- 1Research Center for Analytical Sciences, Frontiers Science Center for New Organic Matter, College of Chemistry, Tianjin Key Laboratory of Biosensing and Molecular Recognition, State Key Laboratory of Medicinal Chemical Biology, Nankai University, Tianjin, China
- 2Haihe Laboratory of Sustainable Chemical Transformations, Tianjin, China
The emergence of drug resistance may increase the death rates in advanced non-small cell lung cancer (NSCLC) patients. The resistance of erlotinib, the effective first-line antitumor drug for NSCLC with the L858R mutation of epidermal growth factor receptor (EGFR), happens after the T790M mutation of EGFR, because this mutation causes the binding of adenosine triphosphate (ATP) to EGFR more favorable than erlotinib. However, the mechanism of the enhancement of the binding affinity of ATP to EGFR, which is of paramount importance for the development of new inhibitors, is still unclear. In this work, to explore the detailed mechanism of the drug resistance due to the T790M mutation, molecular dynamics simulations and absolute binding free energy calculations have been performed. The results show that the binding affinity of ATP with respect to the L858R/T790M mutant is higher compared with the L858R mutant, in good agreement with experiments. Further analysis demonstrates that the T790M mutation significantly changes the van der Waals interaction of ATP and the binding site. We also find that the favorable binding of ATP to the L858R/T790M mutant, compared with the L858R mutant, is due to a conformational change of the αC-helix, the A-loop and the P-loop of the latter induced by the T790M mutation. This change makes the interaction of ATP and P-loop, αC-helix in the L858R/T790M mutant higher than that in the L858R mutant, therefore increasing the binding affinity of ATP to EGFR. We believe the drug-resistance mechanism proposed in this study will provide valuable guidance for the design of drugs for NSCLC.
Introduction
Lung cancer is the leading cause of cancer-related deaths worldwide (Jemal et al., 2011). The most common form of lung cancer is non-small cell lung cancer (NSCLC), which accounts for about 80–85% of lung cancer (Sharma et al., 2007; Inamura, 2017). In NSCLC, overexpression of epidermal growth factor receptor (EGFR) or hyper-activating mutations in its kinase domain have been observed in at least 50% of cases (Normanno et al., 2006). EGFR is a transmembrane receptor protein that has an essential role in cancer cell proliferation, survival, adhesion, migration, and differentiation by activating RAS/RAF/MEK/ERK and PI3K/AKT key downstream signaling pathways (Hirsch et al., 2003; Nagano et al., 2018; Zhou et al., 2022). In addition, among the currently marketed drugs, about 50–60% of drugs use membrane proteins to exert their effects (Santos et al., 2017). Therefore, EGFR and its mutations are one of the most valuable clinically validated drug targets for NSCLC treatment (Liao et al., 2010; Wee and Wang, 2017). A large number of small-molecule inhibitors acting on EGFR were developed to inhibit the kinase domain of EGFR and disrupt the oncogenic cell signaling by competing with adenosine triphosphate (ATP) for the binding site on the intracellular tyrosine kinase domain of EGFR. For example, first-generation EGFR inhibitor gefitinib or erlotinib is widely employed as first-line therapy for NSCLC with EGFR L858R mutation or exon 19 deletions. However, the secondary EGFR mutation T790M detected in NSCLC patients, can induce clinical resistance to gefitinib or erlotinib, greatly limiting the efficacy of these drugs in clinical use (Pao et al., 2005; Kosaka et al., 2006).
Understanding the mechanism of the T790M-induced drug resistance is important for further drug design. To this end, Kobayashi et al. proposed that the source of the acquired drug resistance was steric hindrance produced by the bulky methionine replaced the residue of threonine at position 790 (Kobayashi et al., 2005; Kwak et al., 2005; Pao et al., 2005). Interestingly, a later study demonstrated that the T790M resistance mutation increased the affinity of the receptor for ATP, which in turn diminished the potency of these ATP-competitive inhibitors (Yun et al., 2008). Several theoretical studies have been performed to explain the structural and energetic analyses of drug resistance conferred by the T790M mutation. Saldaña-Rivero and co-workers used the MM-GBSA approach to explain how L858R, T790M and L858R/T790M mutations impact the binding mechanism of ATP (Saldaña-Rivera et al., 2019). The popular MM/GBSA approach has been used to obtain a rough estimate of the binding free energy for a variety of complexes to explicate drug resistance (Zhang et al., 2019; Tan et al., 2022). A mechanistic explanation linking the mutations of the protein induce changes in the conformational free-energy landscape was also investigated by using massive molecular dynamics (MD) simulations together with parallel tempering, metadynamics, and one of the best force-fields available, showing a clear shift toward the active conformation for the T790M mutant and the L858R/T790M mutant (Sutto and Gervasio, 2013). The reason for the different binding affinities of ATP with respect to the L858R mutant and the L858R/T790M mutant, however, is still unclear. In addition, the relationship of the conformation changes of A-loop, αC-helix and P-loop and the difference of binding affinity remains to be further explored.
