- 1Department of Cardiology, Guangzhou Eighth People’s Hospital, Guangzhou Medical University, Guangzhou, China
- 2Department of Cardiology, Huanggang Central Hospital, Huanggang, China
- 3Department of Cardiology, The First Affiliated Hospital of Harbin Medical University, Harbin, China
- 4Guangdong Provincial Key Laboratory of South China Structural Heart Disease, Guangdong Cardiovascular Institute, School of Medicine, Guangdong Provincial People’s Hospital and Guangdong Academy of Medical Sciences, South China University of Technology, Guangzhou, China
- 5School of Forensic Medicine, Guizhou Medical University, Guiyang, China
Objective: Ischemic cardiomyopathy (ICM) is a major cardiovascular state associated with prominently increased morbidity and mortality. Our purpose was to detect reliable gene signatures for ICM through integrated feature selection strategies.
Methods: Transcriptome profiles of ICM were curated from the GEO project. Classification models, including least absolute shrinkage and selection operator (LASSO), support vector machine (SVM), and random forest, were adopted for identifying candidate ICM-specific genes for ICM. Immune cell infiltrates were estimated using the CIBERSORT method. Expressions of candidate genes were verified in ICM and healthy myocardial tissues via Western blotting. JC-1 staining, flow cytometry, and TUNEL staining were presented in hypoxia/reoxygenation (H/R)-stimulated H9C2 cells with TRMT5 deficiency.
Results: Following the integration of three feature selection methods, we identified seven candidate ICM-specific genes including ASPN, TRMT5, LUM, FCN3, CNN1, PCNT, and HOPX. ROC curves confirmed the excellent diagnostic efficacy of this combination of previous candidate genes in ICM. Most of them presented prominent interactions with immune cell infiltrates. Their deregulations were confirmed in ICM than healthy myocardial tissues. TRMT5 expressions were remarkedly upregulated in H/R-stimulated H9C2 cells. TRMT5 deficiency enhanced mitochondrial membrane potential and reduced apoptosis in H/R-exposed H9C2 cells.
Conclusion: Collectively, our findings identified reliable gene signatures through combination strategies of diverse feature selection methods, which facilitated the early detection of ICM and revealed the underlying mechanisms.
Introduction
Ischemic cardiomyopathy (ICM) represents the main cause of deaths and acts as an independent factor of mortality among all cardiomyopathy diseases (Luczak et al., 2020). This disease has the features of diverse clinical manifestations and pathophysiological substrates (Gu et al., 2020). It is diagnosed in accordance with left ventricular dysfunctions following epicardial coronary artery diseases (Cabac-Pogorevici et al., 2020). Currently, therapeutic modalities focus on relief of symptoms, prevention of ICM progression, and improvement of survival and quality of life (Razeghian-Jahromi et al., 2021). Heart transplantation and ventricular assist device implantation are applied for treating patients with severe heart failure (Mori et al., 2019). Nevertheless, issues like donor shortage and dismal complications hinder the effects of previous therapeutic modalities. Thus, more effective therapeutic regimens are urgently developed.
Accumulated evidence demonstrates that numerous fetal and immediate-early genes present deregulation in ICM (Dang et al., 2020). However, genetic causes and molecular mechanisms underlying ICM progression remain partially clarified (Suffee et al., 2020). Evidence suggests that gene signatures exert critical roles in diverse diseases that depend on the leapfrog advance of sequencing technologies (Li et al., 2020). For instance, Dang et al. proposed deregulated genes in ICM and their upstream transcription factors (Dang et al., 2020). Wang et al. reported that transcriptome profile analyses uncovered PHLDA1 as a candidate molecular signature for ICM (Wang et al., 2018). Cao et al. identified key hub genes of ICM in accordance with bioinformatics analyses (Cao et al., 2021). Current studies concerning ICM are limited by inadequate biological specimens from ICM patients. Thus, specific gene expression that involves pathological conditions of ICM requires in-depth exploration based on multiple ICM specimens. In the present study, our combination strategies of diverse feature selection methods identified candidate gene signatures, which contributed to an in-depth understanding of the mechanisms and novel diagnostic and therapeutic targets of ICM.
Materials and Methods
Retrieval of Gene Expression Profiling
From the Gene Expression Omnibus (GEO) repository (https://www.ncbi.nlm.nih.gov/gds/), we harvested the transcriptome data relevant to ICM patients. Under screening, two reliable datasets were retrieved, including GSE5406 and GSE46224 datasets. In brief, microarray expression profiling of left ventricular myocardium from 108 advanced ICM patients and 16 non-failing controls was curated from the GSE5406 dataset as the training set (Hannenhalli et al., 2006). This dataset was on the basis of the platform of GPL96 [HG-U133A] Affymetrix Human Genome U133A Array. High-throughput sequencing data of 16 failing and 8 non-failing control hearts were retrieved from the GSE46224 dataset that was based on the GPL11154 Illumina HiSeq 2000 (Homo sapiens) platform (Yang et al., 2014). The GSE46224 dataset acted as the validation set.
Analysis of ICM-Specific Genes
By using linear models for microarray data (limma) package, expression values were compared between ICM and control left ventricular myocardium tissues (Ritchie et al., 2015). The corresponding p-values of the gene symbols following t-tests were determined. Thereafter, adjusted p < 0.05 and |fold change (FC)|>1.5 were utilized as the filtrating criteria. Using the pheatmap package, ICM-specific genes were visualized into the heatmap.
Functional Enrichment Analyses
Using the clusterProfiler package (Yu et al., 2012), Gene Ontology (GO) enrichment analyses were carried out. The biological process, cell component, and molecular function of ICM-specific genes were separately investigated. The same tool was also adopted for the enrichment analyses of Kyoto Encyclopedia of Genes and Genomes (KEGG) pathways.
Analyses of Protein–Protein Interaction
Using the Search Tool for the Retrieval of Interacting Genes/Proteins (STRING; https://string-db.org/) (Szklarczyk et al., 2021), the interactions of proteins encoded by ICM-specific genes were analyzed. Thereafter, a PPI network was conducted using Cytoscape software (Doncheva et al., 2019). Moreover, Molecular Complex Detection (MCODE), a plug-in in Cytoscape (Bader and Hogue, 2003), was used to screen modules of the PPI network in accordance with degree cutoff = 2, node score cutoff = 0.2, k-core = 2, and max. depth = 100. Nodes and edges in the PPI networks separately meant the proteins encoded by ICM-specific genes and their interactions.
Feature Selection of Candidate Genes
Feature selection algorithms including least absolute shrinkage and selection operator (LASSO) analyses, support vector machine (SVM), and random forest were separately conducted using the glmnet package (Engebretsen and Bohlin, 2019), e1071 package, and random forest package. Following the intersection of characteristic genes from three algorithms, candidate ICM-specific genes were identified. The diagnostic efficacy of this combination model was evaluated by receiver operating characteristic (ROC) curves, and the area under the curve (AUC) was measured for investigations of accuracy, sensitivity, and specificity.
Estimation of Immune Cell Subpopulation Fractions
The abundance of 22 immune cell subpopulations in left ventricular myocardium samples from the GSE5406 dataset was quantified using Cell type Identification By Estimating Relative Subsets Of RNA Transcripts (CIBERSORT; http://cibersort.stanford.edu/) (Newman et al., 2015). This analytical tool provides an estimation of the proportions of immune cell subpopulations across mixed cell populations utilizing normalized expression profiles. The infiltrating immune cells were estimated using CIBERSORT included B cells, T cells, natural killer cells, macrophages, dendritic cells, eosinophils, and neutrophils. The “LM22.txt” matrix comprises the “signature matrix” of 547 genes. For running CIBERSORT, the packages of e1071, parallel and preprocessCore were adopted. The p-value was derived from this method for deconvoluting all specimens utilizing Monte Carlo sampling. With p < 0.05, specimens of the estimated abundance of immune cell subpopulations generated with CIBERSORT were of high accuracy. Finally, the proportions of immune cell subpopulations were inferred across diverse tissues.
Cell Culture
The H9C2 cell line was purchased from Chinese Academy of Sciences. Cells were maintained in high-glucose DMEM containing 10% fetal bovine serum (FBS) and 100 U/mL of penicillin/streptomycin. They were grown in an incubator with 5% CO2 at 37°C.
Real-Time Quantitative Polymerase Chain Reaction
Total RNA was extracted from H9C2 cell specimens with TRIzol, which was quantified and reversely transcribed into cDNA. RT-qPCR was carried out utilizing the QuantStudio 5 Real-Time PCR System (Applied Biosystems, United States) with the SuperScript IV One-Step RT-PCR System (Invitrogen, United States). The qPCR conditions were as follows: initial denaturation at 95°C lasting 3 min, 40 cycles of denaturation at 95°C lasting 15 s, annealing at 60°C lasting 30 s, and elongation at 72°C lasting 15 s. The final cycle included extension at 72°C for 3 min. RT-qPCR primers used are listed in Table 1. The 2−ΔΔCt method was applied for quantification.
