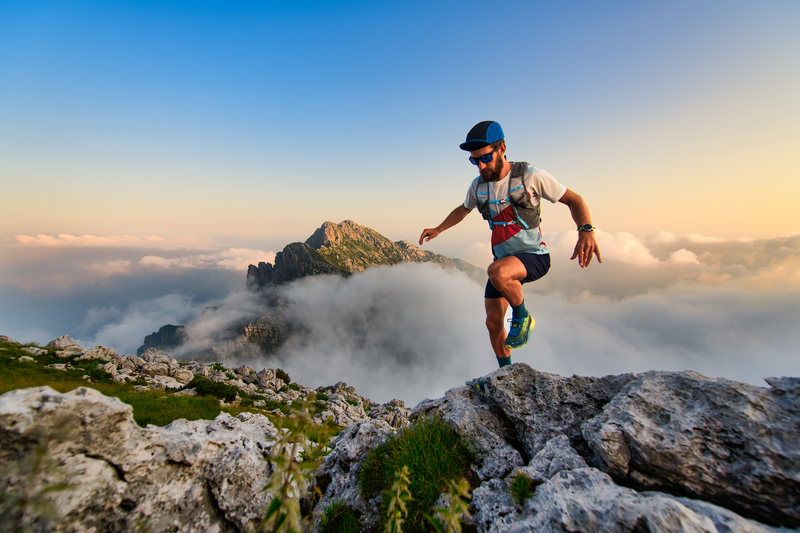
94% of researchers rate our articles as excellent or good
Learn more about the work of our research integrity team to safeguard the quality of each article we publish.
Find out more
MINI REVIEW article
Front. Mol. Biosci. , 11 January 2022
Sec. Metabolomics
Volume 8 - 2021 | https://doi.org/10.3389/fmolb.2021.815410
This article is part of the Research Topic OMICS-Based Approaches in Sports Research View all 9 articles
Together with environment and experience (that is to say, diet and training), the biological and genetic make-up of an athlete plays a major role in exercise physiology. Sports genomics has shown, indeed, that some DNA single nucleotide polymorphisms (SNPs) can be associated with athlete performance and level (such as elite/world-class athletic status), having an impact on physical activity behavior, endurance, strength, power, speed, flexibility, energetic expenditure, neuromuscular coordination, metabolic and cardio-respiratory fitness, among others, as well as with psychological traits. Athletic phenotype is complex and depends on the combination of different traits and characteristics: as such, it requires a “complex science,” like that of metadata and multi-OMICS profiles. Several projects and trials (like ELITE, GAMES, Gene SMART, GENESIS, and POWERGENE) are aimed at discovering genomics-based biomarkers with an adequate predictive power. Sports genomics could enable to optimize and maximize physical performance, as well as it could predict the risk of sports-related injuries. Exercise has a profound impact on proteome too. Proteomics can assess both from a qualitative and quantitative point of view the modifications induced by training. Recently, scholars have assessed the epigenetics changes in athletes. Summarizing, the different omics specialties seem to converge in a unique approach, termed sportomics or athlomics and defined as a “holistic and top-down,” “non-hypothesis-driven research on an individual’s metabolite changes during sports and exercise” (the Athlome Project Consortium and the Santorini Declaration) Not only sportomics includes metabonomics/metabolomics, but relying on the athlete’s biological passport or profile, it would enable the systematic study of sports-induced changes and effects at any level (genome, transcriptome, proteome, etc.). However, the wealth of data is so huge and massive and heterogenous that new computational algorithms and protocols are needed, more computational power is required as well as new strategies for properly and effectively combining and integrating data.
Sport, exercise, and physical activity represent complex, multi-factorial, non-linear activities at the intersection of biology, physiology, and psychology, resulting from the crosstalk of the biological make-up of the individual (genes, proteins, and other molecules), physiological and environmental variables, with psychological factors (such as motivation) also mattering. In the present review, we will introduce readers with state-of-arts applications of OMICS in the field of sports sciences.
Together with environment, experience, and behaviors/lifestyles (that is to say, diet and training), the biological and genetic make-up of an athlete plays a major role in exercise physiology (Bouchard et al., 2011; Guest et al., 2019; Nieman and Wentz, 2019). A genomics super-specialty called “sports genomics” has shown, indeed, that some DNA single nucleotide polymorphisms (SNPs) can be associated with athletes’ status, performance, and level (such as amateur versus elite/world-class athletic status), having an impact on a wide range of physical activity related behaviors and outcomes, including endurance, strength, power, sprint and speed, flexibility, energetic expenditure, neuromuscular coordination, metabolic and cardio-respiratory fitness, among others, as well as with mental characteristics and psychological traits (Ahmetov et al., 2016).