In this article, the standard binding free energies of ATP with respect to two EGFR mutants (L858R, L858R/T790M) have been calculated to investigate the mechanism of the drug resistance induced by the T790M mutation. Pair interaction calculations have been performed to reveal the driving force underlying the change of binding affinity of ATP to EGFR due to the T790M mutation and structural analysis has been carried out to capture the conformational change of the complex. The present study shows the essential reason for the drug resistance induced by the T790M mutation, which can provide useful guidance for the further drug design against drug resistance.
Methods
Structural Modeling
As the cocrystallized structure of EGFR or its mutants in a complex with ATP has not yet been solved, here, we adopted nonhydrolyzable analog AMP of ATP to carry out this research. The crystal structure of an EGFR L858R mutant kinase domain bound with the AMP molecule (PDB: 2EB3) as the structure template to model the EGFR L858R/T790M-AMP complex by CHARMM-GUI (Jo et al., 2008). Neither the protein nor the ligand was protonated. Missing residues in the retrieved structures were also examined and reconstructed using CHARMM-GUI. The atomic coordinates of the EGFR conformations were obtained from the Protein Data Bank (PDB) (http://www.pdb.org).
Molecular Dynamics Simulations
MD simulations for all EGFR models were performed using explicit-solvent periodic boundary conditions using NAMD (Phillips et al., 2020). Each model was solvated in a cubic box of TIP3P water, keeping a distance of 15 Å between the protein and the sides of the solvent box (Jorgensen et al., 1983). Each of the solvated systems was neutralized by adding enough chloride and sodium ions to give a concentration of 250 nM. The CHARMM36m protein force field was used to simulate all protein structures (Huang et al., 2017). The CHARMM General Force Field (CGenFF) force field was used to model the organic molecules (Vanommeslaeghe et al., 2010). All heavy atoms were restrained at the first stage of minimization. After that, the heavy atoms of ligand were fixed in the second step. Finally, all atoms in the system were minimized without any restraint. Production simulations were subsequently performed under the NPT condition at 300 K and 1.013 bar of the system. Temperature and pressure were held constant using Langevin dynamics and the Langevin piston (Uhlenbeck and Ornstein, 1930; Feller et al., 1995). All the trajectories were visualized using the VMD software (Humphrey et al., 1996).
Calculation of Standard Binding Free Energy
The binding free energy acts as a useful index to evaluate the binding affinity between mutants and drugs, and can be used as an important indicator of drug resistance (Zhou et al., 2013; Ma et al., 2015; Khan et al., 2020). In this article, the standard binding free-energy calculations of all systems were performed employing BFEE2 and following a geometrical route (Gumbart et al., 2013; Fu et al., 2021; Fu et al., 2022). BFEE2, which is a graphical user interface-based software, can automatically set up and analyze absolute binding free-energy calculations carried out with the popular MD engine NAMD (Fu et al., 2021; Fu et al., 2022). The calculation process of each protein-ligand complex was divided into eight independent subprocesses. Seven collective variables of geometrical restraints, that is, the root-mean-square deviation (RMSD) for describing the conformational change of the ligand in its bound state with respect to its native conformation, three Euler angles (Θ, Φ, Ψ) for describing the relative orientation of the ligand, the polar and azimuthal angles (θ, φ), together with the distance (r) between the center of mass of the ligand and that of the protein for describing its relative position (Fu et al., 2017), were introduced to accelerate the convergence of free-energy calculations. The contributions of the geometric restraints were evaluated by means of one-dimensional potential of mean force calculations carried out using the well-tempered meta-eABF (WTM-ABF) algorithm (Fu et al., 2016; Lesage et al., 2017; Fu et al., 2018; Fu et al., 2019).
Results and Discussion
Structural Analysis of Ligand-Protein Complexes
Here, after the equilibrated simulations of all systems were completed, the intermolecular interactions of AMP with EGFR mutants were analyzed by the LIGPLOT program. As shown in Figure 1, AMP forms four specific hydrogen bonds with kinase polar residues Gln791, Met793, Arg841, and Asn842 of the L858R mutant and presents a wide hydrophobic contact interface with a number of kinase nonpolar residues, Leu792, Gly796, Val726, Leu718, Ser720, Ala722, Gly721, Ala743, Leu844, and Lys745. Interestingly, AMP also forms four hydrogen bonds with the L858R/T790M mutant, with an average distance shorter than those formed between AMP and the L858R mutant. However, these structural results may not completely explain the experimental observation from kinase assays that AMP has a higher binding affinity with the L858R/T790M mutant compared to the L858R one.
