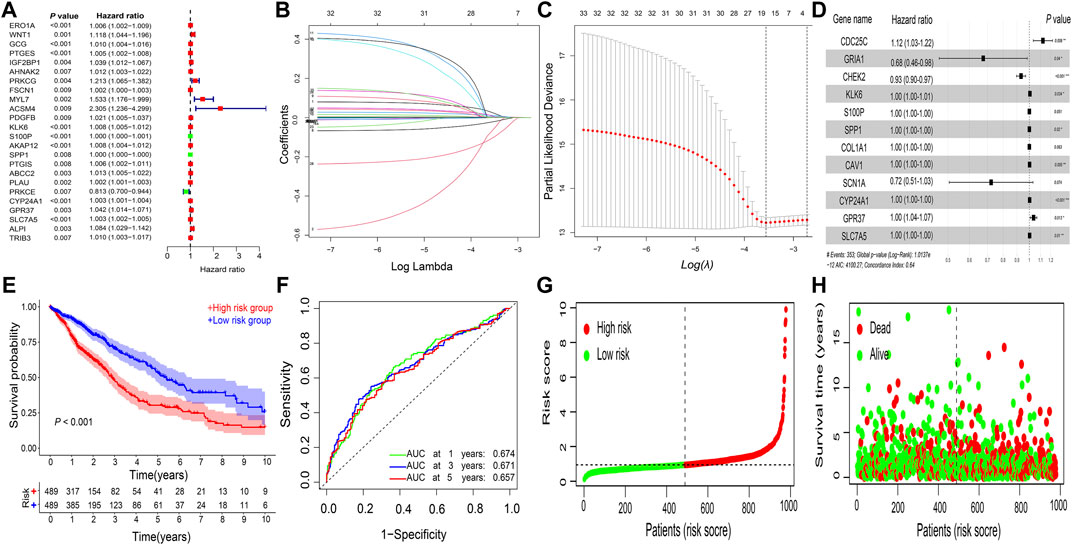
FIGURE 2. Construction of gene signature. Gene signature constructed using (A) univariate Cox regression analysis and (B–D) LASSO regression analysis in NSCLC. (E) Kaplan–Meier survival analysis. (F) ROC curve analysis. (G) risk score distribution. (H) survival status of the prognostic gene signature. LASSO, least absolute shrinkage and selection operator; NSCLC, non-small cell lung cancer; ROC, receiver operating characteristic; AUC, area under the ROC curve.
Validation of the prognostic signature in GEO datasets
In GSE31210 dataset, compared with the low-risk group, the high-risk group had a worse OS (p < 0.001; Figure 3A), and the 1-, 3-, 5-years AUC was 0.687, 0.700, and 0.717, respectively (Figure 3B). Additionally, patients in the high-risk group have also a worse OS in comparison with the low-risk group in both GSE68465 and GSE26939 datasets (p < 0.001; Figures 3C,D).
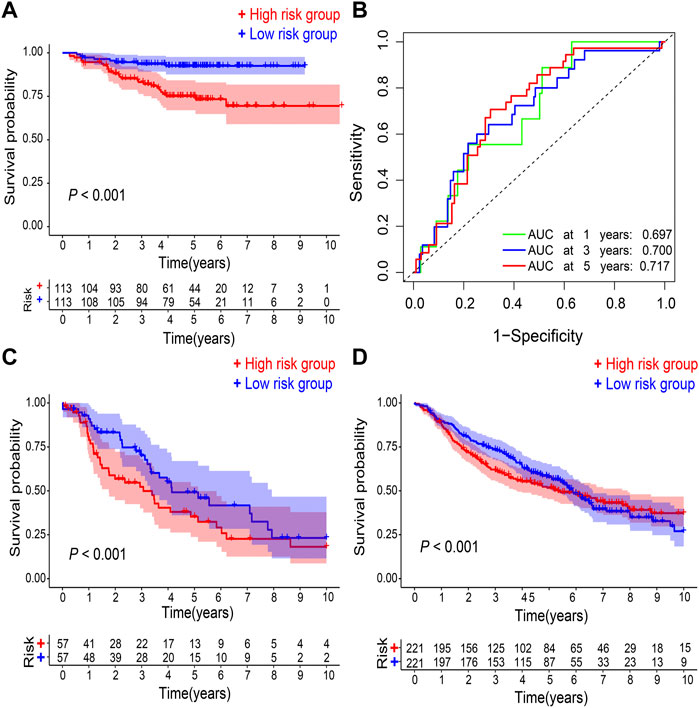
FIGURE 3. Validation of gene signature. (A) Kaplan–Meier survival analysis and (B) ROC curve analysis in GSE31210. Kaplan–Meier survival analysis in (C) GSE26939 and (D) GSE68465, respectively. ROC, receiver operating characteristic. AUC, an area under the ROC curve.
Correlation of risk score with clinical characteristics
The risk score in patients with stage II (p = 0.066), stage III (p < 0.001), and stage IV (p = 0.010) cancer was higher than that in patients with stage I cancer. (Figure 4A). Patients with T2 (p = 0.004) and T3 (p = 0.003) had higher risk scores than those with T1 (Figure 4B). The risk scores of patients with N2-3 were higher than those of patients with N0 (p = 0.002) and N1 (p = 0.002) (Figure 4C). The patients with M1 had a higher risk score than those with M0 (p = 0.036, Figure 4D). The male patients have a higher risk score compared with female patients (p = 0.002 Figure 4E). The risk score did not differ significantly between the different age groups (Figure 4F).
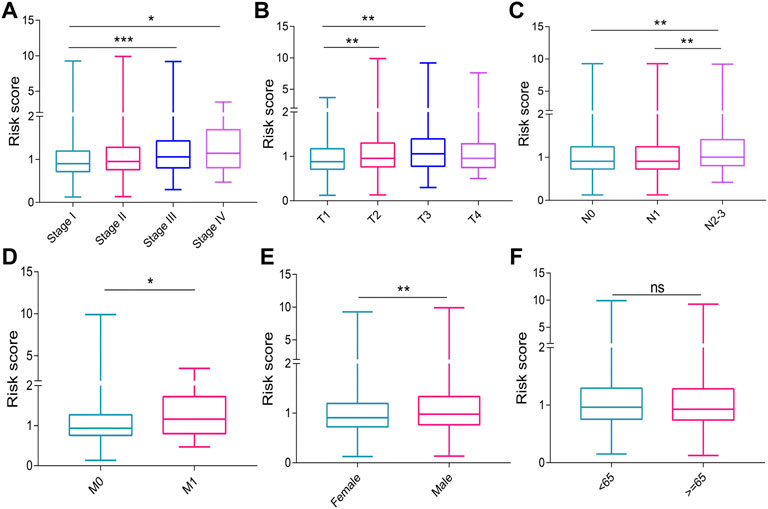
FIGURE 4. Graphs showing the correlation of risk score with clinical characteristics. (A) UICC stages. (B) T stages. (C) N stages. (D) M stages. (E) genders. (F) ages. UICC, Union for International Cancer Control. ns, no significance. *p < 0.05; **p < 0.01; ***p < 0.001.
Risk score as an independent NSCLC prognostic factor
Univariate Cox regression analysis showed that the age (hazard ratio [HR] = 1.014; p = 0.025), UICC stage (HR = 1.475; p < 0.001), and allocated risk score (HR = 1.427; p < 0.001) were correlated with prognosis in TCGA cohort (Figure 5A). This was confirmed using multivariate Cox regression analysis (for age: HR = 1.017; p = 0.006; for UICC stage: HR = 1.453; p < 0.001; for risk score: HR = 1.395; p < 0.001) (Figure 5B). Similar results were obtained in GSE31210 when testing was done using univariate Cox regression analysis (for UICC stage: HR = 4.106; p < 0.001; for risk score: HR = 1.011; p = 0.001) (Figure 5C) and multivariate Cox regression analysis (for UICC stage: HR = 3.301; p < 0.001; for risk score: HR = 1.009; p = 0.014) (Figure 5D). Thus, risk score can independently predict the prognosis of NSCLC.
