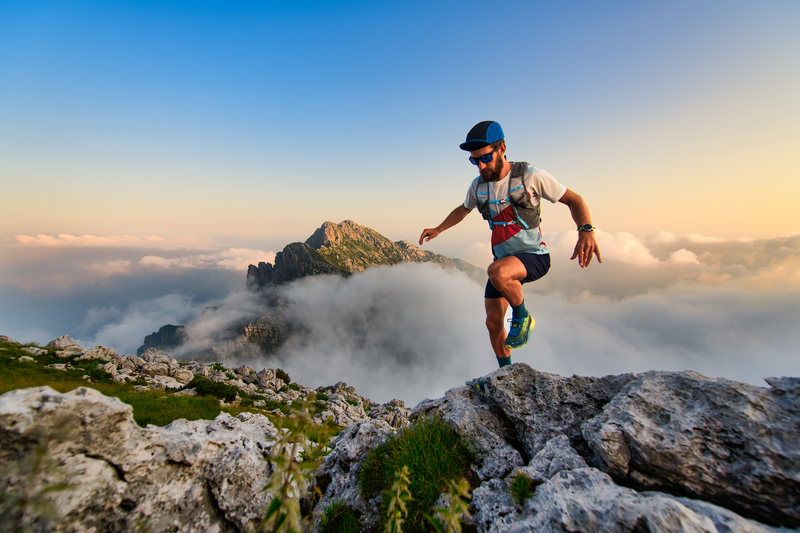
95% of researchers rate our articles as excellent or good
Learn more about the work of our research integrity team to safeguard the quality of each article we publish.
Find out more
ORIGINAL RESEARCH article
Front. Mol. Biosci. , 08 June 2022
Sec. Molecular Diagnostics and Therapeutics
Volume 9 - 2022 | https://doi.org/10.3389/fmolb.2022.875418
This article is part of the Research Topic The Upcoming Complications of COVID-19 on Recovered Patients: Molecular Mechanisms and Therapeutic Opportunities, volume II View all 5 articles
There are still frequent reports that a number of recovered coronavirus disease 2019 (COVID-19) patients following discharge have re-detectable positive (RP) results by RT-PCR. Understanding the clinical and molecular characteristics of RP patients may have implications for curbing the COVID-19 pandemic. In this study, 318 COVID-19 convalescent patients, including 59 RP patients and 259 non-RP (NRP) patients, were enrolled. Among RP patients, women accounted for a significantly high proportion (67.8%), and the titers of IgG and IgM antibodies in this group were also significantly high. Differentially expressed genes (DEGs), including 692 upregulated and 383 downregulated genes, overlapped in two public GEO datasets containing RP and NRP blood cell samples. Enrichment analysis indicated that these DEGs were related to several key signaling pathways, such as viral infection, immune activation, and inflammatory responses. Importantly, 59 indicator genes constituting the core network exhibited high diagnostic values and were correlated with markers of different immune cells. Among these, 12 drug-related genes were associated with the RP results. Our work suggests that, in addition to clinically available features, blood cell transcriptome sequencing can be performed to obtain gene signatures for diagnosis of RP patients.
The emerging acute respiratory disease, widely known as coronavirus disease 2019 (COVID-19), is a highly infectious pandemic caused by coronavirus SARS-CoV-2 infection, which has spread across the world (Wang et al., 2020). Patients infected with SARS-CoV-2 exhibit clinical symptoms including fever, dry cough, and fatigue (Guan et al., 2020; Miró et al., 2021). As of this writing, the pandemic has caused more than 200 million people to be infected, as well as four million deaths (https://covid19.who.int).
Currently, most COVID-19 studies concentrate on clinicopathological and epidemiological characteristics, as well as laboratory tests and efficacy results in infected patients (Chan et al., 2020; Lan et al., 2020; Pan et al., 2020; Wang et al., 2020), leading to a deeper understanding of patients with initial viral infection (Bradley et al., 2020; Liu et al., 2020; Song et al., 2020). Many other researchers have investigated the important roles of innate and adaptive immune responses in SARS-CoV-2 infection and the pathogenesis of COVID-19 (Paces et al., 2020; Zhang et al., 2021). It is believed that production of a large amount of inflammatory cytokines, such as interleukin (IL)-6, interferon (IFN)-γ, and tumor necrosis factor (TNF)-α, known as the “cytokine storm,” correlates with COVID-19 infection (Ragab et al., 2020). In addition, B cells recognize viral proteins and are activated to produce SARS-CoV-2-specific antibodies (Zhang et al., 2020). In addition, the humoral response to SARS-CoV-2 initiates the release of specific immunoglobulins G and M (IgG and IgM) (Wehbe et al., 2021).