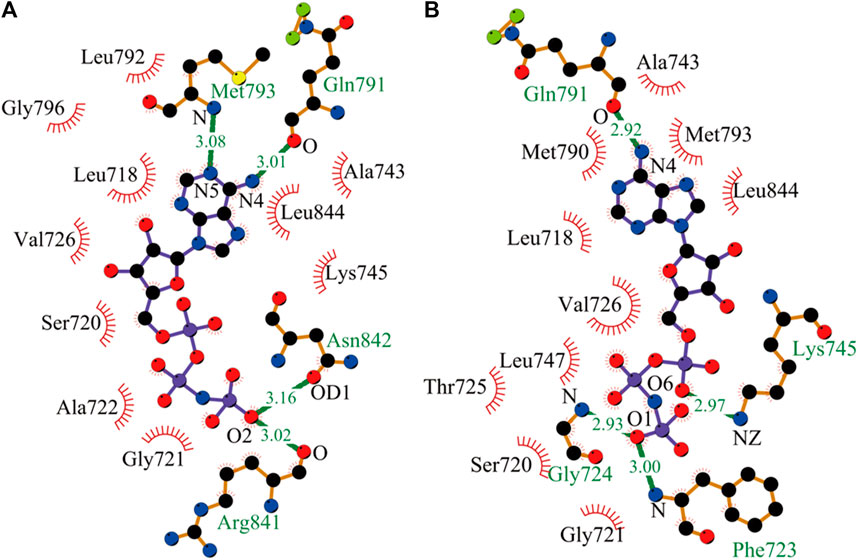
FIGURE 1. Interactions of AMP with its nearby residues in EGFR mutants, plotted by LIGPLOT. (A) Analysis of hydrogen bond and hydrophobic interaction of binding interface in the L858R mutant. (B) The L858R/T790M mutant (Wallace et al., 1995; Laskowski and Swindells, 2011).
Absolute Binding Free Energy of AMP to EGFR Mutants
To evaluate the binding affinity of AMP with EGFR mutants, standard binding free-energy calculations were carried out on two complexes, i.e., AMP-L858R and AMP-L858R/T790M using the CHARMM36m force fields. The computed binding free-energy between AMP and EGFR kinase domains, with the contributions of geometric restraints acting on each degree of freedom, are reported in Table 1. The calculated standard binding free energies of AMP with respect to the L858R and the L858R/T790M double mutant are −5.69 kcal/mol and −6.72 kcal/mol, respectively. These estimates are in good agreement with the experimental values, namely, −5.25 kcal/mol and −6.96 kcal/mol, respectively, suggestive of a remarkable accuracy of BFEE2-based streamlined free-energy calculations. As expected, the binding affinity of AMP to EGFR increased by approximately 1.03 kcal/mol due to the T790M mutation. This result explains that the T790M substitution confers resistance by increasing the affinity for ATP, which was also demonstrated by (Yun et al., 2008). The one-dimensional free-energy profiles for the different contributions are presented in Supplementary Figures S1, S2. As described in Table 1, the major contribution of the absolute binding free energies of AMP with the L858R mutant and L858R/T790M mutant was the -1/β ln (S*I*C°) term in Table 1, which characterizes the separation of the protein and the ligand. The pair interaction energy for the separation was further decoupled into the van der Waals and electrostatic terms, as depicted in Figures 2A,B. It is apparent that electrostatic interactions constitute the driving force for the binding of AMP to the L858R mutant. Both van der Waals and electrostatic interactions, however, are critical to the binding of AMP to the L858R/T790M mutant. In addition, the energy profile characterizing AMP and residue 790 was analyzed. As shown in Figures 2C,D, the T790M mutation increases van der Waals interactions of AMP to EGFR. Based on these results, we conclude that the higher binding affinity of AMP to the L858R/T790M mutant, compared to the L858R one, probably because the T790M mutation increases the van der Waals interaction between AMP and EGFR.