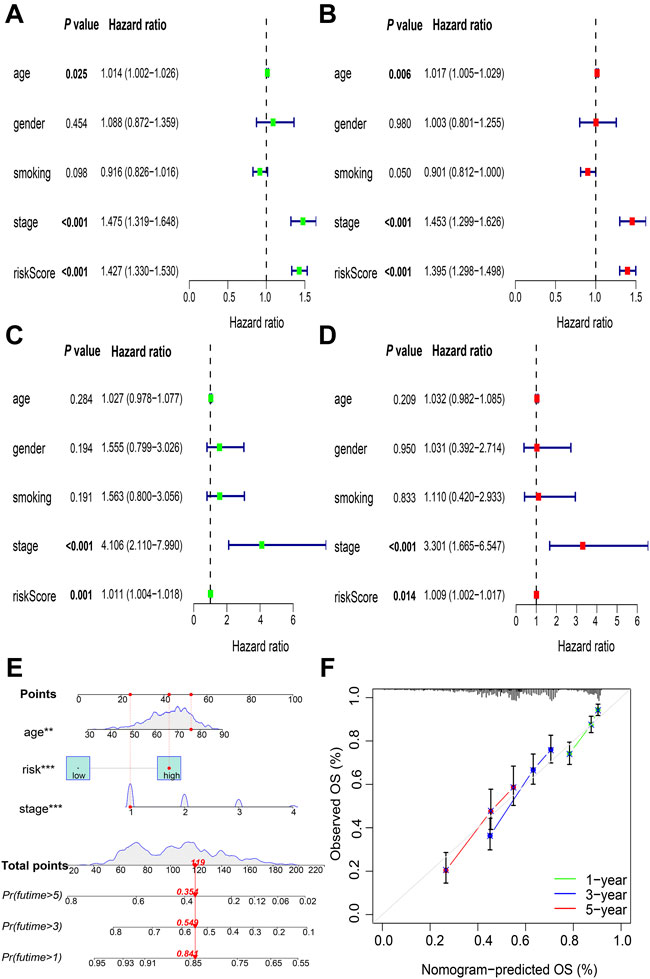
FIGURE 5. Identification of independent prognostic factors and construction of nomogram. Validation of the risk score as an independent prognostic factor using the univariate Cox analysis in (A) TCGA cohorts and (C) GSE31210 cohorts, and multivariate Cox analysis in (B) TCGA cohorts and (D) GSE31210 cohorts (E) Nomogram to predict OS at the end of 1-, 3-, and 5-year periods (F) Calibration plots of the nomogram. TCGA, The Cancer Genome Atlas; OS, overall survival. **p < 0.01; ***p < 0.001.
Nomogram prediction model
A nomogram prediction model was generated using independent prognostic factors, including age, UICC stage, and risk score in patients with NSCLC, and the estimated survival probabilities after 1-, 3-, and 5-years were calculated by plotting a vertical line between the total point axis and each prognostic axis (Figure 5E). Calibration plots of the nomogram showed high agreement between the nomogram-predicted and the actual OS at the end of 1-, 3-, and 5-year periods (Figure 5F).
Results of GSEA
The results of GSEA showed that DNA replication, cell cycle, and ECM receptor interaction were the main pathways enriched in the high-risk group (Figure 6A); drug metabolism, cytochrome P450, ether lipid metabolism, and intestinal immune network for IgA production were the main pathways enriched in the low-risk group (Figure 6B).
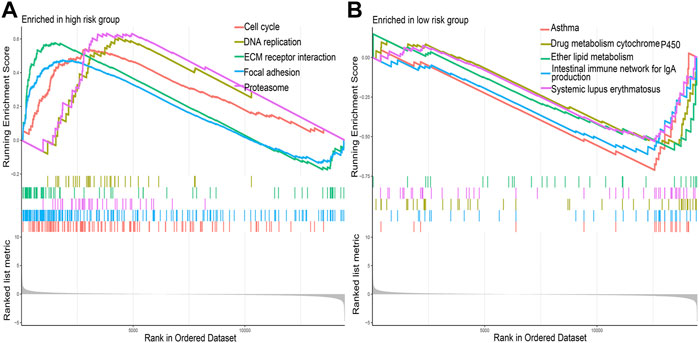
FIGURE 6. The primary Kyoto Encyclopedia of Genes and Genomes pathways enriched in the (A) high-risk group and (B) low-risk group.
Results of DNA methylation and mutation
A total of 753 overlapping patients with NSCLC were identified between the patients with DNA methylation data and the patients with complete follow-up data, among whom 386 patients were in the low-risk group and 367 patients were in the high-risk group. Methylation data of SCN1A and KLK6 are missing and zero, respectively, and thus are excluded. The overall trend of methylation levels of 10 prognostic genes in the high-risk group was lower than that in the low-risk group (Figures 7A–J). The mutation analysis revealed that TP53, MUC16, TTN, and RYR2 were the most frequently mutated genes in both two risk groups of both LUAD (Figures 8A and B) and LUSC (Figures 8C and D). The high-risk group had a higher TMB compared with the low-risk group in NSCLC (p = 0.002, Figure 8E) and a positive correlation existed between risk score and TMB (r = 0.13, p < 0.001, Figure 8F). Kaplan–Meier survival curve showed that the patients with the high-risk score and high TMB had a worse OS than those with the low-risk score and low TMB (p < 0.001; Figure 8G), and the AUC values for OS at 1-, 3-, 5-year periods were 0.739, 0.654, and 0.673, respectively (Figure 8H).
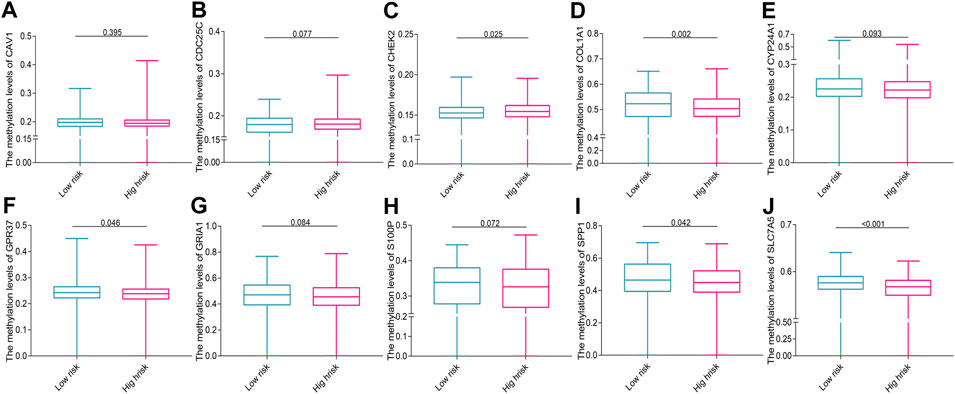
FIGURE 7. The methylation levels of prognostic genes in the two risk groups (A) CAV1, (B) CDC25C, (C) CHEK2, (D) COL1A1, (E) CYP24A1, (F) GPR37, (G) GRIA1, (H) S100P, (I) SPP1, and (J) SLC7A5.
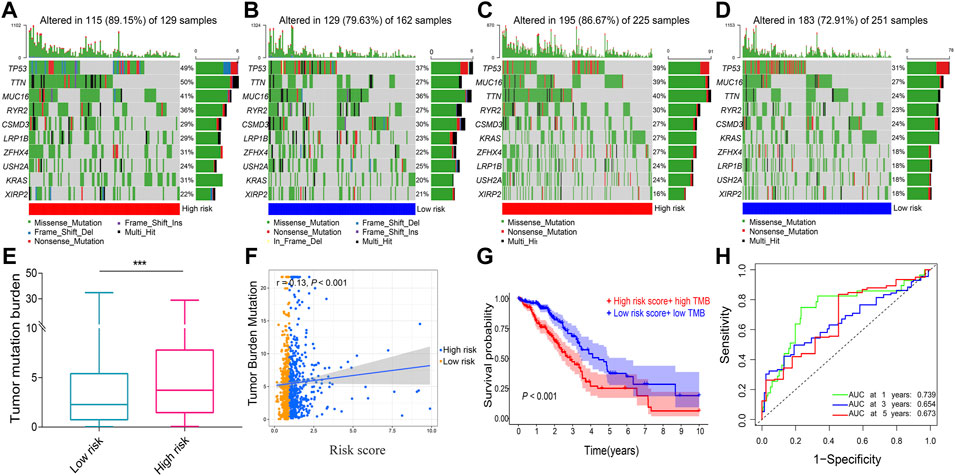
FIGURE 8. The tumor mutation analysis. Comparison of gene mutation frequencies in the high-risk group of (A) LUAD and (C) LUSC. Comparison of mutation frequencies in the low-risk group (B) LUAD and (D) LUSC (E) Boxplot illustrated that the TMB value was significantly higher in the high-risk group in NSCLC (F) Scatter plot of correlations between the TMB value and the risk score in NSCLC (G) Kaplan–Meier survival curve between the high-risk score + high TMB group and the low-risk score + low TMB group (H) ROC curve analysis. LUAD, lung adenocarcinoma. LUSC, lung squamous cell carcinoma; NSCLC, Non-small cell lung cancer; TMB, tumor mutation burden; ROC, receiver operating characteristic. AUC, an area under the ROC curve. ***p < 0.001.
