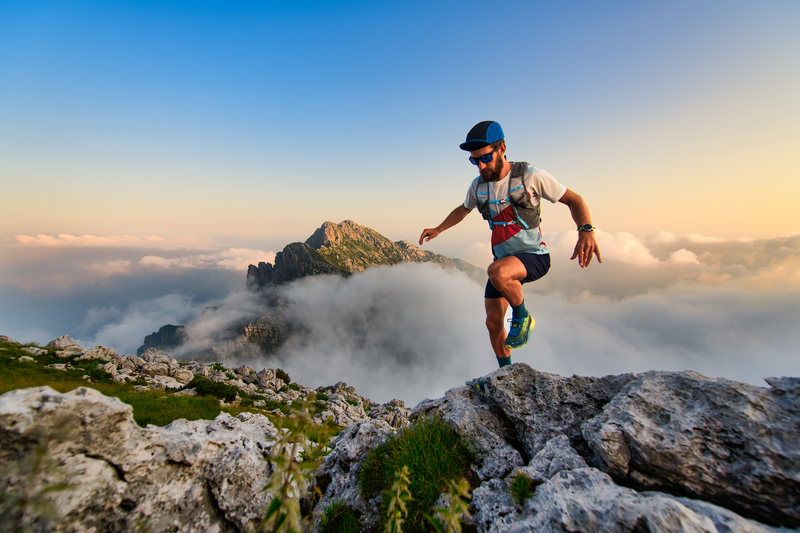
94% of researchers rate our articles as excellent or good
Learn more about the work of our research integrity team to safeguard the quality of each article we publish.
Find out more
ORIGINAL RESEARCH article
Front. Mol. Biosci. , 14 July 2022
Sec. Molecular Diagnostics and Therapeutics
Volume 9 - 2022 | https://doi.org/10.3389/fmolb.2022.917602
This article is part of the Research Topic Ferroptosis in Cancer and Beyond View all 34 articles
Ferroptosis is a form of non-apoptotic and iron-dependent cell death originally identified in cancer cells. Recently, emerging evidence showed that ferroptosis-targeting therapy could be a novel promising anti-tumour treatment. However, systematic analyses of ferroptosis-related genes for the prognosis of non-small cell lung cancer (NSCLC) and the development of antitumor drugs exploiting the ferroptosis process remain rare. This study aimed to identify genes related to ferroptosis and NSCLC and to initially screen lead compounds that induce ferroptosis in tumor cells. We downloaded mRNA expression profiles and NSCLC clinical data from The Cancer Genome Atlas database to explore the prognostic role of ferroptosis-related genes. Four prognosis-associated ferroptosis-related genes were screened using univariate Cox regression analysis and the lasso Cox regression analysis, which could divide patients with NSCLC into high- and low-risk groups. Then, based on differentially expressed risk- and ferroptosis-related genes, the negatively correlated lead compound flufenamic acid (FFA) was screened through the Connective Map database. This project confirmed that FFA induced ferroptosis in A549 cells and inhibited growth and migration in a dose-dependent manner through CCK-8, scratch, and immunofluorescence assays. In conclusion, targeting ferroptosis might be a therapeutic alternative for NSCLC.
Lung cancer is one of the most common malignant tumors in China and the world. Non-small cell lung cancer (NSCLC) accounts for 85% of all lung cancers and is the main cause of cancer-related deaths worldwide (Liu et al., 2017). NSCLC, including adenocarcinoma, squamous cell carcinoma, and large cell carcinoma, is a heterogeneous group of diseases that is frequently diagnosed at advanced or metastatic stages (Best and Sutherland, 2018; La Montagna et al., 2020). It is well known that various prognostic factors based on clinical and pathological characteristics determine the overall prognosis of patients, and the most important prognostic factors remain the stage and presentation of the disease at the time of diagnosis (Gadgeel and Thakur, 2016). The lack of obvious symptoms in the early stages of disease progression and late diagnosis might explain the observed poor prognosis (Liu et al., 2017). Han K and Wang Y et al. demonstrated that ferroptosis-related genes are closely associated with the prognosis of NSCLC patients, which will allow for the effective treatment of patients (Han et al., 2021; Wang et al., 2022).
Ferroptosis is an iron-dependent, non-apoptotic mode of programmed cell death that is driven by the lethal accumulation of lipid peroxides (Liang et al., 2020; Li Y. et al., 2020). Previous studies have reported that ferroptosis plays a vital role in NSCLC, and certain genes, such as SLC7A11 (Ji et al., 2018; Kang et al., 2019) and GPX4 (Lai et al., 2019), are known to negatively regulate ferroptosis; moreover, NFS1, a ferroptosis-related gene, is most highly expressed in well-differentiated lung adenocarcinomas and protect cells from ferroptosis (Alvarez et al., 2017). The activation of ferroptosis by several small molecules and FDA-approved clinical drugs in cancer cells and the efficacy of tumor suppression with ferroptosis inducers in various experimental cancer models underline the potential of ferroptosis to be targeted as a novel anti-cancer therapy (Stockwell et al., 2017). Therefore, this study intended to screen out new lead compounds for the treatment of NSCLC based on the induction of ferroptosis in NSCLC cells using bioinformatics theory.
In this study, we used mRNA expression data from NSCLC patients in The Cancer Genome Atlas (TCGA) database to construct a prognostic multigene signature with ferroptosis-related differentially expressed genes (DEGs). These ferroptosis-related risk signatures could independently and effectively classify patients with NSCLC with a high risk of unfavorable outcomes. Finally, we preliminarily screened and proved that the lead compound, flufenamic acid, could induce ferroptosis with anticancer effects by using biological experiments and bioinformatics analysis. These results provide a new strategy for the treatment of NSCLC, help to reveal the association between ferroptosis-related genes and patient prognosis, and also offer basic research data for targeting ferroptosis in the treatment of NSCLC.
The Cell Counting Kit-8 (CCK-8, C0005) and flufenamic acid (FFA, T0858) were purchased from Taoshu Biotechnology Co., Ltd. Ferrostatin-1(Fer-1, HY-100579) was purchased from MedChemExpress Company. Rabbit anti-human glutathione peroxidase 4 (GPX4, A1933) monoclonal antibody was received from ABclonal Technology Co.,Ltd. The Goat Anti-Rabbit lgG H&L/FITC antibody (bs-0295G-FITC) was acquired from Beijing Boaosen Biotechnology Co., Ltd. Glutathione (GSH, A126-1-1) detection kits was purchased from Nanjing Jiancheng Bioengineering Institute.
The RNA sequencing data of HTSeq-FPKM and relevant clinical information from the NSCLC cohort were downloaded from TCGA database (https://portal.gdc.cancer.gov/repository). RNA-seq data and clinical information from 228 tumor samples were obtained from the GEO database (GSE 37745, GSE102287, https://www.ncbi.nlm.nih.gov/geo).
