- 1Department of Urology, The First Affiliated Hospital of Guangxi Medical University, Nanning, China
- 2Center for Genomic and Personalized Medicine, Guangxi Medical University, Nanning, China
- 3Guangxi Collaborative Innovation Center for Genomic and Personalized Medicine, Nanning, China
- 4Guangxi Key Laboratory for Genomic and Personalized Medicine, Guangxi Key Laboratory of Colleges and Universities, Nanning, China
- 5Collaborative Innovation Center of Regenerative Medicine and Medical BioResource Development and Application, Guangxi Medical University, Nanning, China
Background: Toll-like receptors (TLRs) are important initiators of innate and acquired immune responses. However, its role in kidney renal clear cell carcinoma (KIRC) remains unclear.
Methods: TLRs and their relationships with KIRC were studied in detail by ONCOMINE, UALCAN, GEPIA, cBioPortal, GeneMANIA, FunRich, LinkedOmics, TIMER and TRRUST. Moreover, we used clinical samples to verify the expressions of TLR3 and TLR4 in early stage of KIRC by real-time fluorescence quantitative polymerase chain reaction (RT-qPCR), flow cytometry (FC) and immunohistochemistry (IHC).
Results: The expression levels of TLRs in KIRC were generally different compared with adjacent normal tissues. Moreover, the expressions of TLR3 and TLR4 elevated significantly in the early stage of KIRC. Overexpressions of TLR1, TLR3, TLR4 and TLR8 in KIRC patients were associated with longer overall survival (OS), while inhibition of TLR9 expression was related to longer OS. Additionally, overexpressions of TLR1, TLR3 and TLR4 in KIRC patients were associated with longer disease free survival (DFS). There were general genetic alterations and obvious co-expression correlation of TLRs in KIRC. The PPI network between TLRs was rather complex, and the key gene connecting the TLRs interaction was MYD88. The GO analysis and KEGG pathway analysis indicated that TLRs were closely related to adaptive immunity, innate immunity and other immune-related processes. RELA, NFKB1, IRF8, IRF3 and HIF1A were key transcription factors regulating the expressions of TLRs. What’s more, the expression levels of all TLRs in KIRC were positively correlated with the infiltration levels of dendritic cells, macrophages, neutrophils, B cells, CD4+ T cells and CD8+ T cells. Finally, the results of RT-qPCR, FC and IHC confirmed that TLR3 and TLR4 were significantly elevated in the early stage of KIRC.
Conclusion: The occurrence and development of KIRC are closely related to TLRs, and TLRs have the potential to be early diagnostic biomarkers of KIRC and biomarkers for judging the prognosis and immune status of KIRC. This study may provide new insights into the selection of KIRC immunotherapy targets.
Introduction
Renal cell carcinoma (RCC) can be divided into different subtypes according to its histological characteristics with unique genetic and molecular alterations, different clinical processes and therapeutic responses (Linehan et al., 2010; Linehan and Ricketts, 2013; Moch et al., 2016). RCC is a tumor originating from renal epithelium (Sanchez-Gastaldo et al., 2017; Zou and Mo, 2021). The most common pathological subtype of RCC is kidney renal clear cell carcinoma (KIRC), accounting for about 75% of RCC (Linehan and Ricketts, 2019). Lipid accumulation and storage are the main pathological features of KIRC (Xiao et al., 2019), and KIRC is the most common cause of death associated with RCC (Hsieh et al., 2017). Local KIRC can be treated by surgical resection, but the treatment of advanced KIRC is still a clinical challenge, and the 5-years overall survival (OS) rate is 0–20% (Petitprez et al., 2021). Patients with recurrent or distant metastasis of KIRC have a poor prognosis and a short median survival (Zheng et al., 2017), moreover, the prognosis of KIRC patients is mainly based on tumor lymph node metastasis (TNM) stage (Pichler et al., 2013; Qin et al., 2013; Zheng et al., 2017), and there is a lack of biomarkers to determine the prognosis of patients, so the identification of early diagnostic biomarkers for KIRC and biomarkers that can assess patient prognosis are critical to the management and treatment of patients with KIRC.
Toll-like receptors (TLRs) are considered to be the key to identify pathogens and control immune response (Marin-Acevedo et al., 2018). TLRs play a crucial role in both innate and subsequent adaptive immunity because of its ability to sense foreign substances, known as pathogen-associated molecular patterns (PAMPs) (Shetab Boushehri and Lamprecht, 2018). In addition to PAMPs, TLRs can also recognize endogenous ligands. When tissue damage or cell death occurs, cells secrete alarmins, also known as danger-associated molecular patterns (DAMPs), but excessive release of the substance is associated with autoimmune diseases and cancer (Chan et al., 2012; Zhao et al., 2014; Urban-Wojciuk et al., 2019). TLRs are expressed in a variety of cells, including immune cells, fibroblasts, and epithelial cells, and their primary role is to protect the host against microbial infection (Kawasaki and Kawai, 2014; Chen et al., 2018). More and more studies have shown that TLRs also play an important role in the occurrence and development of cancer (Wang et al., 2018; Wu et al., 2018), and different TLRs play different roles in different cancers (Dajon et al., 2017). However, the effect of TLRs on KIRC and its mechanism are not clear. In this study, we systematically investigated the expressions of TLRs in KIRC by ONCOMINE, UALCAN and GEPIA databases, and analyzed the relationships between the expressions of TLRs and tumor stage and prognosis in patients with KIRC by GEPIA. In addition, we obtained the genetic alteration information of TLRs and spearman’s correlation of co-expression between TLRs through cBioPortal. We explored the protein-protein interaction (PPI) network of TLRs by GeneMANIA, and obtained the most critical gene associated with TLRs by FunRich, and conducted detailed GO analysis and KEGG pathway analysis of TLRs through LinkedOmics database. At the same time, we investigated the key transcription factors regulating TLRs through TRRUST. What’s more, we studied the relationships between the expression levels of TLRs in KIRC and the levels of immune cell infiltration by TIMER, and evaluated the effects of TLRs and immune cell infiltration on the survival risk of KIRC. Finally, we used clinical samples to verify the expressions of TLR3 and TLR4 in early stage of KIRC by RT-qPCR, flow cytometry (FC) and immunohistochemistry (IHC). Our study provides new insights into TLRs and their relationships with KIRC, contributing to the research of early diagnosis and therapeutic targets of KIRC.
Materials and Methods
ONCOMINE
ONCOMINE is a powerful bioinformatics tool for genome-wide expression analysis (Rhodes et al., 2004). The expressions of TLRs in renal cell carcinoma were evaluated by ONCOMINE, so as to speculate the expressions of TLRs in KIRC. In this study, we used the following criteria: p value as 0.05, gene rank as top 10%, fold change as 2 and data type as all (DNA and mRNA).
UALCAN
UALCAN is a website for mining TCGA and MET500 cohort data. It has a variety of functions, including evaluating the expressions of different genes in different cancers and the effect of gene expression on cancer survival (Chandrashekar et al., 2017). Through the “TCGA Gene Analysis” of the UALCAN, this study explored the expressions of TLRs in KIRC compared with normal tissues.
GEPIA
GEPIA is a website that can be used to analyze RNA expression levels in a variety of tumors and corresponding normal tissues. It also has many functions such as evaluating the effect of different RNA expression levels on the prognosis of cancer (Tang et al., 2017). In this study, we compared the expressions of TLRs mRNA in KIRC and corresponding normal tissues, as well as the expression levels of TLRs in different stage of KIRC through the “Expression DIY” module of GEPIA. Additionally, we also investigated the effects of TLRs on the survival of patients with KIRC through the “Survival” module.
cBioPortal
cBioPortal is a powerful website for analyzing multidimensional cancer genome data. It can visualize data from cancer tissues and cells into easy-to-understand genetic and gene co-expression events (Gao et al., 2013). Based on the TCGA database of 538 cases of renal clear cell carcinoma (TCGA, Firehose Legacy), the genetic alteration and co-expression of TLRs were obtained from cBioPortal, the threshold of Protein expression z-scores (RPPA) and mRNA expression z scores (RNA Seq V2 RSEM) was set to ±2.0.
GeneMANIA
GeneMANIA is a convenient and versatile website for analyzing PPI, co-expression, pathways and related functions (Warde-Farley et al., 2010). This study studied the related functions of TLRs and its PPI network through GeneMANIA.
FunRich
FunRich (3.1.3 exe), a bioinformatics tool, can perform analysis of multiple genes or proteins data sets provided (Fonseka et al., 2020). Through FunRich, we obtained the most critical gene associated with TLRs, and carried out relevant research on this gene.
LinkedOmics
LinkedOmics is a tool that can be used to analyze the multi-omics data of 32 cancer types from TCGA (Vasaikar et al., 2018). In this study, “GO analysis” and “KEGG Pathway” enrichment analysis of TLRs were carried out using the “Gene Set Enrichment Analysis” tool in “LinkInterpreter” module. We set the “Rank Criteria” as meta p-value, the “Minimum Number of Genes Size” as 3 and the “Simulations” as 500. Statistical analysis was conducted using Person Correlation Test.
TIMER
TIMER, a reliable utility, allows users to enter specific parameters to systematically assess immune cell infiltration in different tumors and its impact on clinical outcomes (Li et al., 2017). In this study, the correlations between the expressions of TLRs and the levels of immune cell infiltration in KIRC were evaluated using the “Gene” module, and the correlations between the clinical prognosis of KIRC and the expressions of TLRs and immune cells were evaluated by “Survival” module.
TRRUST
TRRUST is a powerful tool for querying transcription factors that regulate gene expression, and can provide regulatory information on the interaction of many transcription factors in humans and mice (Han et al., 2018). In this study, TRRUST was used to query the transcription factors related to regulating TLRs.
Tissue Collection
Six KIRC tumor tissues and adjacent nontumor tissues were obtained from The First Affiliated Hospital of Guangxi Medical University. The study was approved by the Ethics Committee of The First Affiliated Hospital of Guangxi Medical University (Approval Number: 2021KY-E-182) and all the participants in the experiment gave their informed consent.
Real-Time Fluorescence Quantitative Polymerase Chain Reaction
Total RNA was extracted by Total RNA Kit I (R6834, Omega). According to the instructions for the use of PrimeScript RT reagent kit (RR036a, Takara, Kyoto, Japan). RNA was reverse transcribed into cDNA, and then cDNA was detected by RT-qPCR using FastStart Essential DNA Green Master (06,924,204,001, Roche) and LightCycler® 96 Instrument (Roche). Three repeated assays were set for each sample. Using glyceraldehyde-3-phosphate dehydrogenase (GADPH) as internal reference, the relative expressions of target genes were analyzed by 2−ΔΔCt method. The primer sequences of TLR3 were as follows: 5′-TTGCCTTGTATCTACTTTTGGGG-3' (Forward); 5′-TCAACACTGTTATGTTTGTGGGT-3' (Reverse). The primer sequences of TLR4 were as follows: 5′-AGACCTGTCCCTGAACCCTAT-3' (Forward); 5′-CGATGGACTTCTAAACCAGCCA-3' (Reverse).