Western Blotting
Tissues or H9C2 cells were homogenized through precooled RIPA lysis buffer plus 1% protease and phosphatase inhibitors. Thereafter, specimens were centrifuged at 12,000 g lasting 15 min at 4°C. The supernatant was harvested for adding loading buffer, and the extract was boiled at 100°C lasting 5 min. Through sodium dodecyl sulfate polyacrylamide gel electrophoresis (SDS-PAGE), the protein extract was separated. Protein specimens were transferred onto polyvinylidene fluoride (PVDF) membranes. Thereafter, the membranes were under incubation with 5% skim milk lasting 1 h. The primary antibodies targeting ASPN (1/3000; ab154404; Abcam, United States), TRMT5 (1/3000; 18255-1-AP; Proteintech, Wuhan, China), FCN3 (1/1000; 11867-1-AP; Proteintech, Wuhan, China), LUM (1/1000; 10677-1-AP; Proteintech, Wuhan, China), CNN1 (1/3000; 24855-1-AP; Proteintech, Wuhan, China), PCNT (1/2000; ab231958; Abcam, United States), HOPX (1/2000; 11419-1-AP; Proteintech, Wuhan, China), β-actin (1/3000; 20536-1-AP; Proteintech, Wuhan, China), Bcl-2 (1/2000; 12789-1-AP; Proteintech, Wuhan, China), Bax (1/5000; 50599-2-Ig; Proteintech, Wuhan, China), and GAPDH (1/20000; 60004-1-Ig; Proteintech, Wuhan, China) were added for incubation by the membranes at 4°C overnight. Then, the membranes were incubated with the secondary antibody (1/3000; 7074; Cell Signaling Technology, United States) for 1 h at room temperature. The bots were visualized utilizing the enhanced chemiluminescence (ECL) detection system as well as assessed using ImageJ software.
Patients and Specimens
Three patients were diagnosed with ICM, and three healthy controls were recruited from Guangdong Provincial People’s Hospital in our study. Human left ventricular myocardial tissues were collected from the three ICM patients during cardiac transplantation. Meanwhile, three healthy left ventricular myocardial tissues as controls were obtained from unused donor hearts. The study complied with the Declaration of Helsinki. This study gained the approval of the Ethics Committee of Guangdong Provincial People’s Hospital (GDREC2016255H). The signed informed consent of each participant was required.
Establishment of Cell Models
When H9C2 cells grew to 70–80% density, they were digested by trypsin with ethylenediaminetetraacetic acid (EDTA). Thereafter, the cells were seeded onto six-well plates. Hypoxia/reoxygenation (H/R) cellular model was established. In brief, H9C2 cells were maintained in glucose- and serum-free DMEM as well as exposed to hypoxia at 37°C in a hypoxic plastic chamber (5% CO2, 1% O2, and 94% N2) lasting 8 h. Thereafter, H9C2 cells were subjected to reoxygenation at 37°C in a normoxic incubator (95% air and 5% CO2) lasting 24 h.
Transfections
For silencing TRMT5 gene expression, H9C2 cells were transfected by small interfering RNAs (siRNAs; GenePharma, Shanghai, China) in accordance with manufacturer’s instructions. In brief, in a mixture of 100 pmol siRNAs, 5.0 μL Lipofectamine™ 2000 (Thermo Fisher, United States), and 500 μL DMEM, they were incubated lasting 20 min at room temperature. Thereafter, the mixture was transferred onto the plate and incubated lasting 37°C. The culture medium was replaced by a fresh growth medium plus FBS. Cardiomyocytes were collected 24 h following transfections and subjected onto subsequent assays. The specificity or efficiency of TRMT5 deficiency was investigated with RT-qPCR and Western blotting. Scrambled siRNA acted as a negative control (si-NC). Herein, two specific TRMT5 siRNAs (si-TRMT5#1 and si-TRMT5#2) were utilized to silence TRMT5 expression.
JC-1 Staining
JC-1 staining (Thermo Fisher, United States) was presented for investigation of mitochondrial membrane potential. H9C2 cells were incubated by a mixture of working solution of JC-1 dye and cell culture medium. The excess dye was removed by washing with JC-1staining buffer and rinsed with PBS three times. Thereafter, cardiomyocytes were investigated and acquired using a confocal laser scanning microscope. OD values of green fluorescence (490 nm/530 nm) and red fluorescence (525 nm/590 nm) were separately examined. Eventually, data were displayed as a red/green fluorescence ratio.
Flow Cytometric Analyses
Flow cytometric analyses were carried out utilizing FITC-labeled recombinant Annexin V apoptosis detection kits (Beyotime, China). H9C2 cells were washed with PBS and reconstituted with coupling buffer. Thereafter, cardiomyocytes were incubated by 250 ng/ml Annexin V-FITC protecting from light at 4°C lasting 15 min. Cardiomyocytes were washed with PBS and resuspended with 190 µL coupling buffer. Then, cardiomyocytes were exposed to 10 µL propidium iodide (PI) lasting 5 min. Staining cardiomyocytes were assessed using an FACStar plus flow cytometer. Data analyses were carried out utilizing a BD BioSciences FACSCalibur flow cytometer.
Terminal Deoxynucleotidyl Transferase dUTP Nick-End Labeling Assay
H9C2 cells were maintained onto the chamber sections (1 × 104 cells/chamber). Apoptotic cardiomyocytes were examined with TUNEL staining utilizing In Situ Cell Death Detection Kits in accordance with the supplier’s specification. Images were captured using an Olympus BX41 fluorescence microscope. Image quantification was carried out as percentages of TUNEL-positive cardiomyocytes within the entire number of cardiomyocytes.
Statistical Analyses
p-values under 0.05 indicated significant differences. Data were expressed as mean ± SD. R software (version 3.4.0; https://www.r-project.org/) and Bioconductor packages (http://www.bioconductor.org/) and GraphPad Prism software (version 8.0.1) were adopted for statistical analyses. Student’s t-test or Wilcoxon test was utilized for comparing the significant differences between two subgroups. One-way analyses of variance (ANOVA) and Tukey’s post hoc test were presented for comparing multiple subgroups. Pearson or Spearman correlation test was conducted for evaluation of the interactions between variables.
Results
Detection of ICM-specific Genes as well as Their Biological Significance
Byemploying the transcriptome profiling of the left ventricular myocardium from 108 ICM patients and 16 non-failing controls in the GSE5406 dataset, we screened ICM-specific genes with the criteria of an FDR <0.05 and |fold change| >1.5. Consequently, 153 genes were specifically expressed in ICM (Figures 1A,B). Among them, 62 presented upregulation, while 91 presented downregulation in ICM left ventricular myocardium tissues (Tables 2, 3). Functional enrichment analyses conducted using the clusterProfiler package uncovered the ICM-specific genes-relevant biological significance and pathways. GO analyses demonstrated that the biological functions of ICM-specific genes were primarily associated with cellular response to external stimulus, extracellular matrix organization, extracellular structure organization, response to mechanical stimulus, and response to transforming growth factor beta (Figure 1C). In Figure 1D, cellular components of collagen-containing extracellular matrix, contractile fiber, I band, myofibril, and sarcomere were primarily enriched by ICM-specific genes. Analysis of molecular functions revealed the extracellular matrix structural constituent, glycosaminoglycan binding, heparin binding, integrin binding, and proteoglycan binding remarkedly related to ICM-specific genes (Figure 1E). KEGG analyses displayed that ICM-specific genes primarily participated in bladder cancer, cGMP-PKG signaling pathway, focal adhesion, HIF-1 signaling pathway, malaria, protein digestion and absorption, and proteoglycans in cancer (Figure 1F). Table 4 shows detailed information of the previous function enrichment results of ICM-specific genes.