60 ferroptosis-related genes were retrieved from previous literature (Stockwell et al., 2017; Bersuker et al., 2019; Hassannia et al., 2019) and are provided in Supplementary Table S1. The R package “limma” and “sva” were used to extract mRNA expression levels of ferroptosis-related genes in TCGA and GEO databases.
DEGs related to ferroptosis were screened using the R package “limma” (Ritchie et al., 2015). The expression of candidate ferroptosis-related genes in the TCGA cohort was used to identify the DEGs between tumor tissues and adjacent nontumorous tissues. The screening criteria were set as a p-value < 0.05 and false discovery rate (FDR) < 0.05.
Univariate Cox analysis regression was performed to evaluate the association between DGEs and patient survival time in TCGA cohort using the R package “survival”, and to obtain the corresponding p-value and hazard ratio (HR) for each differential gene. When the p-value was <0.05, it was considered that the ferroptosis-related gene was connected with patient prognosis. The higher the HR, the higher the risk of the disease.
The R package “glmnet” was used to perform lasso regression analysis. The candidate ferroptosis-related genes were subjected to univariate Cox linear regression analysis using the R package “survival”. The candidate ferroptosis-related gene was taken as the included signature variable, and the variables were added or deleted until the optimum multigene signature to evaluate the overall survival (OS) outcome was found. The formula for the risk score was as follows: risk score = the sum of each coefficient of mRNA multiple for the expression of each mRNA.
Patients were divided into high and low-risk groups based on the median risk score of TCGA cohort. The R package “survival” and “survminer” was uesd to perform Kaplan–Meier survival analysis and log-rank tests, which were used to evaluate the OS difference between high- and low-risk groups. A p-value of the log-rank test was less than 0.05 indicated a statistically significant difference in survival between the two groups. To validate the survival analysis of TCGA cohort, the Kaplan-Meier method was performed on the GEO cohort to evaluate the OS difference between high- and low-risk groups.
The patient risk scores and clinicopathological characteristics such as age, sex, tumor stage, and grade were taken as variables, and the corresponding survival status was taken as dependent variables. Univariate and multivariate Cox regression analyses were conducted to obtain the risk ratio HR and seek factors that could independently be used to evaluate a patient’s risk of developing the disease. A factor was used as an independent risk factor when the p-value was <0.05.
The R package “survivalROC” was used to generate a receiver operating characteristic curve (ROC curve), which was used to evaluate the specificity and sensitivity of the risk score for patient survival prediction and the diagnostic score for the prediction of patient diagnosis. The robustness of the risk score was evaluated by comparing the area under the curve (AUC) of the ROC curves for different factors.
The differential genes related to ferroptosis and those of high- and low-risk patients were entered into the CMap (https://clue.io/query) database in Grp form. With this comparison, related small molecule compounds or drugs were obtained. Taking p < 0.05 and enrichment <0 as the screening conditions, the potential small molecule lead compounds for the treatment of NSCLC were screened after the intersection of the two groups of screened drugs.
A549 cells were routinely cultured with 1,640 medium containing 10% fetal bovine serum, digested and passaged, inoculated into a 6-well plate according at 5 × 106 cells/well, or inoculated into a 96-well plate with 1 × 104 cells/well. The cells were cultured in a 5% carbon dioxide incubator for 24 h. After 24 h of drug treatment, the activity in each group of cells was detected using a CCK-8 kit. The cells were incubated in a 96-well plate, 10 μL of CCK-8 solution was added to each well, and the culture was continued at 37°C for 4 h. Absorbance was measured at 450 nm. The formula to calculate cell viability was as follows: cell viability (%) = [A (treatment) − A (blank)]/[A (control) − A (blank)]. The cell wound healing assay was performed according to Li’s research (Li Z.-Y. et al., 2020).
A549 cells were divided into a negative control group (NC), FFA-treated group (FFA), and ferrostatin-1 (Fer-1) and combined treatment groups (Fer-1+FFA). After 24 h of drug treatment, the CCK-8 kit was utilized as described. GSH detection kits was used to evaluate the contents of GSH in the cells of each group. The operation steps were performed according to the instructions. Immunofluorescence staining was performed according to Yang’s instructions (Yang et al., 2019). The primary antibody was a rabbit anti-human GPX4 monoclonal antibody, dilution 1:200.
Spss26.0, Prism 8.0, Image J, SigmaPlot 14, and R software were used for statistical analysis of results (Student’s t-test or one-way ANOVA tests). Data were presented as mean ± standard error of the mean. tje Kaplan-Meier method and log-rank tests were used to evaluate the predictive power of the prognostic model. Univariate Cox regression and multivariate Cox regression were used to identify whether the model could be used as an independent prognostic factor. p < 0.05 was considered statistically significant.
In this study, 551 cases were obtained from TCGA database, including 54 normal datasets and 497 tumor datasets. A total of 316 cases with clinical characteristics met the inclusion criteria by removing cases with survival periods of less than 30 days with incomplete clinical information. We downloaded a total of 228 cases from the GEO database, including 161 cases with researchable clinical information. The detailed clinical characteristics of these patients, such as age, gender, survival time, and survival status, are summarized in Table 1.
Sixty ferroptosis-related genes were extracted from TCGA cohort. A nonparametric rank-sum test was performed on 497 patients with tumor tissues and 54 patients with normal lung tissues, and a total of 43 ferroptosis-related genes met the screening criteria of p-value < 0.05 and FDR <0.05, including 29 upregulated and 14 downregulated ferroptosis-related genes. These genes were clustered and presented as heatmaps. A heatmap of these differentially expressed ferroptosis-related genes is shown in Figure 1.
FIGURE 1. The heatmap of differentially expressed ferroptosis-related genes in the TCGA cohort. Compared with normal samples, a total of 29 up-regulated and 14 down-regulated ferroptosis-related genes were screened in tumor samples.
In view of the above differentially expressed ferroptosis-related genes, combined with the clinical information on survival status and OS days patients in the TCGA cohort, five ferroptosis-related genes related to the prognosis of NSCLC were preliminarily screened using univariate Cox analysis (Figure 2A). Irrationally, PHKG2 was excluded from further studies, as its expression in univariate Cox regression analysis predicted it to be a low-risk gene with an excellent prognosis. Four prognostic ferroptosis-related DEGs were identified as key genes using lasso regression analysis and preserved (Figure 2B).
FIGURE 2. Identification of the candidate ferroptosis-related genes in TCGA cohort. (A) Venn diagram to identify differentially expressed genes between tumor and adjacent normal tissue that were correlated with overall survival (OS). The red circle represents the ferroptosis-related genes associated with prognosis, the green circle represents DEGs associated with ferroptosis. (B) Forest plots indicating results of the univariate Cox regression analysis between gene expression and OS. p-values < 0.05 indicate that ferroptosis-related genes are related to the prognosis of NSCLC. When the hazard ratio (HR) is >1, the gene is considered a high-risk gene.
