- 1Laboratory of Molecular Neuropathology, Department of Pharmacology, Jiangsu Key Laboratory of Neuropsychiatric Diseases and College of Pharmaceutical Sciences, Soochow University, Suzhou, Jiangsu, China
- 2Bioinformatics Lab. (Dry), Department of Statistics, University of Rajshahi, Rajshahi, Bangladesh
Triple-negative breast cancer (TNBC) is one of the most lethal subtypes of breast cancer (BC), and it accounts for approximately 10%–20% of all invasive BCs diagnosed worldwide. The survival rate of TNBC in stages III and IV is very low, and a large number of patients are diagnosed in these stages. Therefore, the purpose of this study was to identify TNBC-causing molecular signatures and anti-TNBC drug agents for early diagnosis and therapies. Five microarray datasets that contained 304 TNBC and 109 control samples were collected from the Gene Expression Omnibus (GEO) database, and RNA-Seq data with 116 tumor and 124 normal samples were collected from TCGA database to identify differentially expressed genes (DEGs) between TNBC and control samples. A total of 64 DEGs were identified, of which 29 were upregulated and 35 were downregulated, by using the statistical limma R-package. Among them, seven key genes (KGs) were commonly selected from microarray and RNA-Seq data based on the high degree of connectivity through PPI (protein–protein interaction) and module analysis. Out of these seven KGs, six KGs (TOP2A, BIRC5, AURKB, ACTB, ASPM, and BUB1B) were upregulated and one (EGFR) was downregulated. We also investigated their differential expression patterns with different subtypes and progression stages of BC by the independent datasets of RNA-seq profiles from UALCAN database, which indicated that they may be potential biomarkers for early diagnosis. Gene Ontology (GO) and the Kyoto Encyclopedia of Genes and Genomes (KEGG) pathway enrichment analyses with the proposed DEGs were performed using the online Enrichr database to investigate the pathogenetic processes of TNBC highlighting KGs. Then, we performed gene regulatory network analysis and identified three transcriptional (SOX2, E2F4, and KDM5B) and three post-transcriptional (hsa-mir-1-3p, hsa-mir-124-3p, and hsa-mir-34a-5p) regulators of KGs. Finally, we proposed five KG-guided repurposable drug molecules (imatinib, regorafenib, pazopanib, teniposide, and dexrazoxane) for TNBC through network pharmacology and molecular docking analyses. These drug molecules also showed significant binding performance with some cancer-related PTM-sites (phosphorylation, succinylation, and ubiquitination) of top-ranked four key proteins (EGFR, AURKB, BIRC5, and TOP2A). Therefore, the findings of this computational study may play a vital role in early diagnosis and therapies against TNBC by wet-lab validation.
Introduction
Breast cancer (BC) is a major public health concern as it is one of the most common types of hormone-sensitive cancers and the most commonly diagnosed cancer in women worldwide. GLOBOCAN estimates approximately 2,261,419 (11.7% of all cancers, the most diagnosed cancer type) newly diagnosed cases, and 684,996 (6.9% of all cancers) deaths were reported due to BC in 2020 (Sung et al., 2021). There are four major molecular subtypes of BC, namely, luminal A, luminal B, HER2, and triple negative/basal-like (Yanagawa et al., 2012). TNBC is a specific subtype of BC that is estrogen receptor-negative (ER-), progesterone receptor-negative (PR-), and HER2-negative (HER2-). Although approximately 10–20% of BC is covered by TNBC, it has a higher mortality, is metastatic, and more aggressive compared to other subtypes (Kumar and Aggarwal, 2016). Although there have been significant advances in systematic treatment for BC over the decades, TNBC has not benefited from advanced treatment strategies due to the lack of TNBC-specific therapeutic targets. The 5-year overall survival rate for patients with TNBC is lower than that of other subtypes of BC (Dent et al., 2007). In particular, the 5-year overall survival rate is 91% if the cancer is diagnosed at an early stage, it drops to 65% if the cancer spreads to the lymph nodes near the breast, and eventually it drops to a very low 12% if the cancer spreads to distant sites (Society, 2022). Therefore, it is important to identify biomolecular signatures that can diagnose TNBC at an early stage and play a vital role as therapeutic targets in treatment strategies to reduce mortality in TNBC patients. The TNBC-driving genes may play the key role in this regard. There are some transcriptomics studies exploring TNBC-driving key genes (KGs). However, we observed that their individual KG-sets were not so consistent (see Supplementary Table S1 in Supplementary File S1). It may have happened due to the environmental variations, small sample sizes, and selection of inappropriate statistical models. They did not validate their suggested KGs against the other independent databases. Also, they did not explore their suggested KG-guided candidate drug molecules for the treatment against TNBC. Therefore, the main purpose of this study was to explore more consistent TNBC-driving KGs for early diagnosis and therapies, giving the weight to a large sample size, appropriate statistical model, and the datasets from different environment.
Transcriptomics analysis is one of the most popular platforms for identifying disease-driving genes (Li et al., 2021; Yuan et al., 2021; Alam et al., 2022a; Alam et al., 2022b; Alam et al., 2022c; Ma et al., 2022). In this study, we created transcriptomics datasets of large sample sizes from NCBI-GEO and TCGA databases to identify more consistent TNBC-driving KGs for diagnosis at an early-stage. To make a large sample size, we combined five environmentally independent microarray gene-expression datasets from the NCBI-GEO database that contained 304 TNBC and 109 control samples in total. On the other hand, the RNA-Seq profiles from TCGA database consisted of 116 TNBC and 124 control samples that were generated from different environments. In the case of drug discovery, drug repositioning (DR) is a promising strategy for discovering new therapies through existing drugs (Langedijk et al., 2015). The DR strategy is efficient, safe, less expensive, and less time-consuming than the de novo technique (Rudrapal et al., 2020). Therefore, in this study, we also attempted to explore KG-guided repurposable drug-molecules through the network pharmacology and molecular docking analysis. Network pharmacology is an efficient tool to generate interactions between therapeutic targets (genes) and drug molecules through a network-based approach (Hopkins, 2007; Song et al., 2019). Recently, molecular docking analysis has gained popularity in the field of computational research to identify therapeutic target-related candidate drugs (Alam et al., 2022a; Alam et al., 2022b). In this study, we considered well-established bioinformatics tools and statistical methods to identify biomolecular signatures and candidate drug agents that may play an effective role in the early diagnosis and treatment of TNBC patients. The whole workflow of this study is displayed in Figure 1.
Methods and materials
Data collection
A total of 304 TNBC samples and 109 normal samples were collected from five sets of omics data (gene expression profiles data) with accession numbers GSE65216 (Dubois, 2019), GSE76275 (Fuqua, 2019), GSE38959 (Komatsu and Katagiri, 2018), GSE27447 (Yang et al., 2018), and GSE61724 (Mathe et al., 2018), and 116 tumor samples and 124 normal samples were collected from RNA-Seq data. Datasets were downloaded from the public database Gene Expression Omnibus (GEO) in the National Center of Biotechnology Information (NCBI) (Edgar and Barrett, 2006) and TCGA database (https://www.cancer.gov/about-nci/organization/ccg/research/structural-genomics/tcga). Two datasets (GSE65216 and GSE76275) are based on the platform GPL570 Affymetrix Human Genome U133 Plus 2.0 Array, two datasets (GSE27447 and GSE61724) are based on the platform GPL6244 Affymetrix Human Gene 1.0 ST Array, and the last one (GSE38959) is based on the platform GPL4133 Agilent-014850 Whole Human Genome Microarray. More details of the datasets are given in Table 1.
Data preprocessing and DEG identification
Background correction, standardization, and median polish summarization were performed on the raw data through the robust multichip average (RMA) algorithm analysis (Irizarry et al., 2003). Then, we converted the probe IDs to official gene symbols according to the annotation files and merge all datasets. After combining the five datasets, we obtained a total number of samples of 413 including 304 TNBC and 109 normal. We considered the limma (Linear Models for Microarray Analysis) statistical approach (Ritchie et al., 2015), which was implemented in the limma (v- 3.44.3) R-package to identify differentially expressed genes (DEGs) between tumor and normal samples. The moderated t-statistic was used to test the null hypothesis (H0) (equally expressed gene (EEG) in both tumor and control groups) versus the alternative hypothesis (H1) (differentially expressed gene (DEG) between tumor and control groups). We have considered the threshold to identify the up- and down-regulated DEGs for the combined data as follows:
where adj. p-value: adjusted p-values and Log2FC: Log2 (fold change).