The so-called “athletic phenotype” is complex and depends on the combination of different traits and characteristics: as such, it requires a “complex science,” like that of metadata and multi-OMICS profiles. Several projects and trials (like ELITE, GAMES, Gene SMART, GENESIS, and POWERGENE) are aimed at discovering genomics- and post-genomics-based biomarkers with an adequate predictive power, statistical robustness, and interpretability of discoveries in terms of replicable, physiologically meaningful, and relevant patterns (Pitsiladis et al., 2016).
Sports genomics could enable to identify talents, optimize, and maximize physical performance outcomes, as well as it could predict the risk of sports-related injuries and the timing of return to sport (Tanisawa et al., 2020).
Exercise has a profound impact on proteome too (Sellami and Bragazzi, 2021). Proteomics can assess both from a qualitative and quantitative point of view the modifications induced by training. Recently, scholars have also assessed the epigenetics changes in athletes, establishing a new super-specialty termed as “sports epigenetics/epigenomics” (Zimmer et al., 2016). Other biomarkers have been identified at the level of the metabolome (Khoramipour et al., 2021), immunome (Nieman and Pence, 2020) and microbiome (Mohr et al., 2020), among others.
Summarizing, the different OMICS specialties seem to converge in a unique approach, termed sportomics or athlomics and defined as a “holistic and top-down,” “non-hypothesis-driven research on an individual’s metabolite changes during sports and exercise” (as stated in the Athlome Project Consortium and in the Santorini Declaration) (Bragazzi et al., 2020). Not only sportomics includes metabonomics/metabolomics, but relying on the athlete’s biological passport or profile, it would enable the systematic study of sports-induced changes and effects at any level (genome, transcriptome, proteome, metabolome, etc.) (Pintus et al., 2021).
These aspects will be overviewed and synthesized in the next sub-sections and paragraphs.
A major application of sports genetics/genomics is the dissection of the molecular basis underlying performance outcomes. As previously mentioned, various SNPs have been found to be associated with athletes’ characteristics. For example, vitamin D receptor (VDR) polymorphisms seem to be related to physical strength, bradykinin receptor B2 (BDKRB2) seems associated to strength and efficiency in muscle contraction, DRD2 to physical behavior and motivation, alpha-actinin-3 (ACTN3) to muscle contraction, speed and power, alpha-2B adrenergic receptor (ADRA2B) to metabolism, ATPase Na+/K+ transporting subunit alpha 2 (ATP1A2) with aerobic capacity, peroxisome proliferator activated receptor alpha (PPAR-alpha) with cardiorespiratory fitness and endurance, peroxisome proliferator-activated receptor delta (PPAR-delta) with aerobic capacity, and angiotensin-converting enzyme (ACE) with endurance, among others (Ahmetov and Fedotovskaya, 2015).
Ahmetov et al. (Ahmetov and Fedotovskaya, 2015) have reviewed the genetic/genomic influence on sports success achievement and performance outcomes, synthesizing and appraising currently available knowledge related to 155 genetic biomarkers (involving virtually all chromosomes and even mitochondrial DNA). In general, these markers were associated to elite athlete status, with 93 of them predicting endurance, and 62 being linked to power/strength. Forty-one biomarkers had been recently discovered, conducting genome-wide association studies (GWAS) of non-White Caucasian athletes—Afro-American, individuals from Jamaica, Russia and Japan, emphasizing the importance of investigating different ethnicities. In terms of replicability, at least two and three studies had replicated the findings related to 31 and 12 biomarkers, respectively, with 29 markers not being replicated by at least one study. This may suggest that these markers are false-positive, or that they would contribute to athlete’s status and related phenotype in some but not other ethnicities. The possibility of the influence of other factors and variables not taken into account should also not be ruled out, warranting further high-quality, multi-center research, involving large cohorts of athletes and underlining the importance of large-scale databases and Big Data.