Based on the four ferroptosis-related genes identified using lasso regression analysis, the prognostic risk score of each patient was calculated using the risk score expression described above. The risk score = 0.0415124361172914 × expression of CISD1 + 0.0643218824479954 × expression of FANCD2 + 0.0018714013929312 × expression of GCLC + 0.0139528364527794 × expression of ACSL3. All patients were divided into high-risk and low-risk groups according to the median risk score of TCGA cohort.
Kaplan-Meier survival analysis showed that the OS of the low-risk group was higher than that of the high-risk group in both TCGA (Figure 3A) and GEO cohorts (Figure 3B). p-values < 0.05 indicate that the difference in OS curves between high-risk and low-risk groups is considered statistically significant.
FIGURE 3. Survival analyses of the prognostic ferroptosis-related genes in NSCLC using the Kaplan-Meier method. (A) The Kaplan-Meier curve of TCGA cohort. (B) The Kaplan-Meier curve of the GEO cohort. p-values < 0.05 indicate that the OS differed significantly between high- and low-risk patients.
The patients were separated into high- or low-risk groups based on the median cut-off value (Figures 4A,B). In both TCGA and GEO cohorts, the risk score increased from left to right. The distribution of the survival status demonstrated that the number of deaths increased gradually with the increase in risk value. High-risk patients displayed shorter OS days and a higher probability of early death compared to low-risk patients (Figures 4C,D). Further, the heat maps clearly indicated that the expression of CISD1, FANCD2, GCLC, and ACSL3 were added in the wake of the increase in risk value in both TCGA and GEO cohorts (Figures 4E,F). Based on the expression of this four potential risk genes, we classified patients with NSCLC into high- and low-risk groups. PCA analysis clearly indicated that patients in different risk groups were distributed in two directions (Figures 4G,H).
FIGURE 4. The risk score, survival status distribution, heatmap and PCA plot of TCGA and GEO cohort. (A) The distribution and median value of the risk scores in TCGA cohort. (B) The distribution and median value of the risk scores in the GEO cohort. (C) The distributions of OS status, OS, and risk score in TCGA cohort. (D) The distributions of OS status, OS, and risk score in the GEO cohort. (E) The heatmap for prognosis-associated ferroptosis-related genes in TCGA cohort. (F) The heatmap for prognosis-associated ferroptosis-related genes in the GEO cohort. (G) PCA plot of TCGA cohort. (H) PCA plot of the GEO cohort.
Univariate and multivariate Cox regression analyses were used to examine whether the predicted risk incidence of the risk score was independent of clinical data in both TCGA and GEO cohorts. The risk score was significantly correlated with OS in both TCGA and GEO cohorts in univariate Cox regression analyses (HR = 2.918, p < 0.001; HR = 2.632, p = 0.040, respectively). Surprisingly, in TCGA cohort, stage is considered interrelated to OS, but cannot be verified in the GEO cohort (Figures 5A,B). The risk of poor survival outcomes increased with an increase in the risk score. After adjusting for other intricate factors, multivariate Cox regression analysis demonstrated that the risk score remained an independent predictor of OS in NSCLC patients (HR = 2.407, p = 0.009; HR = 3.900, p = 0.005, respectively, Figures 5C,D.)
FIGURE 5. Forest plot of the univariate and multivariate Cox regression analyses in NSCLC. Forest plot of the univariate Cox regression analysis of TCGA cohort (A) and GEO cohort (B). Forest plot of the multivariate Cox regression analysis of TCGA cohort (C) and GEO cohort (D).
Receiver operating characteristic (ROC) analysis, which is a method combining sensitivity and specificity, was performed to comprehensively evaluate the multivariate Cox regression analysis for long-term survival prediction (7 years). The area under the curve (AUC) of the risk score in TCGA cohorts was 0.611 (Figure 6A) and the area under the curve (AUC) of the risk score in GEO cohorts was 0.651 (Figure 6B), making it clear that the predictive model possessed powerful prognostic capability in forecasting overall survival.
FIGURE 6. The receiver operating characteristic (ROC) curve of TCGA and GEO cohorts. (A) the ROC curve of TCGA cohorts. (B) the ROC curve of GEO cohorts. The AUC of the ROC curve was more than 0.5, which signified that the predictive model possessed extraordinary diagnosis and prediction values.
Based on the query results of the CMap database, the partial potential lead compounds for the treatment of high-risk NSCLC patients are listed in Figure 7A, and the partial potential lead compounds that might trigger ferroptosis in NSCLC are listed in Figure 7B. After the intersection of the two groups of screened drugs, we believed the small molecule compound, FFA, was a therapeutic agent to induce ferroptosis in NSCLC. Previous studies have found that FFA exerts antitumor effects (Matsumoto et al., 2016; Li Z.-Y. et al., 2020), suggesting that our screening strategy and results possess preferable credibility.
FIGURE 7. Partial analysis results from CMap database. (A) Prediction results from cMap for the differential gene profiles of high- and low-risk groups. (B) Prediction results from cMap for the differential gene profiles of DEGs related to ferroptosis.
After exposing cells to different concentrations of FFA (50, 100, 150, 200 μmol/L) for 24 h, as shown in Figure 8A, cell viability was reduced with an increase in the FFA concentration. Cell migration into the wound was measured according to the distance between the wound edges before and after FFA treatment. We found that FFA significantly impaired cell migration into the wound (Figures 8B,C). As shown in Figures 8A,D,E, low doses of FFA (50 and 100 μmol/L) had a negligible effect on the growth of normal lung cells (BEAS-2B).
FIGURE 8. Flufenamic acid inhibits cell viability and migration in A549 cells. (A) Effects of different concentrations of flufenamic acid on the cellular activity in A549 cells and BEAS-2B cells (n = 5).***p < 0.001 vs. NC of A549 cells. ###p < 0.001 vs. NC of BEAS-2B cells. (B) The scratch healing of A549 cells in different time periods. The scale bar is 50 μm. (C) Statistical chart of cell migration ratio in A549 cells (n = 3). ***p < 0.001 vs. NC. (D) The scratch healing of BEAS-2B cells in different time periods. The scale bar is 50 μm. (E) Statistical chart of cell migration ratio in BEAS-2B cells (n = 3). NC, negative control group.