Flow Cytometry
Flow cytometry (FC) was used to analyze the expressions of TLR3 and TLR4 in KIRC relative to adjacent nontumor tissues. Fresh human KIRC tissue and adjacent nontumor tissue samples were collected in the First Affiliated Hospital of Guangxi Medical University and prepared into single cell suspension. Firstly, all tissues were cut into small pieces with scissors. After washing twice by D-PBS (311–425-CL, Wisent), the sample was transferred to digestive juice (0.1 mg/ml collagenase I (10,103,578,001, Roche) and 1 mg/ml dnase I (10,104,159,001, Roche) in HBSS (14,025,092, Gibco) solution) and gently shaken at 37°C for 30 min. Digestion was terminated using 10% fetal bovine serum (FBS; SH30070.03, HyClone) in RPMI 1640 and the disaggregated cell suspensions were passed through a 100 μm cell strainer (352,350, Falcon). The cell suspensions were washed thoroughly with D-PBS containing 1% FBS. Red blood cells were eliminated by 1X red blood cell (RBC) lysis buffer (420,301, BioLegend) for 5 min on ice and lysis was terminated by dilution with D-PBS containing 1% FBS, filtered through a 40 μm cell strainer (352,340, Falcon). Then, the cell suspensions were washed thoroughly with D-PBS containing 1% FBS. Finally, cells obtained by centrifugation were resuspended using PBS. Then, single cell suspensions of normal and cancer tissues of kidney were transferred to Fixation Buffer (420,801, Biolegend) for 20 min at room temperature and dark. Precipitation obtained by centrifugation were washed two times by 1× Intracellular Staining Perm Wash Buffer (421,002, Biolegend). Then, about 106 cells were incubated with TLR3 (bs-1444R, Bioss) or TLR4 (bs-20594R, bioss) diluted by 1× Intracellular Staining Perm Wash Buffer for 20 min at room temperature. Cells were washed two times by 1× Intracellular Staining Perm Wash Buffer. The cells were then incubated with Alexa Fluor 488-conjugated donkey anti-rabbit IgG antibodies (1:2000, ab150061, Abcam) for 20 min at room temperature and dark. After washed twice, the cells were resuspended in PBS for flow cytometry analysis. All samples were loaded on a BD C6 Plus for flow cytometry analysis. The data were analyzed using flowjo V10.0.
Immunohistochemistry
Three cases of KIRC tissues and adjacent nontumor tissues from the First Affiliated Hospital of Guangxi Medical University were collected. After fixation with 4% paraformaldehyde for 24 h, paraffin embedding and section were performed. The sections were dewaxed and hydrated using xylene and gradient alcohol. Then, the sections were treated with EDTA at pH 8.5 (C1034, Solarbio) to induce epitope retrieval by heating. After washing three times with PBS, the sections were incubated with an endogenous peroxidase inhibitor (SP-9001, ZSGB-BIO) for 10 min. Then the sections were washed with PBS three times and incubated with normal goat serum blocking solution (SP-9001, ZSGB-BIO) for 15 min. Primary antibodies (TLR3, 1:200; TLR4, 1:200) were incubated overnight at 4°C. After 30 min of room temperature balance the next day, incubated with Biotinylated Second Antibody (SP-9001, ZSGB-BIO) for 15 min. Then the sections were washed with PBS three times and incubated with Streptavidin-Enzyme Conjugate (SP-9001, ZSGB-BIO) for 15 min. After washing three times with PBS, the sections were incubated with DAB chromogenic fluid (ZLI-9018, ZSGB-BIO) for 5 min. Finally, after redyeing with hematoxylin, the slices were fixed with neutral gum. The images were captured using microscope (Olympus, CX23) and then processed with ImageJ software (NIH).
Statistical Analysis
All experimental data were statistically analyzed using GraphPad Prism 7. t-test was used to analyze the expressions of TLR3 and TLR4 in KIRC tissues relative to adjacent nontumor tissues. In this paper, p < 0.05 was considered statistically significant.
Results
Toll-Like Receptors Expression Levels in Renal Cell Carcinoma and Adjacent Nontumor Tissues
Expressions of TLRs in renal cell carcinoma relative to adjacent nontumor tissues were retrieved from ONCOMINE database. The results demonstrated that the expressions of TLR1, TLR2, TLR3, TLR4, TLR7 and TLR8 were significantly elevated, while the expression of TLR5 was significantly decreased in RCC tissues (Figure 1). We also evaluated the transcript expression levels of TLRs in KIRC by UALCAN. Compared with nontumor tissues, the expressions of TLR1 (p = 5.52E-05), TLR2 (p < 1E-12), TLR3 (p = 1.62E-12), TLR4 (p = 5.63E-07), TLR6 (p = 1.62E-12), TLR7 (p < 1E-12), TLR8 (p = 1.62E-12), TLR9 (p = 1.62E-10) and TLR10 (p = 1.62E-12) transcripts in KIRC were significantly elevated, while the transcript expression level of TLR5 was significantly decreased (Figure 2). Meanwhile, we also used GEPIA to compare the relative expression levels of all TLRs in KIRC tissues. The results showed that the TLR3 expression was the highest compared with other TLRs in KIRC tissues (Figure 3). To further identify TLRs associated with the occurrence, progression, and clinical prognosis of KIRC, we evaluated TLRs expression levels at different pathological stages of KIRC. We found that there were significant correlations of TLR3 (p = 0.008) and TLR4 (p = 0.001) expressions with the pathological stages of KIRC, while there were no significant correlations in the expressions of other TLRs at different pathological stages of KIRC (Figure 4). The expressions of TLR3 and TLR4 elevated significantly in the early stage of KIRC, indicating that TLR3 and TLR4 played an important role in the early diagnosis of KIRC. In addition, all these data indicated that TLRs played a momentous influence in the occurrence and progression of KIRC.
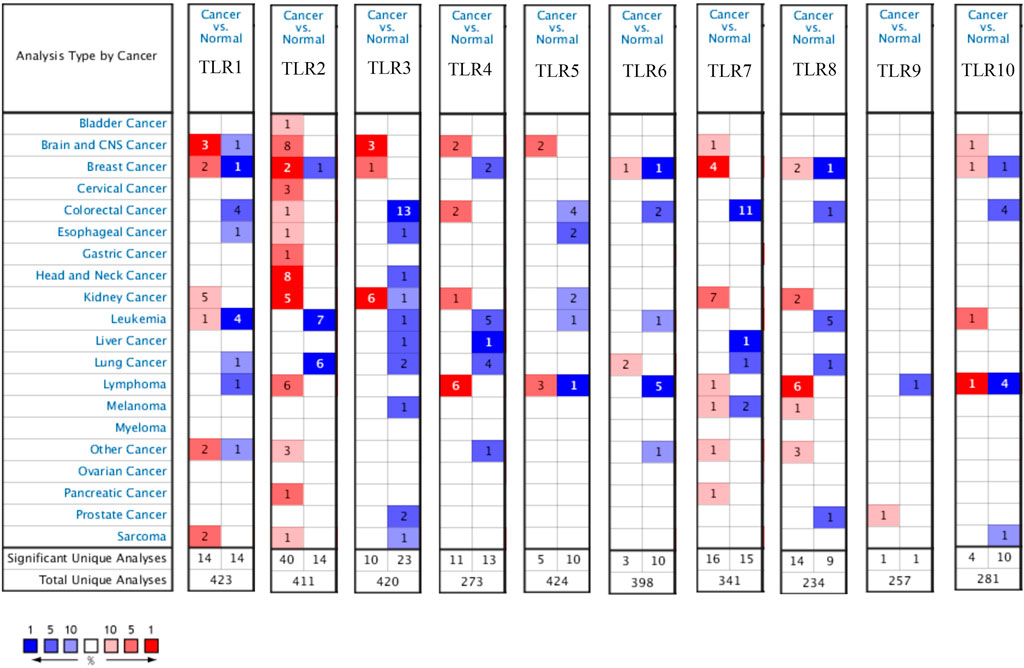
FIGURE 1. Expression levels of TLRs in kidney cancer. The figure showing expression profiles of TLRs in tumor and paired normal tissue samples from the ONCOMINE database.
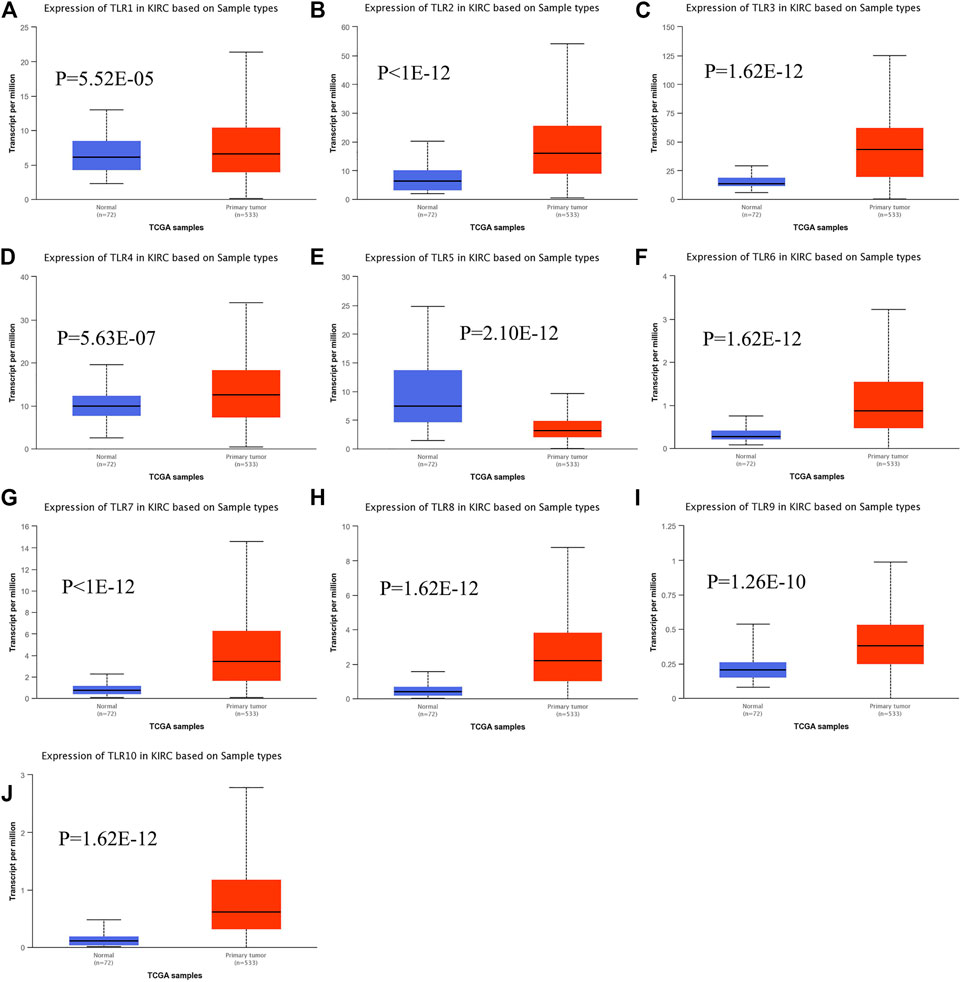
FIGURE 2. The transcript expression levels of TLRs in KIRC. Box plots showing the transcript expression levels of TLR1 (A), TLR2 (B), TLR3 (C), TLR4 (D), TLR5 (E), TLR6 (F), TLR7 (G), TLR8 (H), TLR9 (I) and TLR10 (J) in KIRC compared with normal tissues.