Besides enabling discovery of the genetics underlying physical performance outcomes, sports genetics/genomics could as well predict the risk of sports-related injuries. Genes such as matrix metallopeptidase (MMP) type 3 (MMP-3), bone morphogenetic protein type 4 (BMP-4), fibroblast growth factor type 3 (FGF-3), the receptor Activator of Nuclear Factor κ B (RANK)/receptor Activator of Nuclear Factor κ B ligand (RANKL)/osteoprotegerin (OPG) signaling pathway seem to predict injury risk (Ahmetov and Fedotovskaya, 2015). Apolipoprotein E, interleukins (ILs, like IL-6 and IL-12), toll-like receptors (TLRs, such as TLR-4), nuclear factor (NF-κB), follicle stimulating hormone, chorionic gonadotropin, protein kinase catalytic subunit and solute carrier family 17 member 7 (SLC-17A7), as well as other genes encoding factors/proteins implied in the hypothalamic-pituitary-adrenal (HPA) axis seem to be associated with the prognosis after an injury (Ahmetov and Fedotovskaya, 2015).
For instance, we could consider the example of Achilles tendinopathy, which is one of the commonest sports-related injuries (Kakouris et al., 2021), with a yearly incidence of 7–9% and affecting approximately one third of runners (Ackermann and Renström, 2012). Rahim and others (Rahim et al., 2016) sampled 108 and 87 subjects with Achilles tendinopathy from South Africa and the United Kingdom, respectively. Individuals were genotyped for 5 SNPs at the level of the genes encoding the vascular endothelial growth factor (VEGF) A isoform (VEGFA) and the kinase insert-domain receptor (KDR). Polymorphisms affecting the angiogenesis signaling cascade may be involved in the etiopathogenesis of Achilles tendinopathy. Another common sports injury is given by the rotator cuff pathology (Blevins, 1997). Recent genomics studies have identified some SNPs (Cohen et al., 2020; Tashjian et al., 2021) involving the gene encoding Zinc Finger Protein 804A (ZNF804A), the glucocorticoid induced 1 gene (GLCCI1), and the gene encoding the thrombospondin type-1 domain-containing protein 7A (THSD7A) as molecular factors responsible for the development of shoulder pathologies. Some genes, such as THSD7A, were found to be downregulated, whereas other genes such as those coding the tissue inhibitor of MMP type 2 (TIMP2), Collagen Type V Alpha 1 Chain (Col5A1), Transforming Growth Factor Beta Receptor 1 (TGFBR1), and tenascin C (TNC), were detected to be upregulated.
Genetics/genomics studies have led to the design of polygenic/genotype-based scores for profiling athletes (Sillanpää et al., 2021), in terms of power, sprint, speed, and/or endurance (Ruiz et al., 2009; Ben-Zaken et al., 2015) and predict/forecast the effects of training programs and strategies (Lee et al., 2021).
Summarizing, sports genomics-related studies have covered three major and broad research areas in the field of sports and exercise sciences: namely, i) determinants of performance outcomes, ii) injury susceptibility and prevention, iii) injuries and diseases management (Griswold et al., 2021; McAuley et al., 2021).
MicroRNAs (miRNAs) are small single-stranded non-coding RNA molecules (generally consisting of approximately 22 nucleotides) that are involved in several cellular processes, including RNA silencing and post-transcriptional modulation of gene expression. Physical activity and sports exercise are known to tune and regulate genes as well as miRNAs, even though the expression of human miRNAome as response and adaptation to exercise/training remains to be elucidated. A recent study (Hecksteden et al., 2016) found that, in a sample of endurance and strength athletes, some miRNAs were differentially expressed in blood and plasma before and after exercise, in particular, miR-140-3p, which is involved in the pathogenesis of several malignancies (Kapodistrias et al., 2017). Other miRNAs were miR-140-5p and miR-650, the function of which is related to heart physiology (Hecksteden et al., 2016), and other molecules involved in the tuning of signaling cascades such as the insulin-like growth factor 1 (IGF-1)/phosphoinositide 3-kinase/phosphatidylinositol 3-kinase (PI3K)/protein kinase B (AKT)/mammalian (or mechanistic) target of rapamycin (mTOR) pathway (Domańska-Senderowska et al., 2019). These miRNAs have been found to correlate with some physiological parameters, such as VO2 max, response to training load, and modulation of skeletal muscles (Domańska-Senderowska et al., 2019). These miRNAs are muscle-specific microRNAs and are crucial in skeletal muscle development: as such, they are called myomiRNAs (Horak et al., 2016) and can be found encapsulated in muscle-derived exosomes (Mytidou et al., 2021), which are fundamental for local tissue communication and interactions (McCarthy, 2011). A recent exploration found out that also urine myomiRNAs containing exosomes can be useful for sports performance monitoring (Kuji et al., 2021).