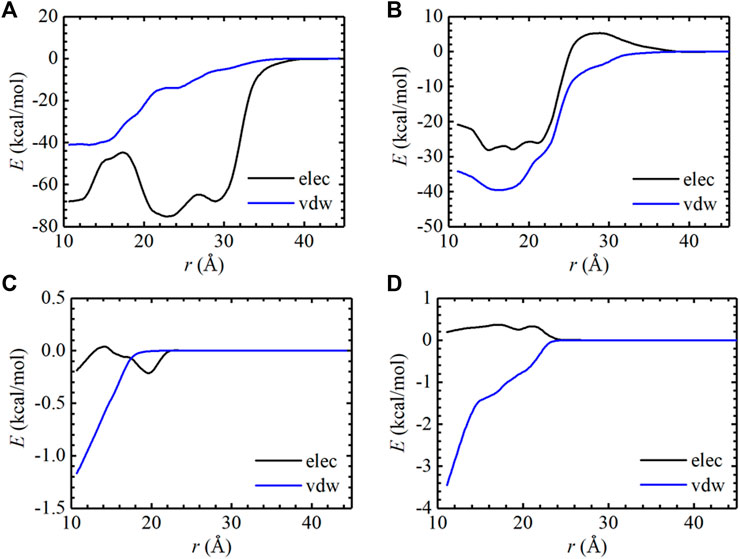
FIGURE 2. Pair interaction energy for the separation of the L858R mutant: AMP (A) and the L858R/T790M mutant: AMP (B) were decoupled into electrostatic and van der Waals contributions. The pair interaction energy for the separation of the Thr790 residue: AMP (C) and the Met790 residue: AMP (D) were decoupled into electrostatic and van der Waals contributions.
Analysis of the Structural Conformational Changes Underlying the Increase of Binding Free Energy
The ATP-binding pocket is composed of a hinge region, A-loop, αC-helix, and P-loop (Figure 3), which are known to be crucial for their conformational stabilities and functional interactions with ATP (Johnson et al., 1996). The conformational changes of A-loop, P-loop, and αC-helix are important events occurring during kinase activation. In this section, we investigated the relationship between the structural changes of these key elements and binding affinity. We characterized the conformational changes of these key elements of EGFR by measuring the distance between these critical elements and ligand (Hu et al., 2022). As shown in Figure 4A, the location of AMP relative to A-loop, αC-helix and P-loop have a shorter distance in the L858R/T790M mutant, contributing to the favorable interactions that existed in the complex. Moreover, Figure 4B shows that the αC-helix is kept in place by a salt bridge formed by E762 and K745 in the L858R/T790M mutant, which is more stable than that observed in the L858R mutant (the average distance between N2 (Lys745) and CD (Glu762) of 3.05 Å vs. 7.86 Å, respectively). Additionally, the pair interaction energy for the separation was further decoupled into the van der Waals and electrostatic terms. As can be seen in Figure 4C, electrostatic interactions constitute the driving force for the binding of αC-helix to AMP in the L858R mutant. Although both electrostatic interactions and van der Waals interactions contribute to the binding of αC-helix to AMP in the L858R/T790M mutant, it is apparent that the effects of electrostatic interactions in higher than van der Waals interactions. The interactions of AMP and A-loop and P-loop are provided in Supplementary Figure S3. Further analysis revealed that the Met790 residue possesses a longer side chain that can has a favorable contact with AMP compared with the Thr790 residue during the conformational change process (Figure 4D). This phenomenon is in agreement with the results of Figures 2C,D. Based on the discussion above, after the T790M mutation, the structural changes of αC-helix and P-loop mainly improve electrostatic interactions and van der Waals interactions, respectively. These are profitable to the binding affinity of AMP to EGFR.
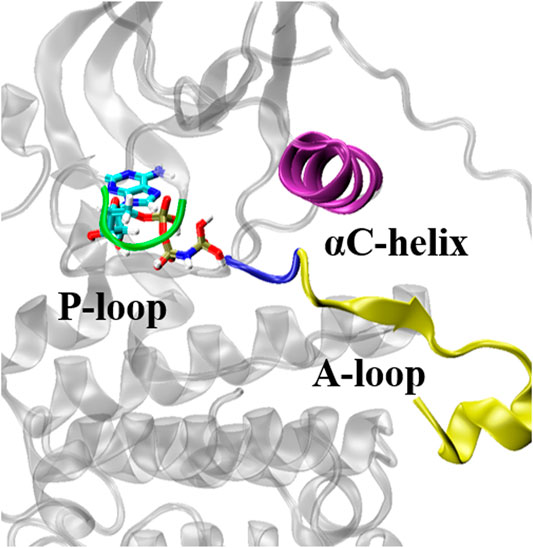
FIGURE 3. Key structural elements structures of EGFR. The key structural elements are highlighted in purple (αC helix), yellow (A-loop), blue (the Asp-Phe-Gly motif) and green (P-loop).