PPI network and module analysis
We used the online database Search Tool for the Retrieval of Interacting Genes (STRING) to construct the PPI network of DEGs and visualized it using Cytoscape software (Shannon et al., 2003; Szklarczyk et al., 2019). Module analysis was performed using the MCODE app in Cytoscape with the threshold degree = 2, haircut cluster, node_score = 0.2, k-core = 2, and max. depth = 100.
The association of KGs with different subtypes and progression stages of BC.
We investigated the association of KGs with different subtypes and progression stages of BC using a box plot of their independent RNA-Seq profiles from the UALCAN online database (http://gepia.cancer-pku.cn/index.html) (Chandrashekar et al., 2022). This RNA-Seq dataset contained a total of 114 normal samples and 719 BC samples (luminal = 566, HER2-positive = 37, and triple-negative = 116). On the other hand, the samples sizes are 183, 615, 247, and 20 in stage 1, stage 2, stage 3, and stage 4, respectively.
GO and KEGG enrichment analysis of DEGs
Gene Ontology (GO) analysis was performed to define and describe genes across species in three categories including biological process (BP), cellular component (CC), and molecular function (MF). The Kyoto Encyclopedia of Genes and Genomes (KEGG) is the databases of drugs, genomes, biological pathways, enzymes etc. GO functional and KEGG pathway enrichment analyses were performed using the online tool Enrichr (Chen et al., 2013).
Regulatory interaction network of KGs
We performed the regulatory interaction network (TF-KG-miRNA) to identify the transcriptional and post-transcriptional regulators of KGs. The regulatory interaction network was constructed using the online tool “NetworkAnalyst” (version: 3.0) (Zhou et al., 2019). The ChEA database was selected for constructing a KG-TF interaction network and miRTarBase database for a KG-miRNA interaction network.
Drug repositioning
To propose candidate drug molecules for treatment against TNBC, we performed network pharmacology and molecular docking analyses. We considered our proposed KGs as drug target receptor proteins. KG-guided drug agents were detected by the Drug–Gene Interaction Database (DGIdb) through network pharmacology analysis (Wagner et al., 2016). Molecular docking analysis was performed to examine structural binding between receptor proteins and drug agents. We collected 3-D (three-dimensional) structures of both protein receptors and drug agents for molecular docking analysis. We downloaded the 3D structures of all receptor proteins such as TOP2A, BIRC5, EGFR, and AURKB with PDB IDs 1zxm, 1xox, 3GKW, and 4af3, respectively, from the Protein Data Bank (PDB) database (Berman et al., 2000). The 3D structures of drug agents were downloaded from the PubChem database (Kim et al., 2019). Pymol was used to preprocess the 3D structures of ligands, compounds, and the water molecules, and co-crystal ligands which were bound to the protein were removed (DeLano and Bromberg, 2004). We performed molecular docking analysis between the proposed receptors and drug molecules to calculate binding affinity scores (kcal/mol) through the AutoDock Vina in PyRx software (Trott and Olson, 2010; Dallakyan and Olson, 2015). The 3D and 2D structures of interaction between the proposed receptors and top-ranked drug molecules were constructed and visualized using USCF Chimera program and Discovery Studio Visualizer 2021 software (Pettersen et al., 2004). Then, we validated the selected drug molecules with the cancer-related post-translational modification (PTM) sites (phosphorylation, ubiquitination, and succinylation) through docking analysis (Hasan et al., 2016; Wang et al., 2020; Holstein et al., 2021; Mu et al., 2021).
Result
Identification of DEGs
We identified a total of 64 DEGs, including 29 up- and 35 down-regulated DEGs between 304 TNBC samples and 109 normal samples in Supplementary File S2, and 306 DEGs including 68 upregulated and 238 downregulated for RNA-Seq data in Supplementary File S3. We visualize the heatmap of hierarchical clustering (HC) for 64 DEGs in Figure 2, where each row represents a single gene and each column represents a sample. We observed that the heatmap clearly classified upregulated and downregulated DEGs, and also tumor and normal samples, where yellow, green, pink, and turquoise indicated downregulated DEGs, upregulated DEGs, normal samples, and tumor samples, respectively.
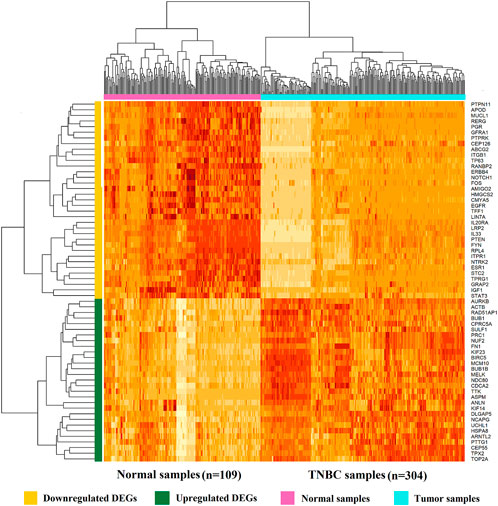
FIGURE 2. Heatmap of 64 identified DEGs based on integrated microarray analysis of five datasets. Each row represents a single gene, and each column represents a tissue (sample).
Identification of key genes (KGs) by PPI network and cluster analysis
The PPI network of DEGs for NCBI data included 64 nodes, 427 edges, average node degree of 13.3, and PPI enrichment p-value < 1.0e-16. The PPI network of DEGs for TCGA data included 298 nodes, 1,371 edges, average node degree 9.2, and PPI enrichment p-value < 1.0e-16. Figure 3A represents the interactions between oncogenes and tumor suppressor genes detected from five NCBI gene-expression profiles, and Figure 3B represents the interactions between oncogenes and tumor suppressor genes detected from TCGA RNA-Seq profiles, where yellow indicates downregulated (tumor suppressor genes), green indicates upregulated (oncogenes), and the parallelogram indicates the common KGs. Then, we selected 10 top-ranked Hub-DEGs for each of NCBI and TCGA datasets. Then, we found seven common Hub-DEGs (TOP2A, BIRC5, AURKB, ACTB, ASPM, BUB1B, and EGFR) between two independent sets of 10 top-ranked Hub-DEGs (see Figures 3A,B). Then, we performed their module analysis and observed that the PPI network of NCBI data produces two modules between oncogenes and tumor suppressor genes with around a 6% clustering error rate including 10 Hub-DEGs with the highest degrees. Furthermore, we found only two modules of DEGs for NCBI data with scores 23 and 12.7. Also, we found a total of 12 modules of DEGs for TCGA data with scores ranging from 31 to 3 through MCODE analysis, and two top-ranked modules for both data are presented in Supplementary Figures S1A,B in Supplementary File S1. Finally, six common upregulated KGs (BIRC5, TOP2A, ASPM, ACTB, BUB1B, and AURKB) and one common downregulated KG (EGFR) were found in module 1 and module 2, respectively, with the highest degree of connectivity for both NCBI and TCGA data in Table 2. The original results of the aforementioned analysis are presented in Supplementary Files S4,S5.
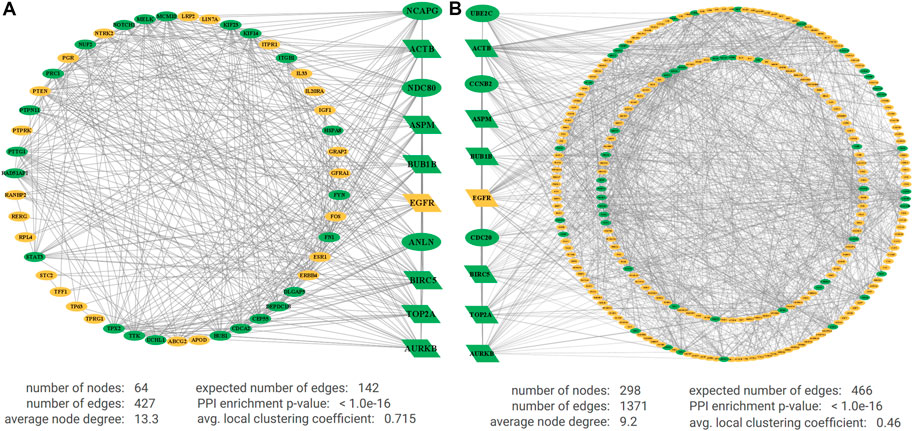
FIGURE 3. Protein–protein interaction (PPI) networks for (A) DEGs for microarray data and (B) DEGs for RNA-Seq data. The green indicates upregulated and yellow indicates downregulated DEGs, and the larger nodes in the center are KGs with the highest degree of connectivity.