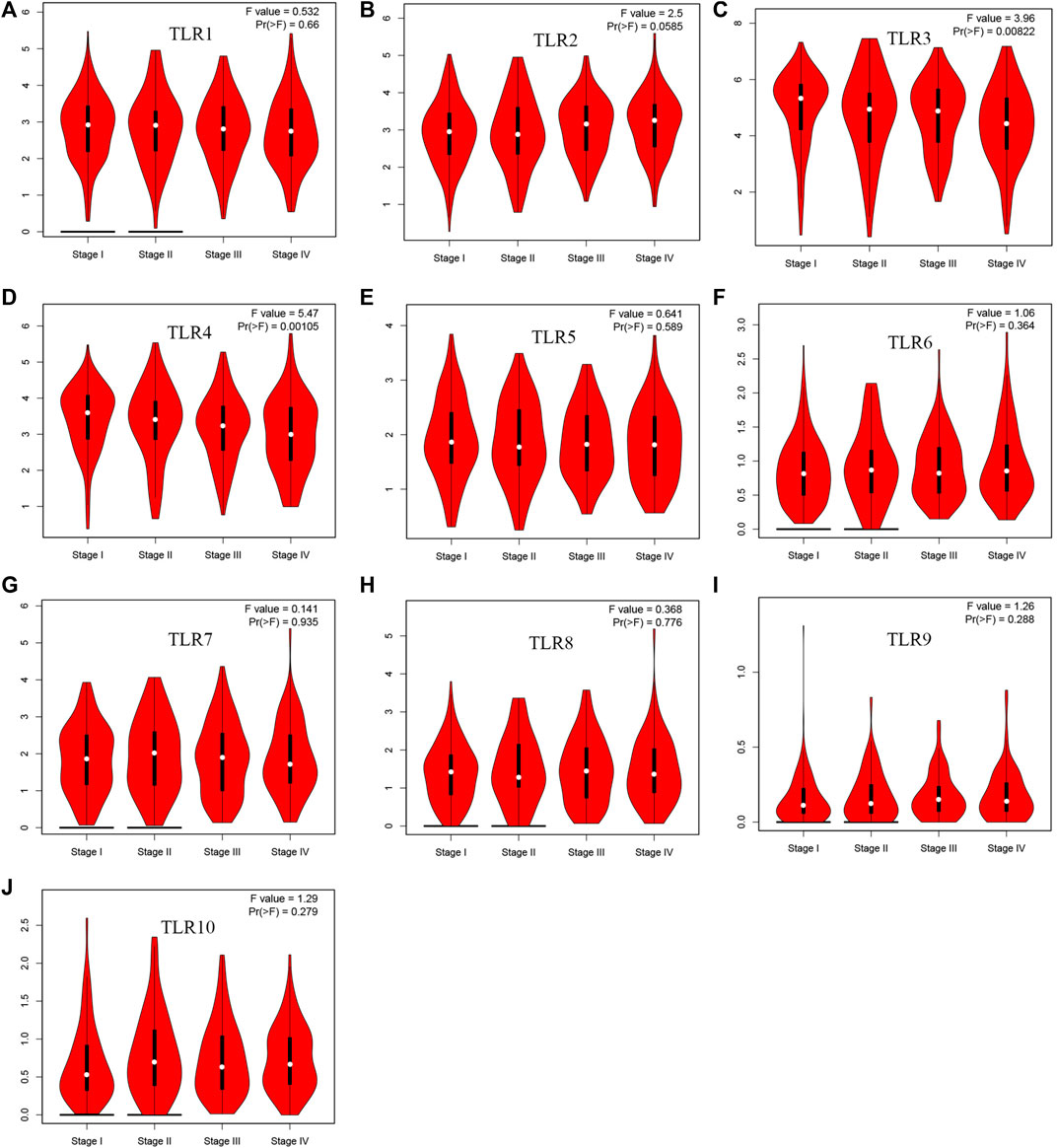
FIGURE 4. The association of TLRs expression levels with different pathological stages of KIRC. (A) TLR1, (B) TLR2, (C) TLR3, (D) TLR4, (E) TLR5, (F) TLR6, (G) TLR7, (H) TLR8, (I) TLR9, (J) TLR10.
The Effects of Toll-Like Receptors on the Prognosis of Kidney Renal Clear Cell Carcinoma
In order to evaluate the effects of TLRs on the prognostic value of KIRC, we used GEPIA to assess the correlations of TLRs with the disease free survival (DFS) and overall survival (OS) of KIRC (Figures 5, 6). High expressions of TLR1 (p = 0.018), TLR3 (p = 2.6e-09), TLR4 (p = 5.4e-05) and TLR8 (p = 0.035) in patients with KIRC were associated with longer OS (Figures 5A,C,D,H), while low expression of TLR9 (p = 0.018) in patients with KIRC was associated with longer OS (Figure 5I). Moreover, we found that high expressions of TLR1 (p = 0.017), TLR3 (p = 0.00013) and TLR4 (p = 0.00078) in patients with KIRC were associated with longer DFS (Figures 6A,C,D), but there were no significant correlations between the expressions of other TLRs and the DFS of KIRC (Figure 6).
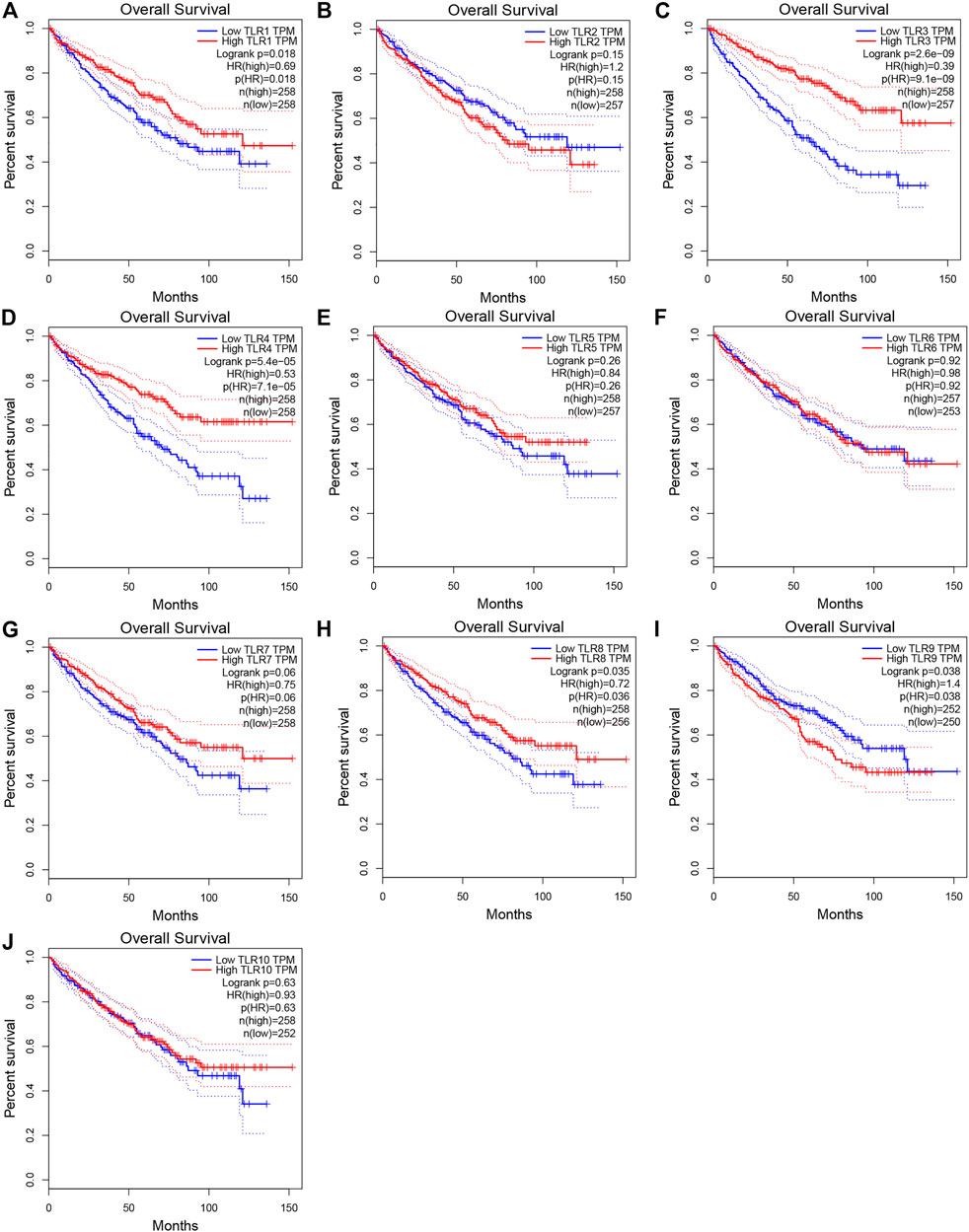
FIGURE 5. Effects of TLRs on overall survival time of patients with KIRC. (A) TLR1, (B) TLR2, (C) TLR3, (D) TLR4, (E) TLR5, (F) TLR6, (G) TLR7, (H) TLR8, (I) TLR9, (J) TLR10.