Results of immune infiltration
The immune-infiltration profiles between the two risk groups were significantly different and displayed in the violin plot (Figure 9A). The correlation analysis showed that risk score was positively correlated with resting NK cells, activated memory CD4+ T cells, M0 macrophages, and activated mast cells, and negatively correlated with resting memory CD4+ T cells, naive and memory B cells, resting dendritic cells, resting mast cells, and monocytes (p < 0.05; Figures 9B–K).
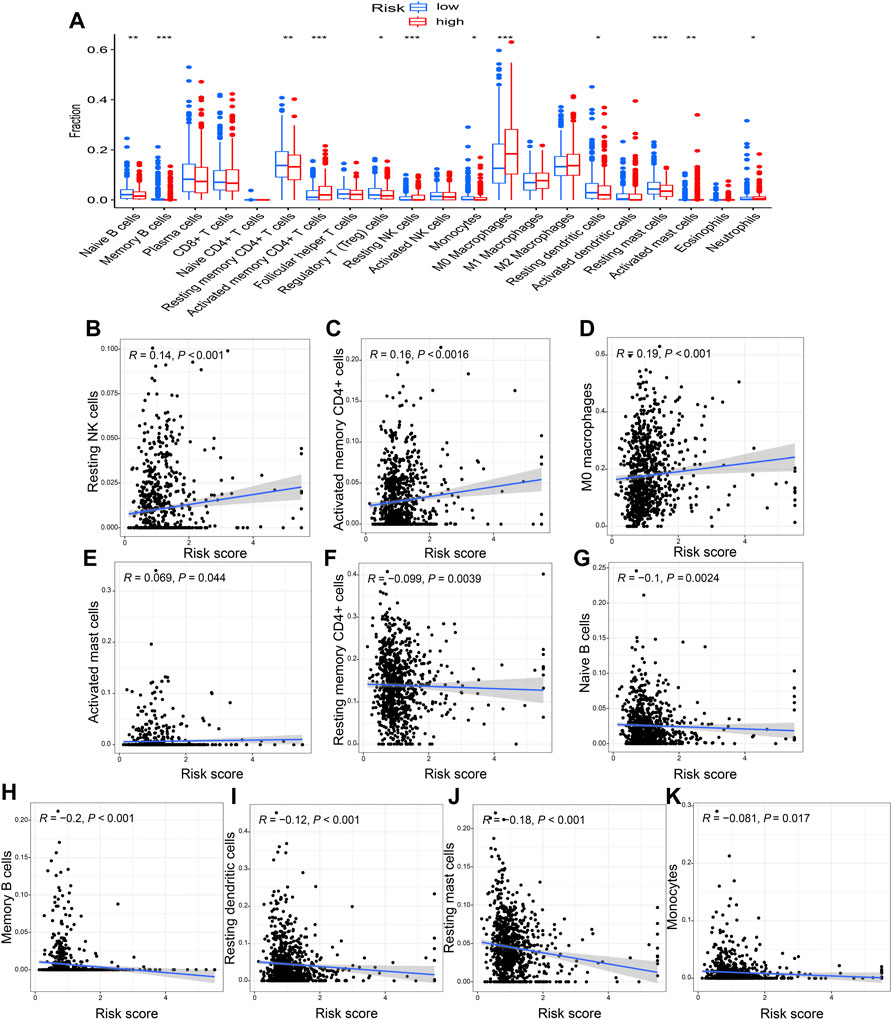
FIGURE 9. The correlation of risk score with immune infiltration (A) The different immune-infiltration profiles between the two risk groups. A positive correlation with the risk score was seen for (B) resting NK cells, (C) activated memory CD4+ T cells, (D) M0 macrophages, and (E) activated mast cells. Whereas (F) resting memory CD4+ T cells, (G) naïve B cells, (H) memory B cells, (I) resting dendritic cells, (J) resting mast cells, and (K) monocytes were negatively correlated with the risk score. *p < 0.05; **p < 0.01; ***p < 0.001.
The risk score correlated with chemotherapy sensitivity
The results of chemotherapy sensitivity analysis indicated that the high-risk group exhibited lower IC50 values for bortezomib, dasatinib, docetaxel, midostaurin, paclitaxel, parthenolide, pazopanib, shikonin, and thapsigargin compared with the low-risk group (p < 0.001, Figures 10A–I).
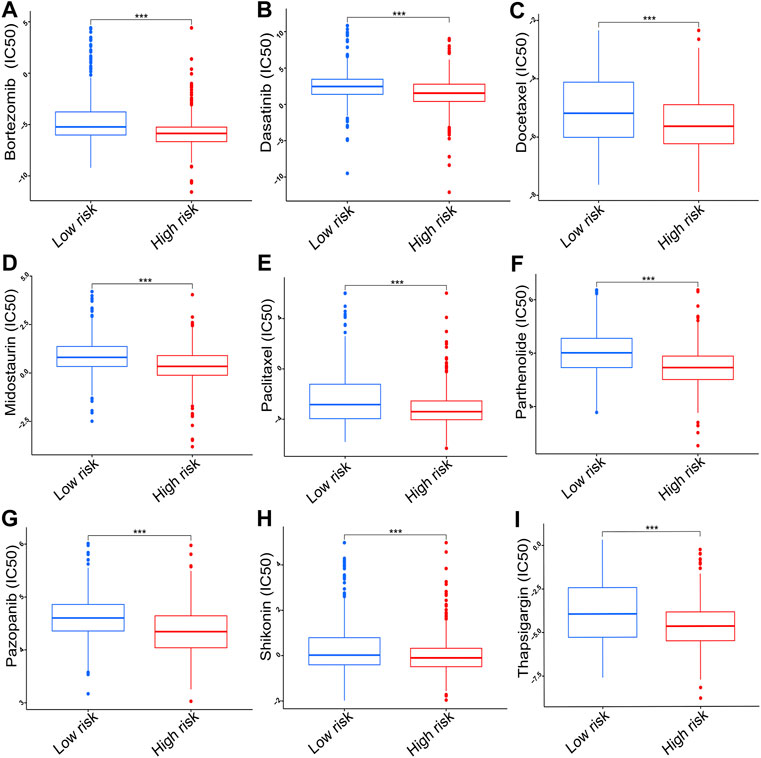
FIGURE 10. The IC50 of chemotherapy drugs including (A) bortezomib, (B) dasatinib, (C) docetaxel, (D) midostaurin, (E) paclitaxel, (F) parthenolide, (G) pazopanib, (H) shikonin, and (I) thapsigargin. IC50, half inhibitory concentration. ***p < 0.001.
The Results of real-time PCR
The levels of CYP24A1 (p < 0.001), CDC25C (p < 0.001), CHEK2 (p < 0.001), KLK6 (p = 0.044), S100P (p < 0.001), COL1A1 (p < 0.001), GPR37 (p < 0.001), and SLC7A5 (p < 0.001) mRNA expression were higher in cancerous tissues of patients with NSCLC than in corresponding controls (Figures 11A–H); whereas the levels of SCN1A (p < 0.001), CAV1 (p < 0.001), and GRIA1 (p < 0.001) mRNA expression in cancerous tissues of patients with NSCLC were lower than those in control tissues (Figures 11I–K). The increased SPP1 mRNA levels in NSCLC were validated in previous research (Miao et al., 2021) (Figure 11L).
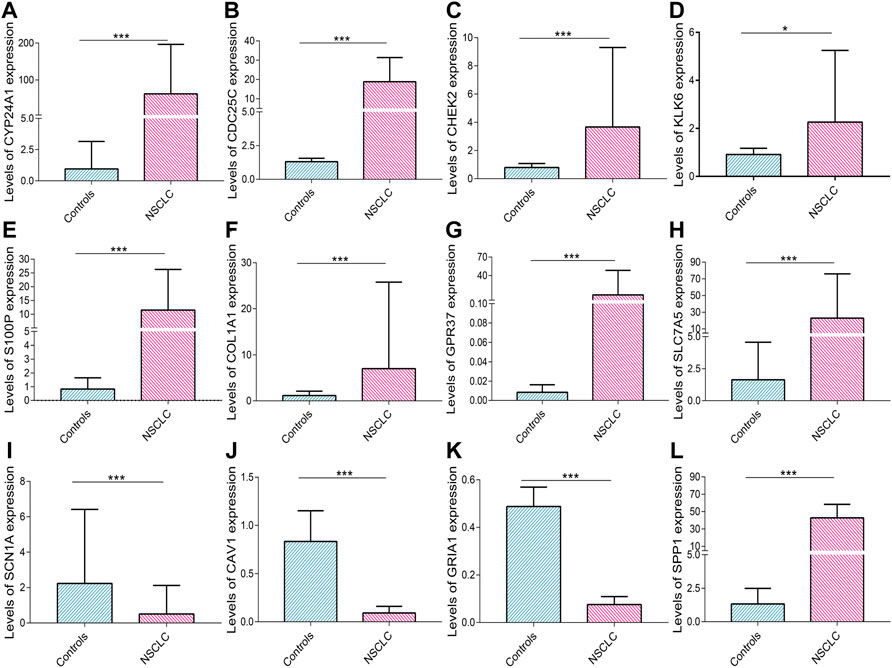
FIGURE 11. Real-time PCR validation. The levels of (A) CYP24A1, (B) CDC25C, (C) CHEK2, (D) KLK6, (E) S100P, (F) COL1A1, (G) GPR37, (H) SLC7A5, (I) SCN1A, (J) CAV1, (K) GRIA1, and (L) SPP1 mRNA expression in NSCLC. Data are presented as median (interquartile range). NSCLC, non-small cell lung cancer, PCR, polymerase chain reaction. *p < 0.05; **p < 0.01; ***p < 0.001.
