Corrigendum: Identification of circRNA biomarker for gastric cancer through integrated analysis
- 1University of Chinese Academy of Sciences, Beijing, China
- 2Center for High Performance Computing, Joint Engineering Research Center for Health Big Data Intelligent Analysis Technology, Shenzhen Institutes of Advanced Technology, Chinese Academy of Sciences, Shenzhen, China
- 3Department of Statistics, Bangabandhu Sheikh Mujibur Rahman Science and Technology University, Gopalganj, Bangladesh
- 4Shenzhen Science & Technology Development Exchange Center, Shenzhen Science and Technology Building, Shenzhen, China
- 5Guangdong Provincial Key Laboratory for Genome Stability & Disease Prevention and Regional Immunity and Diseases, Department of Pathology, Shenzhen University School of Medicine, Shenzhen, China
Gastric cancer (GC) is one of the most common malignant tumors and ranks third in cancer mortality globally. Although, a lot of advancements have been made in diagnosis and treatment of gastric cancer, there is still lack of ideal biomarker for the diagnosis and treatment of gastric cancer. Due to the poor prognosis, the survival rate is not improved much. Circular RNAs (circRNAs) are single-stranded RNAs with a covalently closed loop structure that don’t have the 5′-3′ polarity and a 3′ polyA tail. Because of their circular structure, circRNAs are more stable than linear RNAs. Previous studies have found that circRNAs are involved in several biological processes like cell cycle, proliferation, apoptosis, autophagy, migration and invasion in different cancers, and participate in some molecular mechanisms including sponging microRNAs (miRNAs), protein translation and binding to RNA-binding proteins. Several studies have reported that circRNAs play crucial role in the occurrence and development of different types of cancers. Although, some studies have reported several circRNAs in gastric cancer, more studies are needed in searching new biomarkers for gastric cancer diagnosis and treatment. Here, we investigated potential circRNA biomarkers for GC using next-generation sequencing (NGS) data collected from 5 paired GC samples. A total of 45,783 circRNAs were identified in all samples and among them 478 were differentially expressed (DE). The gene ontology (GO) analysis of the host genes of the DE circRNAs showed that some genes were enriched in several important biological processes, molecular functions and cellular components. The Kyoto encyclopedia of genes and genomes (KEGG) pathway analysis revealed that some host genes were enriched in several GC related pathways. The circRNA-miRNA-gene interaction network analysis showed that two circRNAs circCEACAM5 and circCOL1A1 were interacted with gastric cancer related miRNAs, and their host genes were also the important therapeutic and prognostic biomarkers for GC. The experimental results also validated that these two circRNAs were DE in GC compared to adjacent normal tissues. Overall, our findings suggest that these two circRNAs circCEACAM5 and circCOL1A1 might be the potential biomarkers for the diagnosis and treatment of GC.
Introduction
Gastric cancer (GC) is the fifth most common malignant tumors and third leading cause of cancer related deaths worldwide (Bray et al., 2018). Despite of having advanced diagnosis and treatment strategies, the prognosis of gastric cancer is still poor, and a 5-year survival rate is still less than 30% (Allemani et al., 2015). Due to poor prognosis, most of the patients are diagnosed at advance stages and are not capable of receiving the surgical therapy (Karimi et al., 2014). Therefore, new molecular diagnostic biomarker and therapeutic targets are of great interest to understand the molecular mechanism of gastric cancer diagnosis and treatment for early detection of patients and improving the overall survival rate.
Circular RNAs (circRNAs) are a class of single-stranded RNAs formed by a covalently closed loop structure without 5′-3′ polarities or 3′ polyA tail (Jiao Li et al., 2020). Although most of the circRNAs are generated from back splicing of exons, some are generated from introns and some are from both of exons and introns (Wang and Dong, 2019). Because of the circular structure, circRNAs are more stable than linear RNAs, and are abundant in different species. CircRNAs have the vast potentiality to be a molecular biomarker for diagnosis, treatment and prognosis of different cancers. Previous studies have demonstrated that circRNAs are involved in several biological processes including cell cycle, proliferation, apoptosis, autophagy, migration and invasion, in different types of cancers (Han et al., 2017; Sollott, 2017; Meng et al., 2018; Yu et al., 2018; Zhang et al., 2018). In recent years, circRNAs have been found to participate in several molecular mechanism like sponging microRNAs (miRNAs), protein translation and binding to RNA-binding proteins (Hansen et al., 2013; Kristensen et al., 2018; Chen et al., 2019; Zang et al., 2020). Moreover, some circRNAs can bind protein/peptides (Legnini et al., 2017; Pamudurti et al., 2017). Several studies have identified that circRNAs play key role in the occurrence and progression of a lot of malignancies like glioma (Li et al., 2018), hepatocellular carcinoma (Han et al., 2017), pancreatic carcinoma (Huang et al., 2018), gastric cancer (Chen et al., 2017), colon cancer (Xu et al., 2017), prostate cancer (Tucker et al., 2020), breast cancer (Li et al., 2019) etc. Despite several circRNAs have been reported in GC, more studies are needed to identify new biomarker for the diagnosis and treatment of this cancer.
In this study, to identify potential circRNA biomarker, we used next-generation sequencing (NGS) data from gastric cancer tissue and adjacent normal tissues. Then, differentially expressed circRNAs were identified and circRNA-miRNA-gene interaction network was constructed. GO term and KEGG pathway analyses were performed for the host genes of the DE circRNAs. GO term analysis showed that several genes functioned in some biological processes, molecular functions and cellular components. The KEGG pathway analysis showed that some genes were involved in several gastric cancer related pathways. We found two circRNAs circCEACAM5 and circCOL1A1 which were differentially expressed in all samples and these circRNAs were highly interacted with gastric cancer related miRNAs. In addition, the host genes (CEACAM5 and COL1A1) of these circRNAs were the important therapeutic and prognostic biomarker for gastric cancer (Hu and Chen, 2012; Zhou et al., 2015; Zhaoxing Li et al., 2020). The important properties of circRNAs includes sponging miRNAs and regulating gene transcription. As the host genes of the two circRNAs were important biomarker of GC and interacted with GC related miRNAs, they might have the potential to be important biomarker for GC. The expression of these two circRNAs were also validated by qRT-PCR experiments. Our results suggest that the circRNAs circCEACAM5 and circCOL1A1 might be the potential biomarkers for the diagnosis and treatment of GC.
Methods and Materials
Sample Collection
Fifteen pairs (10 for RT-PCR and 5 for RNA sequencing) of non-neoplastic gastric tissues (NT) and GCs tissues from Shenzhen Second People’s Hospital (The First affiliated Hospital of Shenzhen University) were examined in the study. All tissues received no radiotherapy or chemotherapy before surgery and stored in RNAlater immediately after surgery. All patients provided written informed consent, approved by the ethics committee of Shenzhen University School of medicine.
Ribonucleic Acid Library Preparation
Total RNA was extracted from 5 paired GC samples. Ribosomal RNA was digested using ribo zero kit and linear RNA was removed using rnase R. CircRNA was fragmented and cDNA was synthesized with six base random primers and purified. Strand-specific library is prepared via incorporating a chemical label deoxy-UTP (dUTP) during production of the second-strand cDNA. The second-strand cDNA was specifically digested by UNG enzyme. The constructed library was analyzed by Agilent 2,100 Bioanalyzer and was sequenced on an Illumina Hiseq 2,500 (Chi Biotech, Shenzhen).
Ribonucleic Acid-Seq Data Analysis
We used 10 samples from 5 gastric cancer patients to investigate potential circRNA biomarker. From each patients, two samples were collected: one from the cancer tissue and other from the adjacent normal tissue. After getting the raw data in fastq format, the quality control analysis was performed. The quality control was done using the NGSQCToolkit (Patel and Jain, 2012) and the quality score 20 was used as the cutoff point. Filtering/trimming was carried out if the quality of the reads failed to reach the cutoff point. The fastq reads were aligned to the human reference genome (version GRCh38) using the BWA (Li and Durbin, 2009) aligner. CircRNAs were identified using the software CIRI (version 2) (Gao et al., 2015). The identified circRNAs were then annotated with the gene annotation file corresponding to the reference genome and the full length circRNA sequences were extracted. The full length circRNA sequences for all circRNAs were considered as the reference genome and the fastq reads were mapped using the bowtie2 (Langmead and Salzberg, 2012) aligner. Then count data was generated using bedtools (Quinlan and Hall, 2010) multiBamCov with the output of bowtie2 (converted to bam, sorted and indexed). R package DESeq (Anders and Huber, 2010) and DESeq2 (Love et al., 2014) were used to identify differentially expressed (DE) circRNAs for individual patient and combining all patients together respectively. |Log2FoldChange|>1 and p-value<0.05 were considered as the cut-off for defining significant DE circRNAs. For the DE analysis of combination of all patients, we also considered false discovery rate (FDR) at 10%.