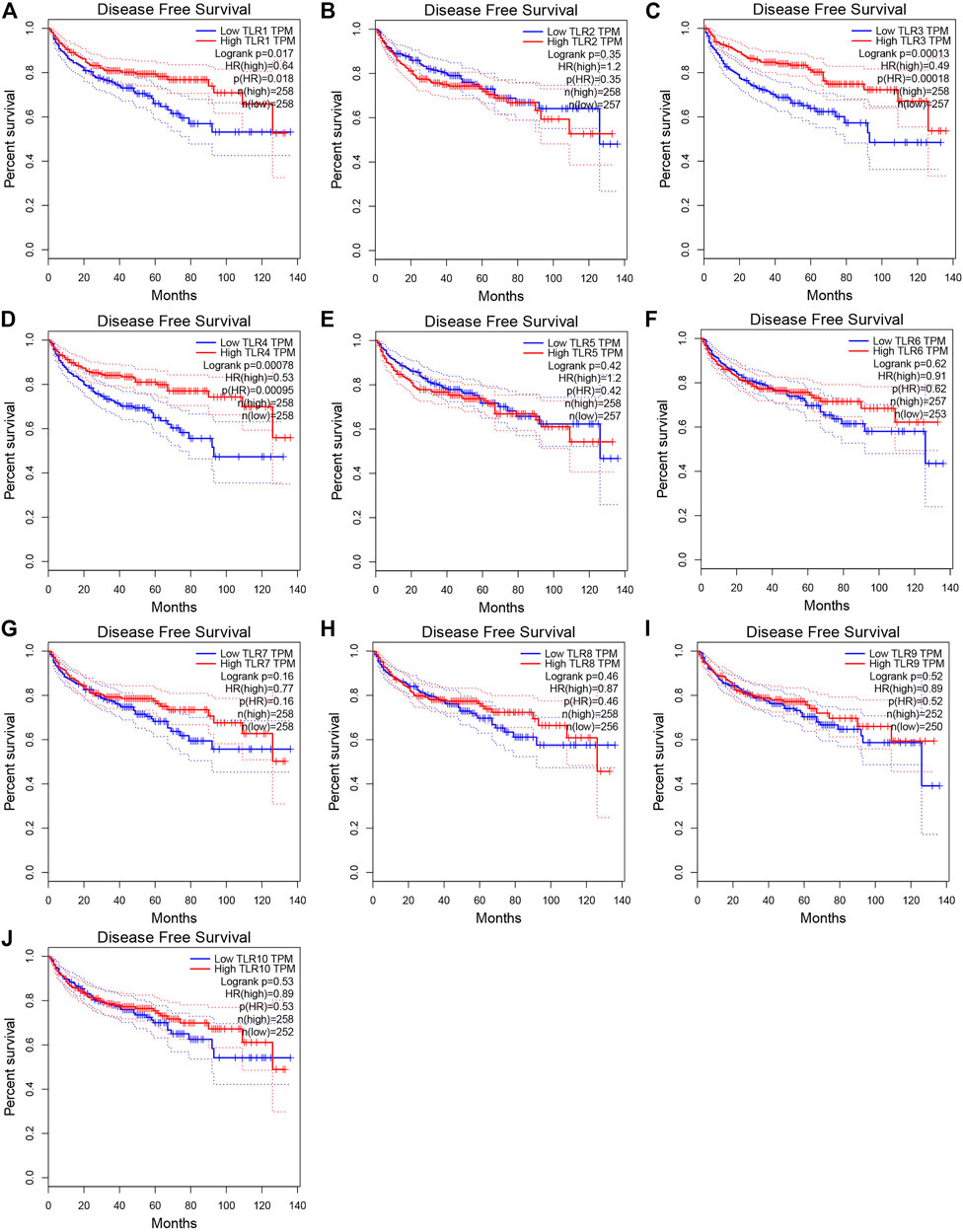
FIGURE 6. Effects of TLRs on disease free survival time of patients with KIRC. (A) TLR1, (B) TLR2, (C) TLR3, (D) TLR4, (E) TLR5, (F) TLR6, (G) TLR7, (H) TLR8, (I) TLR9, (J) TLR10.
Analyses of Genetic Altetation, Co-Expression and Protein-Protein Interaction of Toll-Like Receptors in Patients With Kidney Renal Clear Cell Carcinoma
Next, we systematically analyzed the molecular characteristics of TLRs in patients with KIRC. First of all, we analyzed the genetic alterations and co-expression of TLRs in 538 KIRC patients using cBioPortal. In the KIRC samples, the results showed that the altered/profiled ratio of TLR1, TLR2, TLR3, TLR4, TLR5, TLR6, TLR7, TLR8, TLR9 and TL10 is 5, 4, 6, 3, 5, 5, 4, 4, 12 and 5%, respectively (Figure 7A). High mRNA expression and deep deletion were the most common changes in these samples. Next, we explored the spearman’s correlation of co-expression among TLRs. The results showed that there were general positive correlations between TLRs co-expression (Table 1). Not only that, we also analyzed the PPI network between TLRs through GeneMANIA. The functions of these TLRs were mainly related to toll-like receptor signaling pathway, pattern recognition receptor signaling pathway, innate immune response-activating signal transduction, activation of innate immune response, positive regulation of innate immune response, positive regulation of defense response and regulation of innate immune response (Figure 7B). Next, we further studied the major genes that interacted with TLRs through FunRich. The results showed that MYD88 was the key gene to connect the interaction between TLRs (Figure 7C), indicating that MYD88 plays a crucial role in the expressions of TLRs.
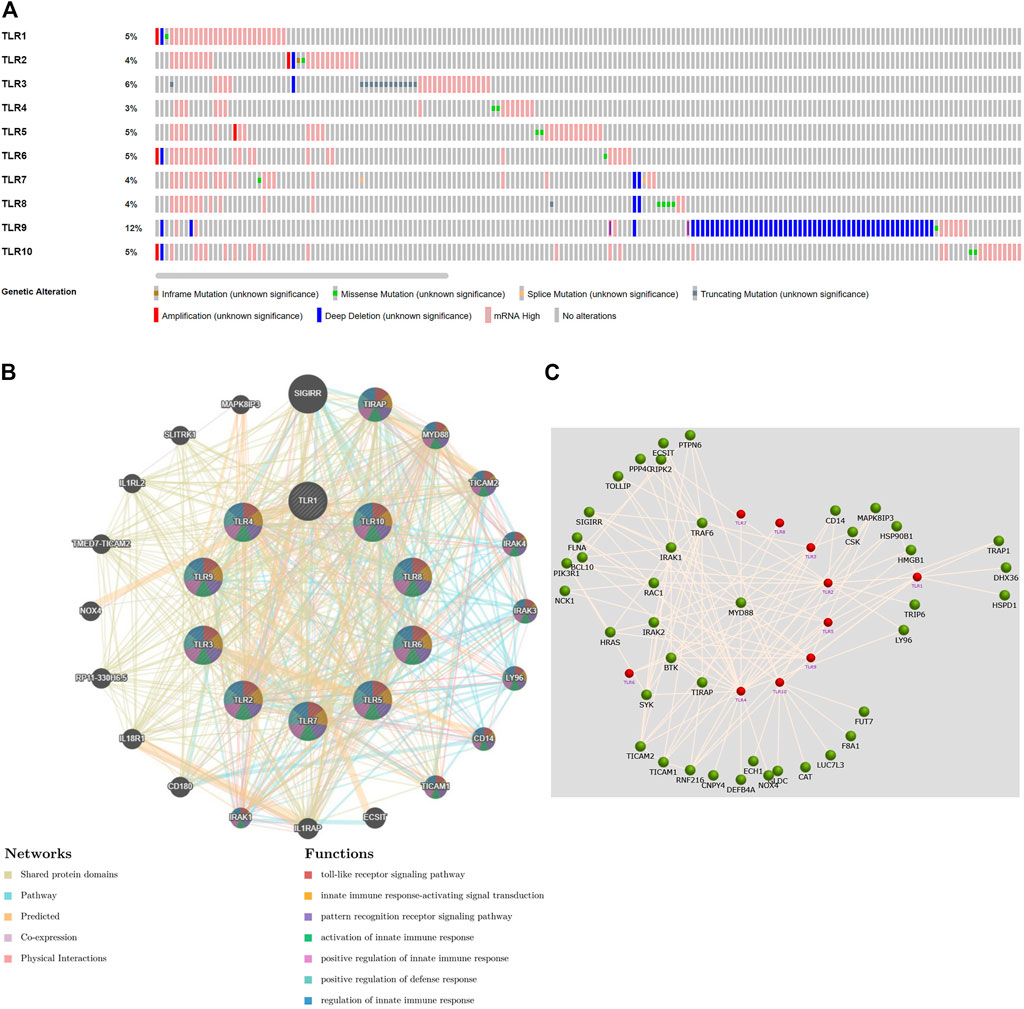
FIGURE 7. Genetic alteration, function enrichment of PPI network, genetic interaction analyses of TLRs in KIRC patients. (A) Genetic alteration of different TLRs in KIRC. (B) Functional enrichment of TLRs-related PPI network in KIRC. (C) The key gene connecting the TLRs interaction was MYD88.
Gene Set Enrichment Analysis of Toll-Like Receptors in Kidney Renal Clear Cell Carcinoma Patients
LinkedOmics was used for gene enrichment analysis of TLRs. We studied TLRs-related GO analysis and KEGG pathway. Many biological processes (BP) of significant enrichment of TLRs were closely related to the occurrence and development of KIRC, including adaptive immune response, regulation of leukocyte activation, immune response-regulating signaling pathway, lymphocyte mediated immunity, leukocyte cell-cell adhesion, positive regulation of cytokine production, interferon-gamma production, regulation of immune effector process, regulation of cell-cell adhesion, positive regulation of defense response, leukocyte differentiation and lymphocyte activation involved in immune response (Figure 8A). In addition, side of membrane, secretory granule membrane, tertiary granule, receptor complex, specific granule, endocytic vesicle, membrane region, mast cell granule, cell leading edge, MHC protein complex, protein complex involved in cell adhesion, neuron spine, immunological synapse, PML body, chromosomal region, phagocytic cup and ficolin-1-rich granule were the most obviously enriched projects in the cellular components (CC) (Figure 8B). The molecular functions (MF) involved in the enrichment of TLRs mainly included cytokine binding, antigen binding, cytokine receptor activity, peptide receptor activity, cytokine receptor binding, MHC protein binding, SH3 domain binding, coreceptor activity, SH2 domain binding, lipopolysaccharide binding, carbohydrate binding and purinergic receptor activity (Figure 8C). Among the TLRs-enriched KEGG pathway, Th17 cell differentiation, Th1 and Th2 cell differentiation, Toll-like receptor signaling pathway, TNF signaling pathway, JAK-STAT signaling pathway and cell adhesion molecules were significantly correlated with the tumorigenesis of KIRC (Figure 8D).