Discussion
In the present study, a prognostic signature was successfully established using genes involved in redox homeostasis. Kaplan–Meier survival curve and ROC curve analysis validated the predictive accuracy of the signature. Furthermore, the constructed nomogram accurately predicted the OS of patients, and there was high conformity between the predicted and actual OS. The risk score is an independent prognostic factor for NSCLC and is correlated with tumor progression, immune infiltration, DNA methylation, TMB, and chemotherapy sensitivity.
The mechanism(s) of action of genes associated with redox homeostasis in the progression and prognosis of NSCLC remains unclear. CDC25C mRNA expression is increased in LUAD and associated with poor prognosis (Xia et al., 2019). KLK6 mRNA expression is enhanced in patients with lung cancer (Ju et al., 2020) and is an indicator of tumor proliferation and poor OS (Nathalie et al., 2009; Michel et al., 2014). The overexpression of the SPP1 was associated with the growth and stage of lung cancer, and lymph node metastasis (Shijubo et al., 1999; Schneider et al., 2004; Boldrini et al., 2005; Donati et al., 2005; Hu et al., 2005). CAV1 is downregulated in lung cancer and thus acts as a tumor suppressor gene (Bélanger et al., 2004; Zhan et al., 2012). CYP24A1 overexpression is associated with accelerated cell growth and invasion (Shiratsuchi et al., 2017) and worse survival in LUAD (Chen et al., 2011), and its polymorphisms are associated with susceptibility to lung cancer (Qu et al., 2019; Xiong et al., 2020). The levels of S100P mRNA and protein expression are higher in lung cancer, and upregulated S100P increases cancer cell migration and metastasis (Hsu et al., 2015). The levels of SLC7A5 mRNA and protein expression are increased in NSCLC and are correlated with grade, pathologic stage, and OS (Takeuchi et al., 2010; Liu et al., 2021). The levels of COL1A1 mRNA expression in lung cancer are increased and are significantly correlated with tumor diameter, metastasis, and OS (Geng et al., 2021; Hou et al., 2021). Our real-time PCR validated dysregulated CDC25C, KLK6, SPP1, CAV1, CYP24A1, S100P, SLC7A5, and COL1A1 expression in NSCLC. Additionally, GPR37 expression is lower in cancer and is a prognostic indicator (Liu et al., 2014). Elevated GRIA1 mRNA levels are significantly associated with worse OS in patients with basal-like urothelial carcinomas (Tilley et al., 2017). CHEK2 is identified as a susceptibility gene for pheochromocytomas and paragangliomas (Gao et al., 2021), and CHEK2 mutations are associated with the survival of patients with stage T1 bladder cancer (Złowocka-Perłowska et al., 2021). SCN1A has not been reported to be involved in cancer origin and progression. The upregulated levels of GPR37 and CHEK2 expressions and reduced levels of GRIA1 and SCN1A expressions in NSCLC were firstly reported in this study. However, the exact molecular mechanisms remain unknown, and further investigations are needed to elucidate the possible mechanisms.
Previous studies have reported the successful construction of the redox-related prognostic signature in clear cell renal cell carcinoma (Wu et al., 2021b), and hepatocellular carcinoma (Tu et al., 2021). However, both of them are only based on bioinformatics analysis and thus, lack reliable experimental verification. Additionally, a redox-related gene signature has been established in LUAD (Xiao et al., 2022b), which is only suitable for a subset of patients with NSCLC and also lacks reliable experimental verification. In the present study, the redox-related gene signature was first constructed in all the patients with NSCLC, and Kaplan–Meier survival curve showed that the high-risk group had a worse OS than the low-risk group, which was different from and cannot be replaced by the previously constructed signature using hypoxia-related gene (Chen et al., 2022), pyroptosis-related gene (Zhang and Yan, 2021; Li et al., 2022), autophagy-related gene (Wu et al., 2021a), and inflammatory response-related gene (Zou et al., 2021) because it could be meaningful in exploring the value of the redox-related gene in initiation, progression, prognosis, and therapy of NSCLC. The previous prognostic signatures in other cancers showed better diagnostic performance, with an AUC value of more than 0.8 (Qian et al., 2022; Zhang et al., 2022). However, in the current study, the AUC value for OS at the 5 year periods was 0.657 in TCGA and 0.717 in GSE31210. Various researches showed that the AUC values of many signatures for lung cancer or NSCLC were more than 0.75 (Wu et al., 2021a; Li et al., 2021b; Ouyang et al., 2021; Qu et al., 2021; Zhang and Yan, 2021; Zou et al., 2021; Wang et al., 2022b; Chen et al., 2022; Li et al., 2022; Yang et al., 2022). Thus, the prognostic biomarkers or signatures with a higher AUC value need to be explored in the future. Moreover, the 12 genes in prognostic signature were validated using real-time PCR in NSCLC; thus its reliability is higher than the above-mentioned redox-related gene signature for clear cell renal cell carcinoma (Wu et al., 2021b), hepatocellular carcinoma (Tu et al., 2021), LUAD (Xiao et al., 2022b), and hypoxia-related gene signature (Chen et al., 2022), pyroptosis-related gene signature (Zhang and Yan, 2021; Li et al., 2022), autophagy-related gene signature (Wu et al., 2021a), and inflammatory response-related signature (Zou et al., 2021) for NSCLC. Moreover, the risk score positively correlated with the progression of NSCLC and was an independent prognostic factor for NSCLC adjusted for age and UICC stage. Importantly, the signature was successfully validated using multiple GEO datasets. Therefore, our prognostic signature can successfully predict prognosis and is not restricted by different groups of patients, sequence platforms, and technologies. These were consistent with the successfully established signatures for NSCLC (Ye et al., 2021; Yang et al., 2022). Finally, a nomogram prediction model was constructed using the risk score, age, and UICC stage to precisely predict OS rate after 1-, 3-, and 5-year periods, and calibration plots of the nomogram showed high agreement between the nomogram-predicted and the actual OS.
The mechanisms of different prognoses of two risk groups were explored using GSEA, and findings showed that DNA replication and cell cycle were the main pathways in the high-risk group. The cell cycle has a vital role in lung cancer pathogenesis and progression (Caputi et al., 2005), and DNA damage-response or DNA-repair genes with germline aberrations induce cancerous tendencies (Brown et al., 2017). Thus, the worse OS in the high-risk group may be correlated with DNA replication and cell cycle. Additionally, DNA methylation alteration could result in cancer development and progression (Kinnaird et al., 2016). Hypomethylation of the promoter is correlated with the overexpression of oncogenes and other genes associated with tumor invasion or metastasis (Ehrlich, 2009). Our results showed that methylation levels of 10 prognostic genes were lower in the high-risk group. Moreover, dysregulated TMB has also been reported to be involved in the prognosis of cancers (Handy and Loscalzo, 2012), and could predict immunotherapy response (Chalmers et al., 2017). The high TMB is correlated with poor prognosis in LUAD (Zhao et al., 2021b). The high-risk group has an increased TMB compared with the low-risk group in the present study, and Kaplan–Meier survival curve showed that the patients with the high-risk score and high TMB had a worse OS than those with the low-risk score and low TMB. Thus, DNA methylation and mutation play a critical role in the progression and prognosis of NSCLC.