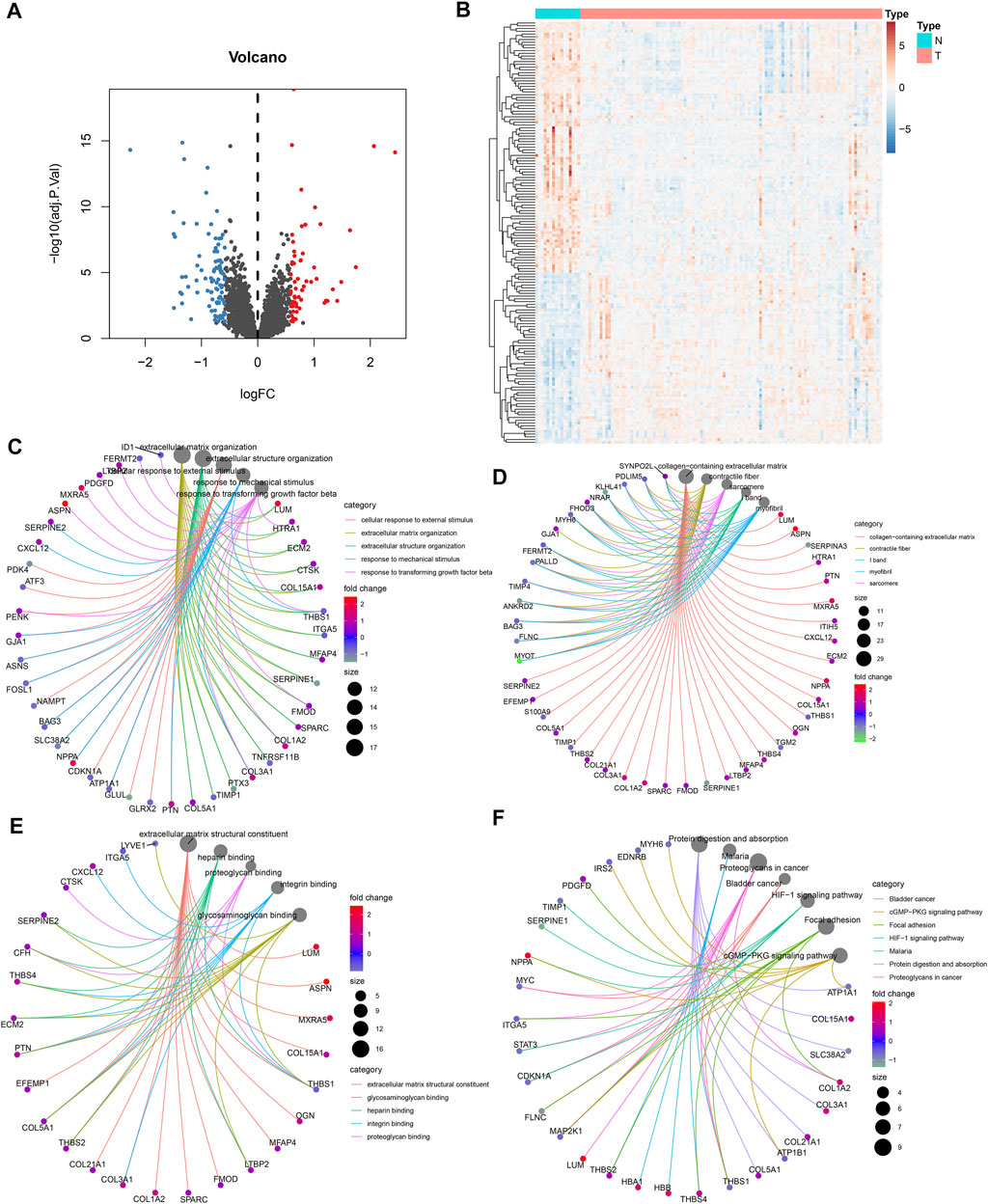
FIGURE 1. Visualization of ICM-specific genes and exploration of functional enrichment analyses. (A) ICM-specific genes screened through thresholds of FDR <0.05 and |fold change| >1.5 were visualized via volcano plots. Blue bots represent downregulated genes, while red bots represent upregulated genes in ICM. (B) Heatmap presents the expression patterns of ICM-specific genes in ICM and control left ventricular myocardium tissues. (C–F) Interaction networks show the most prominent (C) biological processes, (D) cellular components, (E) molecular functions, and (F) KEGG pathways enriched by ICM-specific genes.
Analysis of Interactions Between ICM-Specific Genes
Through the STRING project, we conducted the PPI network of ICM-specific genes. ICM-specific genes with high expression were marked with purple color, while ICM-specific genes with low expression were marked with blue color. There were 322 interaction pairs in the PPI network, uncovering the close interactions between ICM-specific genes (Figure 2A). For figuring out the most prominent module of this complex network, this study presented the MCODE plug-in analyses. This module contained seven upregulated ICM-specific genes (COL3A1, COL1A2, COL5A1, SPARC, FMOD, LUM, and THBS2) and four downregulated genes (ITGA5, THBS1, SERPINE1, and TIMP1), as depicted in Figure 2B.
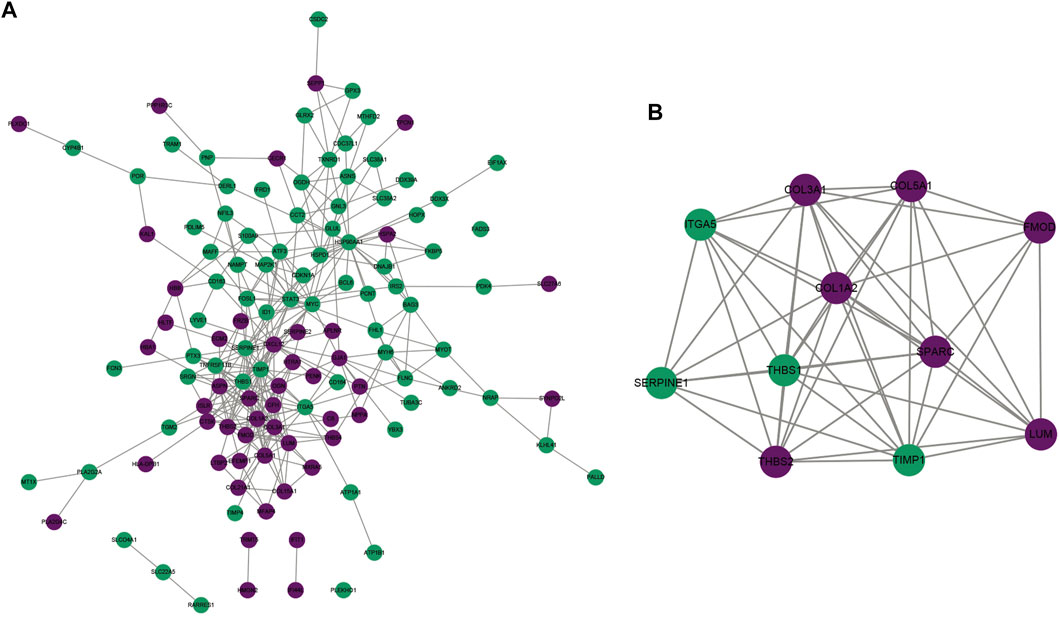
FIGURE 2. Analysis of interactions between ICM-specific genes. (A) The PPI network was conducted for uncovering the interactions between ICM-specific genes. (B) The most prominent module was determined via MCODE plug-in. Upregulated ICM-specific genes are marked purple, whereas downregulated ICM-specific genes are marked green.
Selection of Candidate ICM-Specific Genes Through Integration of Three Algorithms: LASSO, SVM, and Random Forest
This study adopted three feature selection algorithms including LASSO, SVM, and random forest for identifying candidate ICM-specific genes. By using the LASSO method, we identified 12 candidate variables containing HMGN2, FCN3, TRMT5, LUM, ASPN, HOPX, CNN1, GLUL, PCNT, NPPA, THBS1, and GPX3 (Figures 3A,B). The SVM method uncovered 53 feature genes (Figure 3C). Meanwhile, 23 important variables were selected using the random forest method (Figure 3D). Following the integration of results derived from three algorithms, seven candidate ICM-specific genes were finally identified, including ASPN, TRMT5, LUM, FCN3, CNN1, PCNT, and HOPX (Figure 3E).
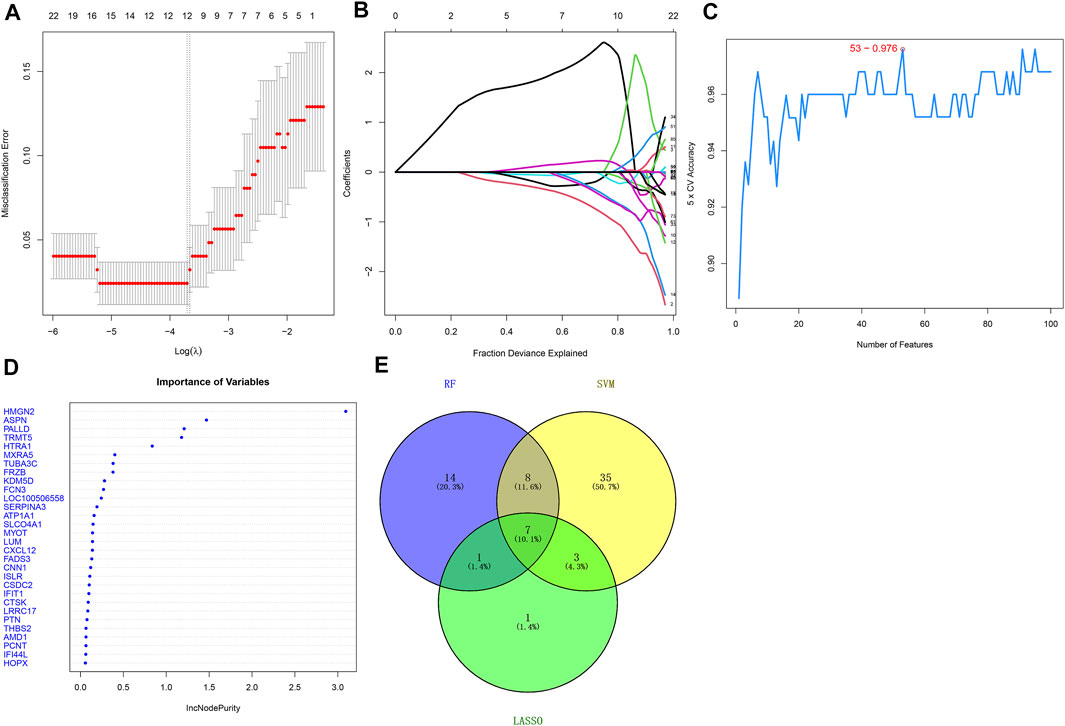
FIGURE 3. Selection of candidate ICM-specific genes through integration of three algorithms: LASSO, SVM, and random forest. (A) LASSO coefficient spectrum of ICM-specific genes across ICM samples. Coefficient distribution diagram was generated regarding logarithmic (λ) sequences. (B) Selection of the optimal variables for ICM within the LASSO model (λ). (C) Screening characteristic genes using the SVM method. (D) Random forest was adopted for selecting important variables. (E) Venn diagram shows candidate ICM-specific genes through integration of results derived from three algorithms.