One emerging problem is that there are several COVID-19 patients with re-detectable positive (RP) results after treatment (Zhou et al., 2020; Huang et al., 2021). Because of their potential infectivity, more attention should be given to RP patients (Tay et al., 2020). Judging from the strict hospital management of RP patients at that time, it was likely that they have a latent infection not detectable temporary from the tests. Previously, Gao et al. (2020). illustrated that the Nucleic acid results might be influenced by patients’ condition, which lead to temporarily undetectable by RT-PCR tests. For patients’ condition, it might be related to underlying diseases and treatment methods. Patients with underlying diseases, such as diabetes and hypertension, once infected with SARS-CoV-2, would have a further weakened in immune system, ultimately, who are more likely to relapse after discharge (Hussain et al., 2020). In addition, the COVID-19 patients were treated with antiviral drugs that clear SARS-CoV-2, whose immune function will be influenced. However, when the antiviral therapy was discontinued, the virus would be activated due to a decreased immune response (Balachandar et al., 2020; Wu et al., 2020). Due to these potential reasons, patients with RP results require longer isolation periods and more frequent nucleic acid tests, leading to a greater burden on hospitals and patients. Therefore, it is critical to explore the internal mechanisms and molecular features of patients with RP to identify sensitive and reliable indicators for RP patient diagnosis.
In this study, we focused on the risk associated with clinical characteristics, related signaling pathways, and indicator genes to understand the clinical and molecular features of patients with RP. We collected and analyzed the epidemiological and laboratory characteristics of 318 COVID-19 convalescent patients, including 59 RP patients. A systematic literature survey on the clinical features was conducted to comprehensively evaluate the application value of these clinical characteristics. We also analyzed two GEO datasets containing RP and NRP samples; differentially expressed genes (DEGs) were enriched in signaling pathways associated with viral infection, immunity, and inflammation in patients with RP. In addition, we performed correlation analysis and identified many high-standard diagnostic indicator genes in RP patients, which can be used as indicative biomarkers for the recurrence of positive SARS-CoV-2.
This study, conducted at the Wuhan Rehabilitation Station of Hubei University, enrolled 318 patients who were instructed to undergo isolation observation from 31 January to 26 March, 2020. Epidemiological information and laboratory test results were also obtained. A total of 318 patients were divided into two groups: 59 patients with RP and 259 patients with NRP. Written informed consent was obtained from all patients, and all methods were performed in accordance with relevant safety guidelines.
Categorical variables were presented as absolute and relative frequencies (%), and continuous variables were presented as median and interquartile range (IQR) and number (%). A univariate logistic regression model was used to compute odds ratio (OR) and p-values. Spearman’s correlation analysis was used to analyze the correlation between categorical and continuous variables. All statistical results were analyzed using IBM SPSS statistics version 23.0, and the results were plotted using GraphPad Prism version 7.0.
Two microarray datasets of patients with RP and NRP were obtained from the GEO database (https://www.ncbi.nlm.nih.gov/geo/). The GSE166253 expression profile contained six NRP samples and 10 RP samples derived from the University of Science and Technology of China. The GSE179627 expression profile included 11 NRP and 15 RP samples from the College of Bioinformatics in China. These samples were used for analysis of gene transcription of their peripheral blood mononuclear cells (PBMCs). Based on the expression levels of all genes in the samples, the correlation between samples were assessed by SangerBox (http://sangerbox.com/), and followed by Pearson correlation analysis. |Cor| ≥ 0.9 was clustered and |Cor| < 0.9 was excluded. Sample GSM5425261 is abnormal and has relatively low correlation with others (|Cor| < 0.9) (Supplementary Figure S1). So, it was removed from the database.
The adjusted p-value < 0.05 and |logFC| > 0.5 were used to define DEGs identified by the limma package in R language (version 4.0.3), which classified expression differences between patients with RP and NRP by analyzing raw data from public GEO databases. DEGs were then visualized by volcanic map. The overlapping genes between GSE166253 and GSE179627 were analyzed using Venn diagram on the website (http://bioinformatics.psb.ugent.be/webtools/Venn/).
The functions of the DEGs were ascertained by Gene Ontology (GO) annotation and Kyoto Encyclopedia of Genes and Genomes (KEGG) pathway analysis using the Estimate package in R (version 4.0.3). Significant pathway terms (p < 0.05) in GO and KEGG were enriched, and the top 10 enrichment terms related to viral infection, immunity, and inflammation were presented with bubble diagrams using Sangerbox (http://www.sangerbox.com/Tool).
Spearman correlation analysis was used to analyze the correlation between the RP results and the level of DEGs. In two RP-related GEO datasets, we performed WGCNA analysis with high correlation and low node count (power = 1, weight = 0.75) to obtain a co-expression network with highly accurate diagnostic indicator genes. Overlapped indicator genes were selected, and their networks were drawn using Cytoscape (http://www.cytoscape.org/; v3.7.1).
The relations between indicator genes and immune markers were examined using Pearson correlation analysis via the R package. In Scatter diagram, |Cor| ≥ 0.4 and p < 0.05, which were consistent in both two datasets, were considered statistically significant.
Drug-gene interactions were analyzed using the drug-gene interaction database (DGIdb, https://dgidb.org/).