In recent years, immunotherapy has become an important therapeutic method for multiple tumor types (Alemohammad et al., 2021; Cai et al., 2021); thus, a possible correlation between the risk score and immune infiltration was assessed. The results showed that a high-risk score was positively correlated with macrophages, activated memory CD4+ T cells, activated mast cells, and resting NK cells. Tumor-associated macrophages (TAMs) are significantly correlated with angiogenesis, progression (Hwang et al., 2020), and a poor prognosis (Montuenga and Pio, 2007; Li et al., 2020b) in NSCLC. A high percentage of CD4+ T cells in the tumor stroma is associated with a poor prognosis in lung cancer (Giatromanolaki et al., 2021). The mast cells were activated in lung cancer by cancer-derived extracellular vesicles (Salamon et al., 2020), and activated mast cells accelerated cancer cell proliferation and migration (Xiao et al., 2014; Xiao et al., 2019). Thus, our results indicated that the high-risk group has a worse OS via increased immune infiltration.
Considering that chemotherapy is still an important therapeutic method for advanced lung cancer, a possible association between the risk score and chemotherapy was also studied. The results showed that the high-risk group had a lower IC50 value for nine chemotherapy drugs, suggesting that the high-risk group may respond better to chemotherapy. Thus, our prognostic signature can be useful for guiding treatment strategies for patients diagnosed with NSCLC.
However, it is worth noting that the results obtained in this study were based on bioinformatic analyses of the public database, which needs to be validated in a clinical prospective, multicenter study. Furthermore, studies are required to validate molecular mechanisms between two risk groups in NSCLC. Moreover, the previous studies showed that the prognostic signature could be used to guide immunotherapy choices (Cao et al., 2022). However, there was not a significant correlation between the risk scores and levels of immune checkpoint inhibitors expression. Despite these limitations, our study has established a promising signature for predicting patient prognosis. The signature was validated using GSE31210, GSE68465, and GSE26939 datasets. Secondly, the 12 prognostic genes were validated using real-time PCR in lung tissue samples. Lastly, the risk score was shown to be correlated with immune infiltration and chemotherapy response; thus, it could be applied to guide treatment modules in the clinical setting.
Conclusion
A redox-related gene signature was established using LASSO regression analysis and was represented as a risk score. The high-risk score in this signature was significantly correlated with worse OS and the risk score was an independent prognostic factor for NSCLC. Moreover, the risk score was correlated with tumor progression, DNA methylation, TMB, immune infiltration, and sensitivity to chemotherapy.
Data availability statement
The original contributions presented in the study are included in the article/Supplementary Material; further inquiries can be directed to the corresponding author.
Ethics statement
The studies involving human participants were reviewed and approved by the Ethics Committee of Zigong First People’s Hospital (No.2021–24). The patients/participants provided their written informed consent to participate in this study.
Author contributions
T-wM, D-qY, and L-jG collected and analyzed data and performed real-time PCR. QZ and JL collected data. Y-qH analyzed the data and edited the language. JY revised the manuscript and edited the language. XC designed the experiment and revised the manuscript.
Funding
This research was supported by Sichuan Provincial Administration of Traditional Chinese Medicine (2021MS462).
Conflict of interest
The authors declare that the research was conducted in the absence of any commercial or financial relationships that could be construed as a potential conflict of interest.
Publisher’s note
All claims expressed in this article are solely those of the authors and do not necessarily represent those of their affiliated organizations, or those of the publisher, the editors, and the reviewers. Any product that may be evaluated in this article, or claim that may be made by its manufacturer, is not guaranteed or endorsed by the publisher.
Supplementary Material
The Supplementary Material for this article can be found online at: https://www.frontiersin.org/articles/10.3389/fmolb.2022.942402/full#supplementary-material
References
Aggarwal, V., Tuli, H. S., Varol, A., Thakral, F., Yerer, M. B., Sak, K., et al. (2019). Role of reactive oxygen species in cancer progression: Molecular mechanisms and recent advancements. Biomolecules 9, E735. doi:10.3390/biom9110735
Alemohammad, H., Najafzadeh, B., Asadzadeh, Z., Baghbanzadeh, A., Ghorbaninezhad, F., Najafzadeh, A., et al. (2021). The importance of immune checkpoints in immune monitoring: A future paradigm shift in the treatment of cancer. Biomed. Pharmacother. = Biomedecine Pharmacother. 146, 112516. doi:10.1016/j.biopha.2021.112516
Bélanger, M. M., Roussel, E., and Couet, J. (2004). Caveolin-1 is down-regulated in human lung carcinoma and acts as a candidate tumor suppressor gene. Chest 125, 106s. doi:10.1378/chest.125.5_suppl.106s
Boldrini, L., Donati, V., Dell'Omodarme, M., Prati, M. C., Faviana, P., Camacci, T., et al. (2005). Prognostic significance of osteopontin expression in early-stage non-small-cell lung cancer. Br. J. Cancer 93, 453–457. doi:10.1038/sj.bjc.6602715
Brown, J. S., O'Carrigan, B., Jackson, S. P., and Yap, T. A. (2017). Targeting DNA repair in cancer: Beyond PARP inhibitors. Cancer Discov. 7, 20–37. doi:10.1158/2159-8290.cd-16-0860
Cai, X., Zhan, H., Ye, Y., Yang, J., Zhang, M., Li, J., et al. (2021). Current progress and future perspectives of immune checkpoint in cancer and infectious diseases. Front. Genet. 12, 785153. doi:10.3389/fgene.2021.785153
Cao, K., Liu, M., Ma, K., Jiang, X., Ma, J., and Zhu, J. (2022). Prediction of prognosis and immunotherapy response with a robust immune-related lncRNA pair signature in lung adenocarcinoma. Cancer Immunol. Immunother. 71, 1295–1311. doi:10.1007/s00262-021-03069-1
Caputi, M., Russo, G., Esposito, V., Mancini, A., and Giordano, A. (2005). Role of cell-cycle regulators in lung cancer. J. Cell. Physiol. 205, 319–327. doi:10.1002/jcp.20424
Chalmers, Z. R., Connelly, C. F., Fabrizio, D., Gay, L., Ali, S. M., Ennis, R., et al. (2017). Analysis of 100, 000 human cancer genomes reveals the landscape of tumor mutational burden. Genome Med. 9, 34. doi:10.1186/s13073-017-0424-2
Chen, G., Kim, S. H., King, A. N., Zhao, L., Simpson, R. U., Christensen, P. J., et al. (2011). CYP24A1 is an independent prognostic marker of survival in patients with lung adenocarcinoma. Clin. Cancer Res. 17, 817–826. doi:10.1158/1078-0432.ccr-10-1789
Chen, J., Fu, Y., Hu, J., and He, J. (2022). Hypoxia-related gene signature for predicting LUAD patients' prognosis and immune microenvironment. Cytokine 152, 155820. doi:10.1016/j.cyto.2022.155820
Cook, K. B., Kazan, H., Zuberi, K., Morris, Q., and Hughes, T. R. (2011). Rbpdb: a database of RNA-binding specificities. Nucleic Acids Res. 39, D301–D308. doi:10.1093/nar/gkq1069
Ctortecka, C., Palve, V., Kuenzi, B. M., Fang, B., Sumi, N. J., Izumi, V., et al. (2018). Functional proteomics and deep network interrogation reveal a complex mechanism of action of midostaurin in lung cancer cells. Mol. Cell. Proteomics 17, 2434–2447. doi:10.1074/mcp.RA118.000713