Combination of Candidate ICM-Specific Genes as a Reliable Diagnostic Model of ICM
ROC curves were conducted for investigations of the diagnostic efficacy of this combination of candidate ICM-specific genes in ICM. As depicted in Figure 4A, the AUC of this combined gene model was 0.995 in the GSE5406 dataset, confirming the excellent diagnostic efficacy in ICM. Additionally, the GSE46224 dataset was adopted for verification of this model. As a result, AUC was 0.922, indicative of its favorable predictive potential (Figure 4B). The expressions of candidate ICM-specific genes were investigated in ICM and control myocardial tissues in the GSE5406 dataset. As a result, ASPN, TRMT5, and LUM expressions were upregulated, while FCN3, CNN1, PCNT, and HOPX expressions were declined in ICM compared with controls (Figure 4C).
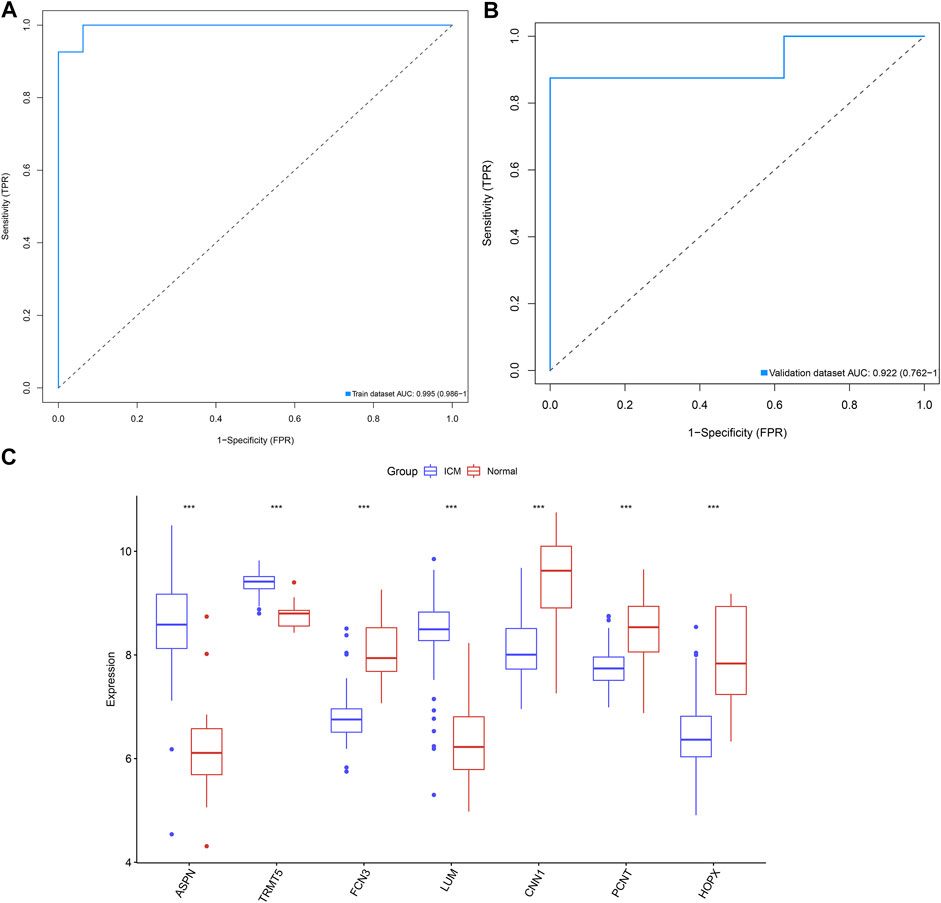
FIGURE 4. Combination of candidate ICM-specific genes as a reliable diagnostic model of ICM. (A) ROC curves were conducted for evaluations of the diagnostic potential of this combination of candidate ICM-specific genes in the GSE5406 dataset. (B) Verification of the diagnostic value of this combination of candidate ICM-specific genes in the GSE46224 dataset. (C) Box plots shows the deregulated expressions of these candidate ICM-specific genes in ICM and controls. ***p < 0.001.
Characterization of Immune Cell Infiltrates in ICM and Control Myocardial Tissues
Using the CIBERSORT algorithm, we estimated the infiltrations of immune cell subpopulations in ICM myocardial tissues. Figure 5A depicts the landscape of 22 immune cell subpopulations across ICM. We also presented investigations about the interactions between immune cell subpopulations. In Figure 5B, there were close interplays between diverse immune cell subpopulations across ICM. In particular, compared with controls, neutrophil presented declined infiltrations in ICM (Figure 5C).
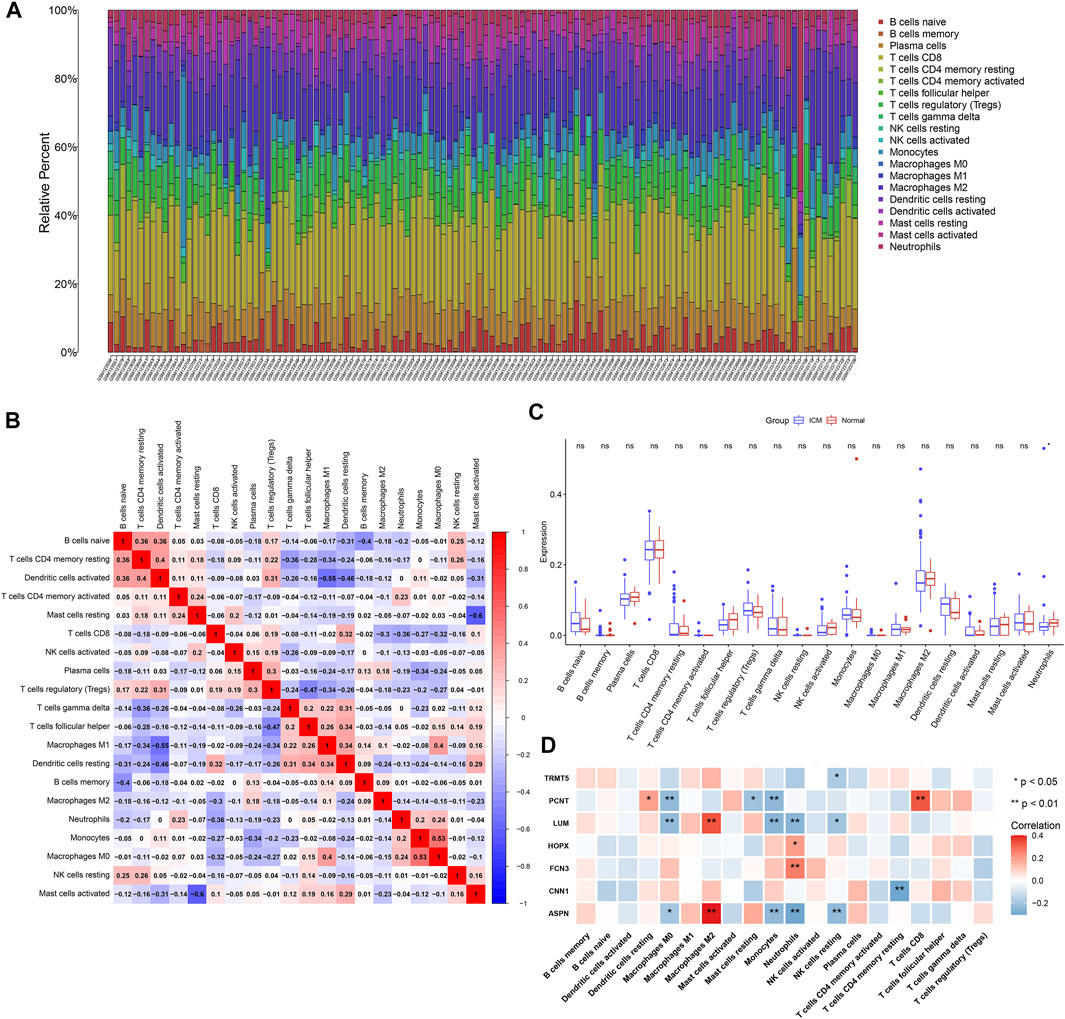
FIGURE 5. Landscape of immune cell infiltrates in ICM and interactions of candidate ICM-specific genes with immune cell infiltrates. (A) Stacked graphs depict the percentages of 22 immune cell subpopulations in ICM myocardial tissues. (B) Heatmap shows the interactions of diverse immune cell subpopulations in ICM myocardial tissues. The deeper the red, the stronger the positive correlation. The darker the blue, the stronger the negative correlation. (C) Box plots depict the differences in immune cell infiltrates in ICM and normal myocardial tissues. (D) Heatmap visualizes the interactions of candidate ICM-specific genes with immune cell infiltrates in ICM. *p < 0.05; **p < 0.01; ns: not significant.