We followed 318 COVID-19 convalescent patients and analyzed their demographic and clinical characteristics. Fifty-nine patients tested positive again (RP rate, 18.6%), and their general features are shown in Table 1. A lower number of men (32.2%) turned RP than women (67.8%). Univariate logistic regression analysis was employed to identify the risk factors associated with RP results. OR with a confidence interval (CI) of general features and laboratory tests was obtained, and the results are shown as forest plots (Figure 1). Consequently, the logistic regression indicated that sex and IgG and IgM titers influenced the risk of RP. Women had a higher risk of having an RP result than men (OR 1.934, 95% CI: 1.063–3.571, p = 0.031). The inflammatory markers CRP (OR 1.500, 95% CI: 0.447–5.038, p = 0.512) and ESR (OR 0.905, 95% CI: 0.189–4.340, p = 0.900) were not statistically different between NRP and RP groups. Recovered COVID-19 patients who had a higher titer of IgG and IgM in their isolation period after discharge had a higher risk of RP (OR 1.068, 95% CI: 1.034–1.102, p = 0.000; OR 1.055, 95% CI: 1.006–1.105, p = 0.026, respectively).
FIGURE 1. Forest plot of the OR, 95% CI of OR, and P value of the general features (A) and laboratory test (B) included in the model.
There were no substantial differences in other general features or laboratory findings between the RP and NRP patients. In addition, for comprehensive comparison and analysis, clinical characteristics of patients with RP reported in previous studies are listed in Table 2, including age, LYM count, IL-6, AST, and D-dimer. Unexpectedly, most of the risk factors listed in the table are inconsistent with our results.
Next, we conducted a more specific analysis at the gene level to identify more accurate signatures for patients with RP. DEGs from the two datasets were screened using filter criteria. In the GSE166253 dataset, 3,485 upregulated and 3,083 downregulated mRNAs were identified in RP patients compared to NRP patients (Figure 2A). Similarly, in the GSE179627 dataset, 3,873 upregulated mRNAs and 3,479 downregulated mRNAs were identified (Figure 2B). Moreover, we found 1,075 overlapping DEGs within the two datasets, including 692 upregulated and 383 downregulated genes, as shown in the Venn diagram (Figures 2C,D).
FIGURE 2. Characteristics of differentially expressed genes (DEGs) between RP and NRP samples. (A,B) Volcano plots of mRNAs in two datasets (GSE166253 and GSE179627) respectively. The red and green dots indicate the up-regulated and down-regulated differentially mRNAs, respectively. 692 upregulated genes (C) and 383 downregulated genes (D) were showed in the overlapping Venn diagram. The blue circle indicates GSE166253, and the red circle indicates GSE179627.
To obtain key information about the biological function of the 1,075 overlapping DEGs between RP and NRP patients, we performed GO and KEGG enrichment analyses. The results of GO annotation analysis indicated that DEGs were significantly enriched in terms of regulation of innate immune response, regulation of NF-κB signaling, antigen processing, and presentation of peptide antigen in the BP subgroup (Figure 3A). In addition, the most significant KEGG pathways were enriched for overlapping DEGs in COVID-19 patients, including human immunodeficiency virus 1 infection, apoptosis, the mTOR signaling pathway and TNF signaling pathway (Figure 3B). In addition, to detect the level of inflammation in RP patients, we analyzed the inflammatory markers TNF and IFNG which were considered to mediate the development of inflammation (Han et al., 2020; Montalvo Villalba et al., 2020). We found that compared with the NRP groups, the expression levels of TNF and IFNG in the RP groups were higher (Supplementary Figure S2). These DEGs are closely related to the antiviral immune response and inflammation-related pathways, suggesting that these DEGs may have useful clinical applications in the diagnosis and prevention of COVID-19.
FIGURE 3. Establishment of database core network. (A) GO enrichment analysis of the overlapping DEGs in biological process (BP) subgroup and the genes enriched on the pathway. (B) KEGG enrichment analysis of the overlapping DEGs the genes enriched on the pathway. (C,D) 59 genes with high correlation coefficient in the two RP-related GEO datasets is visualized in Cytoscape.
To obtain a co-expression network with more and highly accurate diagnostic indicator genes, we performed WGCNA analysis with parameter settings of high correlation and low node count. In the two RP-related GEO datasets, 59 nodes with high correlation coefficients overlapped, and their networks were drawn using Cytoscape (Figures 3C,D). In the network diagram, 23 upregulated genes are represented by red circles, whereas 36 downregulated genes are represented in green. The expression of several key genes is shown in Figure 4, and their correlation with RP results, calculated by univariate logistic regression analysis, is shown in Table 3, which indicates that most of these genes significantly influenced the risk of RP results (p < 0.05). Therefore, these DEGs could be used as indicator genes in patients with RP.
FIGURE 4. The expression of some indicator genes in the two datasets. (A) The down-regulated expression of ARID5B, KMT2C, KMT2E, LAMB1, TBL1XR1 and TFPI. (B) The up-regulated expression of CDKN2D, PGP, KCNQ1, FCGRT, CNPY3 and DHRS4.