Real-Time-Polymerase Chain Reaction and Real-Time Quantitative Polymerase Chain Reaction
According to the manufacturer’s protocol, total RNA was extracted using Trizol reagent (251,808, Invitrogen). Reverse transcription and real-time PCR were performed using GoScript™ Reverse Transcription Mix (A2800 and A6002, Promega). Divergent primers were designed. All primers were synthesized by Sangon Biotech. Results were normalized to the 18S mRNA in each sample. We used the following primer sequences.
CircRNA-miRNA-Gene Interaction Network Analysis
We were interested with those circRNAs which were DE in four or more patients (out of five patients). Then, some top DE circRNAs were selected for miRNA interaction analysis. The circRNA-miRNA interaction was predicted using miRanda (Enright et al., 2003) software. The miRNA sequences were downloaded from mirbase (Kozomara et al., 2019). From the circRNA-miRNA interaction, a sub-network was constructed keeping only the gastric cancer related miRNAs. Then a circRNA-miRNA-gene network was constructed using Cytoscape (Shannon et al., 2003). The genes were the host genes of the circRNAs. Finally, a sub-network was constructed with the top 5 hub circRNAs.
Gene Ontology Term and Kyoto Encyclopedia of Genes and Genomes Pathway Analysis
Search Tool for the Retrieval of Interacting Genes (STRING; http://string-db.org/cgi/input.pl) is a database for obtaining the protein-protein interaction of the provided genes. The host genes of the DE circRNAs were mapped to STRING database, and the protein-protein interaction among the genes was obtained. Then, a PPI network was constructed using the software Cytoscape, and from the network, the top 50 hub genes were selected for GO term and KEGG pathway enrichment analyses. With the selected hub genes, GO term and KEGG pathway enrichment analyses were performed using DAVID (Huang et al., 2009) to know the function of the circRNAs. The threshold p-value<0.05 was used for the significance of the enrichment analysis.
Results
Expression Profiles of circRNAs
A total of 45,783 circRNAs were identified in all samples and 21,652 of them were co-expressed in cancer and normal samples (Figure 1A). Among the total circRNAs, 79% (36,218/45,783) were exonic, 1% (462/45,783) were intronic, 4% (1848/45,783) were intergenic, 15% (6,744/45,783) were sense overlapping and 1% (511/45,783) were antisense (Figure 1C). The length distribution of the circRNAs was shown in Figure 1B and observed that the length of 74% (44 + 30) of the circRNAs was less than 1,000 nucleotides (nt). The length of only 13% circRNAs was greater than 2000 nt. The number of back-spliced reads for the 97% of the circRNAs were less than 20 (Figure 1D). The average number of back spliced reads per circRNA was 6 whereas the minimum and the maximum number of back spliced reads were 2 and 898 respectively. The distribution of circRNAs in different chromosomes were heterogeneous, most circRNAs were originated from chromosome NC_000,001.11 (chromosome 1) (Figure 1E). The average number of circRNAs per chromosome was 1,405. The length of the circRNAs ranged from 48 to 199,579 nt while median and average lengths were 563 nt and 3,042 nt respectively (Table 1).
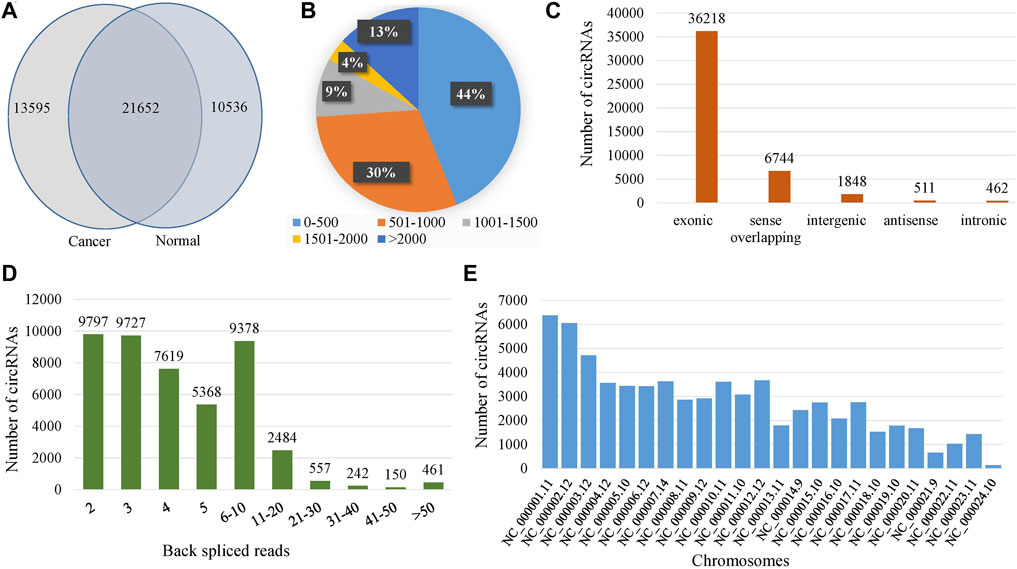
FIGURE 1. Expression profiles of circRNAs. (A) Venn-diagram of the identified circRNAs in normal and cancer samples, (B) Length distribution of circRNAs, (C) Classification of the identified circRNAs, (D) Distribution of the number of back spliced reads, and (E) Chromosome distribution of the identified circRNAs.
The expression values of the 10 samples from the five patients were shown in Figure 2A. The expression values were almost homogeneous across the samples. We also performed the Principal Component Analysis (PCA) of the expression values and the loading of the PC1 and PC2 were shown in Figure 2B. The distribution of circRNAs across the number of exons was shown in Figure 2C. Majority of the circRNAs were composed of 2 or 3 exons. About 76% of the circRNAs were derived from less than or equal to 5 exons. The distribution of the number of circRNAs per gene was given in Figure 2D. Total 45,783 circRNAs were originated from 8,095 genes. Majority of the cases (about 30%), the number of circRNA per gene was 1. About 55% of the cases, the number of circRNAs per gene was less than or equal to 3.
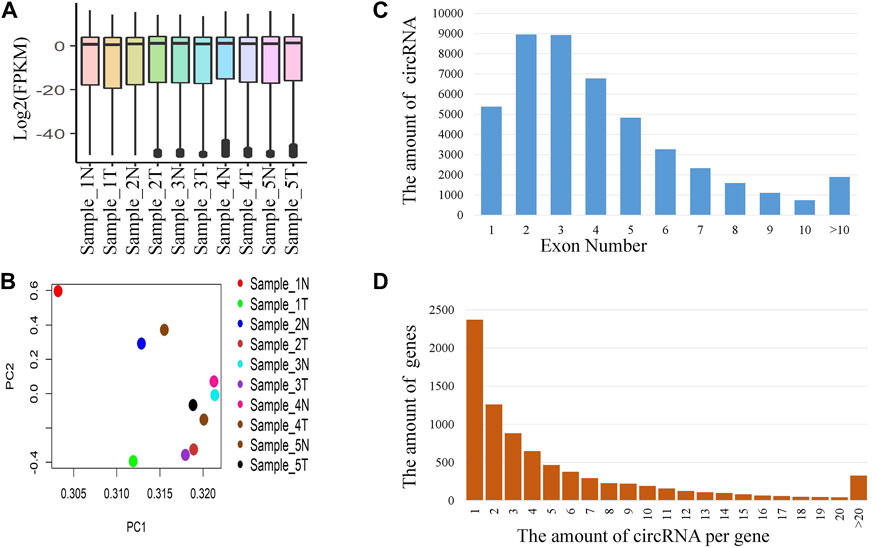
FIGURE 2. Distribution of circRNAs. (A) Expression (FPKM) of circRNAs for different samples, (B) Biplot of PCA loadings for different samples, (C) Distribution of circRNAs derived from different number of exons, and (D) Distribution of the number of circRNAs per gene.