It is well known that the GSH depletion caused by cysteine deficiency directly inactivates glutathione peroxidase 4 (GPX4) and leads to subsequent ferroptosis (He et al., 2022). Our results showed that FFA remarkably reduced the expression of GSH and GPX4 in A549 cells, whereas Fer-1, an inhibitor of ferroptosis, significantly reversed this trend (Figures 9B–D). The A549 cell activity reduced by FFA could not completely reversed by Fer-1, indicating that the antitumor effect of FFA was not only mediated by ferroptosis (Figure 9A). Therefore, we concluded that FFA partially induced ferroptosis in NSCLC cells by repressing GSH-dependent GPX4 signaling.
FIGURE 9. Flufenamic acid(FFA) partially induces ferroptosis in A549 cells by repressing GSH-dependent GPX4 signaling pathway. (A) Effect of Ferrostatin-1(Fer-1) on flufenamic acid-restrained cellular activity in A549 cells (n = 5). (B) Fer-1 reverses the reduced levels of GSH by FFA in A549 cells (n = 3). (C) Observation of GPX4 immunofluorescence staining in each group (×400). (D) Statistical diagram of the average fluorescence intensity of cells in each group (n = 6). NC, negative control group. **p < 0.01 and ***p < 0.001.
Lung cancer is one of the most common malignancies and accounts for nearly one quarter of cancer deaths worldwide (Siegel et al., 2021). The most representative histological type of lung cancer is NSCLC, which is generally diagnosed at an advanced stage owing to finite symptoms at the early stage and the restriction of proteome biomarkers (Wu et al., 2021). Patients with lung cancer generally experience tumor recurrence and metastasis, leading to a comparatively poor OS rate (Zhou et al., 2020). Therefore, there is an urgent need to conduct more in-depth research on the identification of novel diagnostic or prognostic markers and potential drug targets to promote prognosis and personalized treatment. With the innovative development of gene chips and next-generation sequencing technology (NGS), gene signatures based on abnormal mRNA have shown the ability to predict the OS outcomes for malignant tumors (Njoku et al., 2020; Tan et al., 2020). Utilizing genomics and/or transcriptome analysis of neoplasm biopsy samples to infer disease severity is relatively fast and inexpensive. Doctors can use NGS to analyze multiple genes associated with an increased cancer risk at one time (Judkins et al., 2015; Fazio et al., 2020). According to NGS, doctors can accurately obtain the genetic variation information of patients, select potential targeted drugs for patients, evaluate the prognosis of patients, design drug-resistance treatment regimens, and realize individualized treatment (Van Allen et al., 2014; Hagemann et al., 2015; Forschner et al., 2021; Grobbel et al., 2021). In recent years, ferroptosis has attracted much attention because it plays a significant role in the occurrence, development, and multidrug resistance of tumors (Liang et al., 2019; Xia et al., 2019). Convincing evidence suggests that ferroptosis can inhibit tumorigenesis (Mou et al., 2019). Moreover, the genes associated with ferroptosis can be used as candidate biomarkers for tumor therapy (Zhuo et al., 2020). Hence, based on RNA sequencing data from TCGA and GEO, we evaluated the relationship between the expression profile of ferroptosis-related genes and the prognosis of patients with NSCLC. In this study, more than two-thirds of ferroptosis-related genes were differentially expressed between tumor and adjacent normal tissues, indicating that there is an association between ferroptosis and NSCLC. Four prognosis-associated ferroptosis-related genes (FANCD2, ACSL3, GCLC, and CISD1) were identified using univariate Cox regression analysis and a lasso Cox regression analysis. Our study indicated that the OS outcome was worse for patients with high risk score (p < 0.05) in Kaplan-Meier analysis. Analyses such as survival status distribution and PCA showed that the prognostic multigene signature notably divided high- and low-risk patients into two categories. In brief, a prognostic multigene signature could not only be used for risk assessment and the identification of high-risk patients but also to ameliorate nursing care by changing treatment schemes (Judkins et al., 2015), promote individualized treatment, and improve cancer treatment efficiency.
These four prognosis-associated ferroptosis-related genes were up-regulated in NSCLC cancerous tissues and associated with poor prognosis, which are also different from the findings of Han et al. (Lai et al., 2019). Our results further broadened the prognosis-associated ferroptosis-related gene signatures for NSCLC. FANCD2 overexpression increases the risk of metastasis in esophageal squamous cell carcinoma (ESCC) by activating DNA replication and regulating cell cycle progression, which is associated with the negative prognosis of ESCC (Lei et al., 2020). Bone marrow stromal cells (BMSCs) lacking FANCD2 exhibit iron overload and lipid peroxidation with erastin (inducing ferroptosis to selectively kill NSCLC cells) (Song et al., 2016; Gai et al., 2020). Monounsaturated fatty acids activated by ACSL3 can protect cells against ferroptosis (Magtanong et al., 2019). High levels of ACSL3 are frequently positively associated with poor clinical outcomes in patients with advanced NSCLC (Fernández et al., 2020). High mRNA expression of GCLC in tumor tissue significantly shortens the postoperative recurrence survival period of patients, and potentially can predict cisplatin resistance in patients with lung adenocarcinoma (Hiyama et al., 2018). GCLC promotes the ferroptosis-resistant state of NSCLC cells by preserving the glutamate balance (Kang et al., 2021). CISD1, also known as mitoNEET, is instrumental for the proliferation, migration, and invasion of tumor cells and accelerates the occurrence of malignant tumors (Mittler et al., 2019). Genetic inhibition of CISD1 induces iron-mediated lipid peroxidation in the mitochondria, which intensifies the strength of erastin-induced ferroptosis (Yuan et al., 2016). Unfortunately, whether CISD1 has a marked effect on the prognosis of malignant tumors has not been reported. Based on these results, we speculated whether the high-risk ferroptosis-related genes could be genetically suppressed to induce NSCLC cell death. We went a step further by investigating the lead compound with potential therapeutic effects in NSCLC.
At present, the research and development (R&D) costs of new molecular entity drugs have increased significantly, and the R&D cycle appears to be lengthy (Yildirim et al., 2016). Drug repurposing, that is, a novel drug development strategy to discover new drug indications based on drugs already on the market or in the clinical research stage, can mitigate a variety of costs including time, manpower, and material resources (Parvathaneni et al., 2019). Application of the CMap database can reveal drug–gene associations and identify possible therapeutic effects of compounds (Shi et al., 2016; Li H. et al., 2020). Based on gene chip technology and the CMap database for drug repositioning, we identified the potential candidate lead compound FFA to induce ferroptosis in NSCLC cells and cure this disease. We then used biological techniques to investigate whether FFA plays an important role in inhibiting the growth and metastasis of NSCLC. As expected, the results of CCK-8 and scratch experiments found that FFA dramatically induced cell death and suppressed proliferation in A549 cells. The small-molecule lead compound FFA is thus expected to be an effective drug for the treatment of NSCLC.