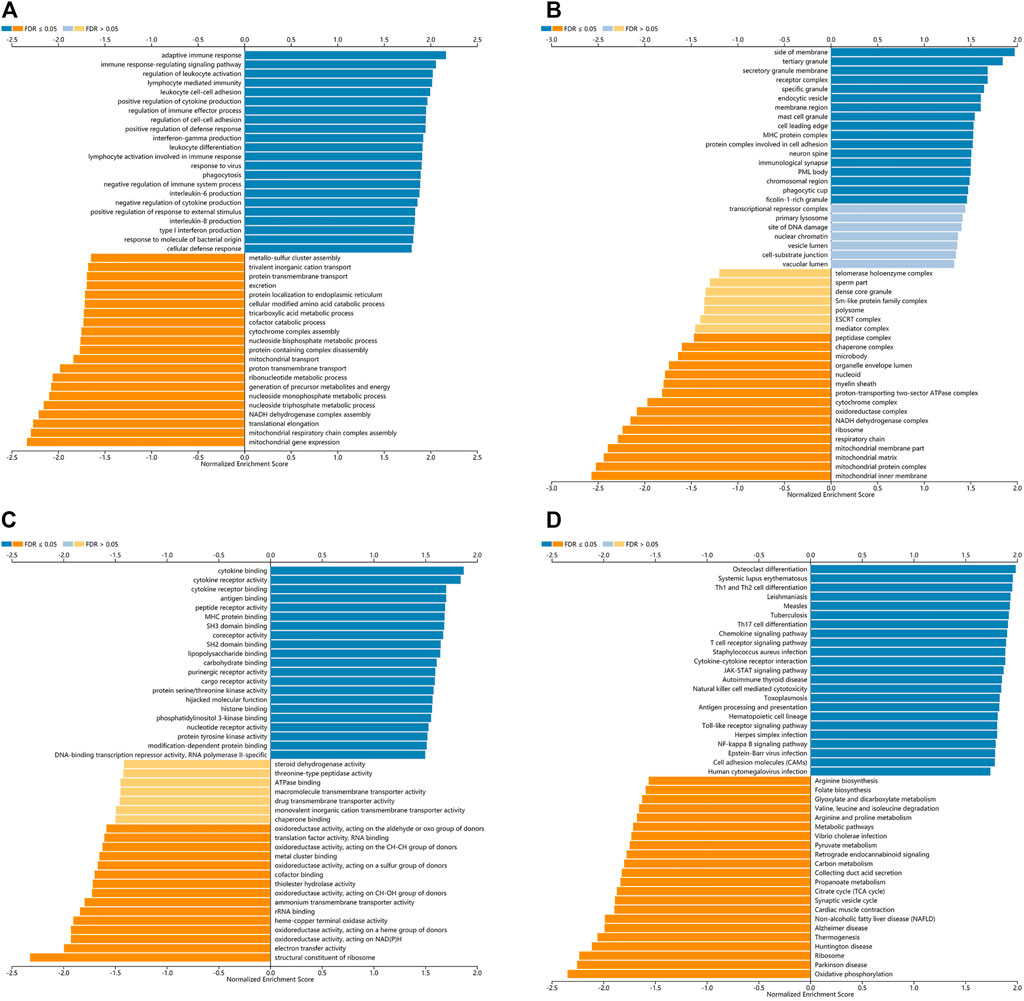
FIGURE 8. The GO and KEGG pathway analyses of TLRs in KIRC. (A) Biological processes involved in TLRs in KIRC. (B) Cellular components involved in TLRs in KIRC. (C) Molecular functions involved in TLRs in KIRC. (D) KEGG pathways involved in TLRs in KIRC.
Key Transcription Factors Associated With Toll-Like Receptors in Kidney Renal Clear Cell Carcinoma Patients
Through TRRUST, we explored the transcription factors that regulated the expression of TLRs in KIRC patients. The results showed that the key transcription factors of TLRs were RELA, NFKB1, IRF8, IRF3 and HIF1A (Table 2). NFKB1 and RELA were key transcription factors that regulated the expressions of TLR2, TLR3, TLR7 and TLR9. IRF3 and IRF8 were key transcription factors that regulated the expressions of TLR3 and TLR4. HIF1A was the key transcription factor that regulated the expressions of TLR2 and TLR6.
Correlations Between the Expressions of Toll-Like Receptors and Immune Cell Infiltration Levels in Kidney Renal Clear Cell Carcinoma Patients
We comprehensively studied the correlations between the expressions of TLRs and the levels of immune cell infiltration in patients with KIRC by TIMER. To our surprise, the expressions of all TLRs in KIRC were positively correlated with the infiltration levels of dendritic cells, neutrophils, B cells, macrophages, CD8+ T cells and CD4+ T cells (Figure 9). Not only that, we also established a cox proportional hazard model of the effects of TLRs and six kinds of immune cells infiltration on patients with KIRC. The results indicated that B cells (coef = −3.497, p = 0.031), CD8+T cells (coef = −1.967, p = 0.026), CD4+T cells (coef = −4.418, p = 0.009), TLR3 (coef = −0.216, p = 0.014), TLR4 (coef = −0.394, p = 0.024) and TLR8 (coef = −0.926, p = 0.002) were negatively associated with the risk of survival in patients with KIRC, while dendritic cells (coef = 4.130, p < 0.001) and TLR9 (coef = 1.311, p < 0.001) were positively associated with the risk of survival in patients with KIRC (Table 3).
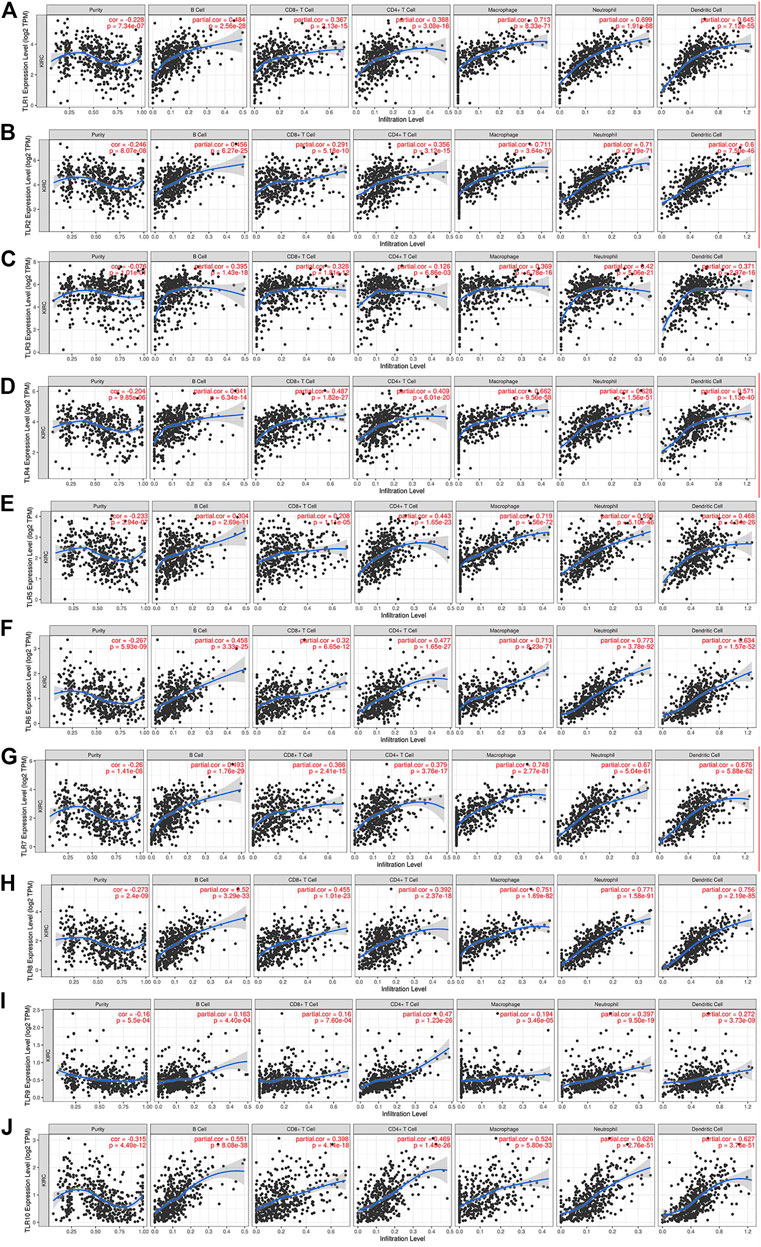
FIGURE 9. The relationships between the expression levels of TLRs and immune cells infiltration in KIRC. The associations of immune cells infiltration levels with the expression levels of TLR1 (A), TLR2 (B), TLR3 (C), TLR4 (D), TLR5 (E), TLR6 (F), TLR7 (G), TLR8 (H), TLR9 (I) and TLR10 (J) in KIRC.
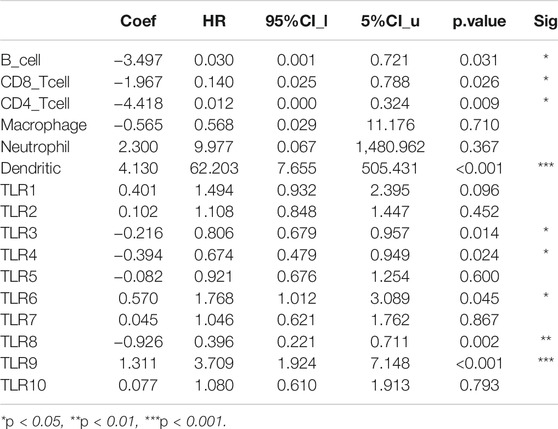
TABLE 3. Cox proportional hazard model of TLRs and 6 kinds of immune cells infiltration in KIRC patients.
Verification the Expressions of TLR3 and TLR4 in Early Stage of Kidney Renal Clear Cell Carcinoma and Adjacent Nontumor Tissues
Finally, we used clinical samples to compare the differences of mRNA and protein expressions of TLR3 and TLR4 in the early stage of KIRC tissues and adjacent nontumor tissues. The characteristics of patients for verifying mRNA and protein expression levels were shown in Tables 4, 5, respectively. The results of RT-qPCR showed that the expression of TLR3 mRNA in KIRC was significantly elevated than that in adjacent nontumor tissues, and TLR4 mRNA also showed the same trend (Figure 10A). What’s more, the results of FC showed that the relative expression of TLR3 at the protein level in KIRC was significantly elevated than that in adjacent nontumor tissues, and the expression of TLR4 at the protein level showed the same trend (Figures 10B,C). In addition, the results of IHC were consistent with the results of RT-qPCR and FC (Figure 10D). Our results confirmed that TLR3 and TLR4 were significantly elevated in the early stage of KIRC compared with adjacent nontumor tissues.