Differential Expression Analysis of circRNAs
We identified the differentially expressed circRNAs between two groups (normal vs cancer). The R package DESeq was used to find the differentially expressed circRNAs. We collected 10 samples from five patients: for each patients two samples were collected one from cancer tissue and other from the adjacent normal tissue. We also performed DE analysis between normal and cancer groups for each patient. The volcano plot was used to find the target circRNAs with the thresholds p-value<0.05 and |log2FoldChange| >1 (Figure 3). The number of DE circRNAs for all samples were given in Table 2. Out of 45,783 total circRNAs, 478 were differentially expressed between normal vs cancer groups. From Table 2, we observed that about half of the DE circRNAs were upregulated, whereas the other half were downregulated. The expression patterns of these circRNAs were shown in the heatmap (Figure 4). The dendrograms showed that the cancer and normal samples were clearly distinguishable. Firstly, we identified DE circRNAs for all patients (5 normal samples vs 5 cancer samples) and performed DE analysis for each individual patient and isolated those circRNAs which were DE in four or more patients. 4 upregulated and 7 downregulated circRNAs were found to be DE in more than four patients. We selected 8 circRNAs (4 upregulated and 4 downregulated) from the DE analysis of individual patient. These 8 circRNAs were not only DE patient-wise but also DE while performed DE analysis combining all patients (5 normal samples vs 5 cancer samples). Finally, these 8 circRNAs were selected for circRNA-miRNA interaction analysis. The details of the 8 circRNAs were given in Table 3.
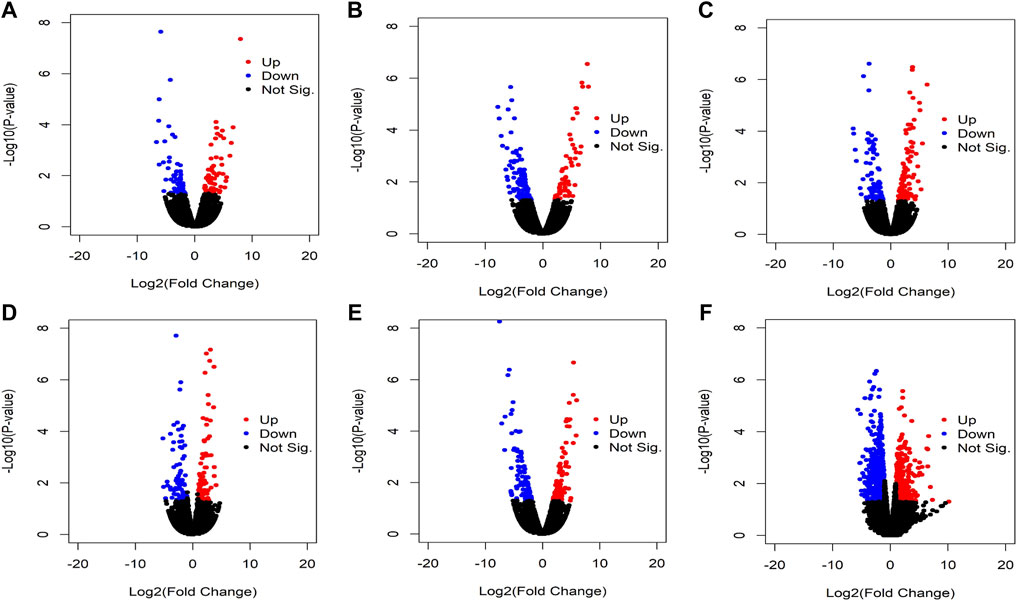
FIGURE 3. Volcano plot of the circRNAs. Red colors indicate upregulated circRNAs while blue colors indicate downregulated circRNAs and black colors indicate not significant. Results of DE analysis of (A) Sample_1N vs Sample_1T, (B) Sample_2N vs Sample_2T, (C) Sample_3N vs Sample_3T, (D) Sample_4N vs Sample_4T, (E) Sample_5N vs Sample_5T, and (F) Sample_N vs Sample_T.
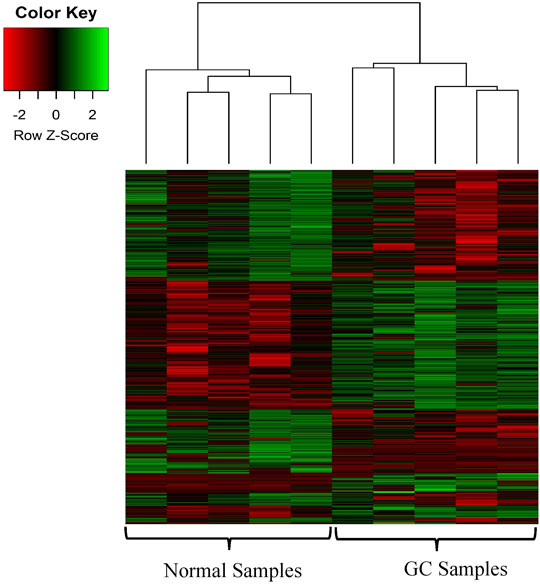
FIGURE 4. Heatmap of the DE circRNAs. The heatmap represents the expression profile of the DE circRNAs in GC compared to the adjacent normal tissues. The color scale indicates the Log2FoldChange of the expression value for each circRNA in cancers vs normal tissues. Red and green colors indicate down-regulation and up-regulation of the circRNAs respectively.
CircRNA-miRNA-Gene Interaction Analysis
We got a total of 478 circRNAs as DE between normal vs cancer groups. Among these circRNAs, we investigated those which were DE in more number of patients. We chose those circRNAs for interaction analysis, which were DE in four or more patients (out of five patients). We selected the 4 upregulated and 4 downregulated (top 4 out of 7 downregulated) circRNAs for miRNA interaction analysis. We got a total of 1990 interactions with 8 circRNAs and 1,278 miRNAs. The miRNA sequences were downloaded from the mirbase database. From the online search we found 64 GC related miRNAs out of 1,278 miRNAs in the network. Next, we extracted the interactions of these 64 miRNAs and obtained a total of 81 interactions. Then, a circRNA-miRNA-gene network was constructed using Cytoscape. Here, the genes were the host genes of the circRNAs. Finally, we constructed a subnetwork with top 5 hub circRNAs (Figure 5). From the network, we observed that two genes were functionally related to GC. Two circRNAs circCEACAM5 and circCOL1A1 were interacted with GC related miRNAs and their host genes were also related to GC. This indicated that these two circRNAs might be potential biomarker for GC. The detail list of top 5 hub circRNAs, their interacted GC related miRNAs and their host genes were given in Table 4.
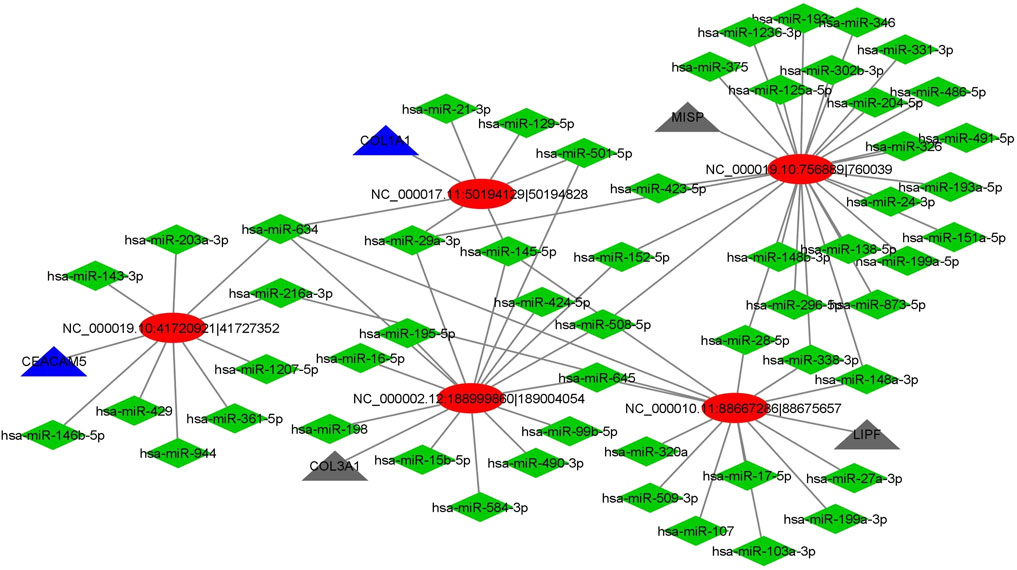
FIGURE 5. CircRNA-miRNA-gene interaction network. Ellipse shape indicates circRNA, diamond shape indicates miRNA while triangle indicates gene. Blue triangle indicates genes related to GC and black triangle indicates other genes.