Ferroptosis is a novel mode of regulated cell death mediated by the iron-dependent accumulation of lipid peroxides and lipid reactive oxygen species (ROS). Tumor suppression can be mediated by iron inducers in various experimental cancer models, underscoring the potential of ferroptosis inducers as a new anticancer therapy (Hassannia et al., 2019; Yu et al., 2019; Badgley et al., 2020; Liao et al., 2020). Regulation of the classic ferroptosis-repressed GSH-dependent GPX4 signaling pathway is the dominating mechanism for causing ferroptosis in NSCLC (Shui et al., 2021; Zou et al., 2021). GSH was suggested to be a pivotal factor in maintaining GPX4 activity (Yan et al., 2021). The function of GPX4 is to prevent cells from amassing lipid hydroperoxides and avoid cellular ferroptosis. Studies clearly show that the inhibitor of GPX4 induces ferroptosis in not only cultured cancer cells but also tumor xenografts implanted in mice (Pu et al., 2020; Wang et al., 2020). In vitro experiments have shown that ferroptosis can be detected via multifarious methods involving the measurement of cell activity, GPX4, GSH, malondialdehyde, and ROS levels (Hu et al., 2020; Pu et al., 2020; Huang et al., 2022). Our study found that FFA decreased the levels of GSH and GPX4 in A549 cells, which was reversed by Fer-1, confirming that FFA caused ferroptosis in NSCLC cells. However, the A549 cell activity reduced by FFA could not completely reversed by Fer-1, suggesting that FFA suppresses tumor development partially by ferroptosis pathway in NSCLC cells.
Tumor-associated inflammation appears to be a new hallmark of cancer therapy (Li Z.-Y. et al., 2020). It has been widely noticed that FFA is an inhibitor of cyclooxygenase-2 (COX-2) (Pal et al., 2021). COX-2, an important inflammatory factor, is considered a key factor in tumorigenesis and might as a potential marker of poor prognosis in NSCLC (Castelao et al., 2003; Sandler and Dubinett, 2004; Liu et al., 2015). Inhibiting the excessive expression of COX-2 suppresses tumor growth and metastasis (Xu, 2002). Vainio H et al. also concluded that evidence for a cancer-preventive effect of COX-2 inhibitors has also been found in a variety of animal models (Vainio, 2001). Therefore, we speculated that the inhibitory effect of FFA on NSCLC may also be related to the improvement of COX-2-associated inflammation (Gridelli et al., 2002). Regrettably, the role of COX-2 in the treatment of NSCLC with FFA was not investigated in this study. In the future, further experiments will be performed to explore the relationship between COX-2 and FFA-suppressed NSCLC growth. In conclusion, these experimental results confirm the feasibility of targeting ferroptosis for the treatment of NSCLC and further verify that our screening strategy.
In summary, we analyzed and verified the ferroptosis-related genes for the prognosis of NSCLC, which can be used as a novel biomarker for targeting ferroptosis in the individualized treatment of NSCLC patients. Further, the potential lead compound FFA for the treatment of NSCLC were detected based on the above screening of high-risk ferroptosis-related genes. We also confirmed that FFA induced ferroptosis in A549 cells and inhibited growth and migration in a dose-dependent manner. Our findings also developed a new strategy for the antitumor drugs exploiting the ferroptosis process.
The original contributions presented in the study are included in the article/Supplementary Material, further inquiries can be directed to the corresponding authors.
XZ and LC mainly wrote and revised the manuscripts, and constructed the framework of the manuscript. YuZ and CG provided constructive opinions on the formation of the manuscript. LD, ZW, ZL, XS, and HX participated in the drawing of manuscript pictures and the investigation and sorting of documents. YL and YiZ participated in topic design, manuscript writing, manuscript editing and providing instructional support. All authors approved the final version of the article.
This work was supported by NSFC (81973378, 82073909), Natural Science Basic Research Plan in Shanxi Province of China (20210302124584, 202103021223233), and Postgraduate Innovation Project in Shanxi Province of China (2021Y408). This research project was supported by FSKSC and 1331KSC.
The authors declare that the research was conducted in the absence of any commercial or financial relationships that could be construed as a potential conflict of interest.
All claims expressed in this article are solely those of the authors and do not necessarily represent those of their affiliated organizations, or those of the publisher, the editors and the reviewers. Any product that may be evaluated in this article, or claim that may be made by its manufacturer, is not guaranteed or endorsed by the publisher.
The Supplementary Material for this article can be found online at: https://www.frontiersin.org/articles/10.3389/fmolb.2022.917602/full#supplementary-material
AUC, area under the curve; BMSCs, bone marrow stromal cells; CCK-8, cell counting kit-8; COX-2, cyclooxygenase-2; CMap database, connective map database; DEGs, differentially expressed genes; ESCC, esophageal squamous cell carcinoma; FFA, flufenamic acid; Fer-1, Ferrostatin-1; GPX4, glutathione peroxidase 4; GSH, Glutathione; HR, hazard ratio; NC, negative control group; NGS, next-generation sequencing technology; NSCLC, non-small cell lung cancer; ROC, receiver operating characteristic; R&D, research and development; ROS, reactive oxygen species; TCGA, the cancer genome atlas.