Interactions of Candidate ICM-Specific Genes With Immune Cell Infiltrates
This study analyzed the interactions of candidate ICM-specific genes with immune cell infiltrates across ICM myocardial tissues. Our data suggested that ASPN and LUM expressions were positively associated with M2 macrophage while negatively correlated to M0 macrophages, monocytes, neutrophils, and resting NK cells (Figure 5D). CNN1 expressions displayed negative correlations to CD4 memory resting T cells as well as FCN3 and HOPX3 expressions exhibited positive associations with neutrophils. PCNT expressions were positively associated with the resting dendritic cells and CD8 T cells while negatively correlated to M0 macrophages, resting mast cells, and monocytes. Additionally, TRMT5 expressions presented negative interactions with resting NK cells.
Verification of Expressions of Candidate ICM-Specific Genes
Herein, we established H/R-stimulated ICM cellular models. By RT-qPCR, we conducted investigations of the mRNA expressions of candidate ICM-specific genes including ASPN, TRMT5, LUM, FCN3, CNN1, PCNT, and HOPX. Figure 6A demonstrates that ASPN, TRMT5, and LUM mRNAs presented the upregulations in H/R-stimulated H9C2 cells compared to controls. In contrast, FCN3, CNN1, PCNT, and HOPX expressions were declined in H/R-stimulated H9C2 cells than controls. Additionally, Western blotting uncovered the elevated expressions of ASPN, TRMT5, and LUM in H/R-stimulated H9C2 cells compared with control cells (Figures 6B–E). Meanwhile, lower FCN3, CNN1, PCNT, and HOPX expressions were observed in H/R-stimulated H9C2 cells compared with controls (Figures 6F–I). We collected three ICM and three healthy left ventricular myocardial tissues. Western blotting confirmed the upregulations of ASPN, TRMT5, and LUM as well as the downregulations of FCN3, CNN1, PCNT, and HOPX in ICM compared with healthy left ventricular myocardial tissues (Figures 6J–Q). These data confirmed the deregulated expressions of candidate ICM-specific genes in ICM.
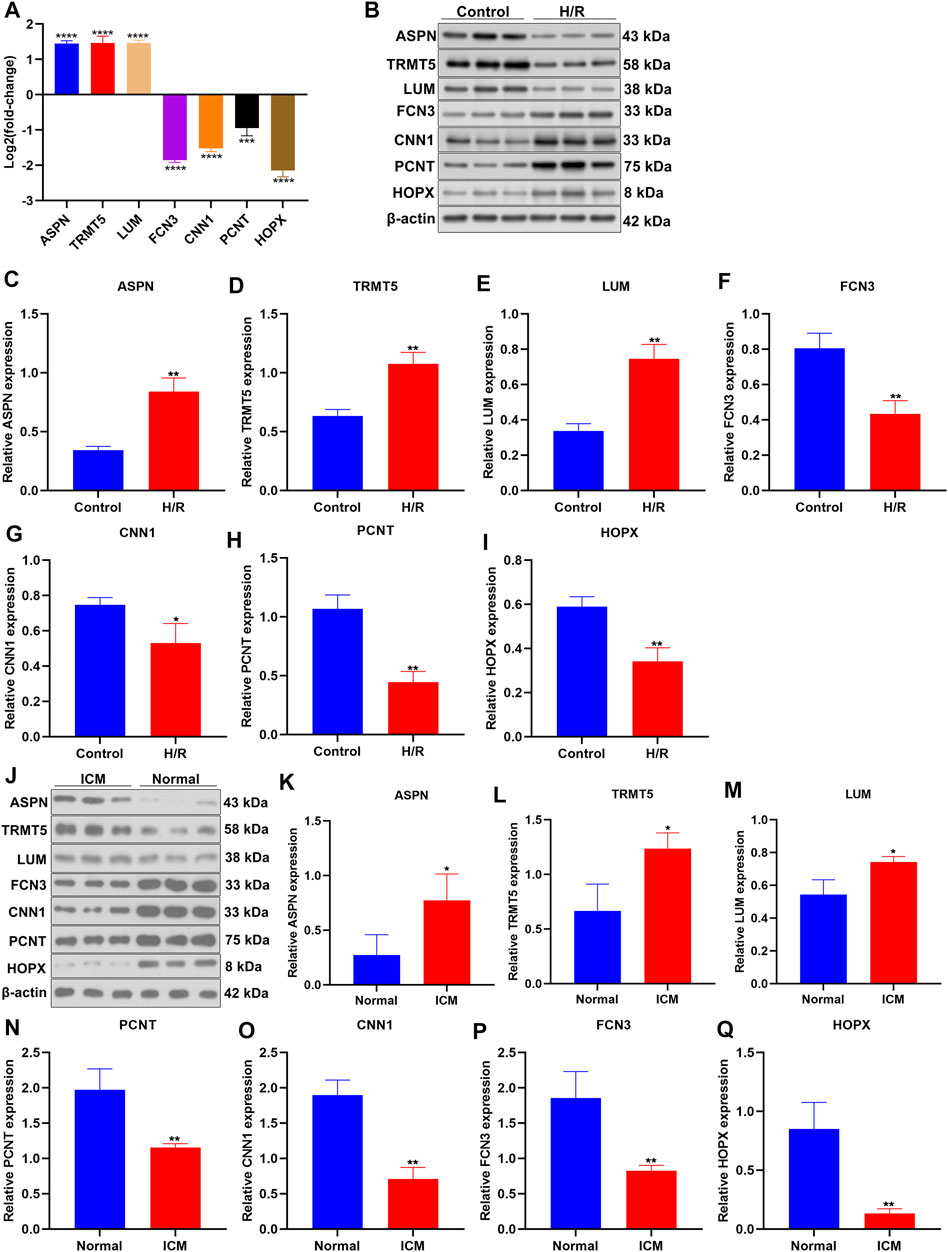
FIGURE 6. Verification of expressions of candidate ICM-specific genes. (A) The mRNA expressions of candidate ICM-specific genes including ASPN, TRMT5, LUM, FCN3, CNN1, PCNT, and HOPX were investigated in H/R-stimulated H9C2 cells and control cells with RT-qPCR. (B–I) Deregulated expressions of ASPN, TRMT5, LUM, FCN3, CNN1, PCNT, and HOPX were examined in H/R-stimulated H9C2 cells and control cells by Western blotting. (J–Q) Abnormal expressions of ASPN, TRMT5, LUM, FCN3, CNN1, PCNT, and HOPX were examined in three human ICM and control left ventricular myocardial tissues by Western blotting. n = 3. p-values were estimated using Student’s test. *p < 0.05; **p < 0.01; ***p < 0.001; ****p < 0.0001.
TRMT5 Deficiency Enhances Mitochondrial Membrane Potential of H/R-Stimulated H9C2 Cells
Among candidate ICM-specific genes, the role of TRMT5 in ICM has not yet been reported. Therefore, we presented an in-depth investigation about the implications of TRMT5 in ICM progression. TRMT5 expressions were first deficient in H9C2 cells through transfections of si-TRMT5. In Figure 7A, TRMT5 deficiency triggered by si-TRMT5 was confirmed in H9C2 cells. Intriguingly, TRMT5 expressions presented upregulation in H/R-stimulated H9C2 cells compared to controls (Figures 7B–D). Nevertheless, si-TRMT5 remarkedly reduced the expressions of TRMT5 stimulated by H/R. JC-1 staining and was employed for the evaluation of mitochondrial membrane potential. In Figures 7E,F, the reduced ratios of red/green fluorescence were noticed in H/R-exposed H9C2 cells than controls, which indicated that the mitochondrial membrane potential of H9C2 cells was decreased following H/R stimulation. Additionally, the ratios of red/green fluorescence were elevated in H/R-exposed H9C2 cells with si-TRMT5 transfections in comparison to H/R-exposed H9C2 cells. This indicated that TRMT5 deficiency enhanced the mitochondrial membrane potential of H/R-stimulated H9C2 cells.