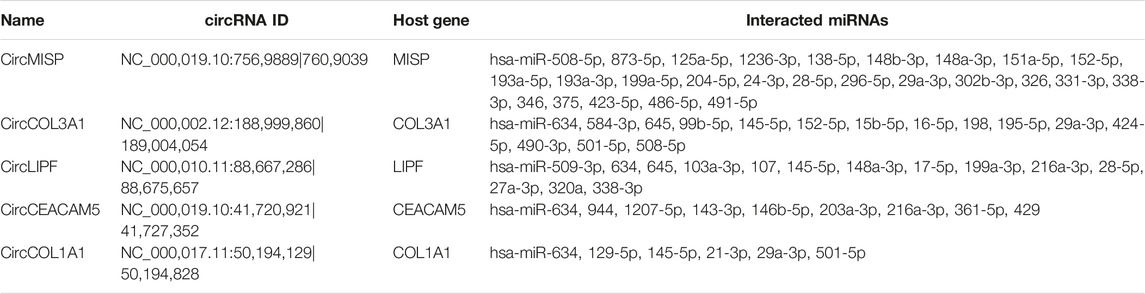
TABLE 4. The five hub circRNAs, their interacted gastric cancer related miRNAs and the host genes of the circRNAs.
Differential Expression Analysis of the Targeted miRNAs Genes and Candidate circRNAs
We performed the differential expression analysis of the miRNAs in gastric cancer tissue and the adjacent normal tissue using the GEO dataset (GSE158315). R package limma (Ritchie et al., 2015) was used to find the DE miRNAs. The expression patterns of the DE miRNAs were shown in Figure 6A. The dendrograms showed that the cancer and normal samples were clearly distinguishable. The volcano plot (Figure 6B) was used to find the DE miRNAs with the threshold p-value<0.05 and |log2FoldChange|>1. We found a total of 176 DE miRNAs of which 60 were upregulated and 116 were downregulated. The number of targeted miRNAs for circRNAs circCEACAM5 and circCOL1A1 were 9 and 5 respectively. The unique targeted miRNAs for these two circRNAs were 13 (Figure 6C). MiRNAs hsa-miR-634 and hsa-miR-429 were common between the 9 targeted miRNAs of circCEACAM5 and the 176 DE miRNAs. Again, miRNAs hsa-miR-634, hsa-miR-21-3p and hsa-miR-145-5p were common between the 5 targeted miRNAs of circCOL1A1 and the 176 DE miRNAs. MiRNAs hsa-miR-429, hsa-miR-21-3p and hsa-miR-145-5p were found to be significantly upregulated in GC (Figures 6D–F). Therefore, miRNA hsa-miR-429 might be the potential target of the circRNA circCEACAM5, and miRNAs hsa-miR-21-3p and hsa-miR-145-5p might be the potential targets of the circRNA circCOL1A1.
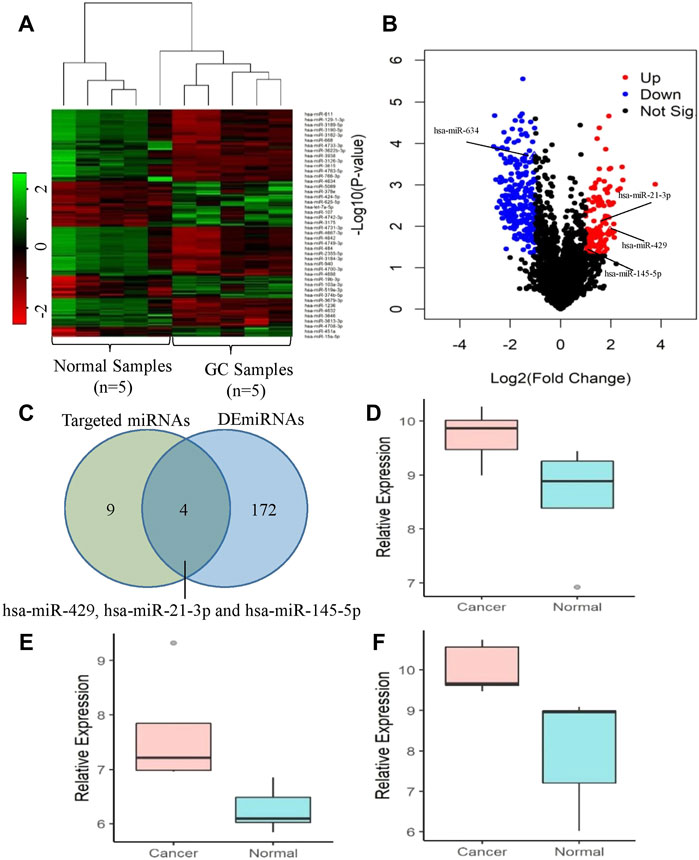
FIGURE 6. Expression analysis of the targeted miRNAs of the circRNAs circCEACAM5 and circCOL1A1. (A) Clustered heatmap represents the expression profile of the DE miRNAs in GC compared to normal tissues. The color scale indicates the Log2FoldChange of the expression value for each miRNA in cancers vs normal tissues. Red color implies down-regulation while green color indicates up-regulation. (B) Volcano plot of the DE miRNAs in GC relative to normal tissues. Red, blue and black dots indicate up-regulation, down-regulation and not significant respectively. (C) Identification of the potential target miRNAs of the circRNAs circCEACAM5 and circCOL1A1. Expression levels of (D) hsa-miR-429, (E) hsa-miR-21-3p and (F) hsa-miR-145-5p in GC compared to normal tissues.
We selected top 8 circRNAs (4 upregulated and 4 downregulated) for the downstream analysis. These 8 circRNAs were originated from 6 host genes. The expression of these 6 host genes were checked by the TCGA data of 408 gastric tumor samples and 36 normal samples obtained from Gepia (version 2) (Tang et al., 2019). We found that 4 genes were upregulated in the GC tissue compared to the normal tissue Figures 7A–D. In addition 2 genes were downregulated in GC compared to the normal samples Figures 7E,F. The 4 upregulated host genes might be the potential targets of their respective circRNAs.