Alvarez, S. W., Sviderskiy, V. O., Terzi, E. M., Papagiannakopoulos, T., Moreira, A. L., Adams, S., et al. (2017). NFS1 Undergoes Positive Selection in Lung Tumours and Protects Cells from Ferroptosis. Nature 551 (7682), 639–643. doi:10.1038/nature24637
Badgley, M. A., Kremer, D. M., Maurer, H. C., DelGiorno, K. E., Lee, H.-J., Purohit, V., et al. (2020). Cysteine Depletion Induces Pancreatic Tumor Ferroptosis in Mice. Science 368 (6486), 85–89. doi:10.1126/science.aaw9872
Bersuker, K., Hendricks, J. M., Li, Z., Magtanong, L., Ford, B., Tang, P. H., et al. (2019). The CoQ Oxidoreductase FSP1 Acts Parallel to GPX4 to Inhibit Ferroptosis. Nature 575 (7784), 688–692. doi:10.1038/s41586-019-1705-2
Best, S. A., and Sutherland, K. D. (2018). "Keaping" a Lid on Lung Cancer: The Keap1-Nrf2 Pathway. Cell Cycle 17 (14), 1696–1707. doi:10.1080/15384101.2018.1496756
Castelao, J. E., Bart, R. D., DiPerna, C. A., Sievers, E. M., and Bremner, R. M. (2003). Lung Cancer and Cyclooxygenase-2. Ann. Thorac. Surg. 76 (4), 1327–1335. doi:10.1016/s0003-4975(03)00334-5
Fazio, M., Ablain, J., Chuan, Y., Langenau, D. M., and Zon, L. I. (2020). Zebrafish Patient Avatars in Cancer Biology and Precision Cancer Therapy. Nat. Rev. Cancer 20 (5), 263–273. doi:10.1038/s41568-020-0252-3
Fernández, L. P., Merino, M., Colmenarejo, G., Moreno-Rubio, J., Sánchez-Martínez, R., Quijada-Freire, A., et al. (2020). Metabolic Enzyme ACSL3 is a Prognostic Biomarker and Correlates with Anticancer Effectiveness of Statins in Non-Small Cell Lung Cancer. Mol. Oncol. 14 (12), 3135–3152. doi:10.1002/1878-0261.12816
Forschner, A., Sinnberg, T., Mroz, G., Schroeder, C., Reinert, C. P., Gatidis, S., et al. (2021). Case Report: Combined CDK4/6 and MEK Inhibition in Refractory CDKN2A and NRAS Mutant Melanoma. Front. Oncol. 11, 643156. doi:10.3389/fonc.2021.643156
Gadgeel, S., and Thakur, M. (2016). Predictive and Prognostic Biomarkers in Non-Small Cell Lung Cancer. Semin. Respir. Crit. Care Med. 37 (5), 760–770. doi:10.1055/s-0036-1592337
Gai, C., Liu, C., Wu, X., Yu, M., Zheng, J., Zhang, W., et al. (2020). MT1DP Loaded by Folate-Modified Liposomes Sensitizes Erastin-Induced Ferroptosis via Regulating miR-365a-3p/NRF2 axis in Non-Small Cell Lung Cancer Cells. Cell Death Dis. 11 (9), 751. doi:10.1038/s41419-020-02939-3
Gridelli, C., Maione, P., Airoma, G., and Rossi, A. (2002). Selective Cyclooxygenase-2 Inhibitors and Non-Small Cell Lung Cancer. Curr. Med. Chem. 9 (21), 1851–1858. doi:10.2174/0929867023368863
Grobbel, H.-P., Merker, M., Köhler, N., Andres, S., Hoffmann, H., Heyckendorf, J., et al. (2021). Design of Multidrug-Resistant Tuberculosis Treatment Regimens Based on DNA Sequencing. Clin. Infect. Dis. 73 (7), 1194–1202. doi:10.1093/cid/ciab359
Hagemann, I. S., Devarakonda, S., Lockwood, C. M., Spencer, D. H., Guebert, K., Bredemeyer, A. J., et al. (2015). Clinical Next-Generation Sequencing in Patients with Non-Small Cell Lung Cancer. Cancer 121 (4), 631–639. doi:10.1002/cncr.29089
Han, K., Wang, J., Qian, K., Zhao, T., Liu, X., and Zhang, Y. (2021). Construction of a Prognostic Model for Non-Small-Cell Lung Cancer Based on Ferroptosis-Related Genes. Biosci. Rep. 41 (5), BSR20210527. doi:10.1042/BSR20210527
Hassannia, B., Vandenabeele, P., and Vanden Berghe, T. (2019). Targeting Ferroptosis to Iron Out Cancer. Cancer Cell 35 (6), 830–849. doi:10.1016/j.ccell.2019.04.002
He, R., Liu, B., Xiong, R., Geng, B., Meng, H., Lin, W., et al. (2022). Itaconate Inhibits Ferroptosis of Macrophage via Nrf2 Pathways against Sepsis-Induced Acute Lung Injury. Cell Death Discov. 8 (1), 43. doi:10.1038/s41420-021-00807-3
Hiyama, N., Ando, T., Maemura, K., Sakatani, T., Amano, Y., Watanabe, K., et al. (2018). Glutamate-Cysteine Ligase Catalytic Subunit is Associated with Cisplatin Resistance in Lung Adenocarcinoma. Jpn. J. Clin. Oncol. 48 (4), 303–307. doi:10.1093/jjco/hyy013
Hu, J.-C., Zhu, T.-P., Gui, Y.-C., Tan, Z.-B., Wei, R.-Q., Hu, B.-L., et al. (2020). miR-28-5p Inhibits Carcinogenesis in Colon Cancer Cells and Is Necessary for Erastin-Induced Ferroptosis. Transl. Cancer Res. TCR 9 (4), 2931–2940. doi:10.21037/tcr-20-1809
Huang, J., Chen, G., Wang, J., Liu, S., and Su, J. (2022). Platycodin D Regulates High Glucose-Induced Ferroptosis of HK-2 Cells through Glutathione Peroxidase 4 (GPX4). Bioengineered 13 (3), 6627–6637. doi:10.1080/21655979.2022.2045834
Ji, X., Qian, J., Rahman, S. M. J., Siska, P. J., Zou, Y., Harris, B. K., et al. (2018). xCT (SLC7A11)-Mediated Metabolic Reprogramming Promotes Non-Small Cell Lung Cancer Progression. Oncogene 37 (36), 5007–5019. doi:10.1038/s41388-018-0307-z
Judkins, T., Leclair, B., Bowles, K., Gutin, N., Trost, J., McCulloch, J., et al. (2015). Development and Analytical Validation of a 25-Gene Next Generation Sequencing Panel that Includes the BRCA1 and BRCA2 Genes to Assess Hereditary Cancer Risk. BMC Cancer 15, 215. doi:10.1186/s12885-015-1224-y
Kang, R., Kroemer, G., and Tang, D. (2019). The Tumor Suppressor Protein P53 and the Ferroptosis Network. Free Radic. Biol. Med. 133, 162–168. doi:10.1016/j.freeradbiomed.2018.05.074
Kang, Y. P., Mockabee-Macias, A., Jiang, C., Falzone, A., Prieto-Farigua, N., Stone, E., et al. (2021). Non-Canonical Glutamate-Cysteine Ligase Activity Protects against Ferroptosis. Cell Metab. 33 (1), 174–189. doi:10.1016/j.cmet.2020.12.007
La Montagna, M., Ginn, L., and Garofalo, M. (2020). Mechanisms of Drug Resistance Mediated by Long Non-Coding RNAs in Non-Small-Cell Lung Cancer. Cancer Gene Ther. 28, 175–187. doi:10.1038/s41417-020-00214-3