TABLE 3. Correlation analysis between COVID-19 patients with or without RP results and indicator genes.
RP patients are usually associated with low autoimmunity; thus, we investigated the correlation between indicator genes and markers of different immune cells. These indicator genes were significantly correlated with hallmarks of immune cells, including CD8B in CD8 + T cells, CD3E in T cells (general), CD9 in B cells, and KIR2DL4 in natural killer cells (Figures 5, 6).
FIGURE 5. Correlation analysis between (A) FCGRT, (B) TFPI, (C) DGCRL6, (D) DHRS4, (E) KLF16 and different immune cell markers in GSE166253.
FIGURE 6. Correlation analysis between (A) FCGRT, (B) TFPI, (C) DGCRL6, (D) DHRS4, (E) KLF16 and different immune cell markers in GSE179627.
Because COVID-19 patients are usually clinically treated with a variety of different drugs, the influence of drugs on these RP indicator genes should be ruled out. The DGIdb website was used to explore drug-gene interactions. Among the 59 highly accurate indicator genes, 12 were targeted by several corresponding interacting drugs, including KCNQ1, TFPI, KMT2C, PGP, ARID5B, LAMB1, TBL1XR1, CDKN2D, KMT2E, FCGRT, PIKFYVE, and DHX36 (Table 4).
In this study, we updated our understanding of COVID-19 RP regarding three parameters: clinical characteristics, signaling pathways, and gene signatures (Figure 7). We collected samples from COVID-19 convalescent patients and analyzed their corresponding clinical features. There were significant differences in sex and IgG and IgM titers between RP and NRP patients. To identify more accurate molecular indicators, we selected two public microarray datasets from China, GSE166253 and GSE179627, and 1,075 overlapping DEGs were screened between the NRP and RP groups. Using GO and KEGG enrichment analyses, we found that the biological functions of the overlapping DEGs were closely related to viral infection, immunity, and inflammation. The mRNA expression levels of 59 indicator genes with diagnostic values were highly correlated with the RP results, and 12 indicator genes, including ARID5B, CDKN2D, KCNQ1, KMT2C, KMT2E, LAMB1, PGP, TBL1XR1, TFPI, FCGRT, PIKFYVE, and DHX36 have corresponding drug interactions. Furthermore, these indicator genes were substantially associated with gene markers of immune cells.
Among all clinical patients from whom samples were collected, 18.6% showed RP results in convalescent patients, similar to results in many previous studies (Zhu et al., 2020; Liu et al., 2021), suggesting that the RP result is a universal phenomenon. Using risk analysis, we found that the RP rate of women was higher than that of men, which is consistent with other studies (Liu et al., 2021; Wu et al., 2021). One possible reason might be that sex hormones act as important modulators of immune responses, causing different reactions to viral reinfection (Roved et al., 2017). Sex steroids, namely estrogens, progesterone, and androgens, are effective immune-modulators. The different concentrations of sex steroids between male and female are likely to influence COVID-19 immune activation and inflammatory responses (Mauvais-Jarvis et al., 2020). Moreover, previous studies illuminated that in COVID-19, testosterone contributed to inhibition of pro-inflammatory cytokines, augmentation of anti-inflammatory cytokines, thereby modulating the immune response (Pozzilli and Lenzi, 2020; Al-Kuraishy et al., 2021). In addition, our results indicated that the titers of IgG and IgM were higher in the RP groups, which may be triggered by the remaining viral RNA (Liu et al., 2021). Several other clinical risk factors associated with COVID-19 RP have been previously documented, such as age, LYM count, IL-6, AST, and D-dimer, which were not confirmed in our study. Table 2 compare the risk factors of the clinical characteristics between RP and NRP patients. Surprisingly, in previous studies, results regarding these risk factors were conflicting. For example, Liu et al. (2021). indicated that men had a lower risk than women of having a re-positive test result (p < 0.05), and it was confirmed in our study. However, Liu et al. (2021). analyzed that gender was no significantly different (p > 0.05) (Luo et al., 2021). The possible reasons for these contradictory results are that collection of clinical information was various. Also, several studies were based on a rather small cohort from a single medical center. Together, these results suggest that COVID-19 RP is likely to be a complex clinical event, and more accurate and reliable molecular indicators are urgently required for diagnosis.
To determine the molecular characteristics predicting RP patients, we compared two gene expression profile databases of peripheral blood cells from patients with RP and NRP. Using GO and KEGG pathway enrichment analyses, we found that 1,075 overlapping DEGs were related mainly to antigen processing, immune modulation, and inflammatory reactions, such as Notch, NF-кB, and mTOR signaling pathways. The NF-кB signaling pathway stimulates pro-inflammatory cytokines and chemokines, which play an important role in regulating the immune response (Kircheis et al., 2020; Montalvo Villalba et al., 2020). The Notch signaling pathway acts as a key regulator of innate immune and inflammatory responses, and it is associated with inflammatory conditions (Shang et al., 2016). The effector responses of innate immune cells can be controlled and shaped by the mTOR signaling pathway, which regulates cytokine responses and antigen presentation (Weichhart et al., 2015). Other pathways are also involved in the regulation of inflammatory cytokine responses, antigen presentation, and cellular metabolism. As these DEGs were mostly related to biological processes associated with the clinical consensus of COVID-19, we believe that they have potential and promising clinical value for the prediction of RP patients.