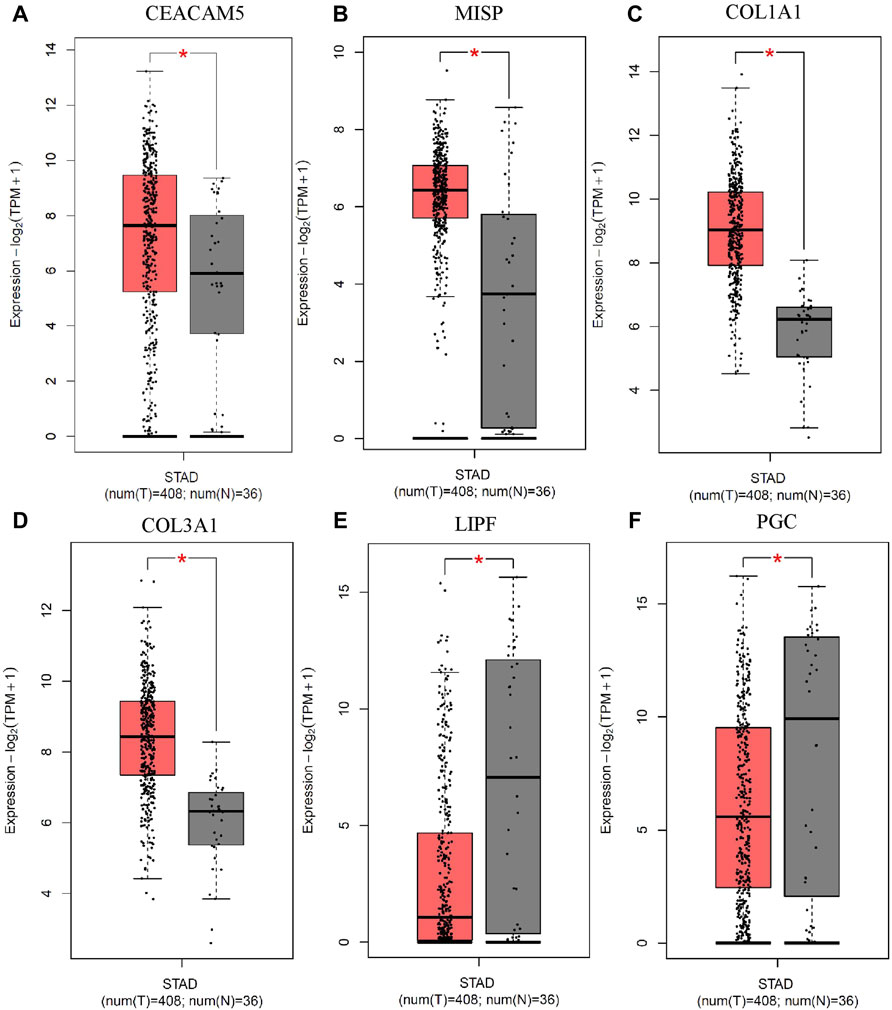
FIGURE 7. Validation of the expression of 6 host genes of the top up and down regulated circRNAs in GC. The expression of (A) CEACAM5, (B) MISP, (C) COL1A1 and (D) COL3A1 was upregulated, and the expression of (E) LIPF and (F) PGC was downregulated in gastric tumor compared to normal samples from the TCGA data. The cutoffs |Log2FoldChange|>1 and p-value<0.05 were considered as statistically significant.
We also performed the receiver operating characteristic (ROC) curve analysis for our two candidate circRNA biomarkers circCEACAM5 and circCOL1A1 (Figures 8A,B). The area under the ROC curves (AUC) for these two circRNAs were significant (p-value<0.05). The AUC values 0.96 and 0.94 represented respectively the accuracy of circCEACAM5 and circCOL1A1 in distinguishing GC and normal patients.
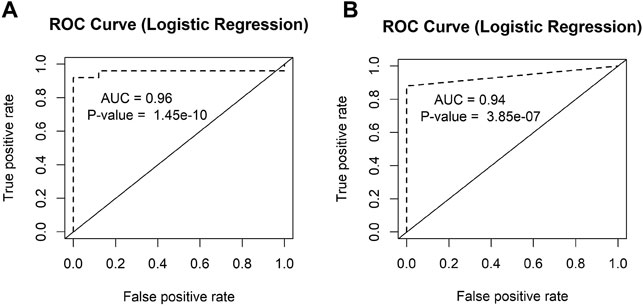
FIGURE 8. ROC analysis of the candidate circRNAs. The AUC values of the ROC curves of (A) circCEACAM5 and (B) circCOL1A1 represented the accuracy of these circRNAs in distinguishing GC and normal patients.
Gene Ontology Term and Kyoto Encyclopedia of Genes and Genomes Pathway Analysis
We constructed the PPI network from STRING database for the host genes of the DE circRNAs and selected the top 50 hub genes using cytoscape. To explore the functions of circRNAs, we performed the GO and KEGG pathway analyses for the top 50 hub genes of the DE circRNAs from the PPI network. We used the cut-off p < 0.05 for finding the significant pathways and GO terms. The GO term biological process analyses showed that some of the genes were enriched cell-cell adhesion, extracellular matrix organization, DNA repair, collagen catabolic process, collagen fibril organization, response to virus etc. The GO term molecular function analyses showed that some host genes were able to bind many kinds of molecules including ATP, protein, platelet-derived growth factor, DNA, microtubule, chromatin DNA, double-stranded RNA etc. Some other genes were enriched in cadherin binding involved in cell-cell adhesion, extracellular matrix structural constituent, microtubule motor activity etc. The GO term cellular component analyses showed that some genes were enriched in nucleus, nucleoplasm, cytosol, cytoplasm, mitotic spindle, basement membrane, mitotic spindle etc. The KEGG pathway analysis indicated that some host genes were enriched in several significant pathways including ECM-receptor interaction, PI3K-Akt signaling pathway, Focal adhesion, Cell cycle etc. The results of the significant GO term (top 30) and KEGG pathway analyses were provided in Tables 5, 6 respectively.
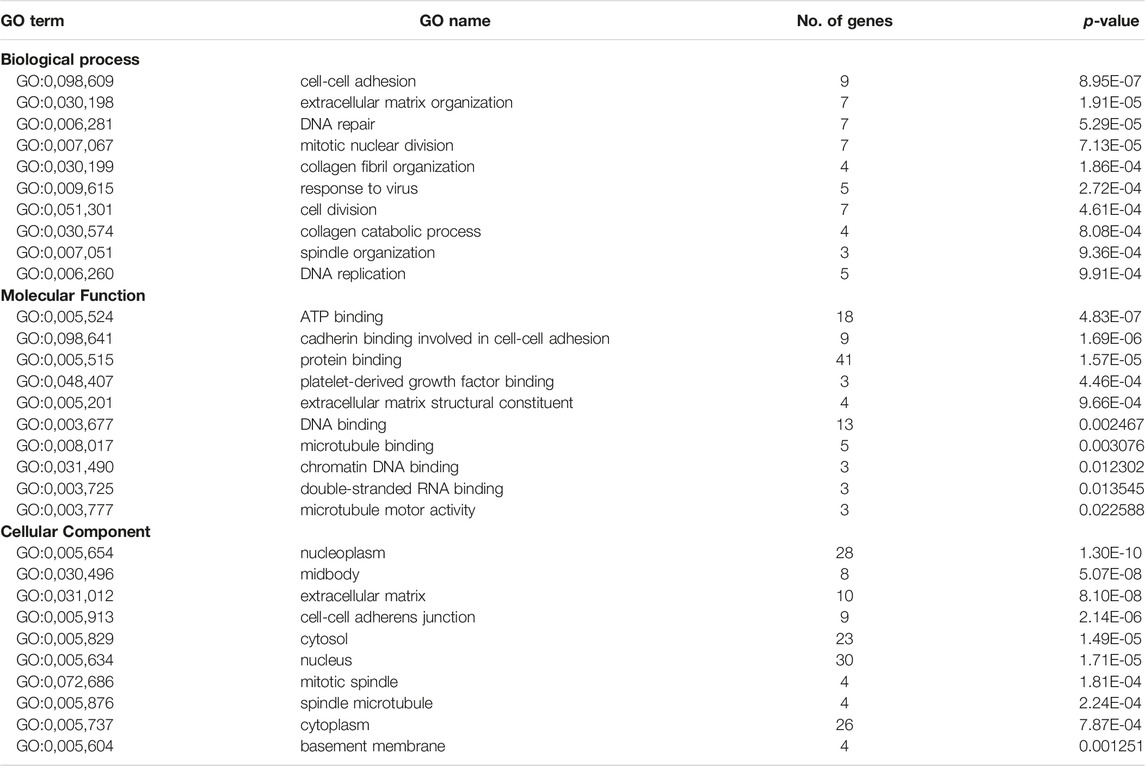
TABLE 5. Top 30 GO terms for the top 50 hub genes from the PPI networks of the host genes of the DE circRNAs.
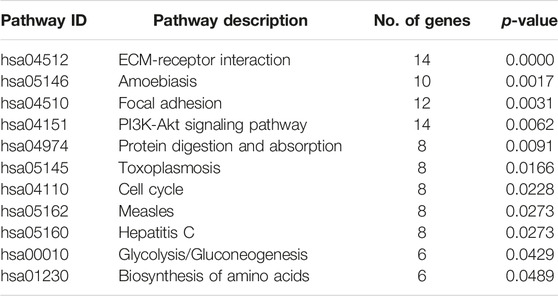
TABLE 6. Significant pathways for the top 50 hub genes from the PPI network of the host genes of the DE circRNAs.