To investigate the similarity and consistency of different computational results with KGs, we summarized them in Table-2. We observed that limma and an FC recommended cluster with the upregulated KGs (TOP2A, BIRC5, and AURKB) and the cluster with downregulated KGs (EGFR) are supported by the PPI and MCODE-mediated clusters 1 and 2, respectively. Evidently, results are consistent and support each other. To validate the differential expression patterns of the identified KGs, we considered both dependent and independent test-datasets. Figure 4 shows the HC results based on the expressions of seven KGs with 304 TNBC and 109 control samples (dependent data). We observed that HC clearly separated KGs into upregulated and downregulated groups, and samples/patients in TNBC and control groups.
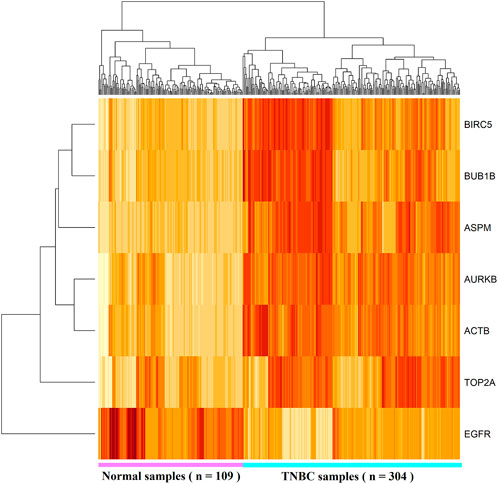
FIGURE 4. Heatmap of hierarchical clustering (HC) results based on the expressions of seven KGs with 304 TNBC and 109 control samples (dependent data).
Association of KGs with different subtypes and progression stages of BC
To investigate the association of KGs (AURKB, BIRC5, TOP2A, ACTB, ASPM, EGFR, and BUB1B) with different subtypes and progression stages of BC by the independent datasets, we performed box plot analysis based on independent RNA-Seq profiles from the UALCAN online database. Figure 5 displayed the box plots for the expressions of KGs with each BC subtypes (normal, luminal, HER2-positive, and TNBC), where blue indicates the normal group and orange, green, and brown indicate luminal, HER2-positive, and triple-negative BC, respectively. We observed the significant differential expression patterns between the normal group and the subtypes of the BC group. Supplementary Figure S2 shows that the expression patterns of KGs with different BC progression stages significantly differentiated from the control group. Therefore, proposed KGs (AURKB, BIRC5, TOP2A, ACTB, ASPM, EGFR, and BUB1B) may play a significant role for the diagnosis of TNBC at the earlier stage.
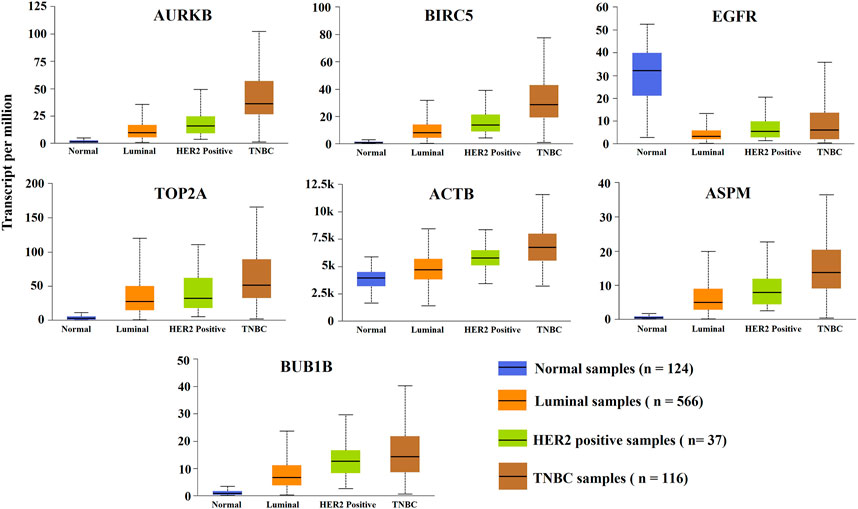
FIGURE 5. Expression levels’ analysis of KGs between the normal group and the three major subtypes of BC (luminal, HER2-positive, and triple-negative BC).
GO terms and KEGG pathway enrichment analysis of DEGs
GO and KEGG enrichment analysis showed that our identified DEGs were enriched by 63 BP-terms, 14 CC-terms, 19 MF-terms, and 48 KEGG-terms, and the threshold p < 0.01 was considered. The top 10 significantly enriched terms for each category are presented in Figures 6A–D. We observed that mitotic spindle organization (p = 2.4E-15, DEGs involved = 25%), microtubule cytoskeleton organization involved in mitosis (p = 9.1E-12, DEGs involved = 21%), intracellular membrane-bound organelle (p = 6.8E-08, DEGs involved = 58%), nucleus (p = 6.3E-08, DEGs involved = 53%), kinase binding (p = 1.9E-06, DEGs involved = 18%), and protein kinase binding (p = 4.3E-06, DEGs involved = 22%) were significantly enriched GO terms in TNBC in Figures 6A–C. Proteoglycans in cancer (p = 9.6E-10, DEGs involved = 21%), estrogen signaling pathway (p = 2.7E-07, DEGs involved = 27%), and pathways in cancer (p = 6.7E-06, DEGs involved = 15%) were significantly enriched KEGG pathways terms in Figure 6D. We included further information of GO and KEGG analysis in Supplementary Table S2 in Supplementary File S1.
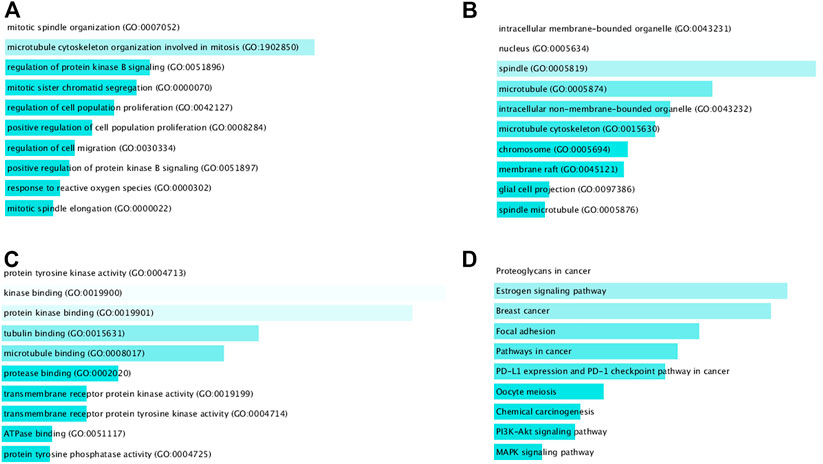
FIGURE 6. Top 10 significantly enriched terms for each category of DEGs. (A) GO BP-terms, (B) GO CC-terms, (C) GO MF-terms, and (D) KEGG pathways.
Regulatory interaction network of KGs
The regulatory interaction network (TF-KG-miRNA) is shown in Figure 7; where pink indicates TFs (diamond) and miRNAs (ellipse) and yellow indicates KGs. Then, we observed that three TFs (SOX2, E2F4, and KDM5B) and three miRNAs (hsa-mir-1-3p, hsa-mir-124-3p, and hsa-mir-34a-5p) are connected with all KGs (degree = 7). Thus, we considered these three TFs and three miRNAs as transcriptional and post-transcriptional factors of KGs.