Because the risk factors reported in recent literature are inconsistent with each other, we conducted a more specific analysis at the gene level to discover more accurate and reliable signatures for RP patients. We found that 59 indicator genes had high correlation coefficients in patients with RP. In addition, most of these genes influenced the risk of an RP result and overlapped in the two RP-related GEO datasets; among which, 12 genes were targeted by several drugs and significantly correlated with the hallmarks of immune cells. One remarkable gene PIKFYVE was identified in PBMCs, but it could also play a essential role in preventing excessive lung inflammation through regulating alveolar macrophage function (Kawasaki et al., 2017). In addition, apilimod, the PIKFYVE inhibitor, has been found to antagonize replication of SARS-CoV-2 in iPSC-derived pneumocyte-like cells (Riva et al., 2020). Therefore, PIKFYVE might exhibit antiviral in the infected lung cells. It is possible that false negatives may be detected in COVID-19 patients who have other underlying diseases after using medicines corresponding to these genes. Although certain genes have not been previously reported to be associated with COVID-19 RP, they have been implicated in viral infections, inflammation, and immunity. For example, tissue factor pathway inhibitor (TFPI), which modulates a key anticoagulant pathway, can be impaired during inflammation (Levi and van der Poll, 2005). KMT2C, widely known as MLL3, activates the NF-κB pathway, inhibits inflammatory diseases, and regulates the secretion of inflammatory cytokines (Fan et al., 2021). KMT2E is a gene related to lysine degradation in mono-CD14+ cells and has been shown to be downregulated in severe COVID-19 patients (Qi et al., 2021).
Several factors influence COVID-19 RP, making it difficult to clarify the underlying mechanism. In this study, we identified relevant characteristics of RP based on clinical features, signaling pathways, and molecular biomarkers. However, due to the limited number of patient samples, some confounding factors, such as virus residue, sensitivity of test reagents, and sampling methods, were not included in the study. Although there were 1,075 overlapping DEGs between RP and NRP samples in the two GEO datasets, their clustering patterns were not the same. Generally, each cluster classification represents a pathway, or even a clinical feature, and it may not be feasible to locate consistent diagnostic features in different databases from the perspective of clinical features and signal pathways. Furthermore, there are many candidate molecular diagnostic genes that have the advantage of early detection and sensitivity, but the impact of other factors, such as drug treatment and underlying diseases, on these diagnostic indicators should be excluded.
In the present study, we showed that sex and IgG and IgM titers in blood were the most distinguishing factors between RP and NRP patients. In addition, DEGs were mainly enriched in antigen recognition, inflammatory modulation, and immune responses. Co-expression networks from two mutually verified RP datasets were constructed; among which, 59 overlapping indicator genes were highly correlated with RP results. Therefore, in addition to long-term isolation and multiple nucleic acid tests, transcriptional sequencing to obtain the signatures of these indicator genes is a complementary strategy for the early and reliable diagnosis of RP patients. However, a limitation of the present study is that we did not measure infection titers in RP patients, which prevents us from evaluating the production of infectious virions. Consequently, it was impossible to confirm that whether RP patients had actively replicating virus infection.
The original contributions presented in the study are included in the article/Supplementary Material, further inquiries can be directed to the corresponding authors.
YP and SW performed the data analysis and aided in writing the manuscript. YC and BZ did sample test. NL, QT, RC, and YL participated in the statistical analysis, and improved the manuscript. KZ and SX assisted in editing the manuscript. WH, SW and XW designed the study, provided financial support, and reviewed the manuscript. All authors read and approved the final manuscript.
This research was funded by the National Natural Science Foundation of China (No. 82073937), Natural Science Foundation of Guangdong Province (No. 2018A030313122), Shenzhen Science and Technology Project (Nos. JCYJ20210324093602007, GJHZ20200731095200003, JCYJ20180305163658916, and JCYJ20180228175059744), Shenzhen Key Medical Discipline Construction Fund (No. SZXK059), and Shenzhen Key Laboratory (No. ZDSYS20200811142804014).
The authors declare that the research was conducted in the absence of any commercial or financial relationships that could be construed as a potential conflict of interest.
All claims expressed in this article are solely those of the authors and do not necessarily represent those of their affiliated organizations, or those of the publisher, the editors and the reviewers. Any product that may be evaluated in this article, or claim that may be made by its manufacturer, is not guaranteed or endorsed by the publisher.
We would like to thank Editage (www.editage.cn) for English language editing.