Validation of the circRNAs by qReal-Time-Polymerase Chain Reaction
We selected 8 circRNAs (4 upregulated and 4 downregulated) for miRNA interaction analysis. These 8 circRNAs were DE in four (out of five) or more patients. Among these 8 circRNAs, we selected 5 circRNAs (3 upregulated and 2 downregulated) for experimental validation by qRT-PCR. The expression of these 5 circRNAs were shown in Figure 9. From Figure 9, we observed that the expression of circCEACAM5, circMISP, circCOL1A1 was upregulated in GC, which is consistent with the sequencing result. The expression of circLIPF and circPGC was decreased in GC in line with the sequencing result. Hence, the computational results for these 5 circRNAs were validated by the qRT_PCR experiments.
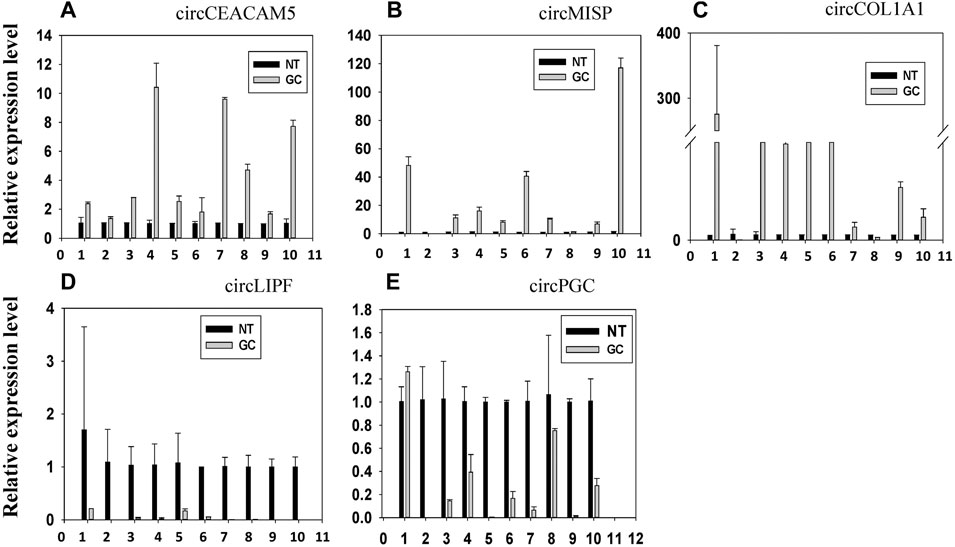
FIGURE 9. The relative expression level of top 5 up and downregulated circRNAs. The expression of (A) circCEACAM5, (B) circMISP, (C) circCOL1A1, (D) circLIPF and (E) circPGC in 10 paired gastric cancer (GC) specimens and the corresponding paired normal adjacent tissues (NT) was determined using real-time quantitative RT-PCR with divergent primers.
Discussion
The role of circRNAs in human cancer is unrevealing gradually in recent years. In the present study, we predicted some circRNA biomarkers for gastric cancer through computational approach. We found a total of 45,783 circRNAs from 5 patients (10 samples). Then we performed DE analysis and found 4 upregulated and 7 downregulated circRNAs which were DE in four or more patients. Among these, we selected 8 circRNAs (4 upregulated and 4 downregulated) for miRNA interaction analysis.
The most important property of circRNA is known to function as miRNA sponges. As miRNA plays a crucial role in cancer progression, we explored the relationship between circRNAs and miRNAs through circRNA-miRNA-gene interaction network analysis. From the interaction network, we selected 5 hub circRNAs and found that the two circRNAs CircCEACAM5 and CircCOL1A1 were interacted with GC related miRNAs and their host genes were the important therapeutic and prognostic biomarker for GC. The circRNA CircCEACAM5 was interacted with 9 GC related miRNAs and among them miR-634 inhibits the proliferation, migration, and invasion of gastric cancer cell lines (Guo et al., 2018), miR-143-3p inhibits GC cell growth and induces apoptosis (Wu et al., 2013), and miR-429 acts as a tumor suppressor in GC cells (Zhang et al., 2016). The circRNA CircCOL1A1 was interacted with 5 GC related miRNAs for instances, miR-129-5p suppresses the proliferation of GC cells (Feng et al., 2020), miR-145-5p is able to inhibit the proliferation, migration and invasion of GC cells (Zhou et al., 2020), miR-501-5p promotes cell proliferation and migration in GC cells (Ma et al., 2020), miR-21-3p is closely related to GC and can be used to predict the prognosis of GC (Sun et al., 2021). We also performed the differential expression analysis of the targeted miRNAs of the circRNAs circCEACAM5 and circCOL1A1. We found that miRNAs miR-429, miR-21-3p and miR-145-5p significantly upregulated in GC compared to normal tissues. Thus, miR-429 might be the potential target of circCEACAM5 and, miR-21-3p and miR-145-5p might be the potential targets of circCOL1A1.
Another important property of circRNA is to regulate gene transcription (Meng et al., 2017). We performed the differential expression analysis of the parental genes of the 8 top up and down regulated circRNAs in GC using TCGA data. We found genes CEACAM5 and COL1A1 as upregulated in GC compared to normal tissues. These two genes were the key therapeutic and prognostic biomarkers for GC (Hu and Chen, 2012; Zhou et al., 2015; Zhaoxing Li et al., 2020). Thus, circCEACAM5 and circCOL1A1 have the potentials to regulate their parental gene transcription.
The GO term analysis of the parental genes of DE circRNAs showed that some host genes were involved in several important biological processes, molecular mechanisms and cellular components. The KEGG pathway analysis showed that some host genes were involved in several significant pathways such as ECM-receptor interaction, Focal adhesion, PI3K-Akt signaling pathway, Cell cycle etc. All of these pathways are involved in important mechanism of GC. ECM-receptor interaction pathway is identified as an important pathway associated with the progression of GC (Hu and Chen, 2012). Focal adhesion plays a key role in regulating cell survival, and proliferation, migration, and invasion of GC cells (Mao et al., 2021). The PI3K-Akt signaling pathway plays an important role in the development and progression of GC (Matsuoka and Yashiro, 2014). And a stronger enrichment of cell cycle pathway is found in GC (Saberi Anvar et al., 2018).
The host genes of these two circRNAs are also functionally related to GC. Gene CEACAM5 is a promising biomarker for prewarning and prognosis of GC (Zhou et al., 2015) and COL1A1 is considered as a potential biomarker for prognosis of GC (Hu and Chen, 2012; Zhaoxing Li et al., 2020). The two circRNAs circCEACAM5 and circCOL1A1 are DE in four or more patients (out of five), are substantially interacted with gastric cancer associated miRNAs, and the host genes of the DE circRNAs are involved in several gastric cancer related pathways. Furthermore the host genes of these two circRNAs are key therapeutic and prognostic biomarker for gastric cancer. The expression of these two circRNAs are also validated by qRT-PCR experiments. Hence, these two circRNAs might be potential biomarker for gastric cancer diagnosis and treatment.
Conclusion
In the current study, we investigated potential circRNA biomarkers for GC through integrated analyses using 10 NGS samples collected from 5 patients. From differential expression and circRNA-miRNA-gene interaction analyses, we found two circRNAs circCEACAM5 and circCOL1A1 as DE and these circRNAs were interacted with GC related miRNAs. The host genes of these circRNAs were also the potential therapeutic and prognostic biomarkers for GC. The GO and KEGG pathway analyses revealed that some host genes of the DE circRNAs were enriched in several significant processes (biological, molecular and cellular) and enriched in several GC related pathways. These two circRNAs were also validated by the qRT-PCR experiments. Combining all the results, we can conclude that these two circRNAs circCEACAM5 and circCOL1A1 might play important role in the diagnosis and treatment of GC.
Data Availability Statement
The datasets presented in this study can be found in online repositories. The names of the repository/repositories and accession number(s) can be found below: China National Center for Bioinformation, accession PRJCA008130.
Ethics Statement
The studies involving human participants were reviewed and approved by the ethics committee of Shenzhen University School of Medicine. The patients/participants provided their written informed consent to participate in this study.