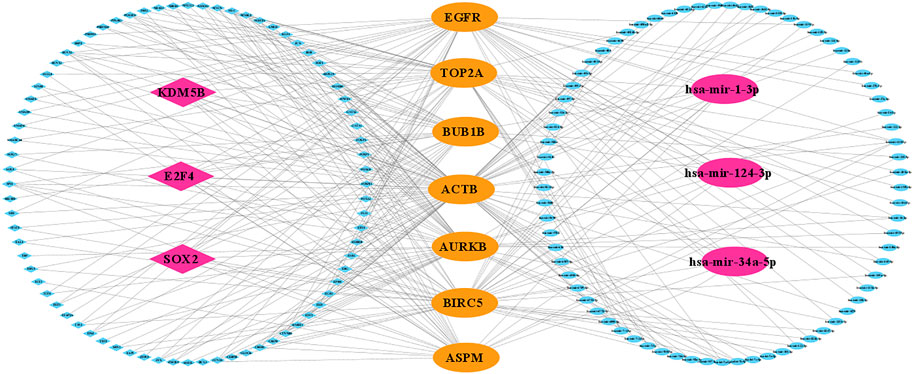
FIGURE 7. KG-regulatory (TF and miRNA) interaction network, where yellow indicates KGs and pink indicates key TFs (diamond) and key miRNAs (ellipse).
Drug repositioning
First, we collected a total of 115 FDA-approved drugs related to KGs from the DGIdb database and published articles and considered them as drug agents for further analysis. Then, structural interactions between KGs and drug agents were performed and binding affinity scores (BAS) (kCal/mol) were calculated by molecular docking analysis. We presented the molecular docking results of the top-ranked interaction with BAS < −7 in Table 3. Five candidate drugs, namely, imatinib, regorafenib, pazopanib, teniposide, and dexrazoxane, are proposed for TNBC based on a threshold of BAS < −10 and highlighted by asterisks (*) in Table 3. The 3D (Three-dimensional) structural interactions of ligands (candidate drugs) and receptors (KGs) and their active sites are presented in Figure 8. The 2D (two-dimensional) structural binding of ligands and receptors and their interaction types are presented in Figure 9.
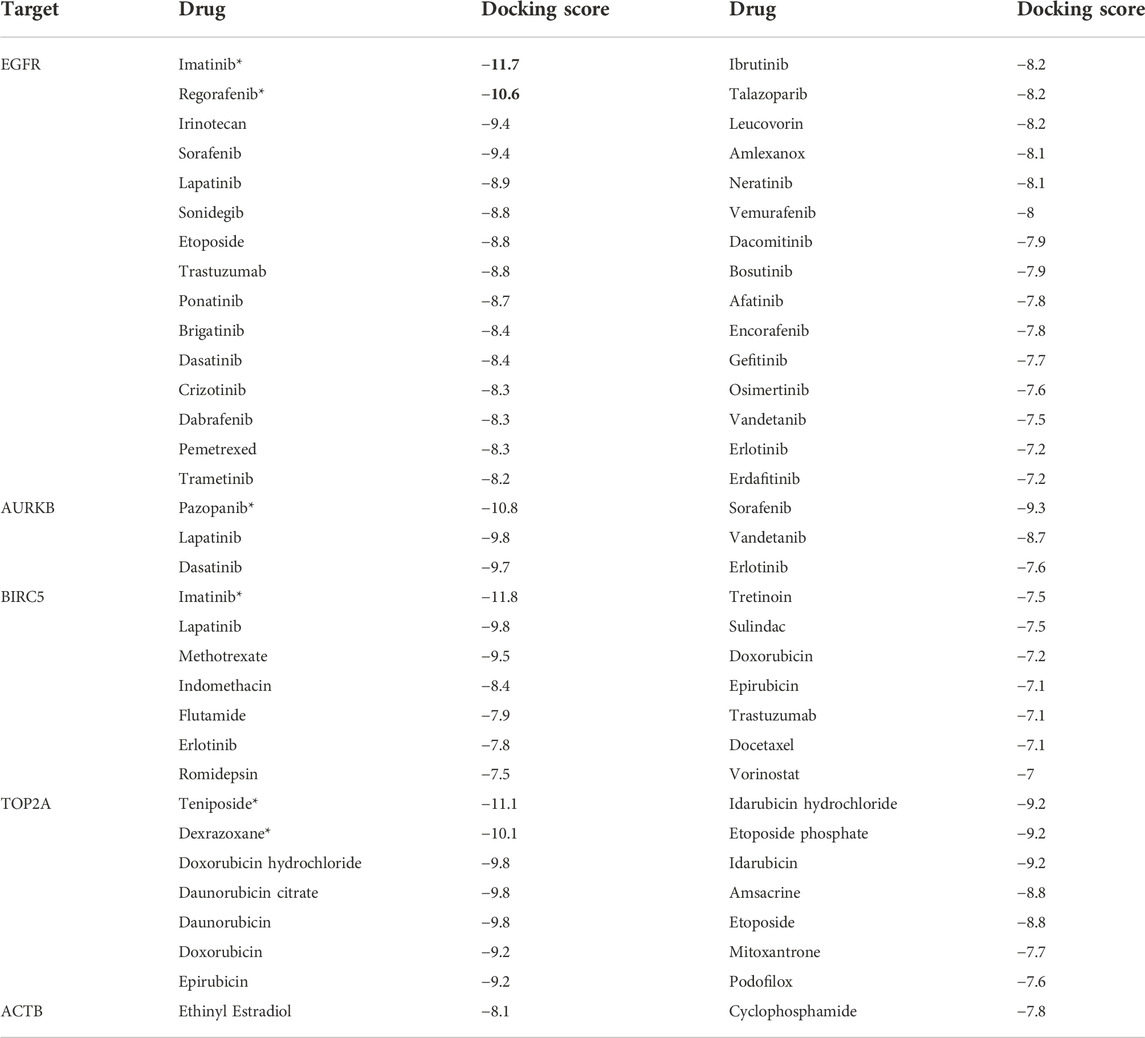
TABLE 3. List of drugs associated with corresponding targets, calculated binding affinity scores (BAS), and proposed candidate drugs highlighted by bold and star (*).
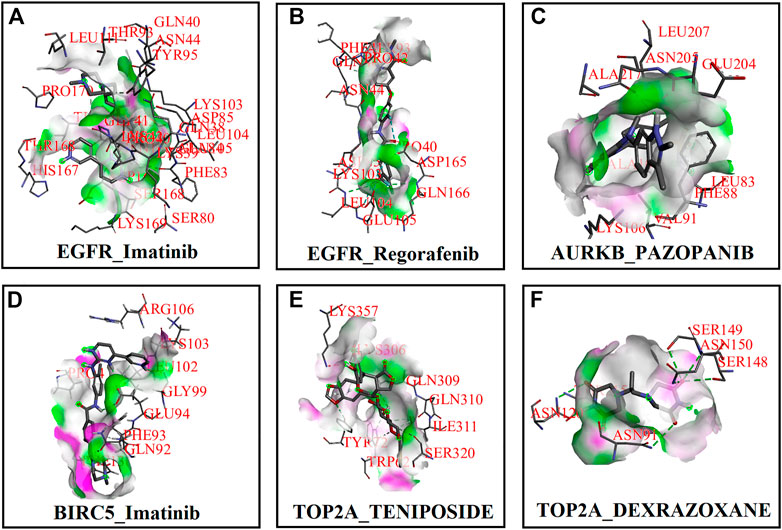
FIGURE 8. Three-dimensional (3D) structures between receptor proteins and candidate drugs and highlighting active site. (A) EGFR–imatinib, (B) EGFR–regorafenib, (C) AURKB–pazopanib, (D) BIRC5–imatinib, (E) TOP2A–teniposide, and (F) TOP2A–dexrazoxane.
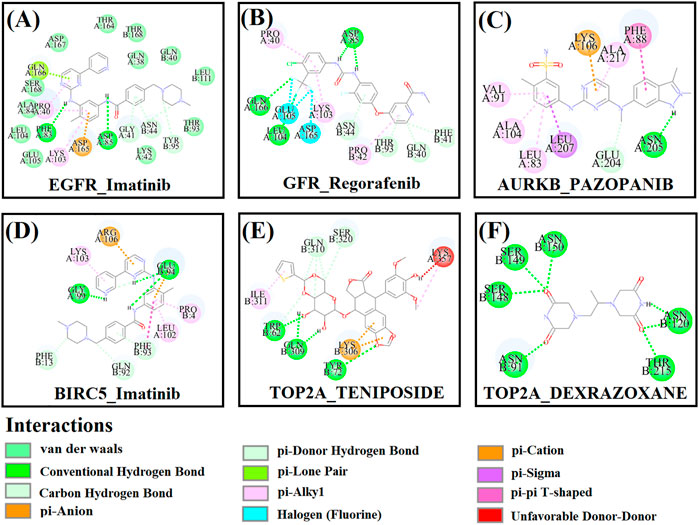
FIGURE 9. Two-dimensional (2D) structures between receptor proteins and candidate drugs and highlighting interaction types. (A) EGFR–imatinib, (B) EGFR–regorafenib, (C) AURKB–pazopanib, (D) BIRC5–imatinib, (E) TOP2A–teniposide, and (F) TOP2A–dexrazoxane.