The Supplementary Material for this article can be found online at: https://www.frontiersin.org/articles/10.3389/fmolb.2022.875418/full#supplementary-material
Al-Kuraishy, H. M., Al-Gareeb, A. I., Faidah, H., Alexiou, A., and Batiha, G. E.-S. (2021). Testosterone in COVID-19: An Adversary Bane or Comrade Boon. Front. Cell. Infect. Microbiol. 11, 666987. doi:10.3389/fcimb.2021.666987
Balachandar, V., Mahalaxmi, I., Subramaniam, M., Kaavya, J., Senthil Kumar, N., Laldinmawii, G., et al. (2020). Follow-up Studies in COVID-19 Recovered Patients - Is it Mandatory? Sci. Total Environ. 729, 139021. doi:10.1016/j.scitotenv.2020.139021
Bradley, B. T., Maioli, H., Johnston, R., Chaudhry, I., Fink, S. L., Xu, H., et al. (2020). Histopathology and Ultrastructural Findings of Fatal COVID-19 Infections in Washington State: a Case Series. Lancet 396 (10247), 320–332. doi:10.1016/S0140-6736(20)31305-2
Chan, J. F.-W., Yuan, S., Kok, K.-H., To, K. K.-W., Chu, H., Yang, J., et al. (2020). A Familial Cluster of Pneumonia Associated with the 2019 Novel Coronavirus Indicating Person-To-Person Transmission: a Study of a Family Cluster. Lancet 395 (10223), 514–523. doi:10.1016/S0140-6736(20)30154-9
Dong, Y., Huang, H., Yang, J., and Yang, L. (2021). Retrospective Analysis on the Clinical Characteristics of Patients Who Were Reinfected with the Corona Virus in 2019. Am. J. Transl. Res. 13 (5), 5505–5511. https://www.ncbi.nlm.nih.gov/pmc/articles/PMC8205718/.
Fan, W., Xu, Z., Liang, S., Zuo, S., Bian, C., Gao, X., et al. (2021). MLL3 Inhibits Apoptosis of Rheumatoid Arthritis Fibroblast-like Synoviocytes and Promotes Secretion of Inflammatory Factors by Activating CCL2 and the NF-Κb Pathway. Inflammation 44 (5), 1803–1814. doi:10.1007/s10753-021-01459-2
Gao, Z., Xu, Y., Guo, Y., Xu, D., Zhang, L., Wang, X., et al. (2020). A Systematic Review of Re-detectable Positive Virus Nucleic Acid Among COVID-19 Patients in Recovery Phase. Infect. Genet. Evol. 85, 104494. doi:10.1016/j.meegid.2020.104494
Guan, W.-J., Ni, Z.-Y., Hu, Y., Liang, W.-H., Ou, C.-Q., He, J.-X., et al. (2020). Clinical Characteristics of Coronavirus Disease 2019 in China. N. Engl. J. Med. 382 (18), 1708–1720. doi:10.1056/NEJMoa2002032
Han, X., Liu, X., Wang, X., Guo, W., Wen, Y., Meng, W., et al. (2020). TNF‐α‐dependent Lung Inflammation Upregulates Superoxide Dismutase‐2 to Promote Tumor Cell Proliferation in Lung Adenocarcinoma. Mol. Carcinog. 59 (9), 1088–1099. doi:10.1002/mc.23239
Hong, L.-X., Liu, L., Lin, A., and Yan, W.-H. (2021). Risk Factors for SARS-CoV-2 Re-positivity in COVID-19 Patients after Discharge. Int. Immunopharmacol. 95, 107579. doi:10.1016/j.intimp.2021.107579
Huang, J., Zheng, L., Li, Z., Hao, S., Ye, F., Chen, J., et al. (2020). Kinetics of SARS-CoV-2 Positivity of Infected and Recovered Patients from a Single Center. Sci. Rep. 10 (1), 18629. doi:10.1038/s41598-020-75629-x
Huang, K., Liu, W., Zhou, J., Wang, Y., Zhang, Y., Tang, X., et al. (2021). Repositive RT-PCR Test in Discharged COVID-19 Patients during Medical Isolation Observation. Int. J. Med. Sci. 18 (12), 2545–2550. doi:10.7150/ijms.58766
Hussain, A., Bhowmik, B., and do Vale Moreira, N. C. (2020). COVID-19 and Diabetes: Knowledge in Progress. Diabetes Res. Clin. Pract. 162, 108142. doi:10.1016/j.diabres.2020.108142
Kawasaki, T., Ito, K., Miyata, H., Akira, S., and Kawai, T. (2017). Deletion of PIK Fyve Alters Alveolar Macrophage Populations and Exacerbates Allergic Inflammation in Mice. Embo J. 36 (12), 1707–1718. doi:10.15252/embj.201695528
Kircheis, R., Haasbach, E., Lueftenegger, D., Heyken, W. T., Ocker, M., and Planz, O. (2020). NF-κB Pathway as a Potential Target for Treatment of Critical Stage COVID-19 Patients. Front. Immunol. 11, 598444. doi:10.3389/fimmu.2020.598444
Lan, L., Xu, D., Ye, G., Xia, C., Wang, S., Li, Y., et al. (2020). Positive RT-PCR Test Results in Patients Recovered from COVID-19. JAMA 323 (15), 1502–1503. doi:10.1001/jama.2020.2783