Author Contributions
TH, SL, YP and YW designed the study. TH and SL performed the bioinformatics pipelines, analysed the data and wrote the manuscript. YP performed the experimental validation. SR, YP, SF, XZ, ZJ and YW extensively edited the manuscript. SF and YW supervised the work. All the authors read and approved the final version of the manuscript for publication.
Funding
This work was partly supported by the National Key Research and Development Program of China under Grant No. 2018YFB0204403; Strategic Priority CAS Project XDB38050100; National Science Foundation of China under grant no. U1813203; the Shenzhen Basic Research Fund under grant nos JCYJ20200109114818703, RCYX20200714114734194, and JSGG20201102163800001; CAS Key Lab under grant no. 2011DP173015 (YW). We would also like to thank the funding support from the Youth Innovation Promotion Association, CAS to YW. This work is also supported by the National Foundation of Science (82172946) to ZJ; the National Foundation of Science (82173290) to XZ; Shenzhen Basic Research Fund (JCYJ20190808163801777) to YP.
Conflict of Interest
The authors declare that the research was conducted in the absence of any commercial or financial relationships that could be construed as a potential conflict of interest.
Publisher’s Note
All claims expressed in this article are solely those of the authors and do not necessarily represent those of their affiliated organizations, or those of the publisher, the editors and the reviewers. Any product that may be evaluated in this article, or claim that may be made by its manufacturer, is not guaranteed or endorsed by the publisher.
Acknowledgments
We would like to thank all the members of Computational Biology and Bioinformatics Lab, Center for High Performance Computing, SIAT, CAS for their valuable suggestions and feedbacks.
References
Allemani, C., Weir, H. K., Carreira, H., Harewood, R., Spika, D., Wang, X. S., et al. (2015). Global Surveillance of Cancer Survival 1995-2009: Analysis of Individual Data for 25 676 887 Patients from 279 Population-Based Registries in 67 Countries (CONCORD-2). Lancet 385, 977–1010. doi:10.1016/S0140-6736(14)62038-9
Anders, S., and Huber, W. (2010). Differential Expression Analysis for Sequence Count Data. Genome Biol. 11. doi:10.1186/gb-2010-11-10-r106
Bray, F., Ferlay, J., Soerjomataram, I., Siegel, R. L., Torre, L. A., and Jemal, A. (2018). Global Cancer Statistics 2018: GLOBOCAN Estimates of Incidence and Mortality Worldwide for 36 Cancers in 185 Countries. CA. Cancer J. Clin. 68, 394–424. doi:10.3322/CAAC.21492
Chen, J., Li, Y., Zheng, Q., Bao, C., He, J., Chen, B., et al. (2017). Circular RNA Profile Identifies circPVT1 as a Proliferative Factor and Prognostic Marker in Gastric Cancer. Cancer Lett. 388, 208–219. doi:10.1016/j.canlet.2016.12.006
Chen, X., Yang, T., Wang, W., Xi, W., Zhang, T., Li, Q., et al. (2019). Circular RNAs in Immune Responses and Immune Diseases. Theranostics 9, 588–607. doi:10.7150/thno.29678
Enright, A. J., John, B., Gaul, U., Tuschl, T., Sander, C., and Marks, D. S. (2003). MicroRNA Targets in Drosophila. Genome Biol. 5, R1. doi:10.1186/gb-2003-5-1-r1
Feng, J., Guo, J., Wang, J. P., and Chai, B. F. (2020). MiR-129-5p Inhibits Proliferation of Gastric Cancer Cells through Targeted Inhibition on HMGB1 Expression. Eur. Rev. Med. Pharmacol. Sci 24, 3665–3673. doi:10.26355/eurrev_202004_20829
Gao, Y., Wang, J., and Zhao, F. (2015). CIRI: An Efficient and Unbiased Algorithm for De Novo Circular RNA Identification. Genome Biol. 16. doi:10.1186/s13059-014-0571-3
Guo, J., Zhang, C.-D., An, J.-X., Xiao, Y.-Y., Shao, S., Zhou, N.-M., et al. (2018). Expression of miR-634 in Gastric Carcinoma and its Effects on Proliferation, Migration, and Invasion of Gastric Cancer Cells. Cancer Med. 7, 776–787. doi:10.1002/cam4.1204
Han, D., Li, J., Wang, H., Su, X., Hou, J., Gu, Y., et al. (2017). Circular RNA circMTO1 Acts as the Sponge of microRNA-9 to Suppress Hepatocellular Carcinoma Progression. Hepatology 66, 1151–1164. doi:10.1002/hep.29270
Hansen, T. B., Jensen, T. I., Clausen, B. H., Bramsen, J. B., Finsen, B., Damgaard, C. K., et al. (2013). Natural RNA Circles Function as Efficient microRNA Sponges. Nature 495, 384–388. doi:10.1038/nature11993
Hu, K., and Chen, F. (2012). Identification of Significant Pathways in Gastric Cancer Based on Protein-Protein Interaction Networks and Cluster Analysis. Genet. Mol. Biol. 35, 701–708. doi:10.1590/S1415-47572012005000045
Huang, D. W., Sherman, B. T., and Lempicki, R. A. (2009). Bioinformatics Enrichment Tools: Paths toward the Comprehensive Functional Analysis of Large Gene Lists. Nucleic Acids Res. 37, 1–13. doi:10.1093/nar/gkn923
Huang, W.-J., Wang, Y., Liu, S., Yang, J., Guo, S.-x., Wang, L., et al. (2018). RETRACTED: Silencing Circular RNA Hsa_circ_0000977 Suppresses Pancreatic Ductal Adenocarcinoma Progression by Stimulating miR-874-3p and Inhibiting PLK1 Expression. Cancer Lett. 422, 70–80. doi:10.1016/j.canlet.2018.02.014
Karimi, P., Islami, F., Anandasabapathy, S., Freedman, N. D., and Kamangar, F. (2014). Gastric Cancer: Descriptive Epidemiology, Risk Factors, Screening, and Prevention. Cancer Epidemiol. Biomarkers Prev. 23, 700–713. doi:10.1158/1055-9965.EPI-13-1057
Kozomara, A., Birgaoanu, M., and Griffiths-Jones, S. (2019). MiRBase: From microRNA Sequences to Function. Nucleic Acids Res. 47, D155–D162. doi:10.1093/nar/gky1141
Kristensen, L. S., Hansen, T. B., Venø, M. T., and Kjems, J. (2018). Circular RNAs in Cancer: Opportunities and Challenges in the Field. Oncogene 37, 555–565. doi:10.1038/onc.2017.361
Langmead, B., and Salzberg, S. L. (2012). Fast Gapped-Read Alignment with Bowtie 2. Nat. Methods 9, 357–359. doi:10.1038/nmeth.1923
Legnini, I., Di Timoteo, G., Rossi, F., Morlando, M., Briganti, F., Sthandier, O., et al. (2017). Circ-ZNF609 Is a Circular RNA that Can Be Translated and Functions in Myogenesis. Mol. Cel 66, 22–37. doi:10.1016/j.molcel.2017.02.017
Li, H., and Durbin, R. (2009). Fast and Accurate Short Read Alignment with Burrows-Wheeler Transform. Bioinformatics 25, 1754–1760. doi:10.1093/bioinformatics/btp324
Li, G.-F., Li, L., Yao, Z.-Q., and Zhuang, S.-J. (2018). Hsa_circ_0007534/miR-761/ZIC5 Regulatory Loop Modulates the Proliferation and Migration of Glioma Cells. Biochem. Biophysical Res. Commun. 499, 765–771. doi:10.1016/j.bbrc.2018.03.219
Li, Z., Chen, Z., Hu, G. H., and Jiang, Y. (2019). Roles of Circular RNA in Breast Cancer: Present and Future. Am. J. Transl. Res. 11, 3945–3954.