To validate the top-ranked drug molecules with some cancer-related PTM sites of top-ranked key proteins (EGFR, AURKB, BIRC5, and TOP2A) by their docking analysis, we predicted the their PTM sites (phosphorylation, succinylation, and ubiquitination) by the web-based prediction models MusiteDeep (https://www.musite.net/) and SuccinSite (http://systbio.cau.edu.cn/SuccinSite/). We found seven, seven, four, and six phosphorylated PTM sites in chain A and three, five, four, and six PTM sites in chain B for proteins EGFR, AURKB, BIRC5, and TOP2A, respectively (Supplementary File S6). Similarly, we found a total of 15 succinylated PTM sites in chain A and 11 PTM sites in chain B, and 11 ubiquitinated PTM sites in chain A and nine PTM sites in chain B (in Supplementary File S7,S8). We observed that almost all active sites of blind docking are common with the predicted PTM sites (Figure 9). Furthermore, we performed the docking analysis between the predicted PTM sites and candidate drug molecules and observed their significant binding affinity scores (Table 4).
Discussion
In this study, we identified 64 DEGs between 304 TNBC and 109 control samples (Supplementary File S2) by combining five transcriptomics datasets of NCBI with GEO accession numbers GSE65216, GSE76275, GSE38959, GSE27447, and GSE61724, and 306 DEGs between 116 TNBC and 124 control samples of RNA-Seq profiles from TCGA dataset (Supplementary File S3). Then, we constructed two sets of 10 top-ranked key-DEGs for both DEGs-sets by PPI network and their module analysis. We found seven common key-DEGs (TOP2A, BIRC5, EGFR, AURKB, ACTB, ASPM, and BUB1B) in both key DEGs-sets (Figures 3A,B, and Supplementary Figures S1A,B), where six KGs (TOP2A, BIRC5, AURKB, ACTB, ASPM, and BUB1B) were upregulated and only one gene (EGFR) was downregulated (Table 2). Several studies have already proposed our identified KGs as TNBC-causing genes, which strongly supports our results. TOP2A encodes topoisomerase (DNA) II alpha, somatic mutations in a TOP2A immunohistochemical score may be important in predicting response to immunotherapy treatment for triple-negative breast cancer (Jiang et al., 2021). Several studies have proposed TOP2A as a prognostic marker and therapeutic target for TNBC through well-established integrated bioinformatics methods (Qiu et al., 2021; Wei et al., 2021; Ma et al., 2022). The gene expression of TOP2A and EGFR identifies efficacy of docetaxel plus epirubicin as neoadjuvant chemotherapy in TNBC patients (Liu et al., 2016). The EGFR gene encodes a receptor protein called the epidermal growth factor receptor, which causes cell proliferation, invasion, angiogenesis, and metastasis associated with poor prognosis in TNBC (Nakai et al., 2016). MicroRNA-203 may function to inhibit the proliferation and migration of TNBC cells by targeting BIRC5, so BIRC5 may be a potential therapeutic target for the treatment of TNBC patients (Wang et al., 2012). AURKB gene polymorphism can predict the overall survival or disease-free survival in TNBC patients treated with taxane-based adjuvant chemotherapy (Liao et al., 2018). The expression patterns of KGs with different subtypes of BC were significantly contrasted from the control group, where TNBC showed highly significant differences (Figure 5). The expression analysis of KGs with different BC progression stages compared to the normal stage indicated that the proposed KGs might be potential biomarkers for early diagnosis of TNBC (Supplementary Figure S2).
To investigate the pathogenetic mechanisms of TNBC with highlighting KGs, we performed GO functional and KEGG pathway enrichment analyses. We found some significant functional terms and pathways that are responsible for TNBC development which involves our identified KGs. The BP term mitotic spindle organization (involved in KG: BUB1B, AURKB, BIRC5, and TOP2A) was reported to be responsible for TNBC (Chimplee et al., 2022). Integrated bioinformatics analysis reported that microtubule cytoskeleton organization involved in mitosis (involved in KG: BUB1B, AURKB, and BIRC5) and kinase binding (involved in KG: AURKB, ACTB, EGFR, and TOP2A) are significant functional term for TNBC progression (Ryall et al., 2015; Huo et al., 2021). Therapeutic targets for TNBC treatment may interfere with the progression of TNBC by participating in the estrogen signaling pathway (involved in KG: EGFR, TOP2A, and BIRC5) (Huang et al., 2021). The regulatory interaction networks (miRNA-KG-TF) revealed three TFs proteins (SOX2, E2F4, and KDM5B) and three miRNAs (hsa-mir-1-3p, hsa-mir-124-3p, and hsa-mir-34a-5p) as the transcriptional and post-transcriptional regulators of KGs in Figure 7. The TF-protein, SOX2, has been found to be a tumor promoter and has a dynamic therapeutic strategy for TNBC (Liu et al., 2018). The expression of E2F4 is associated with lymph node metastasis in TNBC patients (Zou et al., 2022). The expression of KDM5B enhances invasive TNBC by downregulating hsa-mir-448 (Bamodu et al., 2016). Our identified three post-transcriptional regulators (hsa-mir-1-3p, hsa-mir-124-3p, and hsa-mir-34a-5p) are also supported by published articles that are associated with TNBC progression by using bioinformatics analysis and cellular experiments (Volovat et al., 2020; Zhang et al., 2021a; Zhang et al., 2021b; Shadbad et al., 2021).
We performed KG–disease interactions to check the enrichment of KGs in diseases from the DisGeNET database through the online based tool Enrichr (https://maayanlab.cloud/Enrichr/) (Chen et al., 2013), as shown in Figure 10. In the figure, we present the 15 most enriched diseases corresponding to our seven identified KGs. We observed that all KGs are highly enriched in recurrent BC, and one major subtype triple-negative BC highlighted in yellow strongly supports our proposed results. Another four diseases (malignant glioma, sarcoma, small cell carcinoma of lung, and nasopharyngeal carcinoma) also enriched all KGs, and one KG TOP2A enriched by all the top 15 diseases. Overall, the results suggested that our identified KGs may be significant for TNBC and some other diseases.
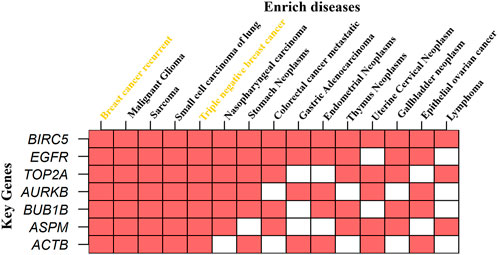
FIGURE 10. KG–disease interaction to check the enrichment of KGs in different diseases, where the X-axis represents diseases, the Y-axis represents KGs, and red indicates enriched.
