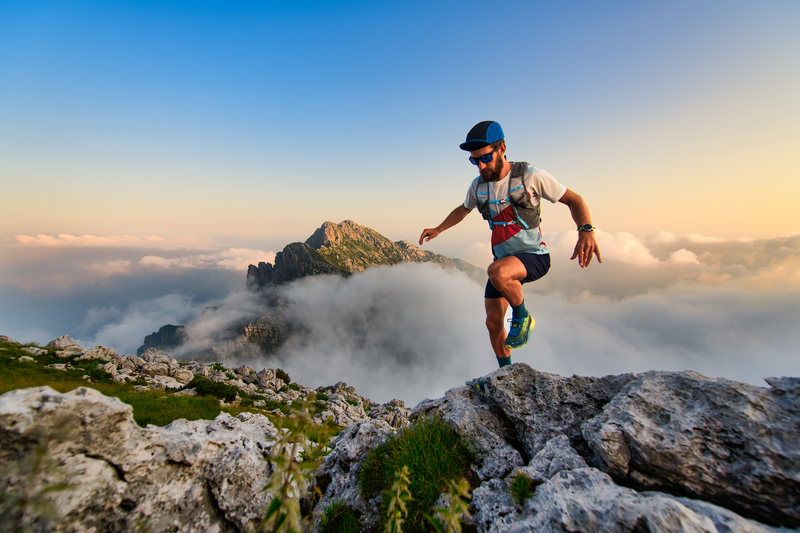
95% of researchers rate our articles as excellent or good
Learn more about the work of our research integrity team to safeguard the quality of each article we publish.
Find out more
EDITORIAL article
Front. Mol. Biosci. , 25 November 2022
Sec. Molecular Diagnostics and Therapeutics
Volume 9 - 2022 | https://doi.org/10.3389/fmolb.2022.1089463
This article is part of the Research Topic Application of Systems Biology in Molecular Characterization and Diagnosis of Cancer, Volume II View all 6 articles
Editorial on the Research Topic
Application of systems biology in molecular characterization and diagnosis of cancer, Volume II
Cancer is the leading death cause worldwide, and it is one of the biggest contributors for premature mortality (Bray et al., 2021). According to the global cancer statistics in 2020, 19.3 million new cancer cases were identified, and 10 million cancer patients were died (Sung et al., 2021). Normally, due to the heterogeneity in individual tumor, many cancer patients have distinct and diverse cancer cells, and are resistant to current drug therapies (Dagogo-Jack and Shaw, 2018). Thus, understanding molecular mechanisms and influencing factors during cancer incidence and development might provide effective prevent, diagnosis, and treatment strategies (Elmore et al., 2021). Systems biology can give insight into the complex biological systems and provide promising tools to recover underlying cancer molecular characterization, which would help establish personalized precise cancer diagnosis and therapy strategies (Shi et al., 2020). Therefore, application of systems biology in molecular characterization and diagnosis of cancer is of great interest.
Breast cancer was the most commonly diagnosed cancers in 2020, and about 2.3 million new breast cancer cases were diagnosed, which composed 11.7% of all the diagnosed cancer. Breast cancer was the fifth leading cause of cancer death, and 684,996 breast cancer patients were died in 2020 (Sung et al., 2021). Liu et al. developed a novel YTHDF3-based model via analyzing expression profiles derived from the cancer genome atlas. This model can be used to predict the overall survival of breast cancer patients and evaluate the treatment of current therapeutic agents for breast cancer patients. Therefore, this novel model would improve the therapeutic effects for breast cancer patients in the future. Asim et al. revealed the underlined mechanism of one epigenetic tumor suppressor gene of Runt-related transcription factor 3 (RUNX3) using formal model and machine learning strategies. Moreover, they identified a potential epigenetic drug target of DNA methyltransferase 1 (DNMT1) for breast cancer patients.
The development of high-throughput sequencing generates abundant multi-omics data of cancer patients, and provides the opportunities to develop effective diagnosis and prognosis strategies for cancers. Gao et al. identified key hub genes in the development and progression of hepatocellular carcinoma using bioinformatic analysis and established a prognostic model. They further verified these key genes and the model using International Cancer Genome Consortium (ICGC) dataset. This might bring effective hepatocellular carcinoma prediction and diagnosis methods for the patients. Guo et al. identified eight necroptosis-related genes related with the glioma immune microenvironment via single-cell and bulk RNA sequencing data. This might be used to predict the prognosis of glioma and provide precise glioma investigation. Fan et al. found that ferroptosis and immunity might participate in the progression of thyroid carcinoma. These results had potential application in prediction of the prognosis and clinical treatment of thyroid carcinoma.
The application of systems biology can recover potential prediction, diagnosis, and therapy treatment strategies for diverse cancers, which would lead to design personalized and precise cancer treatments. As the cancer data are big, machine learning and other artificial intelligence methods should be introduced to investigate cancer diseases (Elemento et al., 2021). Moreover, gut microbiota is associated with diverse diseases and has effects on drug effectiveness (Yang et al., 2021; Sugimura et al., 2022; Yang et al., 2022). Thus, recovering the causality between gut microbiota and cancer and engineering gut microbiota of cancer patients would contribute to future cancer treatments (Zhou et al., 2021).