Li, J., Sun, D., Pu, W., Wang, J., and Peng, Y. (2020). Circular RNAs in Cancer: Biogenesis, Function, and Clinical Significance. Trends Cancer 6, 319–336. doi:10.1016/j.trecan.2020.01.012
Li, Z., Liu, Z., Shao, Z., Li, C., Li, Y., Liu, Q., et al. (2020). Identifying Multiple Collagen Gene Family Members as Potential Gastric Cancer Biomarkers Using Integrated Bioinformatics Analysis. PeerJ 8, e9123. doi:10.7717/peerj.9123
Love, M. I., Huber, W., and Anders, S. (2014). Moderated Estimation of Fold Change and Dispersion for RNA-Seq Data with DESeq2. Genome Biol. 15. doi:10.1186/s13059-014-0550-8
Ma, X., Feng, J., Lu, M., Tang, W., Han, J., Luo, X., et al. (2020). microRNA-501-5p Promotes Cell Proliferation and Migration in Gastric Cancer by Downregulating LPAR1. J. Cel. Biochem. 121, 1911–1922. doi:10.1002/jcb.29426
Mao, D., Xu, R., Chen, H., Chen, X., Li, D., Song, S., et al. (2021). Cross-Talk of Focal Adhesion-Related Gene Defines Prognosis and the Immune Microenvironment in Gastric Cancer. Front. Cel Dev. Biol. 9. doi:10.3389/fcell.2021.716461
Matsuoka, T., and Yashiro, M. (2014). The Role of PI3K/Akt/mTOR Signaling in Gastric Carcinoma. Cancers 6, 1441–1463. doi:10.3390/cancers6031441
Meng, S., Zhou, H., Feng, Z., Xu, Z., Tang, Y., Li, P., et al. (2017). CircRNA: Functions and Properties of a Novel Potential Biomarker for Cancer. Mol. Cancer 16. doi:10.1186/s12943-017-0663-2
Meng, J., Chen, S., Han, J.-X., Qian, B., Wang, X.-R., Zhong, W.-L., et al. (2018). Twist1 Regulates Vimentin through Cul2 Circular RNA to Promote EMT in Hepatocellular Carcinoma. Cancer Res. 78, 4150–4162. doi:10.1158/0008-5472.CAN-17-3009
Pamudurti, N. R., Bartok, O., Jens, M., Ashwal-Fluss, R., Stottmeister, C., Ruhe, L., et al. (2017). Translation of CircRNAs. Mol. Cel 66, 9–21. doi:10.1016/j.molcel.2017.02.021
Patel, R. K., and Jain, M. (2012). NGS QC Toolkit: A Toolkit for Quality Control of Next Generation Sequencing Data. PLoS One 7, e30619. doi:10.1371/journal.pone.0030619
Quinlan, A. R., and Hall, I. M. (2010). BEDTools: A Flexible Suite of Utilities for Comparing Genomic Features. Bioinformatics 26, 841–842. doi:10.1093/bioinformatics/btq033
Ritchie, M. E., Phipson, B., Wu, D., Hu, Y., Law, C. W., Shi, W., et al. (2015). Limma powers Differential Expression Analyses for RNA-Sequencing and Microarray Studies. Nucleic Acids Res. 43, e47. doi:10.1093/nar/gkv007
Saberi Anvar, M., Minuchehr, Z., Shahlaei, M., and Kheitan, S. (2018). Gastric Cancer Biomarkers; A Systems Biology Approach. Biochem. Biophys. Rep. 13, 141–146. doi:10.1016/j.bbrep.2018.01.001
Shannon, P., Markiel, A., Ozier, O., Baliga, N. S., Wang, J. T., Ramage, D., et al. (2003). Cytoscape: A Software Environment for Integrated Models of Biomolecular Interaction Networks. Genome Res. 13, 2498–2504. doi:10.1101/gr.1239303
Sollott, M. A. A. S. C. M. J. (2017). 乳鼠心肌提取 HHS Public Access. Physiol. Behav. 176, 139–148. doi:10.1158/0008-5472.CAN-16-1883
Sun, X., Zhang, K., and Li, D. (2021). Prognostic Potential of miR-21-3p in Gastric Cancer. J. BUON 25, 2678.
Tang, Z., Kang, B., Li, C., Chen, T., and Zhang, Z. (2019). GEPIA2: an Enhanced Web Server for Large-Scale Expression Profiling and Interactive Analysis. Nucleic Acids Res. 47, W556–W560. doi:10.1093/nar/gkz430
Tucker, D., Zheng, W., Zhang, D.-H., and Dong, X. (2020). Circular RNA and its Potential as Prostate Cancer Biomarkers. Wjco 11, 563–572. doi:10.5306/wjco.v11.i8.563
Wang, K.-W., and Dong, M. (2019). Role of Circular RNAs in Gastric Cancer: Recent Advances and Prospects. Wjgo 11, 459–469. doi:10.4251/wjgo.v11.i6.459
Wu, X.-L., Cheng, B., Li, P. Y., Huang, H. J., Zhao, Q., Dan, Z. L., et al. (2013). MicroRNA-143 Suppresses Gastric Cancer Cell Growth and Induces Apoptosis by Targeting COX-2. Wjg 19, 7758. doi:10.3748/wjg.v19.i43.7758
Xu, Y.-H., Zhang, M., Dong, B.-b., Lu, M., Zheng, M.-j., Ding, J.-z., et al. (2016). miR-429 Functions as a Tumor Suppressor by Targeting FSCN1 in Gastric Cancer Cells. Ott 9, 1123–1133. doi:10.2147/OTT.S91879
Xu, T., Wu, J., Han, P., Zhao, Z., and Song, X. (2017). Circular RNA Expression Profiles and Features in Human Tissues: A Study Using RNA-Seq Data. BMC Genomics 18. doi:10.1186/s12864-017-4029-3
Yu, J., Xu, Q.-g., Wang, Z.-g., Yang, Y., Zhang, L., Ma, J.-z., et al. (2018). Circular RNA cSMARCA5 Inhibits Growth and Metastasis in Hepatocellular Carcinoma. J. Hepatol. 68, 1214–1227. doi:10.1016/j.jhep.2018.01.012
Zang, J., Lu, D., and Xu, A. (2020). The Interaction of circRNAs and RNA Binding Proteins: An Important Part of circRNA Maintenance and Function. J. Neurosci. Res. 98, 87–97. doi:10.1002/jnr.24356
Zhang, M., Zhao, K., Xu, X., Yang, Y., Yan, S., Wei, P., et al. (2018). A Peptide Encoded by Circular Form of LINC-PINT Suppresses Oncogenic Transcriptional Elongation in Glioblastoma. Nat. Commun. 9, 1123–1133. doi:10.1038/s41467-018-06862-2
Zhou, J., Fan, X., Chen, N., Zhou, F., Dong, J., Nie, Y., et al. (2015). Identification of CEACAM5 as a Biomarker for Prewarning and Prognosis in Gastric Cancer. J. Histochem. Cytochem. 63, 922–930. doi:10.1369/0022155415609098
Zhou, K., Song, B., Wei, M., Fang, J., and Xu, Y. (2020). MiR-145-5p Suppresses the Proliferation, Migration and Invasion of Gastric Cancer Epithelial Cells via the ANGPT2/NOD_LIKE_RECEPTOR axis. Cancer Cel Int 20. doi:10.1186/s12935-020-01483-6
Keywords: gastric cancer, circular RNA, computational approach, circRNA biomarker, circRNA-miRNA-gene interaction
Citation: Hossain MT, Li S, Reza MS, Feng S, Zhang X, Jin Z, Wei Y and Peng Y (2022) Identification of circRNA Biomarker for Gastric Cancer through Integrated Analysis. Front. Mol. Biosci. 9:857320. doi: 10.3389/fmolb.2022.857320
Received: 18 January 2022; Accepted: 07 February 2022;
Published: 10 March 2022.
Edited by:
Hem Chandra Jha, Indian Institute of Technology Indore, IndiaReviewed by:
Tae Jin Lee, Augusta University, United StatesDinesh Yadav, University of Alabama at Birmingham, United States
Copyright © 2022 Hossain, Li, Reza, Feng, Zhang, Jin, Wei and Peng. This is an open-access article distributed under the terms of the Creative Commons Attribution License (CC BY). The use, distribution or reproduction in other forums is permitted, provided the original author(s) and the copyright owner(s) are credited and that the original publication in this journal is cited, in accordance with accepted academic practice. No use, distribution or reproduction is permitted which does not comply with these terms.
*Correspondence: Yin Peng, eXBlbmdAc3p1LmVkdS5jbg==; Yanjie Wei, eWoud2VpQHNpYXQuYWMuY24=
†These authors have contributed equally to this work