Levi, M., and van der Poll, T. (2005). Two-Way Interactions between Inflammation and Coagulation. Trends Cardiovasc. Med. 15 (7), 254–259. doi:10.1016/j.tcm.2005.07.004
Liu, H.-Q., Yuan, B., An, Y.-W., Chen, K.-J., Hu, Q., Hu, X.-P., et al. (2021). Clinical Characteristics and Follow-Up Analysis of 324 Discharged COVID-19 Patients in Shenzhen during the Recovery Period. Int. J. Med. Sci. 18 (2), 347–355. doi:10.7150/ijms.50873
Liu, Y., Liu, L., Wang, Y., Du, X., Ma, H., and Yao, J. (2020). Clinical Course and Characteristics of Patients with Coronavirus Disease 2019 in Wuhan, China: a Single-Centered, Retrospective, Observational Study. Aging 12 (16), 15946–15953. doi:10.18632/aging.103745
Lu, J., Peng, J., Xiong, Q., Liu, Z., Lin, H., Tan, X., et al. (2020). Clinical, Immunological and Virological Characterization of COVID-19 Patients that Test Re-positive for SARS-CoV-2 by RT-PCR. EBioMedicine 59, 102960. doi:10.1016/j.ebiom.2020.102960
Luo, L., Liu, D., Zhang, Z., Li, Z., Xie, C., Wang, Z., et al. (2021). Probable Causes and Risk Factors for Positive SARS-CoV-2 Testing in Recovered Patients: Evidence from Guangzhou, China. Front. Med. 8, 684101. doi:10.3389/fmed.2021.684101
Mauvais-Jarvis, F., Klein, S. L., and Levin, E. R. (2020). Estradiol, Progesterone, Immunomodulation, and COVID-19 Outcomes. Endocrinology 161 (9), bqaa127. doi:10.1210/endocr/bqaa127
Miró, Ò., Llorens, P., Jiménez, S., Piñera, P., Burillo-Putze, G., Martín, A., et al. (2021). Frequency, Risk Factors, Clinical Characteristics, and Outcomes of Spontaneous Pneumothorax in Patients with Coronavirus Disease 2019: A Case-Control, Emergency Medicine-Based Multicenter Study. Chest 159 (3), 1241–1255. doi:10.1016/j.chest.2020.11.013
Montalvo Villalba, M. C., Valdés Ramírez, O., Muné Jiménez, M., Arencibia Garcia, A., Martinez Alfonso, J., González Baéz, G., et al. (2020). Interferon Gamma, TGF-Β1 and RANTES Expression in Upper Airway Samples from SARS-CoV-2 Infected Patients. Clin. Immunol. 220, 108576. doi:10.1016/j.clim.2020.108576
Paces, J., Strizova, Z., Smrz, D., and Cerny, J. (2020). COVID-19 and the Immune System. Physiol. Res. 69 (3), 379–388. doi:10.33549/physiolres.934492
Pan, F., Ye, T., Sun, P., Gui, S., Liang, B., Li, L., et al. (2020). Time Course of Lung Changes at Chest CT during Recovery from Coronavirus Disease 2019 (COVID-19). Radiology 295 (3), 715–721. doi:10.1148/radiol.2020200370
Pozzilli, P., and Lenzi, A. (2020). Commentary: Testosterone, a Key Hormone in the Context of COVID-19 Pandemic. Metabolism 108, 154252. doi:10.1016/j.metabol.2020.154252
Qi, F., Zhang, W., Huang, J., Fu, L., and Zhao, J. (2021). Single-Cell RNA Sequencing Analysis of the Immunometabolic Rewiring and Immunopathogenesis of Coronavirus Disease 2019. Front. Immunol. 12, 651656. doi:10.3389/fimmu.2021.651656
Ragab, D., Salah Eldin, H., Taeimah, M., Khattab, R., and Salem, R. (2020). The COVID-19 Cytokine Storm; what We Know So Far. Front. Immunol. 11, 1446. doi:10.3389/fimmu.2020.01446
Riva, L., Yuan, S., Yin, X., Martin-Sancho, L., Matsunaga, N., Pache, L., et al. (2020). Discovery of SARS-CoV-2 Antiviral Drugs through Large-Scale Compound Repurposing. Nature 586 (7827), 113–119. doi:10.1038/s41586-020-2577-1
Roved, J., Westerdahl, H., and Hasselquist, D. (2017). Sex Differences in Immune Responses: Hormonal Effects, Antagonistic Selection, and Evolutionary Consequences. Hormones Behav. 88, 95–105. doi:10.1016/j.yhbeh.2016.11.017
Shang, Y., Smith, S., and Hu, X. (2016). Role of Notch Signaling in Regulating Innate Immunity and Inflammation in Health and Disease. Protein Cell 7 (3), 159–174. doi:10.1007/s13238-016-0250-0
Song, Y., Zhang, M., Yin, L., Wang, K., Zhou, Y., Zhou, M., et al. (2020). COVID-19 Treatment: Close to a Cure? A Rapid Review of Pharmacotherapies for the Novel Coronavirus (SARS-CoV-2). Int. J. Antimicrob. agents 56 (2), 106080. doi:10.1016/j.ijantimicag.2020.106080