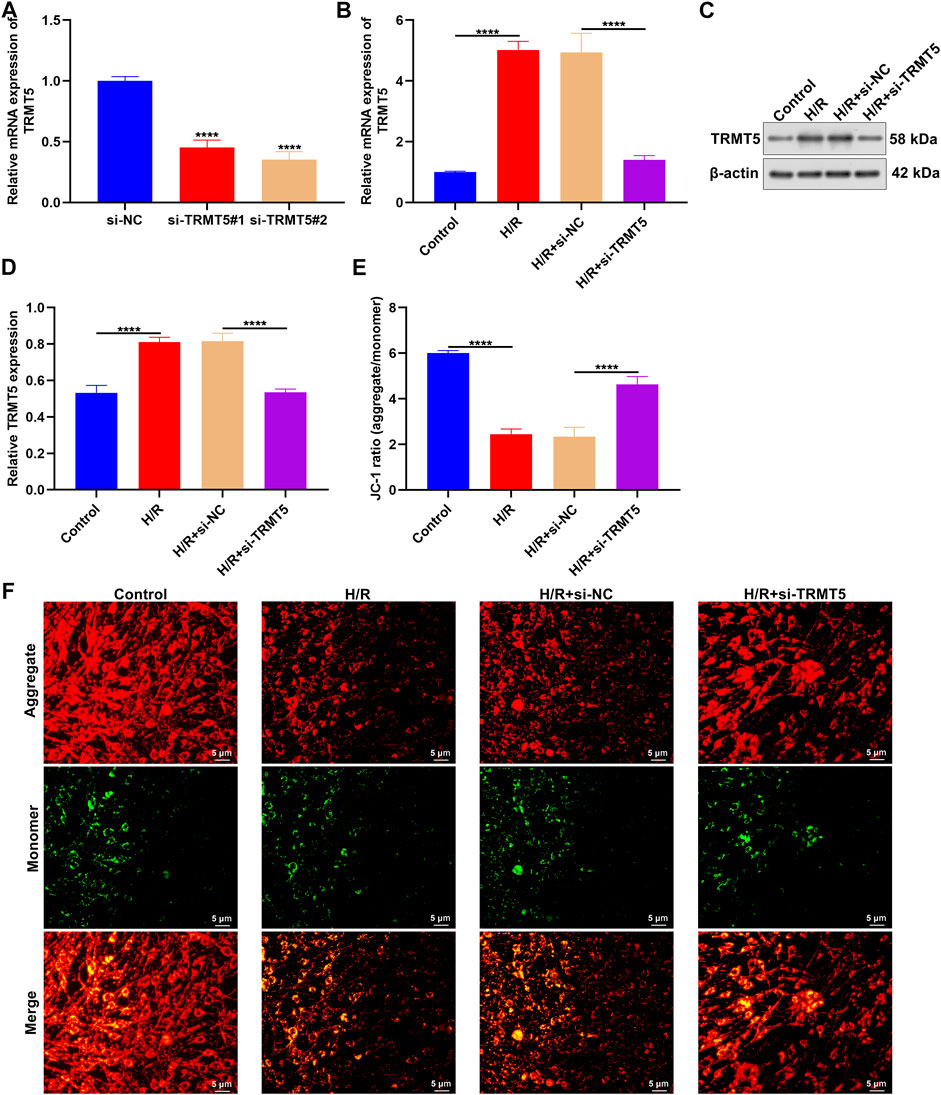
FIGURE 7. TRMT5 deficiency enhances mitochondrial membrane potential of H/R-stimulated H9C2 cells. (A) The mRNA expressions of TRMT5 were examined in H9C2 cells with specific siRNAs against TRMT5 transfections. (B–D) RT-qPCR and Western blotting was presented for quantification of TRMT5 expressions in H9C2 cells with H/R stimulation and si-TRMT5 transfections. (E,F) JC-1 staining was adopted for the determination of mitochondrial membrane potential of H9C2 cells with H/R stimulation and si-TRMT5 transfections. The ratio of red (aggregate) to green (monomer) fluorescence represented mitochondrial membrane potential. Magnification, ×200; scale bar, 5 μm. n = 3. p-values were estimated using ANOVA with Tukey’s post hoc test. ****p < 0.0001.
TRMT5 Deficiency Alleviates H/R-Triggered H9C2 Cell Apoptosis
Flow cytometric analyses showed the increased apoptotic levels of H/R-stimulated H9C2 cells than controls (Figures 8A,B). Nevertheless, the apoptosis of H9C2 cells was remarkedly declined following TRMT5 deficiency. Additionally, we presented TUNEL assay for investigations of cardiomyocyte apoptosis. More TUNEL-positive cells were detected in H/R-exposed H9C2 cells (Figures 8C,D). But TRMT5 deficiency reduced the ratios of TUNEL-positive H/R-exposed H9C2 cells. We also noted that the Bcl-2 expression was downregulated, while Bax expression was upregulated in H/R-exposed H9C2 cells (Figures 8E–G). However, TRMT5 blockage significantly reversed the expression of Bcl-2 and Bax in H/R-exposed H9C2 cells. Hence, TRMT5 deficiency alleviated H/R-triggered H9C2 cell apoptosis.
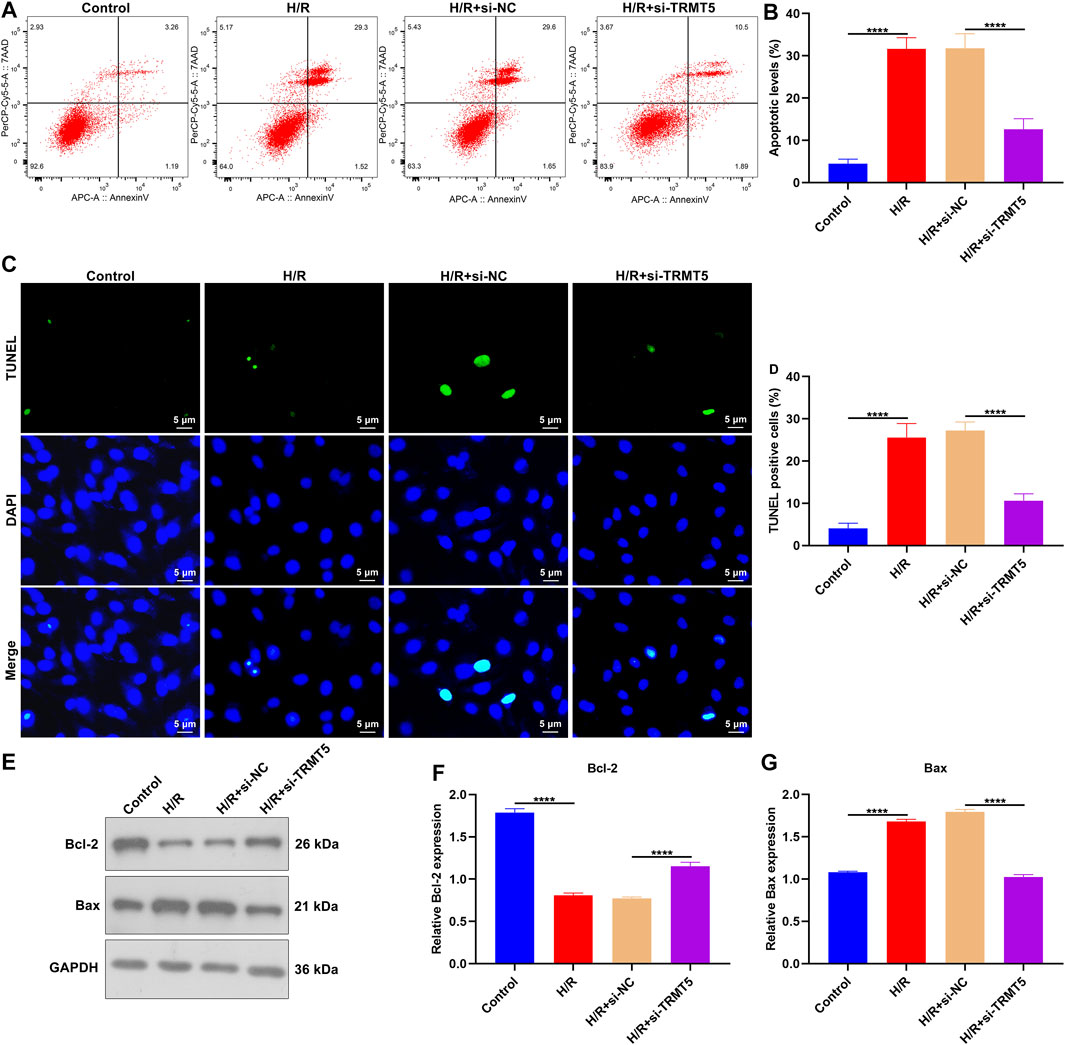
FIGURE 8. TRMT5 deficiency alleviates H/R-triggered H9C2 cell apoptosis. (A,B) Apoptotic H9C2 cells were estimated by flow cytometric analyses following H/R exposure and TRMT5 deficiency. (C,D) Apoptosis was tested utilizing TUNEL staining in H9C2 cells with H/R exposure and TRMT5 deficiency. Magnification, ×200; scale bar, 5 μm. (E–G) Western blotting was used for detecting the expression of Bcl-2 and Bax in H9C2 cells with H/R exposure as well as TRMT5 deficiency. N = 3. p-values were estimated using ANOVA with Tukey’s post hoc test. ****p < 0.0001.