Di Vincenzo, S., Sangiorgi, C., Ferraro, M., Buscetta, M., Cipollina, C., and Pace, E. (2021). Cigarette smoke extract reduces FOXO3a promoting tumor progression and cell migration in lung cancer. Toxicology 454, 152751. doi:10.1016/j.tox.2021.152751
Donati, V., Boldrini, L., Dell'Omodarme, M., Prati, M. C., Faviana, P., Camacci, T., et al. (2005). Osteopontin expression and prognostic significance in non-small cell lung cancer. Clin. Cancer Res. 11, 6459–6465. doi:10.1158/1078-0432.ccr-05-0541
Duan, L., Perez, R. E., Calhoun, S., and Maki, C. G. (2022). Inhibitors of Jumonji C domain-containing histone lysine demethylases overcome cisplatin and paclitaxel resistance in non-small cell lung cancer through APC/Cdh1-dependent degradation of CtIP and PAF15. Cancer Biol. Ther. 23, 65–75. doi:10.1080/15384047.2021.2020060
Ehrlich, M. (2009). DNA hypomethylation in cancer cells. Epigenomics 1, 239–259. doi:10.2217/epi.09.33
Facchinetti, F., Marabelle, A., Rossi, G., Soria, J. C., Besse, B., and Tiseo, M. (2016). Moving immune checkpoint blockade in thoracic tumors beyond NSCLC. J. Thorac. Oncol. 11, 1819–1836. doi:10.1016/j.jtho.2016.05.027
Feng, Z., Zhang, Y., He, M., Han, Y., Cai, C., Liu, S., et al. (2022). AMICA1 is a diagnostic and prognostic biomarker and induces immune cells infiltration by activating cGAS-STING signaling in lung adenocarcinoma. Cancer Cell Int. 22, 111. doi:10.1186/s12935-022-02517-x
Forman, H. J., and Zhang, H. (2021). Targeting oxidative stress in disease: Promise and limitations of antioxidant therapy. Nat. Rev. Drug Discov. 20, 689–709. doi:10.1038/s41573-021-00233-1
Gao, P., Zhang, H., Dinavahi, R., Li, F., Xiang, Y., Raman, V., et al. (2007). HIF-dependent antitumorigenic effect of antioxidants in vivo. Cancer Cell 12, 230–238. doi:10.1016/j.ccr.2007.08.004
Gao, Y., Ling, C., Ma, X., Wang, H., Cui, Y., Nie, M., et al. (2021). Recurrent germline mutations of CHEK2 as a new susceptibility gene in patients with pheochromocytomas and paragangliomas. Int. J. Endocrinol. 2021, 1392386. doi:10.1155/2021/1392386
Geng, Q., Shen, Z., Li, L., and Zhao, J. (2021). COL1A1 is a prognostic biomarker and correlated with immune infiltrates in lung cancer. PeerJ 9, e11145. doi:10.7717/peerj.11145
Gerstberger, S., Hafner, M., and Tuschl, T. (2014). A census of human RNA-binding proteins. Nat. Rev. Genet. 15, 829–845. doi:10.1038/nrg3813
Giatromanolaki, A., Anestopoulos, I., Panayiotidis, M. I., Mitrakas, A., Pappa, A., and Koukourakis, M. I. (2021). Prognostic relevance of the relative presence of CD4, CD8 and CD20 expressing tumor infiltrating lymphocytes in operable non-small cell lung cancer patients. Anticancer Res. 41, 3989–3995. doi:10.21873/anticanres.15196
Hedyed, E., Aryan, Z., Saeedi Moghaddam, S., Bisignano, C., Rezaei, S., Pishgar, F., et al. (2021). Global, regional, and national burden of respiratory tract cancers and associated risk factors from 1990 to 2019: a systematic analysis for the global burden of disease study 2019. Lancet Respir. Med. 9, 1030–1049. doi:10.1016/s2213-2600(21)00164-8
Goldstraw, P., Chansky, K., Crowley, J., Rami-Porta, R., Asamura, H., Eberhardt, W. E. E., et al. (2016). The IASLC lung cancer staging project: Proposals for revision of the TNM stage groupings in the forthcoming (eighth) edition of the TNM classification for lung cancer. J. Thorac. Oncol. 11, 39–51. doi:10.1016/j.jtho.2015.09.009
Halliwell, B., and Chirico, S. (1993). Lipid peroxidation: Its mechanism, measurement, and significance. Am. J. Clin. Nutr. 57, 715S–724S. discussion 724S-725S; discussion 24S-25S. doi:10.1093/ajcn/57.5.715S
Han, M., Zhang, T., Yang, L., Wang, Z., Ruan, J., and Chang, X. (2016). Association between NADPH oxidase (NOX) and lung cancer: a systematic review and meta-analysis. J. Thorac. Dis. 8, 1704–1711. doi:10.21037/jtd.2016.06.31
Handy, D. E., and Loscalzo, J. (2012). Redox regulation of mitochondrial function. Antioxid. Redox Signal. 16, 1323–1367. doi:10.1089/ars.2011.4123
Hou, L., Lin, T., Wang, Y., Liu, B., and Wang, M. (2021). Collagen type 1 alpha 1 chain is a novel predictive biomarker of poor progression-free survival and chemoresistance in metastatic lung cancer. J. Cancer 12, 5723–5731. doi:10.7150/jca.59723
Hsu, Y. L., Hung, J. Y., Liang, Y. Y., Lin, Y. S., Tsai, M. J., Chou, S. H., et al. (2015). S100P interacts with integrin α7 and increases cancer cell migration and invasion in lung cancer. Oncotarget 6, 29585–29598. doi:10.18632/oncotarget.4987
Hu, Z., Lin, D., Yuan, J., Xiao, T., Zhang, H., Sun, W., et al. (2005). Overexpression of osteopontin is associated with more aggressive phenotypes in human non-small cell lung cancer. Clin. Cancer Res. 11, 4646–4652. doi:10.1158/1078-0432.ccr-04-2013
Hwang, I., Kim, J. W., Ylaya, K., Chung, E. J., Kitano, H., Perry, C., et al. (2020). Tumor-associated macrophage, angiogenesis and lymphangiogenesis markers predict prognosis of non-small cell lung cancer patients. J. Transl. Med. 18, 443. doi:10.1186/s12967-020-02618-z
Jaskulska, A., Janecka, A. E., and Gach-Janczak, K. (2020). Thapsigargin-from traditional medicine to anticancer drug. Int. J. Mol. Sci. 22, E4. doi:10.3390/ijms22010004
Ju, Q., Zhao, Y. J., Ma, S., Li, X. M., Zhang, H., Zhang, S. Q., et al. (2020). Genome-wide analysis of prognostic-related lncRNAs, miRNAs and mRNAs forming a competing endogenous RNA network in lung squamous cell carcinoma. J. Cancer Res. Clin. Oncol. 146, 1711–1723. doi:10.1007/s00432-020-03224-8
Kim, C., Liu, S. V., Crawford, J., Torres, T., Chen, V., Thompson, J., et al. (2021). A phase I trial of dasatinib and osimertinib in TKI naïve patients with advanced EGFR-mutant non-small-cell lung cancer. Front. Oncol. 11, 728155. doi:10.3389/fonc.2021.728155
Kinnaird, A., Zhao, S., Wellen, K. E., and Michelakis, E. D. (2016). Metabolic control of epigenetics in cancer. Nat. Rev. Cancer 16, 694–707. doi:10.1038/nrc.2016.82
Kwon, J., Lee, S. R., Yang, K. S., Ahn, Y., Kim, Y. J., Stadtman, E. R., et al. (2004). Reversible oxidation and inactivation of the tumor suppressor PTEN in cells stimulated with peptide growth factors. Proc. Natl. Acad. Sci. U. S. A. 101, 16419–16424. doi:10.1073/pnas.0407396101
LeDoux, S. P., Driggers, W. J., Hollensworth, B. S., and Wilson, G. L. (1999). Repair of alkylation and oxidative damage in mitochondrial DNA. Mutat. Res. 434, 149–159. doi:10.1016/s0921-8777(99)00026-9
Lee, S. R., Yang, K. S., Kwon, J., Lee, C., Jeong, W., and Rhee, S. G. (2002). Reversible inactivation of the tumor suppressor PTEN by H2O2. J. Biol. Chem. 277, 20336–20342. doi:10.1074/jbc.M111899200
Li, B., Huang, Y., Ming, H., Nice, E. C., Xuan, R., and Huang, C. (2021). Redox control of the dormant cancer cell life cycle. Cells 10, 2707. doi:10.3390/cells10102707
Li, M., Zhang, Y., Fan, M., Ren, H., Chen, M., and Shi, P. (2021). Identification of the ferroptosis-related long non-coding RNAs signature to improve the prognosis prediction and immunotherapy response in patients with NSCLC. BMC Med. Genomics 14, 286. doi:10.1186/s12920-021-01133-4
Li, T., Liu, H., Dong, C., and Lyu, J. (2022). Prognostic implications of pyroptosis-related gene signatures in lung squamous cell carcinoma. Front. Pharmacol. 13, 806995. doi:10.3389/fphar.2022.806995
Li, X., Huang, R., Li, M., Zhu, Z., Chen, Z., Cui, L., et al. (2020). Parthenolide inhibits the growth of non-small cell lung cancer by targeting epidermal growth factor receptor. Cancer Cell Int. 20, 561. doi:10.1186/s12935-020-01658-1