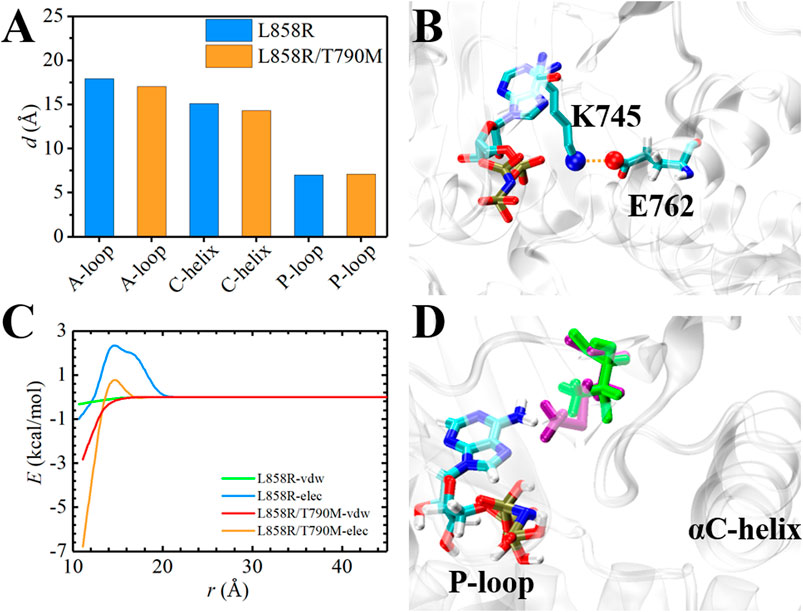
FIGURE 4. Structural analysis of EGFR mutants and AMP. (A) Time-evolution of the average distance between A-loop, αC-helix and P-loop and AMP, respectively. (B) The interaction of E762 and K745 in L858R/T790M mutant. (C) The pair interaction energy for the separation between the ligand and αC-helix of the EGFR mutants. (D) Superposition and comparison between the structures of Thr790-AMP pair and mutant Met790-AMP pair. Thr790 and Met790 are colored in green and purple, respectively.
Conclusion
Here, a powerful tool, BFEE2, was used to calculate the standard binding free energies of AMP to EGFR mutants. The results are well-consistent with the experiment. We found that the kinase affinity for AMP increased after the T790M mutation. In addition, our results indicate that electrostatic interaction plays a leading role in the binding of AMP to the L858R mutant, while both electrostatic interaction and van der Waals interaction are equally important for the binding of AMP to the L858R/T790M mutant. The present work emphasizes that the increased affinity of AMP to the L858R/T790M mutant compared with the L858R mutant is due to better stabilization of the active state for the mutant. This change may increase the interactions of AMP and P-loop, αC helix after the T790M mutation, therefore enhancing the binding affinity of AMP to EGFR. Although the calculated standard binding free energies are in good agreement with experimental values, there are challenges in the calculation of the standard binding free energies of EGFR inhibitors, especially for some of the fourth generation EGFR inhibitors without accurate binding sites. Still, the present work offers a perspective of the binding affinity of AMP to EGFR mutants and opens an avenue for further exploration of anticancer drugs acting on the EGFR to overcome drug resistance caused by the T790M mutation.
Data Availability Statement
The original contributions presented in the study are included in the article/Supplementary Material, further inquiries can be directed to the corresponding authors.
Author Contributions
HZ, HF, XS, and WC designed the project. HZ performed all the MD simulations and analyses. HZ and HF wrote the manuscript. HL participated in the writing and discussion of the manuscript. All authors participated in editing the manuscript.
Funding
The work was supported by the National Natural Science Foundation of China (Grants 22073050, 22174075, and 22103041), the China Post-doctoral Science Foundation (Grants bs6619012).
Conflict of Interest
The authors declare that the research was conducted in the absence of any commercial or financial relationships that could be construed as a potential conflict of interest.
Publisher’s Note
All claims expressed in this article are solely those of the authors and do not necessarily represent those of their affiliated organizations, or those of the publisher, the editors and the reviewers. Any product that may be evaluated in this article, or claim that may be made by its manufacturer, is not guaranteed or endorsed by the publisher.
Acknowledgments
We thank the Haihe Laboratory of Sustainable Chemical Transformations for financial support (ZYTS202105).