Lai, Y., Zhang, Z., Li, J., Li, W., Huang, Z., Zhang, C., et al. (2019). STYK1/NOK Correlates with Ferroptosis in Non-small Cell Lung Carcinoma. Biochem. Biophys. Res. Commun. 519 (4), 659–666. doi:10.1016/j.bbrc.2019.09.032
Lei, L. C., Yu, V. Z., Ko, J. M. Y., Ning, L., and Lung, M. L. (2020). FANCD2 Confers a Malignant Phenotype in Esophageal Squamous Cell Carcinoma by Regulating Cell Cycle Progression. Cancers 12 (9), 2545. doi:10.3390/cancers12092545
Li, Y., Yan, H., Xu, X., Liu, H., Wu, C., and Zhao, L. (2020). Erastin/Sorafenib Induces Cisplatin-Resistant Non-Small Cell Lung Cancer Cell Ferroptosis through Inhibition of the Nrf2/xCT Pathway. Oncol. Lett. 19 (1), 323–333. doi:10.3892/ol.2019.11066
Li, Z.-Y., Yin, Y.-F., Guo, Y., Li, H., Xu, M.-Q., Liu, M., et al. (2020). Enhancing Anti-Tumor Activity of Sorafenib Mesoporous Silica Nanomatrix in Metastatic Breast Tumor and Hepatocellular Carcinoma via the Co-Administration with Flufenamic Acid. Int. J. Nanomedicine 15, 1809–1821. doi:10.2147/IJN.S240436
Li, H., Shi, X., Jiang, H., Kang, J., Yu, M., Li, Q., et al. (2020). CMap Analysis Identifies Atractyloside as a Potential Drug Candidate for Type 2 Diabetes Based on Integration of Metabolomics and Transcriptomics. J. Cell Mol. Med. 24 (13), 7417–7426. doi:10.1111/jcmm.15357
Liang, C., Zhang, X., Yang, M., and Dong, X. (2019). Recent Progress in Ferroptosis Inducers for Cancer Therapy. Adv. Mat. 31 (51), e1904197. doi:10.1002/adma.201904197
Liang, J.-Y., Wang, D.-S., Lin, H.-C., Chen, X.-X., Yang, H., Zheng, Y., et al. (2020). A Novel Ferroptosis-Related Gene Signature for Overall Survival Prediction in Patients with Hepatocellular Carcinoma. Int. J. Biol. Sci. 16 (13), 2430–2441. doi:10.7150/ijbs.45050
Liao, D., Yang, G., Yang, Y., Tang, X., Huang, H., Shao, J., et al. (2020). Identification of Pannexin 2 as a Novel Marker Correlating with Ferroptosis and Malignant Phenotypes of Prostate Cancer Cells. Onco. Targets Ther. 13, 4411–4421. doi:10.2147/OTT.S249752
Liu, R., Xu, K.-P., and Tan, G.-S. (2015). Cyclooxygenase-2 Inhibitors in Lung Cancer Treatment: Bench to Bed. Eur. J. Pharmacol. 769, 127–133. doi:10.1016/j.ejphar.2015.11.007
Liu, G., Pei, F., Yang, F., Li, L., Amin, A., Liu, S., et al. (2017). Role of Autophagy and Apoptosis in Non-Small-Cell Lung Cancer. Int. J. Mol. Sci. 18 (2), 367. doi:10.3390/ijms18020367
Magtanong, L., Ko, P.-J., To, M., Cao, J. Y., Forcina, G. C., Tarangelo, A., et al. (2019). Exogenous Monounsaturated Fatty Acids Promote a Ferroptosis-Resistant Cell State. Cell Chem. Biol. 26 (3), 420–432. doi:10.1016/j.chembiol.2018.11.016
Matsumoto, R., Tsuda, M., Yoshida, K., Tanino, M., Kimura, T., Nishihara, H., et al. (2016). Aldo-keto Reductase 1C1 Induced by Interleukin-1β Mediates the Invasive Potential and Drug Resistance of Metastatic Bladder Cancer Cells. Sci. Rep. 6, 34625. doi:10.1038/srep34625
Mittler, R., Darash-Yahana, M., Sohn, Y. S., Bai, F., Song, L., Cabantchik, I. Z., et al. (2019). NEET Proteins: A New Link between Iron Metabolism, Reactive Oxygen Species, and Cancer. Antioxidants Redox Signal. 30 (8), 1083–1095. doi:10.1089/ars.2018.7502
Mou, Y., Wang, J., Wu, J., He, D., Zhang, C., Duan, C., et al. (2019). Ferroptosis, a New Form of Cell Death: Opportunities and Challenges in Cancer. J. Hematol. Oncol. 12 (1), 34. doi:10.1186/s13045-019-0720-y
Njoku, K., Sutton, C. J. J., Whetton, A. D., and Crosbie, E. J. (2020). Metabolomic Biomarkers for Detection, Prognosis and Identifying Recurrence in Endometrial Cancer. Metabolites 10 (8), 314. doi:10.3390/metabo10080314
Pal, R., Kumar, A., and Misra, G. (2021). Exploring TEAD2 as a Drug Target for Therapeutic Intervention of Cancer: A Multi-Computational Case Study. Brief. Bioinform 22 (5), bbab007. doi:10.1093/bib/bbab007
Parvathaneni, V., Kulkarni, N. S., Muth, A., and Gupta, V. (2019). Drug Repurposing: A Promising Tool to Accelerate the Drug Discovery Process. Drug Discov. Today 24 (10), 2076–2085. doi:10.1016/j.drudis.2019.06.014
Pu, F., Chen, F., Zhang, Z., Shi, D., Zhong, B., Lv, X., et al. (2020). Ferroptosis as a Novel Form of Regulated Cell Death: Implications in the Pathogenesis, Oncometabolism and Treatment of Human Cancer. Genes Dis. 9 (2), 347–357. doi:10.1016/j.gendis.2020.11.019
Ritchie, M. E., Phipson, B., Wu, D., Hu, Y., Law, C. W., Shi, W., et al. (2015). Limma Powers Differential Expression Analyses for RNA-Sequencing and Microarray Studies. Nucleic Acids Res. 43 (7), e47. doi:10.1093/nar/gkv007
Sandler, A. B., and Dubinett, S. M. (2004). COX-2 Inhibition and Lung Cancer. Seminars Oncol. 31 (2 Suppl. 7), 45–52. doi:10.1053/j.seminoncol.2004.03.045
Shi, J., Jiang, S., Qiu, D., Le, W., Wang, X., Lu, Y., et al. (2016). Rapid Identification of Potential Drugs for Diabetic Nephropathy Using Whole-Genome Expression Profiles of Glomeruli. BioMed Res. Int. 2016, 1634730. doi:10.1155/2016/1634730
Shui, S., Zhao, Z., Wang, H., Conrad, M., and Liu, G. (2021). Non-Enzymatic Lipid Peroxidation Initiated by Photodynamic Therapy Drives a Distinct Ferroptosis-Like Cell Death Pathway. Redox Biol. 45, 102056. doi:10.1016/j.redox.2021.102056
Siegel, R. L., Miller, K. D., Fuchs, H. E., and Jemal, A. (2021). Cancer Statistics, 2021. CA A Cancer J. Clin. 71 (1), 7–33. doi:10.3322/caac.21654
Song, X., Xie, Y., Kang, R., Hou, W., Sun, X., Epperly, M. W., et al. (2016). FANCD2 Protects against Bone Marrow Injury from Ferroptosis. Biochem. Biophys. Res. Commun. 480 (3), 443–449. doi:10.1016/j.bbrc.2016.10.068