Discussion
Deregulated expression of genes may trigger ICM progression and dismal clinical prognosis. This study detected 153 ICM-specific genes; among them, 62 presented upregulation, while 91 presented downregulation in ICM myocardium tissues. In particular, these genes participated in ICM-relevant processes and signals like extracellular matrix and structure organization and HIF-1 signaling pathway. The data highlighted the underlying implications of ICM-specific genes during ICM progression.
Through combination strategies of LASSO, SVM, and random forest algorithms, we eventually identified seven reliable candidate ICM-specific genes (ASPN, TRMT5, LUM, FCN3, CNN1, PCNT, and HOPX). ROC curves confirmed that the combination of previous seven candidate ICM-specific genes acted as an accurate diagnostic tool for ICM patients. ASPN, TRMT5, and LUM expressions presented the upregulations in ICM than controls. In contrast, FCN3, CNN1, PCNT, and HOPX expressions were declined in ICM than controls. Previously, ASPN protein displayed increased expressions in ICM left ventricular tissues that are highly correlated to left ventricular ejection fraction (Zhang et al., 2021). Moreover, ASPN expressions are upregulated in cardiac remodeling mouse models (Wang et al., 2019). The upregulations are proposed in the myocardium of diabetic mice and in glycated low-density lipoprotein-triggered cardiomyocytes (Li et al., 2019). Moreover, ASPN triggers the apoptosis of H9C2 cardiomyocytes. The downregulation of circulating FCN3 levels is correlated to advanced heart failure and outcomes (Prohászka et al., 2013). CNN1 suppresses dilated cardiomyopathy progression in mice via εPKC signaling (Lu et al., 2014). Moreover, it modulates TNF-α-triggered apoptosis of vascular endothelial cells (Zhang et al., 2016). Single-cell sequence analyses of cardiac differentiation from human pluripotent stem cells uncover HOPX-mediated maturation of cardiomyocytes (Friedman et al., 2018). The previous evidence suggested the functions of these candidate ICM-specific genes in ICM progression.
ICM acts as a state of chronic immune system activation (Sweet et al., 2018; Bansal et al., 2019; Chelko et al., 2019). Here, we quantified immune cell infiltrates across ICM myocardial tissues using the CIBERSORT method. ICM myocardial tissues had declined infiltrations of neutrophils than controls, indicating that deregulated neutrophils might participate in ICM pathogenesis. Innate immune response and leukocyte recruitment modulate inflammation and healing, playing a critical function in acute myocardial infarction (Eghbalzadeh et al., 2019). Neutrophils participate in the healing following myocardial infarction by polarizing macrophages into a repair phenotype (Horckmans et al., 2017). Deregulated expressions of ASPN, TRMT5, LUM, FCN3, CNN1, PCNT, and HOPX were in relation to diverse immune cell subpopulations across ICM myocardial tissues. This indicated that the previous candidate ICM-specific genes might modulate inflammatory response during ICM progression. In particular, we paid close attention to the implications of TRMT5 in ICM. Experimental evidence showed that H/R stimulation triggered the upregulation of TRMT5 in H9C2 cells (Powell et al., 2015). TRMT5 deficiency enhanced mitochondrial membrane potential and reduced apoptosis in H/R-exposed H9C2 cells. Thus, TRMT5 possessed the potential as a therapeutic target against ICM. Nevertheless, this study presented several disadvantages. The diagnostic potential of this combination of candidate ICM-specific genes will be verified in larger ICM cohorts. Additionally, biological functions and mechanisms involving these ICM-specific genes will be investigated through experiments. Conclusion
This study proposed candidate ICM-specific genes through combination strategies of LASSO, SVM, and random forest algorithms. Our in-depth analyses uncovered that ASPN, TRMT5, LUM, FCN3, CNN1, PCNT, and HOPX might be exploited as reliable diagnostic signatures of ICM. Hence, our findings offered an underlying basis for understanding the etiology and mechanisms of ICM as well as therapeutic targets for clinic therapy.
Data Availability Statement
The original contributions presented in the study are included in the article/supplementary material; further inquiries can be directed to the corresponding authors.
Ethics Statement
The studies involving human participants were reviewed and approved by Guangdong Provincial People’s Hospital (GDREC2016255H). The patients/participants provided their written informed consent to participate in this study.
Author Contributions
YL, YW, and JW conceived and designed the study. HS, SZC, XH, and QZ conducted most of the experiments and data analysis, and wrote the manuscript. CL, CC, SXC, and DL participated in collecting data and helped draft the manuscript. All authors reviewed and approved the manuscript.
Funding
This work was funded by the National Natural Science Foundation of China (81671746 and 81871402); Postdoctoral Science Foundation of Heilongjiang Province (LBH-Q19037).
Conflict of Interest
The authors declare that the research was conducted in the absence of any commercial or financial relationships that could be construed as a potential conflict of interest.
Publisher’s Note
All claims expressed in this article are solely those of the authors and do not necessarily represent those of their affiliated organizations, or those of the publisher, the editors, and the reviewers. Any product that may be evaluated in this article, or claim that may be made by its manufacturer, is not guaranteed or endorsed by the publisher.
Abbreviations
ICM, ischemic cardiomyopathy; GEO, gene expression omnibus; limma, linear models for microarray data; FC, fold change; GO, gene ontology; KEGG, kyoto encyclopedia of genes and genomes; PPI, protein–protein interaction; STRING, search tool for the retrieval of interacting genes/proteins; MCODE, molecular complex detection; LASSO, least absolute shrinkage and selection operator; SVM, support vector machine; ROC, receiver operating characteristic; AUC, area under the curve; CIBERSORT, cell type identification by estimating relative subsets of rna transcripts; RT-qPCR, real-time quantitative polymerase chain reaction; h/r, hypoxia/reoxygenation; TUNEL, terminal deoxynucleotidyl transferase dUTP nick-end labeling.
Reference
Bader, G. D., and Hogue, C. W. (2003). An Automated Method for Finding Molecular Complexes in Large Protein Interaction Networks. BMC Bioinformatics 4, 2. doi:10.1186/1471-2105-4-2
Bansal, S. S., Ismahil, M. A., Goel, M., Zhou, G., Rokosh, G., Hamid, T., et al. (2019). Dysfunctional and Proinflammatory Regulatory T-Lymphocytes Are Essential for Adverse Cardiac Remodeling in Ischemic Cardiomyopathy. Circulation 139 (2), 206–221. doi:10.1161/circulationaha.118.036065
Cabac‐Pogorevici, I., Muk, B., Rustamova, Y., Kalogeropoulos, A., Tzeis, S., and Vardas, P. (2020). Ischaemic Cardiomyopathy. Pathophysiological Insights, Diagnostic Management and the Roles of Revascularisation and Device Treatment. Gaps and Dilemmas in the Era of Advanced Technology. Eur. J. Heart Fail. 22 (5), 789–799. doi:10.1002/ejhf.1747
Cao, J., Liu, Z., Liu, J., Li, C., Zhang, G., and Shi, R. (2021). Bioinformatics Analysis and Identification of Genes and Pathways in Ischemic Cardiomyopathy. Ijgm 14, 5927–5937. doi:10.2147/ijgm.S329980
Chelko, S. P., Asimaki, A., Lowenthal, J., Bueno-Beti, C., Bedja, D., Scalco, A., et al. (2019). Therapeutic Modulation of the Immune Response in Arrhythmogenic Cardiomyopathy. Circulation 140 (18), 1491–1505. doi:10.1161/circulationaha.119.040676
Dang, H., Ye, Y., Zhao, X., and Zeng, Y. (2020). Identification of Candidate Genes in Ischemic Cardiomyopathy by Gene Expression Omnibus Database. BMC Cardiovasc. Disord. 20 (1), 320. doi:10.1186/s12872-020-01596-w