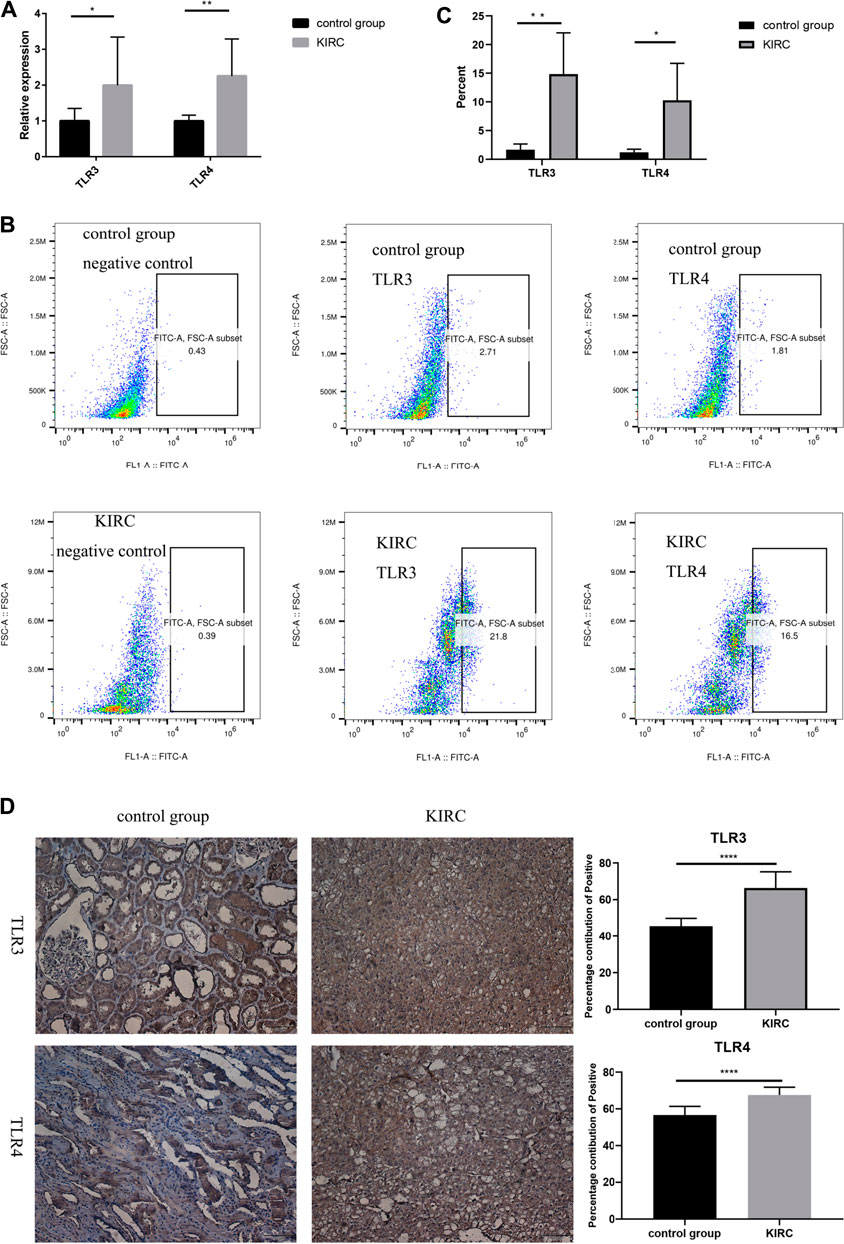
FIGURE 10. Differential expressions of TLR3 and TLR4 in KIRC and adjacent nontumor tissues. (A) Expression levels of TLR3 mRNA and TLR4 mRNA in KIRC relative to adjacent nontumor tissues. (B, C) The proportion of TLR3+ or TLR4+ cells in KIRC and adjacent nontumor tissues was detected by flow cytometry. (D) The percentage contribution of TLR3+ or TLR4+ cells in KIRC and adjacent nontumor tissues was detected by IHC. Control group: adjacent nontumor tissues. *p < 0.05; **p < 0.01; ***p < 0.001; ****p < 0.0001.
Discussion
KIRC can be cured in early diagnosis, but when the disease is metastatic, it is the cancer with the worst prognosis in the urinary system (Angulo et al., 2021). Therefore, early diagnosis of KIRC is important for its therapeutic efficacy and prognosis. However, there is currently a lack of clear clinical biomarkers that can be used to diagnose the early stage of KIRC (Siegel et al., 20182018), and prognosis of patients is mainly determined by TNM stage (Pichler et al., 2013; Qin et al., 2013; Zheng et al., 2017). In addition, molecular biomarkers can provide the possibility of accurate prediction of cancer prognosis and early diagnosis (Tamayo et al., 2011). Therefore, the research on molecular biomarkers for early diagnosis and prognosis of KIRC patients could bring great benefits to the majority of KIRC patients and provide a refined management strategy for KIRC patients.
Toll-like receptor (TLRs) are important initiators of innate and acquired immune responses (Zhang et al., 2021). Ten kinds of TLRs have been identified in humans, and they are expressed in varieties of cells, including B cells, T cells and many other non-immune cells (Nouri et al., 2021; Rameshbabu et al., 2021). There is growing evidence that TLRs play a significance role in a variety of pathological processes, including inflammation, tumor, autoimmune diseases, immunotherapy and vaccination (Vidya et al., 2018). Although the studies of the associations between TLRs and cancer have increased in recent years, there are few studies on the effects of TLRs on KIRC.
Therefore, we used multiple databases to study the relationships between TLRs and KIRC. First of all, we preliminarily studied the expressions of TLRs in kidney cancer through ONCOMINE, and found that the expressions of TLR1, TLR2, TLR3, TLR4, TLR7 and TLR8 in kidney cancer were significantly higher than those in the corresponding normal tissues, while the expression of TLR5 was significantly lower in kidney cancer. Moreover, we further studied the expressions of TLRs in KIRC by UALCAN. The results showed that the expressions of TLRs transcripts in KIRC were elevated than that in adjacent nontumor tissues, except for TLR5. We then evaluated the expressions of all TLRs in KIRC tumor tissues by GEPIA and found that TLR3 was particularly highly expressed in KIRC, followed by TLR4 and TLR2, which was consistent with the results of UALCAN database. Then we further evaluated the expression levels of TLRs at different stages of KIRC, and discovered that the expressions of TLR3 and TLR4 were significantly different at different stages, and TLR3 and TLR4 were significantly increased in the early stage of KIRC. Finally, we also used clinical samples to verify that the expressions of TLR3 and TLR4 were significantly elevated in the early stage of KIRC by RT-qPCR, FC and IHC. These results suggested that TLRs were likely to be important biomarkers for early diagnosis of KIRC, especially TLR3 and TLR4.
Next, we continued to study the effects of TLRs on the survival outcome of KIRC. Overexpressions of TLR1, TLR3, TLR4 and TLR8 significantly prolonged the OS in patients with KIRC, while downregulation of TLR9 significantly prolonged the OS. Moreover, Overexpressions of TLR1, TLR3 and TLR4 significantly prolonged the DFS in patients with KIRC. All of these results suggested that TLRs had the potential to become important biomarkers for predicting prognosis in patients with KIRC, especially TLR1, TLR3, and TLR4.
In order to learn more about TLRs and to understand the possible mechanisms of the effects of TLRs on KIRC patients, we investigated the genetic alteration of TLRs and co-expression of TLRs in KIRC using cBioPortal. There were frequent genetic alterations of TLRs in KIRC. Elevated mRNA expression and deep deletion were the most common changes. Some studies have shown that many factors contribute to the occurrence and development of tumors, and genetic alterations play an indispensable role in this process (Yap et al., 2015; Zeng et al., 2019). What’s more, co-expression of TLRs was found a clear association, suggesting that all of these TLRs play a momentous synergistic role in the occurrence and development of KIRC.
Next, we concentrated on the PPI network, GO analysis and KEGG pathway analysis of TLRs. Not surprisingly, the functions of these TLRs were mainly related to toll-like receptor signaling pathway, activation of innate immune response, pattern recognition receptor signaling pathway, positive regulation of innate immune response, innate immune response-activating signal transduction, positive regulation of defense response and regulation of innate immune response. The GO and KEGG pathway analyses of TLRs indicated that TLRs were mainly associated with regulation of leukocyte activation, immune response-regulating signaling pathway, adaptive immune response, lymphocyte mediated immunity, leukocyte cell-cell adhesion, positive regulation of cytokine production, interferon-gamma production, regulation of immune effector process, regulation of cell-cell adhesion, cytokine binding, positive regulation of defense response, MHC protein complex, protein complex involved in cell adhesion, cytokine receptor activity, cytokine receptor binding, antigen binding, TNF signaling pathway, Th1 and Th2 cell differentiation, Toll-like receptor signaling pathway, Th17 cell differentiation, cell adhesion molecules and JAK-STAT signaling pathway. Some studies have demonstrated that tumorigenesis is closely related to immune dysfunction (Raja et al., 2018; Nakamura et al., 2020). Moreover, our study indicated that TLRs were closely related to adaptive immunity, innate immunity and other immune-related processes, and that genetic alterations in TLRs were very common in KIRC, so we have every reason to believe that the occurrence of KIRC is closely related to TLRs.
Next, through FunRich, we found that the most critical gene that affected the interaction between TLRs was MYD88. Most TLRs depend on MYD88 for the regulation of multiple signal pathways and immune responses (Kim et al., 2019). MyD88 is involved in the development of various cancers by acting downstream of TLRs (Skorka et al., 2021). The results of our study and previous conclusions suggested that MYD88 played a bridging role in human immune homeostasis mediated by TLRs.
In order to learn more about TLRs-related information, we have also explored TLRs-related transcription factors. Our study found that the key transcription factors of TLRs were RELA, NFKB1, IRF8, IRF3 and HIF1A. Previous studies demonstrated that RELA phosphorylation involved in the progression of various diseases including inflammatory disease and cancer by regulating NF-κB signaling (Lu and Yarbrough, 2015) and RELA also played a key role in mediating oncogene-induced aging (Lesina et al., 2016). NFKB1 is a cancer and inflammation inhibitor that plays an inhibitory role in the occurrence and development of a number of cancers by inhibiting the NF-κB signaling pathway (Cartwright et al., 2016; Concetti and Wilson, 2018). In addition, studies have shown that the loss of NFKB1 can lead to inflammation and the progression of cancer by increasing the expression of TNF (Low et al., 2020). IRF8, a tumor suppressor, is also a potential therapeutic option to overcome tumor drug resistance (Wu et al., 2020). IRF3, interferon regulatory factor 3, a tumor suppressor, plays an important role in inhibiting infection and cancer (King et al., 2017; Tian et al., 2020). HIF1A is a hypoxia inducible factor, and its absence increases tumor aggressiveness and metastatic activity (Tiwari et al., 2020). All these results provide insight into the complicated relationship among KIRC, TLRs and transcription factors. In addition, it also provides a further basis for TLRs to become early diagnostic biomarkers and judge the prognosis of patients with KIRC.