Stockwell, B. R., Friedmann Angeli, J. P., Bayir, H., Bush, A. I., Conrad, M., Dixon, S. J., et al. (2017). Ferroptosis: A Regulated Cell Death Nexus Linking Metabolism, Redox Biology, and Disease. Cell 171 (2), 273–285. doi:10.1016/j.cell.2017.09.021
Tan, Z., Lei, Y., Xu, J., Shi, S., Hua, J., Zhang, B., et al. (2020). The Value of a Metabolic Reprogramming-Related Gene Signature for Pancreatic Adenocarcinoma Prognosis Prediction. Aging 12 (23), 24228–24241. doi:10.18632/aging.104134
Vainio, H. (2001). Is COX-2 Inhibition a Panacea for Cancer Prevention? Int. J. Cancer 94 (5), 613–614. doi:10.1002/ijc.1518
Van Allen, E. M., Wagle, N., Stojanov, P., Perrin, D. L., Cibulskis, K., Marlow, S., et al. (2014). Whole-Exome Sequencing and Clinical Interpretation of Formalin-Fixed, Paraffin-Embedded Tumor Samples to Guide Precision Cancer Medicine. Nat. Med. 20 (6), 682–688. doi:10.1038/nm.3559
Wang, L., Chen, X., and Yan, C. (2020). Ferroptosis: An Emerging Therapeutic Opportunity for Cancer. Genes Dis. 9 (2), 334–346. doi:10.1016/j.gendis.2020.09.005
Wang, Y., Pan, Y., Wu, J., Luo, Y., Fang, Z., Xu, R., et al. (2022). A Novel Predictive Model Incorporating Ferroptosis-Related Gene Signatures for Overall Survival in Patients with Lung Adenocarcinoma. Med. Sci. Monit. 27, e934050. doi:10.12659/MSM.934050
Wu, W., Yu, X., Wu, J., Wu, T., Fan, Y., Chen, W., et al. (2021). Surface Plasmon Resonance Imaging-Based Biosensor for Multiplex and Ultrasensitive Detection of NSCLC-Associated Exosomal miRNAs Using DNA Programmed Heterostructure of Au-On-Ag. Biosens. Bioelectron. 175, 112835. doi:10.1016/j.bios.2020.112835
Xia, X., Fan, X., Zhao, M., and Zhu, P. (2019). The Relationship between Ferroptosis and Tumors: A Novel Landscape for Therapeutic Approach. Curr. Gene Ther. 19 (2), 117–124. doi:10.2174/1566523219666190628152137
Xu, X.-C. (2002). COX-2 Inhibitors in Cancer Treatment and Prevention, a Recent Development. Anti-Cancer Drugs 13 (2), 127–137. doi:10.1097/00001813-200202000-00003
Yan, H.-F., Zou, T., Tuo, Q.-Z., Xu, S., Li, H., Belaidi, A. A., et al. (2021). Ferroptosis: Mechanisms and Links with Diseases. Sig Transduct. Target Ther. 6 (1), 49. doi:10.1038/s41392-020-00428-9
Yang, W.-Z., Yu, W.-Y., Chen, T., Wang, X.-F., Dong, F., Xie, M.-E., et al. (2019). A Single-Cell Immunofluorescence Method for the Division Patterns Research of Mouse Bone Marrow-Derived Hematopoietic Stem Cells. Stem Cells Dev. 28 (14), 954–960. doi:10.1089/scd.2018.0239
Yildirim, O., Gottwald, M., Schüler, P., and Michel, M. C. (2016). Opportunities and Challenges for Drug Development: Public-Private Partnerships, Adaptive Designs and Big Data. Front. Pharmacol. 7, 461. doi:10.3389/fphar.2016.00461
Yu, M., Gai, C., Li, Z., Ding, D., Zheng, J., Zhang, W., et al. (2019). Targeted Exosome‐Encapsulated Erastin Induced Ferroptosis in Triple Negative Breast Cancer Cells. Cancer Sci. 110 (10), 3173–3182. doi:10.1111/cas.14181
Yuan, H., Li, X., Zhang, X., Kang, R., and Tang, D. (2016). CISD1 Inhibits Ferroptosis by Protection against Mitochondrial Lipid Peroxidation. Biochem. Biophys. Res. Commun. 478 (2), 838–844. doi:10.1016/j.bbrc.2016.08.034
Zhou, Y., Guo, D., and Zhang, Y. (2020). Association of MicroRNA-21 with P53 at Mutant Sites R175H and R248Q, Clinicopathological Features, and Prognosis of NSCLC. Mol. Ther. Oncolytics 19, 208–217. doi:10.1016/j.omto.2020.10.005
Zhuo, S., Chen, Z., Yang, Y., Zhang, J., Tang, J., and Yang, K. (2020). Clinical and Biological Significances of a Ferroptosis-Related Gene Signature in Glioma. Front. Oncol. 10, 590861. doi:10.3389/fonc.2020.590861
Keywords: ferroptosis, prognostic, non-small cell lung cancer, CMap database, antitumor drug
Citation: Zhao X, Cui L, Zhang Y, Guo C, Deng L, Wen Z, Lu Z, Shi X, Xing H, Liu Y and Zhang Y (2022) Screening for Potential Therapeutic Agents for Non-Small Cell Lung Cancer by Targeting Ferroptosis. Front. Mol. Biosci. 9:917602. doi: 10.3389/fmolb.2022.917602
Received: 11 April 2022; Accepted: 23 June 2022;
Published: 14 July 2022.
Edited by:
Yanqing Liu, Columbia University, United StatesReviewed by:
Yili Chen, Sun Yat-sen University, ChinaCopyright © 2022 Zhao, Cui, Zhang, Guo, Deng, Wen, Lu, Shi, Xing, Liu and Zhang. This is an open-access article distributed under the terms of the Creative Commons Attribution License (CC BY). The use, distribution or reproduction in other forums is permitted, provided the original author(s) and the copyright owner(s) are credited and that the original publication in this journal is cited, in accordance with accepted academic practice. No use, distribution or reproduction is permitted which does not comply with these terms.
*Correspondence: Yunfeng Liu, bmVjdGFybGl1QDE2My5jb20=; Yi Zhang, eWl6aGFuZ0BzeG11LmVkdS5jbg==, b3JjaWQub3JnLzAwMDAtMDAwMy0wMzA1LTMxMjc=
†These authors have contributed equally to this work
Disclaimer: All claims expressed in this article are solely those of the authors and do not necessarily represent those of their affiliated organizations, or those of the publisher, the editors and the reviewers. Any product that may be evaluated in this article or claim that may be made by its manufacturer is not guaranteed or endorsed by the publisher.
Research integrity at Frontiers
Learn more about the work of our research integrity team to safeguard the quality of each article we publish.