Doncheva, N. T., Morris, J. H., Gorodkin, J., and Jensen, L. J. (2019). Cytoscape StringApp: Network Analysis and Visualization of Proteomics Data. J. Proteome Res. 18 (2), 623–632. doi:10.1021/acs.jproteome.8b00702
Eghbalzadeh, K., Georgi, L., Louis, T., Zhao, H., Keser, U., Weber, C., et al. (2019). Compromised Anti-inflammatory Action of Neutrophil Extracellular Traps in PAD4-Deficient Mice Contributes to Aggravated Acute Inflammation after Myocardial Infarction. Front. Immunol. 10, 2313. doi:10.3389/fimmu.2019.02313
Engebretsen, S., and Bohlin, J. (2019). Statistical Predictions with Glmnet. Clin. Epigenet 11 (1), 123. doi:10.1186/s13148-019-0730-1
Friedman, C. E., Nguyen, Q., Lukowski, S. W., Helfer, A., Chiu, H. S., Miklas, J., et al. (2018). Single-Cell Transcriptomic Analysis of Cardiac Differentiation from Human PSCs Reveals HOPX-Dependent Cardiomyocyte Maturation. Cell Stem Cell 23 (4), 586–598.e8. doi:10.1016/j.stem.2018.09.009
Gu, S., Tan, J., Li, Q., Liu, S., Ma, J., Zheng, Y., et al. (2020). Downregulation of LAPTM4B Contributes to the Impairment of the Autophagic Flux via Unopposed Activation of mTORC1 Signaling During Myocardial Ischemia/Reperfusion Injury. Circ. Res. 127 (7), e148–e165. doi:10.1161/circresaha.119.316388
Hannenhalli, S., Putt, M. E., Gilmore, J. M., Wang, J., Parmacek, M. S., Epstein, J. A., et al. (2006). Transcriptional Genomics Associates FOX Transcription Factors with Human Heart Failure. Circulation 114 (12), 1269–1276. doi:10.1161/circulationaha.106.632430
Horckmans, M., Ring, L., Duchene, J., Santovito, D., Schloss, M. J., Drechsler, M., et al. (2017). Neutrophils Orchestrate post-myocardial Infarction Healing by Polarizing Macrophages towards a Reparative Phenotype. Eur. Heart J. 38 (3), ehw002–197. doi:10.1093/eurheartj/ehw002
Li, X. L., Yu, F., Li, B. Y., Fu, C. L., Yu, X., Xu, M., et al. (2019). The Protective Effects of Grape Seed Procyanidin B2 against Asporin Mediates Glycated Low‐density Lipoprotein Induced‐cardiomyocyte Apoptosis and Fibrosis. Cell Biol Int. 44, 268–277. doi:10.1002/cbin.11229
Li, D., Lin, H., and Li, L. (2020). Multiple Feature Selection Strategies Identified Novel Cardiac Gene Expression Signature for Heart Failure. Front. Physiol. 11, 604241. doi:10.3389/fphys.2020.604241
Lu, D., Zhang, L., Bao, D., Lu, Y., Zhang, X., Liu, N., et al. (2014). Calponin1 Inhibits Dilated Cardiomyopathy Development in Mice through the εPKC Pathway. Int. J. Cardiol. 173 (2), 146–153. doi:10.1016/j.ijcard.2014.02.032
Luczak, E. D., Wu, Y., Granger, J. M., Joiner, M.-l. A., Wilson, N. R., Gupta, A., et al. (2020). Mitochondrial CaMKII Causes Adverse Metabolic Reprogramming and Dilated Cardiomyopathy. Nat. Commun. 11 (1), 4416. doi:10.1038/s41467-020-18165-6
Mori, D., Miyagawa, S., Matsuura, R., Sougawa, N., Fukushima, S., Ueno, T., et al. (2019). Pioglitazone Strengthen Therapeutic Effect of Adipose-Derived Regenerative Cells against Ischemic Cardiomyopathy through Enhanced Expression of Adiponectin and Modulation of Macrophage Phenotype. Cardiovasc. Diabetol. 18 (1), 39. doi:10.1186/s12933-019-0829-x
Newman, A. M., Liu, C. L., Green, M. R., Gentles, A. J., Feng, W., Xu, Y., et al. (2015). Robust Enumeration of Cell Subsets from Tissue Expression Profiles. Nat. Methods 12 (5), 453–457. doi:10.1038/nmeth.3337
Powell, C. A., Kopajtich, R., D’Souza, A. R., Rorbach, J., Kremer, L. S., Husain, R. A., et al. (2015). TRMT5 Mutations Cause a Defect in Post-transcriptional Modification of Mitochondrial tRNA Associated with Multiple Respiratory-Chain Deficiencies. Am. J. Hum. Genet. 97 (2), 319–328. doi:10.1016/j.ajhg.2015.06.011
Prohászka, Z., Munthe-Fog, L., Ueland, T., Gombos, T., Yndestad, A., Förhécz, Z., et al. (2013). Association of Ficolin-3 with Severity and Outcome of Chronic Heart Failure. PLoS One 8 (4), e60976. doi:10.1371/journal.pone.0060976
Razeghian-Jahromi, I., Matta, A. G., Canitrot, R., Zibaeenezhad, M. J., Razmkhah, M., Safari, A., et al. (2021). Surfing the Clinical Trials of Mesenchymal Stem Cell Therapy in Ischemic Cardiomyopathy. Stem Cel Res Ther 12 (1), 361. doi:10.1186/s13287-021-02443-1
Ritchie, M. E., Phipson, B., Wu, D., Hu, Y., Law, C. W., Shi, W., et al. (2015). Limma powers Differential Expression Analyses for RNA-Sequencing and Microarray Studies. Nucleic Acids Res. 43 (7), e47. doi:10.1093/nar/gkv007
Suffee, N., Moore-Morris, T., Jagla, B., Mougenot, N., Dilanian, G., Berthet, M., et al. (2020). Reactivation of the Epicardium at the Origin of Myocardial Fibro-Fatty Infiltration During the Atrial Cardiomyopathy. Circ. Res. 126 (10), 1330–1342. doi:10.1161/circresaha.119.316251
Sweet, M. E., Cocciolo, A., Slavov, D., Jones, K. L., Sweet, J. R., Graw, S. L., et al. (2018). Transcriptome Analysis of Human Heart Failure Reveals Dysregulated Cell Adhesion in Dilated Cardiomyopathy and Activated Immune Pathways in Ischemic Heart Failure. BMC Genomics 19 (1), 812. doi:10.1186/s12864-018-5213-9
Szklarczyk, D., Gable, A. L., Nastou, K. C., Lyon, D., Kirsch, R., Pyysalo, S., et al. (2021). The STRING Database in 2021: Customizable Protein-Protein Networks, and Functional Characterization of User-Uploaded Gene/measurement Sets. Nucleic Acids Res. 49 (D1), D605–d612. doi:10.1093/nar/gkaa1074
Wang, J., Wang, F., Zhu, J., Song, M., An, J., and Li, W. (2018). Transcriptome Profiling Reveals PHLDA1 as a Novel Molecular Marker for Ischemic Cardiomyopathy. J. Mol. Neurosci. 65 (1), 102–109. doi:10.1007/s12031-018-1066-6
Wang, H. B., Huang, R., Yang, K., Xu, M., Fan, D., Liu, M. X., et al. (2019). Identification of Differentially Expressed Genes and Preliminary Validations in Cardiac Pathological Remodeling Induced by Transverse Aortic Constriction. Int. J. Mol. Med. 44 (4), 1447–1461. doi:10.3892/ijmm.2019.4291
Yang, K.-C., Yamada, K. A., Patel, A. Y., Topkara, V. K., George, I., Cheema, F. H., et al. (2014). Deep RNA Sequencing Reveals Dynamic Regulation of Myocardial Noncoding RNAs in Failing Human Heart and Remodeling with Mechanical Circulatory Support. Circulation 129 (9), 1009–1021. doi:10.1161/circulationaha.113.003863
Yu, G., Wang, L.-G., Han, Y., and He, Q.-Y. (2012). clusterProfiler: an R Package for Comparing Biological Themes Among Gene Clusters. OMICS: A J. Integr. Biol. 16 (5), 284–287. doi:10.1089/omi.2011.0118
Zhang, J., Wu, G., and Dai, H. (2016). The Matricellular Protein CCN1 Regulates TNF-α Induced Vascular Endothelial Cell Apoptosis. Cel. Biol. Int. 40 (1), 1–6. doi:10.1002/cbin.10469
Keywords: ischemic cardiomyopathy, gene signature, feature selection, TRMT5, mitochondrial membrane potential, apoptosis
Citation: Song H, Chen S, Zhang T, Huang X, Zhang Q, Li C, Chen C, Chen S, Liu D, Wang J, Tu Y, Wu Y and Liu Y (2022) Integrated Strategies of Diverse Feature Selection Methods Identify Aging-Based Reliable Gene Signatures for Ischemic Cardiomyopathy. Front. Mol. Biosci. 9:805235. doi: 10.3389/fmolb.2022.805235
Received: 30 October 2021; Accepted: 24 January 2022;
Published: 01 March 2022.
Edited by:
Leming Sun, Northwestern Polytechnical University, ChinaReviewed by:
Weijian Huang, First Affiliated Hospital of Wenzhou Medical University, ChinaHaichang Li, The Ohio State University, United States
Copyright © 2022 Song, Chen, Zhang, Huang, Zhang, Li, Chen, Chen, Liu, Wang, Tu, Wu and Liu. This is an open-access article distributed under the terms of the Creative Commons Attribution License (CC BY). The use, distribution or reproduction in other forums is permitted, provided the original author(s) and the copyright owner(s) are credited and that the original publication in this journal is cited, in accordance with accepted academic practice. No use, distribution or reproduction is permitted which does not comply with these terms.
*Correspondence: Jiawen Wang, d2p3d2ZzQDEyNi5jb20=; Yingfeng Tu, dHlmZG9jdG9yQDE2My5jb20=; Yueheng Wu, d3V5dWVoZW5nQGdkcGgub3JnLmNu; Youbin Liu, bGl1eW91YmluMjAyMUAxNjMuY29t
†These authors have contributed equally to this work