The tumor promoting or anticancer effects of TLRs may be related to the tumor microenvironment of immune cell infiltration and the types of cancers (Patra et al., 2020), therefore, we investigated the relationship between the expressions of TLRs in KIRC and the levels of immune cells infiltration by TIMER. Surprisingly, our results showed that the expressions of all TLRs in KIRC were significant positively correlated with the infiltration levels of dendritic cells, macrophages, neutrophils, B cells, CD8+ T cells and CD4+ T cells. There is growing evidence that immune cells infiltration is an important determinant of tumor therapeutic response and clinical outcome (Bindea et al., 2013; Liu et al., 2017). These results, combined with the differential expression of TLRs in KIRC and the significant effects of TLRs expressions on the prognosis of patients with KIRC, indicate that TLRs have the potential to be early diagnostic biomarkers of KIRC and biomarkers for judging the prognosis and immune status of KIRC patients. Further studies are needed to verify our results and explore how TLRs affect the immune microenvironment of KIRC.
Conclusion
In summary, the expression levels of TLRs in KIRC were generally different compared with adjacent normal tissues. Moreover, the expressions of TLR3 and TLR4 elevated significantly in the early stage of KIRC. Different TLRs had different effects on the prognosis of KIRC patients. TLRs can be used as important biomarkers for early diagnosis and prognosis assessment in patients with KIRC, especially TLR3 and TLR4. There were general genetic alterations and obvious co-expression correlation of TLRs in KIRC. The PPI network between TLRs was rather complex, and the key gene connecting the TLRs interaction was MYD88. The GO analysis and KEGG pathway analysis indicated that TLRs were closely related to adaptive immunity, innate immunity and other immune-related processes. RELA, NFKB1, IRF8, IRF3 and HIF1A were key transcription factors regulating the expressions of TLRs. What’s more, the expressions of all TLRs in KIRC were significantly positively correlated with the infiltration levels of dendritic cells, macrophages, neutrophils, B cells, CD8+ T cells and CD4+ T cells. Taken together, the occurrence and development of KIRC is closely related to TLRs, and TLRs have the potential to be early diagnostic biomarkers of KIRC and biomarkers for judging the prognosis and immune status of KIRC patients. The results of our study may provide new insights into the selection of KIRC immunotherapy targets.
Data Availability Statement
The original contributions presented in the study are included in the article/Supplementary Material, further inquiries can be directed to the corresponding author.
Ethics Statement
The studies involving human participants were reviewed and approved by the Ethics Committee of The First Affiliated Hospital of Guangxi Medical University (Approval Number: 2021KY-E-182). The patients/participants provided their written informed consent to participate in this study.
Author Contributions
XZ, BG and ZM contributed to conception and design of the study. XZ and BG performed RT-qPCR, flow cytometry and IHC. XZ, BG QL and ZM performed the statistical analysis. QL collected the samples. XZ and BG wrote the manuscript. All authors have read and approved the manuscript.
Funding
This work was supported by the grants from the National Natural Science Foundation of China (81,770,759), the National Key Research and Development Program of China (2017YFC0908000), Major Project of Guangxi Innovation Driven (AA18118016), Guangxi key Laboratory for Genomic and Personalized Medicine (grant number 16–380-54, 17–259–45, 19–050–22, 19–185–33, 20–065–33), Guangxi Science and Technology base and Talent Project (2019AC17009) and Guangxi Clinical Research Center for Urology and Nephrology (2020AC03006).
Conflict of Interest
The authors declare that the research was conducted in the absence of any commercial or financial relationships that could be construed as a potential conflict of interest.
Publisher’s Note
All claims expressed in this article are solely those of the authors and do not necessarily represent those of their affiliated organizations, or those of the publisher, the editors and the reviewers. Any product that may be evaluated in this article, or claim that may be made by its manufacturer, is not guaranteed or endorsed by the publisher.
References
Angulo, J. C., Manini, C., Lopez, J. I., Pueyo, A., Colas, B., and Ropero, S. (2021). The Role of Epigenetics in the Progression of Clear Cell Renal Cell Carcinoma and the Basis for Future Epigenetic Treatments. Cancers (Basel) 13 (9). doi:10.3390/cancers13092071
Bindea, G., Mlecnik, B., Tosolini, M., Kirilovsky, A., Waldner, M., Obenauf, A. C., et al. (2013). Spatiotemporal Dynamics of Intratumoral Immune Cells Reveal the Immune Landscape in Human Cancer. Immunity 39 (4), 782–795. doi:10.1016/j.immuni.2013.10.003
Cartwright, T., Perkins, N. D., and Wilson, C. L. (2016). NFKB1: a Suppressor of Inflammation, Ageing and Cancer. FEBS J. 283 (10), 1812–1822. doi:10.1111/febs.13627
Chan, J. K., Roth, J., Oppenheim, J. J., Tracey, K. J., Vogl, T., Feldmann, M., et al. (2012). Alarmins: Awaiting a Clinical Response. J. Clin. Invest. 122 (8), 2711–2719. doi:10.1172/JCI62423
Chandrashekar, D. S., Bashel, B., Balasubramanya, S. A. H., Creighton, C. J., Ponce-Rodriguez, I., Chakravarthi, B., et al. (2017). UALCAN: A Portal for Facilitating Tumor Subgroup Gene Expression and Survival Analyses. Neoplasia 19 (8), 649–658. doi:10.1016/j.neo.2017.05.002
Chen, C. Y., Kao, C. L., and Liu, C. M. (2018). The Cancer Prevention, Anti-inflammatory and Anti-oxidation of Bioactive Phytochemicals Targeting the TLR4 Signaling Pathway. Int. J. Mol. Sci. 19 (9), 1. doi:10.3390/ijms19092729
Concetti, J., and Wilson, C. L. (2018). NFKB1 and Cancer: Friend or Foe. Cells 7 (9), 1. doi:10.3390/cells7090133
Dajon, M., Iribarren, K., and Cremer, I. (2017). Toll-like Receptor Stimulation in Cancer: A Pro- and Anti-tumor Double-Edged Sword. Immunobiology 222 (1), 89–100. doi:10.1016/j.imbio.2016.06.009
Fonseka, P., Pathan, M., Chitti, S. V., Kang, T., and Mathivanan, S. (2020). FunRich Enables Enrichment Analysis of OMICs Datasets. J. Mol. Biol. 433, 166747. doi:10.1016/j.jmb.2020.166747
Gao, J., Aksoy, B. A., Dogrusoz, U., Dresdner, G., Gross, B., Sumer, S. O., et al. (2013). Integrative Analysis of Complex Cancer Genomics and Clinical Profiles Using the cBioPortal. Sci. Signal. 6 (269), pl1. doi:10.1126/scisignal.2004088
Han, H., Cho, J. W., Lee, S., Yun, A., Kim, H., Bae, D., et al. (2018). TRRUST V2: an Expanded Reference Database of Human and Mouse Transcriptional Regulatory Interactions. Nucleic Acids Res. 46 (D1), D380–D6. doi:10.1093/nar/gkx1013
Hsieh, J. J., Purdue, M. P., Signoretti, S., Swanton, C., Albiges, L., Schmidinger, M., et al. (2017). Renal Cell Carcinoma. Nat. Rev. Dis. Primers 3, 17009. doi:10.1038/nrdp.2017.9
Kawasaki, T., and Kawai, T. (2014). Toll-like Receptor Signaling Pathways. Front. Immunol. 5, 461. doi:10.3389/fimmu.2014.00461
Kim, Y. C., Lee, S. E., Kim, S. K., Jang, H. D., Hwang, I., Jin, S., et al. (2019). Toll-like Receptor Mediated Inflammation Requires FASN-dependent MYD88 Palmitoylation. Nat. Chem. Biol. 15 (9), 907–916. doi:10.1038/s41589-019-0344-0
King, K. R., Aguirre, A. D., Ye, Y. X., Sun, Y., Roh, J. D., Ng, R. P., et al. (2017). IRF3 and Type I Interferons Fuel a Fatal Response to Myocardial Infarction. Nat. Med. 23 (12), 1481–1487. doi:10.1038/nm.4428
Lesina, M., Wormann, S. M., Morton, J., Diakopoulos, K. N., Korneeva, O., Wimmer, M., et al. (2016). RelA Regulates CXCL1/CXCR2-dependent Oncogene-Induced Senescence in Murine Kras-Driven Pancreatic Carcinogenesis. J. Clin. Invest. 126 (8), 2919–2932. doi:10.1172/JCI86477
Li, T., Fan, J., Wang, B., Traugh, N., Chen, Q., Liu, J. S., et al. (2017). TIMER: A Web Server for Comprehensive Analysis of Tumor-Infiltrating Immune Cells. Cancer Res. 77 (21), e108–e10. doi:10.1158/0008-5472.CAN-17-0307
Linehan, W. M., and Ricketts, C. J. (2019). The Cancer Genome Atlas of Renal Cell Carcinoma: Findings and Clinical Implications. Nat. Rev. Urol. 16 (9), 539–552. doi:10.1038/s41585-019-0211-5
Linehan, W. M., and Ricketts, C. J. (2013). The Metabolic Basis of Kidney Cancer. Semin. Cancer Biol. 23 (1), 46–55. doi:10.1016/j.semcancer.2012.06.002
Linehan, W. M., Srinivasan, R., and Schmidt, L. S. (2010). The Genetic Basis of Kidney Cancer: a Metabolic Disease. Nat. Rev. Urol. 7 (5), 277–285. doi:10.1038/nrurol.2010.47
Liu, X., Wu, S., Yang, Y., Zhao, M., Zhu, G., and Hou, Z. (2017). The Prognostic Landscape of Tumor-Infiltrating Immune Cell and Immunomodulators in Lung Cancer. Biomed. Pharmacother. 95, 55–61. doi:10.1016/j.biopha.2017.08.003
Low, J. T., Christie, M., Ernst, M., Dumoutier, L., Preaudet, A., Ni, Y., et al. (2020). Loss of NFKB1 Results in Expression of Tumor Necrosis Factor and Activation of Signal Transducer and Activator of Transcription 1 to Promote Gastric Tumorigenesis in Mice. Gastroenterology 159 (4), 1444–1458. e15. doi:10.1053/j.gastro.2020.06.039