Supplementary Material
The Supplementary Material for this article can be found online at: https://www.frontiersin.org/articles/10.3389/fmolb.2022.922839/full#supplementary-material
References
Feller, S. E., Zhang, Y., Pastor, R. W., and Brooks, B. R. (1995). Constant Pressure Molecular Dynamics Simulation: The Langevin Piston Method. J. Chem. Phys. 103, 4613–4621. doi:10.1063/1.470648
Fu, H., Cai, W., Hénin, J., Roux, B., and Chipot, C. (2017). New Coarse Variables for the Accurate Determination of Standard Binding Free Energies. J. Chem. Theory Comput. 13, 5173–5178. doi:10.1021/acs.jctc.7b00791
Fu, H., Chen, H., Blazhynska, M., de Lacam, E. G. C., Szczepaniak, F., Pavlova, A., et al. (2022). Accurate Determination of Protein:ligand Standard Binding Free Energies from Molecular Dynamics Simulations. Nat. Protoc. 17, 1114–1141. doi:10.1038/s41596-021-00676-1
Fu, H., Chen, H., Cai, W., Shao, X., and Chipot, C. (2021). BFEE2: Automated, Streamlined, and Accurate Absolute Binding Free-Energy Calculations. J. Chem. Inf. Model. 61, 2116–2123. doi:10.1021/acs.jcim.1c00269
Fu, H., Shao, X., Cai, W., and Chipot, C. (2019). Taming Rugged Free Energy Landscapes Using an Average Force. Acc. Chem. Res. 52, 3254–3264. doi:10.1021/acs.accounts.9b00473
Fu, H., Shao, X., Chipot, C., and Cai, W. (2016). Extended Adaptive Biasing Force Algorithm. An On-The-Fly Implementation for Accurate Free-Energy Calculations. J. Chem. Theory Comput. 12, 3506–3513. doi:10.1021/acs.jctc.6b00447
Fu, H., Zhang, H., Chen, H., Shao, X., Chipot, C., and Cai, W. (2018). Zooming across the Free-Energy Landscape: Shaving Barriers, and Flooding Valleys. J. Phys. Chem. Lett. 9, 4738–4745. doi:10.1021/acs.jpclett.8b01994
Gumbart, J. C., Roux, B., and Chipot, C. (2013). Standard Binding Free Energies from Computer Simulations: What Is the Best Strategy? J. Chem. Theory Comput. 9, 794–802. doi:10.1021/ct3008099
Hirsch, F. R., Varella-Garcia, M., Bunn, P. A., Di Maria, M. V., Veve, R., Bremnes, R. M., et al. (2003). Epidermal Growth Factor Receptor in Non-small-cell Lung Carcinomas: Correlation between Gene Copy Number and Protein Expression and Impact on Prognosis. Jco 21, 3798–3807. doi:10.1200/Jco.2003.11.069
Hu, X., Pang, J., Zhang, J., Shen, C., Chai, X., Wang, E., et al. (2022). Discovery of Novel GR Ligands toward Druggable GR Antagonist Conformations Identified by MD Simulations and Markov State Model Analysis. Adv. Sci. 9, 2102435. doi:10.1002/advs.202102435
Huang, J., Rauscher, S., Nawrocki, G., Ran, T., Feig, M., de Groot, B. L., et al. (2017). CHARMM36m: An Improved Force Field for Folded and Intrinsically Disordered Proteins. Nat. Methods. 14, 71–73. doi:10.1038/Nmeth.4067
Humphrey, W., Dalke, A., and Schulten, K. (1996). VMD: Visual Molecular Dynamics. J. Mol. Graph. 14, 33–38. doi:10.1016/0263-7855(96)00018-5
Inamura, K. (2017). Lung Cancer: Understanding its Molecular Pathology and the 2015 WHO Classification. Front. Oncol. 7, 193. doi:10.3389/fonc.2017.00193
Jemal, A., Bray, F., Center, M. M., Ferlay, J., Ward, E., and Forman, D. (2011). Global Cancer Statistics. CA A Cancer J. Clin. 61, 69–90. doi:10.3322/caac.20107
Jo, S., Kim, T., Iyer, V. G., and Im, W. (2008). CHARMM-GUI: A Web-Based Graphical User Interface for CHARMM. J. Comput. Chem. 29, 1859–1865. doi:10.1002/jcc.20945
Johnson, L. N., Noble, M. E. M., and Owen, D. J. (1996). Active and Inactive Protein Kinases: Structural Basis for Regulation. Cell 85, 149–158. doi:10.1016/s0092-8674(00)81092-2
Jorgensen, W. L., Chandrasekhar, J., Madura, J. D., Impey, R. W., and Klein, M. L. (1983). Comparison of Simple Potential Functions for Simulating Liquid Water. J. Chem. Phys. 79, 926–935. doi:10.1063/1.445869