YW, CZ, AM, and PZ conceived the study. YW wrote the manuscript, and others revised the manuscript. All authors approved the manuscript.
This work was supported by grants from the National Key Research and Development Program of China (Grant No. 2019YFB1503904), Knut and Alice Wallenberg Foundation (No: 72110) and the National Natural Science Foundation of China (Grant No. 32111530179).
The authors declare that the research was conducted in the absence of any commercial or financial relationships that could be construed as a potential conflict of interest.
All claims expressed in this article are solely those of the authors and do not necessarily represent those of their affiliated organizations, or those of the publisher, the editors and the reviewers. Any product that may be evaluated in this article, or claim that may be made by its manufacturer, is not guaranteed or endorsed by the publisher.
Bray, F., Laversanne, M., Weiderpass, E., and Soerjomataram, I. (2021). The ever-increasing importance of cancer as a leading cause of premature death worldwide. Cancer 127, 3029–3030. doi:10.1002/cncr.33587
Dagogo-Jack, I., and Shaw, A. T. (2018). Tumour heterogeneity and resistance to cancer therapies. Nat. Rev. Clin. Oncol. 15, 81–94. doi:10.1038/nrclinonc.2017.166
Elemento, O., Leslie, C., Lundin, J., and Tourassi, G. (2021). Artificial intelligence in cancer research, diagnosis and therapy. Nat. Rev. Cancer 21, 747–752. doi:10.1038/s41568-021-00399-1
Elmore, L. W., Greer, S. F., Daniels, E. C., Saxe, C. C., Melner, M. H., Krawiec, G. M., et al. (2021). Blueprint for cancer research: Critical gaps and opportunities. Ca. Cancer J. Clin. 71, 107–139. doi:10.3322/caac.21652
Shi, X., Wei, Y., and Ji, B. (2020). Systems biology of gastric cancer: Perspectives on the omics-based diagnosis and treatment. Front. Mol. Biosci. 7, 203. doi:10.3389/fmolb.2020.00203
Sugimura, N., Li, Q., Chu, E. S. H., Lau, H. C. H., Fong, W., Liu, W., et al. (2022). Lactobacillus gallinarum modulates the gut microbiota and produces anti-cancer metabolites to protect against colorectal tumourigenesis. Gut 71, 2011–2021. doi:10.1136/gutjnl-2020-323951
Sung, H., Ferlay, J., Siegel, R. L., Laversanne, M., Soerjomataram, I., Jemal, A., et al. (2021). Global cancer statistics 2020: GLOBOCAN estimates of incidence and mortality worldwide for 36 cancers in 185 countries. Ca. Cancer J. Clin. 71, 209–249. doi:10.3322/caac.21660
Yang, Y., Li, X., Yang, Y., Shoaie, S., Zhang, C., Ji, B., et al. (2021). Advances in the relationships between cow’s milk protein allergy and gut microbiota in infants. Front. Microbiol. 12, 716667. doi:10.3389/fmicb.2021.716667
Yang, Y., Qu, L., Mijakovic, I., and Wei, Y. (2022). Advances in the human skin microbiota and its roles in cutaneous diseases. Microb. Cell Fact. 21, 176. doi:10.1186/s12934-022-01901-6
Keywords: cancer, systems biology, precision medicine, multi-omics, molecular subtype
Citation: Wei Y, Zhang C, Mardinoglu A and Zhang P (2022) Editorial: Application of systems biology in molecular characterization and diagnosis of cancer, Volume II. Front. Mol. Biosci. 9:1089463. doi: 10.3389/fmolb.2022.1089463
Received: 04 November 2022; Accepted: 21 November 2022;
Published: 25 November 2022.
Edited by:
William C. Cho, QEH, Hong Kong SAR, ChinaReviewed by:
Muhammad Khan, University of the Punjab, PakistanCopyright © 2022 Wei, Zhang, Mardinoglu and Zhang. This is an open-access article distributed under the terms of the Creative Commons Attribution License (CC BY). The use, distribution or reproduction in other forums is permitted, provided the original author(s) and the copyright owner(s) are credited and that the original publication in this journal is cited, in accordance with accepted academic practice. No use, distribution or reproduction is permitted which does not comply with these terms.
*Correspondence: Yongjun Wei, WW9uZ2p1bndlaUB6enUuZWR1LmNu; Cheng Zhang, Y2hlbmcuemhhbmdAc2NpbGlmZWxhYi5zZQ==
Disclaimer: All claims expressed in this article are solely those of the authors and do not necessarily represent those of their affiliated organizations, or those of the publisher, the editors and the reviewers. Any product that may be evaluated in this article or claim that may be made by its manufacturer is not guaranteed or endorsed by the publisher.
Research integrity at Frontiers
Learn more about the work of our research integrity team to safeguard the quality of each article we publish.