To discover the potential repurposable candidate drug molecules for the treatment against TNBC, 115 KG-associated FDA-approved drugs were collected from the DGIdb database and published articles. Among them, five drugs (imatinib, regorafenib, pazopanib, teniposide, and dexrazoxane) have been proposed as the candidate drug molecules for TNBC through the molecular docking analysis (Table 3). Then, we validated them by molecular docking analysis with some cancer-related PTM-sites (phosphorylation, succinylation, and ubiquitination) of the four top-ranked key proteins EGFR, AURKB, BIRC5, and TOP2A (Table 4). Imatinib was approved by the US Food and Drug Administration (FDA) on 1 February 2001 for the treatment of malignant metastatic and unresectable gastrointestinal stromal tumors (GISTs) (Dagher et al., 2002). Imatinib (also known as Glivec and Gleevec) is used to treat GISTs, acute lymphocytic leukemia (ALL), chronic myelogenous leukemia (CML), and other malignancies (Iqbal and Iqbal, 2014). It may be a novel treatment strategy for BC patients (including lethal subtype TNBC) overexpressing geminin and nuclear c-Abl (Blanchard et al., 2014). Regorafenib, sold under the brand names Stivarga and Regonix (in Bangladesh), was approved by the FDA in 2012 and 2013 for the treatment of metastatic colorectal cancer and GISTs, respectively (Regorafenib, 2017). Mehta et al. proposed that a combination of regorafenib, angiogenesis inhibitors, and radiation may be effective in inhibiting TNBC cells (Mehta et al., 2021). The drug pazopanib, also known as Votrient, was approved by the FDA on 19 October 2009, the EMA on 14 June 2010, the MHRA on 14 June 2010, and Australia’s TGA on 30 June 2010 for the treatment of advanced renal cell carcinoma and advanced soft tissue sarcomas (Bukowski et al., 2010; Nieto et al., 2011). It may play an important role in keeping the disease stable in advanced BC (under phase II clinical trial) and also TNBC (Taylor et al., 2010; Van Swearingen et al., 2017). Teniposide (known as Vumon), commonly used for the treatment of cancer diseases in children, was approved by the FDA for the treatment of second-line therapy of ALL in combination with other antineoplastic drugs (Drugs, 2007). Dexrazoxane was approved and designated by the FDA for the treatment of extravasation resulting from IV anthracycline chemotherapy and cardiomyopathy for children (0–16 years) and adults (FDA, 2013). Our five proposed candidate drugs are not approved for TNBC/BC yet, but several computational studies have suggested that they might be effective for TNBC/BC patients. This study lends further validation for the proposed candidate drugs and target proteins in the experimental lab as a proper treatment plan against TNBC.
Conclusion
The selection of few potential target proteins and drug agents from a huge number of alternatives are equally important in drug discovery by wet-lab experiments. In this study, we identified triple-negative breast cancer (TNBC) causing seven KGs (TOP2A, BIRC5, AURKB, EGFR, ACTB, ASPM, and BUB1B) based on five NCBI-GEO microarray gene expression datasets and TCGA RNA-Seq datasets for early diagnosis and therapies of TNBC by using the integrated bioinformatics and systems biology approaches. We also confirmed their higher differential expression between normal and TNBC compared to other subtypes of BC from the other independent data source. The enrichment analysis of GO-terms and KEGG pathways with the key genes detected some crucial TNBC-related biological processes, molecular functions, cellular components, and pathways. For example, the detected mitotic spindle organization function and estrogen signaling pathway are both significantly associated with TNBC progression. The key gene (KG) regulatory network analysis detected some transcriptional and post-transcriptional regulators of KGs that are associated with TNBC progression. Finally, we proposed five KG-guided repurposable drug molecules (imatinib, regorafenib, pazopanib, teniposide, and dexrazoxane) for TNBC through network pharmacology and molecular docking analyses. Therefore, our findings would be more reliable for the wet-lab researchers and medical doctors in TNBC diagnosis and therapies at the earlier stage.
Data availability statement
The microarray gene expression datasets that analyzed in this study are available in the publicly accessible NCBI-GEO data repository as mentioned below: https://www.ncbi.nlm.nih.gov/geo/query/acc.cgi?acc=GSE65216, https://www.ncbi.nlm.nih.gov/geo/query/acc.cgi?acc=GSE76275, https://www.ncbi.nlm.nih.gov/geo/query/acc.cgi?acc=GSE38959, https://www.ncbi.nlm.nih.gov/geo/query/acc.cgi?acc=GSE27447, https://www.ncbi.nlm.nih.gov/geo/query/acc.cgi?acc=GSE61724. The PC related RNA-Seq profiles were downloaded from the TCGA data repository link https://portal.gdc.cancer.gov/projects.
Author contributions
MA, GW, and MM designed the study. MA and AS participated in data collection. MA participated in the data analysis and result validation. MA drafted the manuscript. MA, AS, GW, and MM revised the manuscript. All authors read and approved the manuscript.
Funding
This work was supported by the National Natural Science Foundation of China (No. 32070970), Taicang Science and Technology Bureau (TC2018JCYL20), and the Project Funded by the Priority Academic Program Development of Jiangsu Higher Education Institutions.
Conflict of interest
The authors declare that the research was conducted in the absence of any commercial or financial relationships that could be construed as a potential conflict of interest.
Publisher’s note
All claims expressed in this article are solely those of the authors and do not necessarily represent those of their affiliated organizations, or those of the publisher, the editors, and the reviewers. Any product that may be evaluated in this article, or claim that may be made by its manufacturer, is not guaranteed or endorsed by the publisher.
Supplementary material
The Supplementary Material for this article can be found online at: https://www.frontiersin.org/articles/10.3389/fmolb.2022.1049741/full#supplementary-material
References
Alam, M. S., Rahaman, M. M., Sultana, A., Wang, G., and Mollah, M. N. H. (2022a). Statistics and network-based approaches to identify molecular mechanisms that drive the progression of breast cancer. Comput. Biol. Med. 145, 105508. doi:10.1016/j.compbiomed.2022.105508
Alam, M. S., Sultana, A., Reza, M. S., Amanullah, M., Kabir, S. R., and Mollah, M. N. H. (2022b). Integrated bioinformatics and statistical approaches to explore molecular biomarkers for breast cancer diagnosis, prognosis and therapies. PLoS One 17, e0268967. doi:10.1371/journal.pone.0268967
Alam, M. S., Sultana, A., Sun, H., Wu, J., Guo, F., Li, Q., et al. (2022c). Bioinformatics and network-based screening and discovery of potential molecular targets and small molecular drugs for breast cancer. Front. Pharmacol. 13, 942126. doi:10.3389/fphar.2022.942126
Bamodu, O. A., Huang, W. C., Lee, W. H., Wu, A., Wang, L. S., Hsiao, M., et al. (2016). Aberrant KDM5B expression promotes aggressive breast cancer through MALAT1 overexpression and downregulation of hsa-miR-448. BMC Cancer 16, 160. doi:10.1186/s12885-016-2108-5
Berman, H. M., Westbrook, J., Feng, Z., Gilliland, G., Bhat, T. N., Weissig, H., et al. (2000). The protein Data Bank. Nucleic Acids Res. 28, 235–242. doi:10.1093/nar/28.1.235
Blanchard, Z., Mullins, N., Ellipeddi, P., Lage, J. M., McKinney, S., El-Etriby, R., et al. (2014). Geminin overexpression promotes imatinib sensitive breast cancer: A novel treatment approach for aggressive breast cancers, including a subset of triple negative. PLoS One 9, e95663. doi:10.1371/journal.pone.0095663
Bukowski, R. M., Yasothan, U., and Kirkpatrick, P. (2010). Pazopanib. Nat. Rev. Drug Discov. 9, 17–18. doi:10.1038/nrd3073
Chandrashekar, D. S., Karthikeyan, S. K., Korla, P. K., Patel, H., Shovon, A. R., Athar, M., et al. (2022). Ualcan: An update to the integrated cancer data analysis platform. Neoplasia 25, 18–27. doi:10.1016/j.neo.2022.01.001
Chen, E. Y., Tan, C. M., Kou, Y., Duan, Q., Wang, Z., Meirelles, G. V., et al. (2013). Enrichr: Interactive and collaborative HTML5 gene list enrichment analysis tool. BMC Bioinforma. 14, 128. doi:10.1186/1471-2105-14-128
Chimplee, S., Roytrakul, S., Sukrong, S., Srisawat, T., Graidist, P., and Kanokwiroon, K. (2022). Anticancer effects and molecular action of 7-alpha-Hydroxyfrullanolide in G2/M-phase Arrest and apoptosis in triple negative breast cancer cells. Molecules 27, 407. doi:10.3390/molecules27020407
Dagher, R., Cohen, M., Williams, G., Rothmann, M., Gobburu, J., Robbie, G., et al. (2002). Approval summary: Imatinib mesylate in the treatment of metastatic and/or unresectable malignant gastrointestinal stromal tumors. Clin. Cancer Res. 8, 3034–3038. doi:10.1634/theoncologist.2008-0255
Dallakyan, S., and Olson, A. J. (2015). Small-molecule library screening by docking with PyRx. Methods Mol. Biol. 1263, 243–250. doi:10.1007/978-1-4939-2269-7_19
DeLano, W. L., and Bromberg, S. (2004). PyMOL user's guide. [Online]. Available: http://pymol.sourceforge.net/newman/userman.pdf.