Khan, M. T., Ali, S., Zeb, M. T., Kaushik, A. C., Malik, S. I., and Wei, D.-Q. (2020). Gibbs Free Energy Calculation of Mutation in PncA and RpsA Associated with Pyrazinamide Resistance. Front. Mol. Biosci. 7, 52. doi:10.3389/fmolb.2020.00052
Kobayashi, S., Boggon, T. J., Dayaram, T., Jänne, P. A., Kocher, O., Meyerson, M., et al. (2005). EGFRMutation and Resistance of Non-small-cell Lung Cancer to Gefitinib. N. Engl. J. Med. 352, 786–792. doi:10.1056/NEJMoa044238
Kosaka, T., Yatabe, Y., Endoh, H., Yoshida, K., Hida, T., Tsuboi, M., et al. (2006). Analysis of Epidermal Growth Factor Receptor Gene Mutation in Patients with Non-small Cell Lung Cancer and Acquired Resistance to Gefitinib. Clin. Cancer Res. 12, 5764–5769. doi:10.1158/1078-0432.CCR-06-0714
Kwak, E. L., Sordella, R., Bell, D. W., Godin-Heymann, N., Okimoto, R. A., Brannigan, B. W., et al. (2005). Irreversible Inhibitors of the EGF Receptor May Circumvent Acquired Resistance to Gefitinib. Proc. Natl. Acad. Sci. U.S.A. 102, 7665–7670. doi:10.1073/pnas.0502860102
Laskowski, R. A., and Swindells, M. B. (2011). LigPlot+: Multiple Ligand-Protein Interaction Diagrams for Drug Discovery. J. Chem. Inf. Model. 51, 2778–2786. doi:10.1021/ci200227u
Lesage, A., Lelièvre, T., Stoltz, G., and Hénin, J. (2017). Smoothed Biasing Forces Yield Unbiased Free Energies with the Extended-System Adaptive Biasing Force Method. J. Phys. Chem. B 121, 3676–3685. doi:10.1021/acs.jpcb.6b10055
Liao, P. L., Cheng, Y. W., Li, C. H., Wang, Y. T., and Kang, J. J. (2010). 7-Ketocholesterol and Cholesterol-5α,6α-Epoxide Induce Smooth Muscle Cell Migration and Proliferation through the Epidermal Growth Factor Receptor/phosphoinositide 3-kinase/Akt Signaling Pathways. Toxicol. Lett. 197, 88–96. doi:10.1016/j.toxlet.2010.05.002
Ma, L., Wang, D. D., Huang, Y., Yan, H., Wong, M. P., and Lee, V. H. (2015). EGFR Mutant Structural Database: Computationally Predicted 3D Structures and the Corresponding Binding Free Energies with Gefitinib and Erlotinib. BMC Bioinforma. 16, 85. doi:10.1186/s12859-015-0522-3
Nagano, T., Tachihara, M., and Nishimura, Y. (2018). Mechanism of Resistance to Epidermal Growth Factor Receptor-Tyrosine Kinase Inhibitors and a Potential Treatment Strategy. Cells 7, 212. doi:10.3390/cells7110212
Normanno, N., De Luca, A., Bianco, C., Strizzi, L., Mancino, M., Maiello, M. R., et al. (2006). Epidermal Growth Factor Receptor (EGFR) Signaling in Cancer. Gene 366, 2–16. doi:10.1016/j.gene.2005.10.018
Pao, W., Miller, V. A., Politi, K. A., Riely, G. J., Somwar, R., Zakowski, M. F., et al. (2005). Acquired Resistance of Lung Adenocarcinomas to Gefitinib or Erlotinib Is Associated with a Second Mutation in the EGFR Kinase Domain. Plos Med. 2, 225–235. doi:10.1371/journal.pmed.0020073
Phillips, J. C., Hardy, D. J., Maia, J. D. C., Stone, J. E., Ribeiro, J. V., Bernardi, R. C., et al. (2020). Scalable Molecular Dynamics on CPU and GPU Architectures with NAMD. J. Chem. Phys. 153, 044130. doi:10.1063/5.0014475
Saldaña-Rivera, L., Bello, M., and Méndez-Luna, D. (2019). Structural Insight into the Binding Mechanism of ATP to EGFR and L858R, and T790M and L858R/T790 Mutants. J. Biomol. Struct. Dyn. 37, 4671–4684. doi:10.1080/07391102.2018.1558112
Santos, R., Ursu, O., Gaulton, A., Bento, A. P., Donadi, R. S., Bologa, C. G., et al. (2017). A Comprehensive Map of Molecular Drug Targets. Nat. Rev. Drug Discov. 16, 19–34. doi:10.1038/nrd.2016.230
Sharma, S. V., Bell, D. W., Settleman, J., and Haber, D. A. (2007). Epidermal Growth Factor Receptor Mutations in Lung Cancer. Nat. Rev. Cancer. 7, 169–181. doi:10.1038/nrc2088