Li, Y., You, M. J., Yang, Y., Hu, D., and Tian, C. (2020). The role of tumor-associated macrophages in leukemia. Acta Haematol. 143, 112–117. doi:10.1159/000500315
Liu, F., Zhu, C., Huang, X., Cai, J., Wang, H., Wang, X., et al. (2014). A low level of GPR37 is associated with human hepatocellular carcinoma progression and poor patient survival. Pathol. Res. Pract. 210, 885–892. doi:10.1016/j.prp.2014.07.011
Liu, Y. H., Li, Y. L., Shen, H. T., Chien, P. J., Sheu, G. T., Wang, B. Y., et al. (2021). L-type Amino acid transporter 1 regulates cancer stemness and the expression of programmed cell death 1 ligand 1 in lung cancer cells. Int. J. Mol. Sci. 22, 10955. doi:10.3390/ijms222010955
Luengo, A., Abbott, K. L., Davidson, S. M., Hosios, A. M., Faubert, B., Chan, S. H., et al. (2019). Reactive metabolite production is a targetable liability of glycolytic metabolism in lung cancer. Nat. Commun. 10, 5604. doi:10.1038/s41467-019-13419-4
Matsumoto, K., Tamiya, A., Inagaki, Y., Taniguchi, Y., Matsuda, Y., Kawachi, H., et al. (2022). Efficacy and safety of ramucirumab plus docetaxel in older patients with advanced non-small cell lung cancer: a multicenter retrospective cohort study. J. Geriatr. Oncol. 13, 207–213. doi:10.1016/j.jgo.2021.09.004
Miao, T. W., Xiao, W., Du, L. Y., Mao, B., Huang, W., Chen, X. M., et al. (2021). High expression of SPP1 in patients with chronic obstructive pulmonary disease (COPD) is correlated with increased risk of lung cancer. FEBS open bio 11, 1237–1249. doi:10.1002/2211-5463.13127
Michel, N., Heuzé-Vourc'h, N., Lavergne, E., Parent, C., Jourdan, M. L., Vallet, A., et al. (2014). Growth and survival of lung cancer cells: Regulation by kallikrein-related peptidase 6 via activation of proteinase-activated receptor 2 and the epidermal growth factor receptor. Biol. Chem. 395, 1015–1025. doi:10.1515/hsz-2014-0124
Moloney, J. N., and Cotter, T. G. (2018). ROS signalling in the biology of cancer. Semin. Cell Dev. Biol. 80, 50–64. doi:10.1016/j.semcdb.2017.05.023
Montuenga, L. M., and Pio, R. (2007). Tumour-associated macrophages in nonsmall cell lung cancer: the role of interleukin-10. Eur. Respir. J. 30, 608–610. doi:10.1183/09031936.00091707
Nathalie, H. V., Chris, P., Serge, G., Catherine, C., Benjamin, B., Claire, B., et al. (2009). High kallikrein-related peptidase 6 in non-small cell lung cancer cells: an indicator of tumour proliferation and poor prognosis. J. Cell. Mol. Med. 13, 4014–4022. doi:10.1111/j.1582-4934.2009.00763.x
Ni, M., Liu, X., Wu, J., Zhang, D., Tian, J., Wang, T., et al. (2018). Identification of candidate biomarkers correlated with the pathogenesis and prognosis of non-small cell lung cancer via integrated bioinformatics analysis. Front. Genet. 9, 469. doi:10.3389/fgene.2018.00469
Niu, H., Chen, P., Fan, L., and Sun, B. (2022). Comprehensive pan-cancer analysis on CBX3 as a prognostic and immunological biomarker. BMC Med. Genomics 15, 29. doi:10.1186/s12920-022-01179-y
Ogrunc, M., Di Micco, R., Liontos, M., BombardeLLi, L., MioneM., , FuMagalliM., , et al. (2014). Oncogene-induced reactive oxygen species fuel hyperproliferation and DNA damage response activation. Cell Death Differ. 21, 998–1012. doi:10.1038/cdd.2014.16
Ouyang, W., Jiang, Y., Bu, S., Tang, T., Huang, L., Chen, M., et al. (2021). A prognostic risk score based on hypoxia-immunity-and epithelialto-mesenchymal transition-related genes for the prognosis and immunotherapy response of lung adenocarcinoma. Front. Cell Dev. Biol. 9, 758777. doi:10.3389/fcell.2021.758777
Pan, J., Li, M., Yu, F., Zhu, F., Wang, L., Ning, D., et al. (2021). Up-Regulation of p53/miR-628-3p pathway, a novel mechanism of shikonin on inhibiting proliferation and inducing apoptosis of A549 and PC-9 non-small cell lung cancer cell lines. Front. Pharmacol. 12, 766165. doi:10.3389/fphar.2021.766165
Qian, L., Ni, T., Fei, B., Sun, H., and Ni, H. (2022). An immune-related lncRNA pairs signature to identify the prognosis and predict the immune landscape of laryngeal squamous cell carcinoma. BMC cancer 22, 545. doi:10.1186/s12885-022-09524-1
Qu, J., Wang, L., Jiang, M., Wei, Z., Fu, G., and Zhang, X. (2021). Survival-associated N(6)-adenosine methyltransferase signatures in lung squamous cell carcinoma and clinical verification. BMC cancer 21, 1265. doi:10.1186/s12885-021-08939-6
Qu, R., Li, X., Quan, X., Xia, L., Fang, X., Li, H., et al. (2019). Polymorphism in CYP24A1 is associated with lung cancer risk: a case-control study in Chinese female nonsmokers. DNA Cell Biol. 38, 243–249. doi:10.1089/dna.2018.4510
Redin, E., Garmendia, I., Lozano, T., Serrano, D., Senent, Y., Redrado, M., et al. (2021). SRC family kinase (SFK) inhibitor dasatinib improves the antitumor activity of anti-PD-1 in NSCLC models by inhibiting Treg cell conversion and proliferation. J. Immunother. Cancer 9, e001496. doi:10.1136/jitc-2020-001496
Salamon, P., Mekori, Y. A., and Shefler, I. (2020). Lung cancer-derived extracellular vesicles: a possible mediator of mast cell activation in the tumor microenvironment. Cancer Immunol. Immunother. 69, 373–381. doi:10.1007/s00262-019-02459-w
Schneider, S., Yochim, J., Brabender, J., Uchida, K., Danenberg, K. D., Metzger, R., et al. (2004). Osteopontin but not osteonectin messenger RNA expression is a prognostic marker in curatively resected non-small cell lung cancer. Clin. Cancer Res. 10, 1588–1596. doi:10.1158/1078-0432.ccr-0565-3
Shijubo, N., Uede, T., Kon, S., MaedaM., , Segawa, T., ImAdA, A., et al. (1999). Vascular endothelial growth factor and osteopontin in stage I lung adenocarcinoma. Am. J. Respir. Crit. Care Med. 160, 1269–1273. doi:10.1164/ajrccm.160.4.9807094
Shiratsuchi, H., Wang, Z., Chen, G., Ray, P., Lin, J., Zhang, Z., et al. (2017). Oncogenic potential of CYP24A1 in lung adenocarcinoma. J. Thorac. Oncol. 12, 269–280. doi:10.1016/j.jtho.2016.10.010
Subramanian, A., Tamayo, P., Mootha, V. K., Mukherjee, S., Ebert, B. L., Gillette, M. A., et al. (2005). Gene set enrichment analysis: a knowledge-based approach for interpreting genome-wide expression profiles. Proc. Natl. Acad. Sci. U. S. A. 102, 15545–15550. doi:10.1073/pnas.0506580102
Sung, H., Ferlay, J., Siegel, R. L., Laversanne, M., Soerjomataram, I., Jemal, A., et al. (2021). Global cancer statistics 2020: GLOBOCAN estimates of incidence and mortality worldwide for 36 cancers in 185 countries. Ca. Cancer J. Clin. 71, 209–249. doi:10.3322/caac.21660
Takeuchi, K., Ogata, S., Nakanishi, K., Ozeki, Y., Hiroi, S., Tominaga, S., et al. (2010). LAT1 expression in non-small-cell lung carcinomas: analyses by semiquantitative reverse transcription-PCR (237 cases) and immunohistochemistry (295 cases). Lung cancer (Amsterdam, Neth. 68, 58–65. doi:10.1016/j.lungcan.2009.05.020
Tanimoto, A., Takeuchi, S., Kotani, H., Yamashita, K., Yamada, T., Ohtsubo, K., et al. (2018). Pulmonary carcinosarcoma showing an obvious response to pazopanib: a case report. BMC Pulm. Med. 18, 193. doi:10.1186/s12890-018-0757-7
The Cancer Genome Atlas Research Network (2014). Comprehensive molecular profiling of lung adenocarcinoma. Nature 511, 543–550. doi:10.1038/nature13385
Tilley, S. K., Kim, W. Y., and Fry, R. C. (2017). Analysis of bladder cancer tumor CpG methylation and gene expression within the Cancer Genome Atlas identifies GRIA1 as a prognostic biomarker for basal-like bladder cancer. Am. J. Cancer Res. 7, 1850–1862.