Dent, R., Trudeau, M., Pritchard, K. I., Hanna, W. M., Kahn, H. K., Sawka, C. A., et al. (2007). Triple-negative breast cancer: Clinical features and patterns of recurrence. Clin. Cancer Res. 13, 4429–4434. doi:10.1158/1078-0432.CCR-06-3045
Drugs (2007). Teniposide. [Online]. Available: https://www.drugs.com/monograph/teniposide.html.
Dubois, T. (2019). Expression profiling of breast cancer samples from Institut Curie (Maire cohort). [Online]. Available: https://www.ncbi.nlm.nih.gov/geo/query/acc.cgi?acc=GSE65216.
Edgar, R., and Barrett, T. (2006). NCBI GEO standards and services for microarray data. Nat. Biotechnol. 24, 1471–1472. doi:10.1038/nbt1206-1471
FDA (2013). Orphan drug designations and approvals. [Online]. Available: https://www.accessdata.fda.gov/scripts/opdlisting/oopd/detailedIndex.cfm?cfgridkey=441314.
Fuqua, S. (2019). Comprehensive genomic analysis identify novel subtypes and targets of triple-negative breast cancer. [Online]. Available: https://www.ncbi.nlm.nih.gov/geo/query/acc.cgi?acc=GSE76275.
Hasan, M. M., Yang, S., Zhou, Y., and Mollah, M. N. (2016). SuccinSite: A computational tool for the prediction of protein succinylation sites by exploiting the amino acid patterns and properties. Mol. Biosyst. 12, 786–795. doi:10.1039/c5mb00853k
Holstein, E., Dittmann, A., Kaariainen, A., Pesola, V., Koivunen, J., Pihlajaniemi, T., et al. (2021). The burden of post-translational modification (PTM)-Disrupting mutations in the tumor matrisome. Cancers (Basel) 13, 1081. doi:10.3390/cancers13051081
Hopkins, A. L. (2007). Network pharmacology. Nat. Biotechnol. 25, 1110–1111. doi:10.1038/nbt1007-1110
Huang, P., Zhou, P., Liang, Y., Wu, J., Wu, G., Xu, R., et al. (2021). Exploring the molecular targets and mechanisms of [10]-Gingerol for treating triple-negative breast cancer using bioinformatics approaches, molecular docking, and in vivo experiments. Transl. Cancer Res. 10, 4680–4693. doi:10.21037/tcr-21-1138
Huo, Q., Chen, S., Li, Z., Wang, J., Li, J., and Xie, N. (2021). Inhibiting of TACC3 promotes cell proliferation, cell invasion and the EMT pathway in breast cancer. Front. Genet. 12, 640078. doi:10.3389/fgene.2021.640078
Iqbal, N., and Iqbal, N. (2014). Imatinib: A breakthrough of targeted therapy in cancer. Chemother. Res. Pract. 2014, 357027. doi:10.1155/2014/357027
Irizarry, R. A., Hobbs, B., Collin, F., Beazer-Barclay, Y. D., Antonellis, K. J., Scherf, U., et al. (2003). Exploration, normalization, and summaries of high density oligonucleotide array probe level data. Biostatistics 4, 249–264. doi:10.1093/biostatistics/4.2.249
Jiang, Y. Z., Liu, Y., Xiao, Y., Hu, X., Jiang, L., Zuo, W. J., et al. (2021). Molecular subtyping and genomic profiling expand precision medicine in refractory metastatic triple-negative breast cancer: The FUTURE trial. Cell Res. 31, 178–186. doi:10.1038/s41422-020-0375-9
Kim, S., Chen, J., Cheng, T., Gindulyte, A., He, J., He, S., et al. (2019). PubChem 2019 update: Improved access to chemical data. Nucleic Acids Res. 47, D1102–D1109. doi:10.1093/nar/gky1033
Komatsu, M., and Katagiri, T. (2018). Gene expression profiling of triple negative breast cancer, normal ductal cells, and normal tissues. [Online]. Available: https://www.ncbi.nlm.nih.gov/geo/query/acc.cgi?acc=GSE38959.
Kumar, P., and Aggarwal, R. (2016). An overview of triple-negative breast cancer. Arch. Gynecol. Obstet. 293, 247–269. doi:10.1007/s00404-015-3859-y
Langedijk, J., Mantel-Teeuwisse, A. K., Slijkerman, D. S., and Schutjens, M. H. (2015). Drug repositioning and repurposing: Terminology and definitions in literature. Drug Discov. Today 20, 1027–1034. doi:10.1016/j.drudis.2015.05.001
Li, L., Huang, H., Zhu, M., and Wu, J. (2021). Identification of hub genes and pathways of triple negative breast cancer by expression profiles analysis. Cancer Manag. Res. 13, 2095–2104. doi:10.2147/CMAR.S295951
Liao, Y., Liao, Y., Li, J., Li, J., Fan, Y., and Xu, B. (2018). Polymorphisms in AURKA and AURKB are associated with the survival of triple-negative breast cancer patients treated with taxane-based adjuvant chemotherapy. Cancer Manag. Res. 10, 3801–3808. doi:10.2147/CMAR.S174735
Liu, L., Li, X. R., Hu, Y. H., and Zhang, J. (2016). [Relevance between TOP2A, EGFR gene expression and efficacy of docetaxel plus epirubicin as neoadjuvant chemotherapy in triple negative breast cancer patients]. Zhonghua Yi Xue Za Zhi 96, 940–943. doi:10.3760/cma.j.issn.0376-2491.2016.12.007
Liu, P., Tang, H., Song, C., Wang, J., Chen, B., Huang, X., et al. (2018). SOX2 promotes cell proliferation and metastasis in triple negative breast cancer. Front. Pharmacol. 9, 942. doi:10.3389/fphar.2018.00942
Ma, J., Chen, C., Liu, S., Ji, J., Wu, D., Huang, P., et al. (2022). Identification of a five genes prognosis signature for triple-negative breast cancer using multi-omics methods and bioinformatics analysis. Cancer Gene Ther. 29, 1578–1589. doi:10.1038/s41417-022-00473-2
Mathe, A., Avery-Kiejda, K., Wong-Brown, M., Morten, B., Forbes, J., Braye, S., et al. (2018). Novel transcripts associated with lymph node metastasis in triple negative breast cancer [validation cohort]. [Online]. Available: https://www.ncbi.nlm.nih.gov/geo/query/acc.cgi?acc=GSE61724.
Mehta, M., Griffith, J., Panneerselvam, J., Babu, A., Mani, J., Herman, T., et al. (2021). Regorafenib sensitizes human breast cancer cells to radiation by inhibiting multiple kinases and inducing DNA damage. Int. J. Radiat. Biol. 97, 1109–1120. doi:10.1080/09553002.2020.1730012
Mu, R., Ma, Z., Lu, C., Wang, H., Cheng, X., Tuo, B., et al. (2021). Role of succinylation modification in thyroid cancer and breast cancer. Am. J. Cancer Res. 11, 4683–4699.
Nakai, K., Hung, M. C., and Yamaguchi, H. (2016). A perspective on anti-EGFR therapies targeting triple-negative breast cancer. Am. J. Cancer Res. 6, 1609–1623.
Nieto, M., Borregaard, J., Ersboll, J., ten Bosch, G. J., van Zwieten-Boot, B., Abadie, E., et al. (2011). The European medicines agency review of pazopanib for the treatment of advanced renal cell carcinoma: Summary of the scientific assessment of the committee for medicinal products for human use. Clin. Cancer Res. 17, 6608–6614. doi:10.1158/1078-0432.CCR-11-1734
Pettersen, E. F., Goddard, T. D., Huang, C. C., Couch, G. S., Greenblatt, D. M., Meng, E. C., et al. (2004). UCSF Chimera-a visualization system for exploratory research and analysis. J. Comput. Chem. 25, 1605–1612. doi:10.1002/jcc.20084
Qiu, P., Guo, Q., Yao, Q., Chen, J., and Lin, J. (2021). Hsa-mir-3163 and CCNB1 may be potential biomarkers and therapeutic targets for androgen receptor positive triple-negative breast cancer. PLoS One 16, e0254283. doi:10.1371/journal.pone.0254283
Regorafenib (2017). Regorafenib approved for liver cancer. Cancer Discov. 7, 660. doi:10.1158/2159-8290.CD-NB2017-070
Ritchie, M. E., Phipson, B., Wu, D., Hu, Y., Law, C. W., Shi, W., et al. (2015). Limma powers differential expression analyses for RNA-sequencing and microarray studies. Nucleic Acids Res. 43, e47. doi:10.1093/nar/gkv007
Rudrapal, M., Khairnar, S. J., and Jadhav, A. G. (2020). “Drug repurposing (DR): An emerging approach in drug discovery,” in Drug repurposing - hypothesis, molecular aspects and therapeutic applications. Editor F. A. Badria (Norderstedt, Germany: Books on Demand).