Sutto, L., and Gervasio, F. L. (2013). Effects of Oncogenic Mutations on the Conformational Free-Energy Landscape of EGFR Kinase. Proc. Natl. Acad. Sci. U.S.A. 110, 10616–10621. doi:10.1073/pnas.1221953110
Tan, S., Zhang, Q., Wang, J., Gao, P., Xie, G., Liu, H., et al. (2022). Molecular Modeling Study on the Interaction Mechanism between the LRRK2 G2019S Mutant and Type I Inhibitors by Integrating Molecular Dynamics Simulation, Binding Free Energy Calculations, and Pharmacophore Modeling. ACS Chem. Neurosci. 13, 599–612. doi:10.1021/acschemneuro.1c00726
Uhlenbeck, G. E., and Ornstein, L. S. (1930). On the Theory of the Brownian Motion. Phys. Rev. 36, 823–841. doi:10.1103/physrev.36.823
Vanommeslaeghe, K., Hatcher, E., Acharya, C., Kundu, S., Zhong, S., Shim, J., et al. (2010). CHARMM General Force Field: A Force Field for Drug-like Molecules Compatible with the CHARMM All-Atom Additive Biological Force Fields. J. Comput. Chem. 31, 671–690. doi:10.1002/jcc.21367
Wallace, A. C., Laskowski, R. A., and Thornton, J. M. (1995). LIGPLOT: A Program to Generate Schematic Diagrams of Protein-Ligand Interactions. Protein Eng. Des. Sel. 8, 127–134. doi:10.1093/protein/8.2.127
Wee, P., and Wang, Z. (2017). Epidermal Growth Factor Receptor Cell Proliferation Signaling Pathways. Cancers 9, 52. doi:10.3390/cancers9050052
Yun, C.-H., Mengwasser, K. E., Toms, A. V., Woo, M. S., Greulich, H., Wong, K.-K., et al. (2008). The T790M Mutation in EGFR Kinase Causes Drug Resistance by Increasing the Affinity for ATP. Proc. Natl. Acad. Sci. U.S.A. 105, 2070–2075. doi:10.1073/pnas.0709662105
Zhang, Q., An, X., Liu, H., Wang, S., Xiao, T., and Liu, H. (2019). Uncovering the Resistance Mechanism of Mycobacterium tuberculosis to Rifampicin Due to RNA Polymerase H451D/Y/R Mutations from Computational Perspective. Front. Chem. 7, 819. doi:10.3389/fchem.2019.00819
Zhou, H., Fu, J., Jia, Q., Wang, S., Liang, P., Wang, Y., et al. (2022). Magnetic Nanoparticles Covalently Immobilizing Epidermal Growth Factor Receptor by SNAP-Tag Protein as a Platform for Drug Discovery. Talanta 240, 123204. doi:10.1016/j.talanta.2021.123204
Zhou, W., Wang, D. D., Yan, H., Wong, M., and Lee, V. (2013). “Prediction of Anti-EGFR Drug Resistance Base on Binding Free Energy and Hydrogen Bond Analysis,” in 2013 IEEE Symposium on Computational Intelligence in Bioinformatics and Computational Biology (CIBCB), Singapore, 16-19 April 2013, 193–197. doi:10.1109/CIBCB.2013.6595408
Keywords: absolute binding free energy calculation, Epidermal Growth Factor Receptor (EGFR), T790M mutation, drug resistance, molecular dynamics simulation, BFEE2
Citation: Zhou H, Fu H, Liu H, Shao X and Cai W (2022) Uncovering the Mechanism of Drug Resistance Caused by the T790M Mutation in EGFR Kinase From Absolute Binding Free Energy Calculations. Front. Mol. Biosci. 9:922839. doi: 10.3389/fmolb.2022.922839
Received: 18 April 2022; Accepted: 16 May 2022;
Published: 30 May 2022.
Edited by:
Weiliang Zhu, Shanghai Institute of Materia Medica (CAS), ChinaReviewed by:
Shan Chang, Jiangsu University of Technology, ChinaXuemei Pu, Sichuan University, China
Xiao Jun Yao, Macau University of Science and Technology, Macao SAR, China
Copyright © 2022 Zhou, Fu, Liu, Shao and Cai. This is an open-access article distributed under the terms of the Creative Commons Attribution License (CC BY). The use, distribution or reproduction in other forums is permitted, provided the original author(s) and the copyright owner(s) are credited and that the original publication in this journal is cited, in accordance with accepted academic practice. No use, distribution or reproduction is permitted which does not comply with these terms.
*Correspondence: Xueguang Shao, eHNoYW9AbmFua2FpLmVkdS5jbg==; Wensheng Cai, d3NjYWlAbmFua2FpLmVkdS5jbg==