Travis, W. D., Brambilla, E., Burke, A. P., et al. (2015). Introduction to the 2015 world health organization classification of tumors of the lung, pleura, thymus, and heart. J. Thorac. Oncol. 10, 1240–1242. official publication of the International Association for the Study of Lung Cancer. doi:10.1097/jto.0000000000000663
Travis, W. D., Brambilla, E., Nicholson, A. G., Yatabe, Y., Austin, J. H. M., Beasley, M. B., et al. (2015). The 2015 world health organization classification of lung tumors: Impact of genetic, clinical and radiologic advances since the 2004 classification. J. Thorac. Oncol. 10, 1243–1260. doi:10.1097/jto.0000000000000630
Tu, K., Li, J., Mo, H., Xian, Y., Xu, Q., and Xiao, X. (2021). Identification and validation of redox-immune based prognostic signature for hepatocellular carcinoma. Int. J. Med. Sci. 18, 2030–2041. doi:10.7150/ijms.56289
Wang, W. H., Zhan, J. M., Tang, Y. L., Zhou, N., Liu, W. Y., and Jiang, D. W. (2022). miR-466 contributes to the enhanced antitumor effect of bortezomib on non-small-cell lung cancer by inhibiting CCND1. Chemotherapy 67, 110–122. doi:10.1159/000518936
Wang, Y., Zhao, X., Li, J., Wang, X., Hu, W., and Zhang, X. (2022). Four m6A RNA methylation gene signatures and their prognostic values in lung adenocarcinoma. Technol. Cancer Res. Treat. 21, 15330338221085373. doi:10.1177/15330338221085373
Weng, M. S., Chang, J. H., Hung, W. Y., Yang, Y. C., and Chien, M. H. (2018). The interplay of reactive oxygen species and the epidermal growth factor receptor in tumor progression and drug resistance. J. Exp. Clin. Cancer Res. 37, 61. doi:10.1186/s13046-018-0728-0
Wu, Y., Wei, X., Feng, H., Hu, B., Liu, B., Luan, Y., et al. (2021). Integrated analysis to identify a redox-related prognostic signature for clear cell renal cell carcinoma. Oxid. Med. Cell. Longev. 2021, 6648093. doi:10.1155/2021/6648093
Wu, Z. X., Huang, X., Cai, M. J., Huang, P. D., and Guan, Z. (2021). Development and validation of a prognostic index based on genes participating in autophagy in patients with lung adenocarcinoma. Front. Oncol. 11, 799759. doi:10.3389/fonc.2021.799759
Xia, Z., Ou-Yang, W., Hu, T., and Du, K. (2019). Prognostic significance of CDC25C in lung adenocarcinoma: an analysis of TCGA data. Cancer Genet. 233-234, 67–74. doi:10.1016/j.cancergen.2019.04.001
Xiao, D., Hu, X., and Zhang, J. (2022). Tumour targeted polymer nanoparticles co-loaded with docetaxel and siCCAT2 for combination therapy of lung cancer. J. Drug Target. 30, 534–543. doi:10.1080/1061186x.2021.2016773
Xiao, H., He, M., Xie, G., Liu, Y., Zhao, Y., Ye, X., et al. (2019). The release of tryptase from mast cells promote tumor cell metastasis via exosomes. BMC cancer 19, 1015. doi:10.1186/s12885-019-6203-2
Xiao, H., Lässer, C., Shelke, G. V., Wang, J., Radinger, M., Lunavat, T. R., et al. (2014). Mast cell exosomes promote lung adenocarcinoma cell proliferation - role of KIT-stem cell factor signaling. Cell Commun. Signal. 12, 64. doi:10.1186/s12964-014-0064-8
Xiao, L., Li, Q., Huang, Y., Fan, Z., Ma, L., Liu, B., et al. (2022). Construction of a redox-related prognostic model with predictive value in survival and therapeutic response for patients with lung adenocarcinoma. J. Healthc. Eng. 2022, 7651758. doi:10.1155/2022/7651758
Xiong, Q., Jiao, Y., Yang, P., Liao, Y., Gu, X., Hu, F., et al. (2020). The association study between CYP24A1 gene polymorphisms and risk of liver, lung and gastric cancer in a Chinese population. Pathol. Res. Pract. 216, 153237. doi:10.1016/j.prp.2020.153237
Yang, C., Wei, Y., Li, W., Wei, J., Chen, G., Xu, M., et al. (2022). Prognostic risk signature and comprehensive analyses of endoplasmic reticulum stress-related genes in lung adenocarcinoma. J. Immunol. Res. 2022, 6567916. doi:10.1155/2022/6567916
Ye, Q., Falatovich, B., Singh, S., Ivanov, A. V., Eubank, T. D., and Guo, N. L. (2021). A multi-omics network of a seven-gene prognostic signature for non-small cell lung cancer. Int. J. Mol. Sci. 23, 219. doi:10.3390/ijms23010219
Zalewska-Ziob, M., Adamek, B., Kasperczyk, J., Romuk, E., Hudziec, E., Chwalinska, E., et al. (2019). Activity of antioxidant enzymes in the tumor and adjacent noncancerous tissues of non-small-cell lung cancer. Oxid. Med. Cell. Longev. 2019, 2901840. doi:10.1155/2019/2901840
Zhan, P., Shen, X. K., Qian, Q., Wang, Q., Zhu, J. P., Zhang, Y., et al. (2012). Expression of caveolin-1 is correlated with disease stage and survival in lung adenocarcinomas. Oncol. Rep. 27, 1072–1078. doi:10.3892/or.2011.1605
Zhang, G., and Yan, Z. (2021). A new definition of pyroptosis-related gene markers to predict the prognosis of lung adenocarcinoma. Biomed. Res. Int. 2021, 8175003. doi:10.1155/2021/8175003
Zhang, H., Davies, K. J. A., and Forman, H. J. (2015). Oxidative stress response and Nrf2 signaling in aging. Free Radic. Biol. Med. 88, 314–336. doi:10.1016/j.freeradbiomed.2015.05.036
Zhang, J., Ling, X., Fang, C., and Ma, J. (2022). Identification and validation of an eight-lncRNA signature that predicts prognosis in patients with esophageal squamous cell carcinoma. Cell. Mol. Biol. Lett. 27, 39. doi:10.1186/s11658-022-00331-x
Zhao, N., Xin, H., and Zhang, L. (2021). Advanced biomedical applications of reactive oxygen species-based nanomaterials in lung cancer. Front. Chem. 9, 649772. doi:10.3389/fchem.2021.649772
Zhao, Z., He, B., Cai, Q., Zhang, P., Peng, X., Zhang, Y., et al. (2021). Combination of tumor mutation burden and immune infiltrates for the prognosis of lung adenocarcinoma. Int. Immunopharmacol. 98, 107807. doi:10.1016/j.intimp.2021.107807
Zhou, X., Wang, N., Zhang, Y., Yu, H., and Wu, Q. (2022). KAT2B is an immune infiltration-associated biomarker predicting prognosis and response to immunotherapy in non-small cell lung cancer. Invest. New Drugs 40, 43–57. doi:10.1007/s10637-021-01159-6
Złowocka-Perłowska, E., Dębniak, T., Słojewski, M., van de Wetering, T., Toloczko-Grabarek, A., Cybulski, C., et al. (2021). Survival of bladder or renal cancer in patients with CHEK2 mutations. PloS one 16, e0257132. doi:10.1371/journal.pone.0257132
Keywords: gene signature, NSCLC, cancer prognosis, immune infiltration, redox homeostasis
Citation: Miao T-w, Yang D-q, Gao L-j, Yin J, Zhu Q, Liu J, He Y-q and Chen X (2022) Construction of a redox-related gene signature for overall survival prediction and immune infiltration in non-small-cell lung cancer. Front. Mol. Biosci. 9:942402. doi: 10.3389/fmolb.2022.942402
Received: 12 May 2022; Accepted: 29 June 2022;
Published: 16 August 2022.
Edited by:
Guihong Sun, Wuhan University, ChinaReviewed by:
Nagarjun Vijay, Indian Institute of Science Education and Research, IndiaQin Xu, Shanghai Jiao Tong University, China
Copyright © 2022 Miao, Yang, Gao, Yin, Zhu, Liu, He and Chen. This is an open-access article distributed under the terms of the Creative Commons Attribution License (CC BY). The use, distribution or reproduction in other forums is permitted, provided the original author(s) and the copyright owner(s) are credited and that the original publication in this journal is cited, in accordance with accepted academic practice. No use, distribution or reproduction is permitted which does not comply with these terms.
*Correspondence: Xin Chen, NDY1MTc0MEBxcS5jb20=
†These authors have contributed equally to this work and share first authorship