Lu, X., and Yarbrough, W. G. (2015). Negative Regulation of RelA Phosphorylation: Emerging Players and Their Roles in Cancer. Cytokine Growth Factor. Rev. 26 (1), 7–13. doi:10.1016/j.cytogfr.2014.09.003
Marin-Acevedo, J. A., Dholaria, B., Soyano, A. E., Knutson, K. L., Chumsri, S., and Lou, Y. (2018). Next Generation of Immune Checkpoint Therapy in Cancer: New Developments and Challenges. J. Hematol. Oncol. 11 (1), 39. doi:10.1186/s13045-018-0582-8
Moch, H., Cubilla, A. L., Humphrey, P. A., Reuter, V. E., and Ulbright, T. M. (2016). The 2016 WHO Classification of Tumours of the Urinary System and Male Genital Organs-Part A: Renal, Penile, and Testicular Tumours. Eur. Urol. 70 (1), 93–105. doi:10.1016/j.eururo.2016.02.029
Nakamura, K., Smyth, M. J., and Martinet, L. (2020). Cancer Immunoediting and Immune Dysregulation in Multiple Myeloma. Blood 136 (24), 2731–2740. doi:10.1182/blood.2020006540
Nouri, Y., Weinkove, R., and Perret, R. (2021). T-cell Intrinsic Toll-like Receptor Signaling: Implications for Cancer Immunotherapy and CAR T-Cells. J. Immunother. Cancer 9 (11), e003065. doi:10.1136/jitc-2021-003065
Patra, M. C., Shah, M., and Choi, S. (2020). Toll-like Receptor-Induced Cytokines as Immunotherapeutic Targets in Cancers and Autoimmune Diseases. Semin. Cancer Biol. 64, 61–82. doi:10.1016/j.semcancer.2019.05.002
Petitprez, F., Ayadi, M., de Reyniès, A., Fridman, W. H., Sautès-Fridman, C., and Job, S. (2021). Review of Prognostic Expression Markers for Clear Cell Renal Cell Carcinoma. Front. Oncol. 11, 643065. doi:10.3389/fonc.2021.643065
Pichler, M., Hutterer, G. C., Chromecki, T. F., Jesche, J., Kampel-Kettner, K., Groselj-Strele, A., et al. (2013). Predictive Ability of the 2002 and 2010 Versions of the Tumour-Node-Metastasis Classification System Regarding Metastasis-free, Cancer-specific and Overall Survival in a European Renal Cell Carcinoma Single-centre Series. BJU Int. 111 (4 Pt B), E191–E195. doi:10.1111/j.1464-410X.2012.11584
Qin, C., Sun, L. J., Cui, L., Cao, Q., Zhu, J., Li, P., et al. (2013). Application of the Revised Tumour Node Metastasis (TNM) Staging System of clear Cell Renal Cell Carcinoma in Eastern China: Advantages and Limitations. Asian J. Androl. 15 (4), 550–557. doi:10.1038/aja.2012.161
Raja, J., Ludwig, J. M., Gettinger, S. N., Schalper, K. A., and Kim, H. S. (2018). Oncolytic Virus Immunotherapy: Future Prospects for Oncology. J. Immunother. Cancer 6 (1), 140. doi:10.1186/s40425-018-0458-z
Rameshbabu, S., Labadie, B. W., Argulian, A., and Patnaik, A. (2021). Targeting Innate Immunity in Cancer Therapy. Vaccines 9 (2), 138. doi:10.3390/vaccines9020138
Rhodes, D. R., Yu, J., Shanker, K., Deshpande, N., Varambally, R., Ghosh, D., et al. (2004). ONCOMINE: a Cancer Microarray Database and Integrated Data-Mining Platform. Neoplasia 6 (1), 1–6. doi:10.1016/s1476-5586(04)80047-2
Sanchez-Gastaldo, A., Kempf, E., Gonzalez Del Alba, A., and Duran, I. (2017). Systemic Treatment of Renal Cell Cancer: A Comprehensive Review. Cancer Treat. Rev. 60, 77–89. doi:10.1016/j.ctrv.2017.08.010
Shetab Boushehri, M. A., and Lamprecht, A. (2018). TLR4-Based Immunotherapeutics in Cancer: A Review of the Achievements and Shortcomings. Mol. Pharm. 15 (11), 4777–4800. doi:10.1021/acs.molpharmaceut.8b00691
Siegel, R. L., Miller, K. D., and Jemal, A. (2018). Cancer Statistics. CA Cancer J. Clin. 68 (1), 7–30. doi:10.3322/caac.21442
Skorka, K., Wlasiuk, P., Karczmarczyk, A., and Giannopoulos, K. (2021). Aberrant Expression of TLR2, TLR7, TLR9, Splicing Variants of TLR4 and MYD88 in Chronic Lymphocytic Leukemia Patients. J. Clin. Med. 10 (4). doi:10.3390/jcm10040867
Tamayo, P., Cho, Y. J., Tsherniak, A., Greulich, H., Ambrogio, L., Schouten-van Meeteren, N., et al. (2011). Predicting Relapse in Patients with Medulloblastoma by Integrating Evidence from Clinical and Genomic Features. J. Clin. Oncol. 29 (11), 1415–1423. doi:10.1200/JCO.2010.28.1675
Tang, Z., Li, C., Kang, B., Gao, G., Li, C., and Zhang, Z. (2017). GEPIA: a Web Server for Cancer and normal Gene Expression Profiling and Interactive Analyses. Nucleic Acids Res. 45 (W1), W98–W102. doi:10.1093/nar/gkx247
Tian, M., Wang, X., Sun, J., Lin, W., Chen, L., Liu, S., et al. (2020). IRF3 Prevents Colorectal Tumorigenesis via Inhibiting the Nuclear Translocation of Beta-Catenin. Nat. Commun. 11 (1), 5762. doi:10.1038/s41467-020-19627-7
Tiwari, A., Tashiro, K., Dixit, A., Soni, A., Vogel, K., Hall, B., et al. (2020). Loss of HIF1A from Pancreatic Cancer Cells Increases Expression of PPP1R1B and Degradation of P53 to Promote Invasion and Metastasis. Gastroenterology 159 (5), 1882–1897. e5. doi:10.1053/j.gastro.2020.07.046
Urban-Wojciuk, Z., Khan, M. M., Oyler, B. L., Fahraeus, R., Marek-Trzonkowska, N., Nita-Lazar, A., et al. (2019). The Role of TLRs in Anti-cancer Immunity and Tumor Rejection. Front. Immunol. 10, 2388. doi:10.3389/fimmu.2019.02388
Vasaikar, S. V., Straub, P., Wang, J., and Zhang, B. (2018). LinkedOmics: Analyzing Multi-Omics Data within and across 32 Cancer Types. Nucleic Acids Res. 46 (D1), D956–D63. doi:10.1093/nar/gkx1090
Vidya, M. K., Kumar, V. G., Sejian, V., Bagath, M., Krishnan, G., and Bhatta, R. (2018). Toll-like Receptors: Significance, Ligands, Signaling Pathways, and Functions in Mammals. Int. Rev. Immunol. 37 (1), 20–36. doi:10.1080/08830185.2017.1380200
Wang, L., Yu, K., Zhang, X., and Yu, S. (2018). Dual Functional Roles of the MyD88 Signaling in Colorectal Cancer Development. Biomed. Pharmacother. 107, 177–184. doi:10.1016/j.biopha.2018.07.139
Warde-Farley, D., Donaldson, S. L., Comes, O., Zuberi, K., Badrawi, R., Chao, P., et al. (2010). The GeneMANIA Prediction Server: Biological Network Integration for Gene Prioritization and Predicting Gene Function. Nucleic Acids Res. 38 (Web Server issue), W214–W220. doi:10.1093/nar/gkq537
Wu, H., You, L., Li, Y., Zhao, Z., Shi, G., Chen, Z., et al. (2020). Loss of a Negative Feedback Loop between IRF8 and AR Promotes Prostate Cancer Growth and Enzalutamide Resistance. Cancer Res. 80 (13), 2927–2939. doi:10.1158/0008-5472.CAN-19-2549
Wu, K., Zhang, H., Fu, Y., Zhu, Y., Kong, L., Chen, L., et al. (2018). TLR4/MyD88 Signaling Determines the Metastatic Potential of Breast Cancer Cells. Mol. Med. Rep. 18 (3), 3411–3420. doi:10.3892/mmr.2018.9326
Xiao, W., Xiong, Z., Xiong, W., Yuan, C., Xiao, H., Ruan, H., et al. (2019). Melatonin/PGC1A/UCP1 Promotes Tumor Slimming and Represses Tumor Progression by Initiating Autophagy and Lipid browning. J. Pineal Res. 67 (4), e12607. doi:10.1111/jpi.12607
Yap, N. Y., Rajandram, R., Ng, K. L., Pailoor, J., Fadzli, A., and Gobe, G. C. (2015). Genetic and Chromosomal Aberrations and Their Clinical Significance in Renal Neoplasms. Biomed. Res. Int. 2015, 476508. doi:10.1155/2015/476508
Zeng, Q., Sun, S., Li, Y., Li, X., Li, Z., and Liang, H. (2019). Identification of Therapeutic Targets and Prognostic Biomarkers Among CXC Chemokines in the Renal Cell Carcinoma Microenvironment. Front. Oncol. 9, 1555. doi:10.3389/fonc.2019.01555
Zhang, Y., Liu, J., Wang, C., Liu, J., and Lu, W. (2021). Toll-Like Receptors Gene Polymorphisms in Autoimmune Disease. Front. Immunol. 12, 672346. doi:10.3389/fimmu.2021.672346
Zhao, S., Zhang, Y., Zhang, Q., Wang, F., and Zhang, D. (2014). Toll-like Receptors and Prostate Cancer. Front. Immunol. 5, 352. doi:10.3389/fimmu.2014.00352
Zheng, J., Wang, L., Peng, Z., Yang, Y., Feng, D., and He, J. (2017). Low Level of PDZ Domain Containing 1 (PDZK1) Predicts Poor Clinical Outcome in Patients with clear Cell Renal Cell Carcinoma. EBioMedicine 15, 62–72. doi:10.1016/j.ebiom.2016.12.003
Keywords: KIRC, TLRs, prognosis, diagnosis, biomarkers
Citation: Zou X, Guo B, Ling Q and Mo Z (2022) Toll-Like Receptors Serve as Biomarkers for Early Diagnosis and Prognosis Assessment of Kidney Renal Clear Cell Carcinoma by Influencing the Immune Microenvironment: Comprehensive Bioinformatics Analysis Combined With Experimental Validation. Front. Mol. Biosci. 9:832238. doi: 10.3389/fmolb.2022.832238
Received: 09 December 2021; Accepted: 06 January 2022;
Published: 21 January 2022.
Edited by:
Matteo Becatti, University of Firenze, ItalyReviewed by:
Sriram Chitta, University of Texas MD Anderson Cancer Center, United StatesYusuke Murakami, Musashino University, Japan
Madhu Chhanda Mohanty, National Institute of Virology (ICMR), India
Copyright © 2022 Zou, Guo, Ling and Mo. This is an open-access article distributed under the terms of the Creative Commons Attribution License (CC BY). The use, distribution or reproduction in other forums is permitted, provided the original author(s) and the copyright owner(s) are credited and that the original publication in this journal is cited, in accordance with accepted academic practice. No use, distribution or reproduction is permitted which does not comply with these terms.
*Correspondence: Zengnan Mo, bW96ZW5nbmFuQGd4bXUuZWR1LmNu
†These authors have contributed equally to this work