Tay, M. Z., Poh, C. M., Rénia, L., MacAry, P. A., and Ng, L. F. P. (2020). The Trinity of COVID-19: Immunity, Inflammation and Intervention. Nat. Rev. Immunol. 20 (6), 363–374. doi:10.1038/s41577-020-0311-8
Wang, D., Hu, B., Hu, C., Zhu, F., Liu, X., Zhang, J., et al. (2020). Clinical Characteristics of 138 Hospitalized Patients with 2019 Novel Coronavirus-Infected Pneumonia in Wuhan, China. JAMA 323 (11), 1061–1069. doi:10.1001/jama.2020.1585
Wehbe, Z., Hammoud, S. H., Yassine, H. M., Fardoun, M., El-Yazbi, A. F., and Eid, A. H. (2021). Molecular and Biological Mechanisms Underlying Gender Differences in COVID-19 Severity and Mortality. Front. Immunol. 12, 659339. doi:10.3389/fimmu.2021.659339
Weichhart, T., Hengstschläger, M., and Linke, M. (2015). Regulation of Innate Immune Cell Function by mTOR. Nat. Rev. Immunol. 15 (10), 599–614. doi:10.1038/nri3901
Wu, F., Zhang, W., Zhang, L., Wang, D., and Wan, Y. (2020). Discontinuation of Antiviral Drugs May Be the Reason for Recovered COVID-19 Patients Testing Positive Again. Br. J. Hosp. Med. Lond. Engl. 2005) 81 (4), 1–2. doi:10.12968/hmed.2020.0156
Wu, X., Wang, Z., He, Z., Li, Y., Wu, Y., Wang, H., et al. (2021). A Follow-Up Study Shows that Recovered Patients with Re-positive PCR Test in Wuhan May Not Be Infectious. BMC Med. 19 (1), 77. doi:10.1186/s12916-021-01954-1
Zhang, J., Lin, D., Li, K., Ding, X., Li, L., Liu, Y., et al. (2021). Transcriptome Analysis of Peripheral Blood Mononuclear Cells Reveals Distinct Immune Response in Asymptomatic and Re-detectable Positive COVID-19 Patients. Front. Immunol. 12, 716075. doi:10.3389/fimmu.2021.716075
Zhang, Y., Xu, J., Jia, R., Yi, C., Gu, W., Liu, P., et al. (2020). Protective Humoral Immunity in SARS-CoV-2 Infected Pediatric Patients. Cell Mol. Immunol. 17 (7), 768–770. doi:10.1038/s41423-020-0438-3
Zhou, J., Zhang, J., Zhou, J., Yi, H., Lin, Z., Liu, Y., et al. (2020). Clinical Characteristics of Re-positive COVID-19 Patients in Huangshi, China: A Retrospective Cohort Study. PloS one 15 (11), e0241896. doi:10.1371/journal.pone.0241896
Keywords: COVID-19, SARS-CoV-2, re-positive, immune, inflammation
Citation: Peng Y, Wang S, Chai R, Chen Y, Li N, Zeng B, Tang Q, Zheng K, Liang Y, Xie S, Huang W, Wang S and Wang X (2022) Clinical and Gene Features of SARS-CoV-2-Positive Recurrence in Patients Recovered From COVID-19. Front. Mol. Biosci. 9:875418. doi: 10.3389/fmolb.2022.875418
Received: 14 February 2022; Accepted: 22 April 2022;
Published: 08 June 2022.
Edited by:
Hem Chandra Jha, Indian Institute of Technology Indore, IndiaReviewed by:
Alexander V. Ivanov, Engelhardt Institute of Molecular Biology (RAS), RussiaCopyright © 2022 Peng, Wang, Chai, Chen, Li, Zeng, Tang, Zheng, Liang, Xie, Huang, Wang and Wang. This is an open-access article distributed under the terms of the Creative Commons Attribution License (CC BY). The use, distribution or reproduction in other forums is permitted, provided the original author(s) and the copyright owner(s) are credited and that the original publication in this journal is cited, in accordance with accepted academic practice. No use, distribution or reproduction is permitted which does not comply with these terms.
*Correspondence: Wei Huang, c2NyZWVuaW5nX3N6QDE2My5jb20=; Shaoxiang Wang, d3N4QHN6dS5lZHUuY24=; Xiao Wang, d2FuZ3hpYW8wNzE5QDE2My5jb20=
†These authors have contributed equally to this work
Disclaimer: All claims expressed in this article are solely those of the authors and do not necessarily represent those of their affiliated organizations, or those of the publisher, the editors and the reviewers. Any product that may be evaluated in this article or claim that may be made by its manufacturer is not guaranteed or endorsed by the publisher.
Research integrity at Frontiers
Learn more about the work of our research integrity team to safeguard the quality of each article we publish.