Ryall, K. A., Kim, J., Klauck, P. J., Shin, J., Yoo, M., Ionkina, A., et al. (2015). An integrated bioinformatics analysis to dissect kinase dependency in triple negative breast cancer. BMC Genomics 16 (12), S2. doi:10.1186/1471-2164-16-S12-S2
Shadbad, M. A., Safaei, S., Brunetti, O., Derakhshani, A., Lotfinejad, P., Mokhtarzadeh, A., et al. (2021). A systematic review on the therapeutic potentiality of PD-L1-inhibiting MicroRNAs for triple-negative breast cancer: Toward single-cell sequencing-guided biomimetic delivery. Genes (Basel) 12, 1206. doi:10.3390/genes12081206
Shannon, P., Markiel, A., Ozier, O., Baliga, N. S., Wang, J. T., Ramage, D., et al. (2003). Cytoscape: A software environment for integrated models of biomolecular interaction networks. Genome Res. 13, 2498–2504. doi:10.1101/gr.1239303
Society, A. C. (2022). Triple-negative breast cancer. [Online]. Available: https://www.cancer.org/cancer/breast-cancer/about/types-of-breast-cancer/triple-negative.html.
Song, X., Zhang, Y., Dai, E., Du, H., and Wang, L. (2019). Mechanism of action of celastrol against rheumatoid arthritis: A network pharmacology analysis. Int. Immunopharmacol. 74, 105725. doi:10.1016/j.intimp.2019.105725
Sung, H., Ferlay, J., Siegel, R. L., Laversanne, M., Soerjomataram, I., Jemal, A., et al. (2021). Global cancer statistics 2020: GLOBOCAN estimates of incidence and mortality worldwide for 36 cancers in 185 countries. Ca. Cancer J. Clin. 71, 209–249. doi:10.3322/caac.21660
Szklarczyk, D., Gable, A. L., Lyon, D., Junge, A., Wyder, S., Huerta-Cepas, J., et al. (2019). STRING v11: Protein-protein association networks with increased coverage, supporting functional discovery in genome-wide experimental datasets. Nucleic Acids Res. 47, D607–D613. doi:10.1093/nar/gky1131
Taylor, S. K., Chia, S., Dent, S., Clemons, M., Agulnik, M., Grenci, P., et al. (2010). A phase II study of pazopanib in patients with recurrent or metastatic invasive breast carcinoma: A trial of the princess margaret hospital phase II consortium. Oncologist 15, 810–818. doi:10.1634/theoncologist.2010-0081
Trott, O., and Olson, A. J. (2010). AutoDock vina: Improving the speed and accuracy of docking with a new scoring function, efficient optimization, and multithreading. J. Comput. Chem. 31, 455–461. doi:10.1002/jcc.21334
Van Swearingen, A. E. D., Sambade, M. J., Siegel, M. B., Sud, S., McNeill, R. S., Bevill, S. M., et al. (2017). Combined kinase inhibitors of MEK1/2 and either PI3K or PDGFR are efficacious in intracranial triple-negative breast cancer. Neuro. Oncol. 19, 1481–1493. doi:10.1093/neuonc/nox052
Volovat, S. R., Volovat, C., Hordila, I., Hordila, D. A., Mirestean, C. C., Miron, O. T., et al. (2020). MiRNA and LncRNA as potential biomarkers in triple-negative breast cancer: A review. Front. Oncol. 10, 526850. doi:10.3389/fonc.2020.526850
Wagner, A. H., Coffman, A. C., Ainscough, B. J., Spies, N. C., Skidmore, Z. L., Campbell, K. M., et al. (2016). DGIdb 2.0: Mining clinically relevant drug-gene interactions. Nucleic Acids Res. 44, D1036–D1044. doi:10.1093/nar/gkv1165
Wang, C., Zheng, X., Shen, C., and Shi, Y. (2012). MicroRNA-203 suppresses cell proliferation and migration by targeting BIRC5 and LASP1 in human triple-negative breast cancer cells. J. Exp. Clin. Cancer Res. 31, 58. doi:10.1186/1756-9966-31-58
Wang, D., Liu, D., Yuchi, J., He, F., Jiang, Y., Cai, S., et al. (2020). MusiteDeep: A deep-learning based webserver for protein post-translational modification site prediction and visualization. Nucleic Acids Res. 48, W140–W146. doi:10.1093/nar/gkaa275
Wei, L. M., Li, X. Y., Wang, Z. M., Wang, Y. K., Yao, G., Fan, J. H., et al. (2021). Identification of hub genes in triple-negative breast cancer by integrated bioinformatics analysis. Gland. Surg. 10, 799–806. doi:10.21037/gs-21-17
Yanagawa, M., Ikemot, K., Kawauchi, S., Furuya, T., Yamamoto, S., Oka, M., et al. (2012). Luminal A and luminal B (HER2 negative) subtypes of breast cancer consist of a mixture of tumors with different genotype. BMC Res. Notes 5, 376. doi:10.1186/1756-0500-5-376
Yang, L., Wu, X., and Yen, Y. (2018). FZD7 plays a critical role in triple negative breast cancer proliferation. [Online]. Available:[Accessed] https://www.ncbi.nlm.nih.gov/geo/query/acc.cgi?acc=GSE27447.
Yuan, Q., Zheng, L., Liao, Y., and Wu, G. (2021). Overexpression of CCNE1 confers a poorer prognosis in triple-negative breast cancer identified by bioinformatic analysis. World J. Surg. Oncol. 19, 86. doi:10.1186/s12957-021-02200-x
Zhang, J., Liu, D., Deng, G., Wang, Q., Li, L., Zhang, J., et al. (2021a). lncRNA prostate cancer-associated transcript 18 upregulates activating transcription factor 7 to prevent metastasis of triple-negative breast cancer via sponging miR-103a-3p. Bioengineered 12, 12070–12086. doi:10.1080/21655979.2021.2003928
Zhang, J., Zhang, X., Li, Z., Wang, Q., Shi, Y., Jiang, X., et al. (2021b). The miR-124-3p/neuropilin-1 Axis contributes to the proliferation and metastasis of triple-negative breast cancer cells and Co-activates the TGF-beta pathway. Front. Oncol. 11, 654672. doi:10.3389/fonc.2021.654672
Zhou, G., Soufan, O., Ewald, J., Hancock, R. E. W., Basu, N., and Xia, J. (2019). NetworkAnalyst 3.0: A visual analytics platform for comprehensive gene expression profiling and meta-analysis. Nucleic Acids Res. 47, W234–W241. doi:10.1093/nar/gkz240
Keywords: triple-negative breast cancer, microarray gene expression profiles, key genes (KGs), diagnosis, therapies, integrated bioinformatics and system biology approaches
Citation: Alam MS, Sultana A, Wang G and Haque Mollah MN (2022) Gene expression profile analysis to discover molecular signatures for early diagnosis and therapies of triple-negative breast cancer. Front. Mol. Biosci. 9:1049741. doi: 10.3389/fmolb.2022.1049741
Received: 21 September 2022; Accepted: 21 November 2022;
Published: 07 December 2022.
Edited by:
Jiandong Zhou, University of Oxford, United KingdomReviewed by:
Chaowang Lan, Guilin University of Electronic Technology, ChinaSushil Kumar, Indian Institute of Technology Bombay, India
Copyright © 2022 Alam, Sultana, Wang and Haque Mollah. This is an open-access article distributed under the terms of the Creative Commons Attribution License (CC BY). The use, distribution or reproduction in other forums is permitted, provided the original author(s) and the copyright owner(s) are credited and that the original publication in this journal is cited, in accordance with accepted academic practice. No use, distribution or reproduction is permitted which does not comply with these terms.
*Correspondence: Guanghui Wang, d2FuZ2doQHN1ZGEuZWR1LmNu; Md Nurul Haque Mollah, bW9sbGFoLnN0YXQuYmlvQHJ1LmFjLmJk