Transcriptomic profiling studies have identified unique signatures as adaption/response to exercise, consisting of various transcription factors (most of which are belonging to the activating transcription factor (ATF)/cyclic AMP-responsive element-binding (CREB)/activator protein type 1 (AP-1) superfamily) and co-activators (Fisher et al., 2017; Pillon et al., 2020; Makhnovskii et al., 2021).
Long non-coding RNAs (lncRNAs) (Sun et al., 2017; Yao et al., 2019) are emerging as novel biomarkers of response and adaption to exercise and training (De Sanctis et al., 2021). lncRNAs are molecules that do not have any protein-coding potential but are considered to be major key players in gene expression networks by finely tuning nuclear architecture as well as transcription in the nucleus and controlling and preserving mRNA stability. lncRNAs are involved as well in other cellular and biological processes, including translation and post-translational modifications at the level of the cytoplasm (Sun et al., 2017; Yao et al., 2019).
Summarizing, complex networks consisting of interactions between a wide range of molecules—including mRNAs, miRNAs, and non-coding RNAs such as lncRNAs—are being dissected as important molecular mechanisms underlying physiological performance outcomes (De Sanctis et al., 2021), even though this research field is still young and warrants further exploration.
These networks can represent potential druggable targets for cardiovascular diseases (Correia et al., 2021), obesity and other metabolic impairments (Banitalebi et al., 2021), which impose a dramatically high, economic, and societal burden (Dai et al., 2020a; Dai et al., 2020b; Dai et al., 2020c; Dai et al., 2021a; Dai et al., 2021b; Bragazzi et al., 2021).
Epigenetics represents a bridge between the individual genetic make-up of the individual and the external surrounding environment. Epigenetics biomarkers have been recently studied as predictors/determinants of the response/adaptation to exercise and training (Seaborne and Sharples, 2020). Jacques and others (Jacques et al., 2019) performed a systematic literature review aimed to identify changes at the level of the epigenome detected in skeletal muscle after exercise training among healthy populations and found twenty-two studies, exploring a wide range of epigenetics markers, including DNA methylation and histone modifications.
Tarnowski et al. (Tarnowski et al., 2021) have reported epigenetics changes at the level of inflammatory response after exercise, while Denham and others (Denham et al., 2016) conducted a clinical trial and assessed the epigenetics modifications in a sample of eight young (aged 21.1 ± 2.2 years) men after 8 weeks of a program of resistance exercise training. Authors reported changes in methylation patterns of genes related to cellular and biological processes such as axon guidance, diabetes and metabolic impairments, and immunity as well as CpG islands, in more detail, growth factor genes—growth-hormone releasing hormone (GHRH) and fibroblast growth factor type 1 (FGF-1). These modifications were studied at the level of leukocytes (Sellami et al., 2021a). Kashimoto and coworkers (Kashimoto et al., 2016) in a mouse model found that physical activity exerts a significant impact on DNA methylation patterns at the level of brain structures, like hypothalamus, hippocampus, and cortex, emphasizing the role of exercise and physical activity as epigenetics regulators of brain plasticity and cognitive processes (Fernandes et al., 2017) and interventional measures counteracting ageing and neurodegeneration (Xu et al., 2021).
Concerning unhealthy populations, Nguyen and others (Nguyen et al., 2016) found epigenetic changes at the level of glutathione peroxidase 1 gene in a severely dyslipidemic mouse model, suggesting potential pharmacological pathways and druggable targets.
Interestingly, Grzywacz and others (Grzywacz et al., 2021) explored the correlation between DNA methylation and personality characteristics in a sample of 100 European sports male individuals aged 22.88 ± 6.35 years, age- and gender-matched with 239 healthy controls. Authors found associations between methylation patterns of the promoter sequence of the dopamine transporter gene (DAT-1) and some personality traits/features.
However, the causality and the replicability of such markers are still unknown, warranting further research in the field (Akberdin et al., 2021).
Exercise has a profound impact on proteome, finely tuning energy metabolism cascades, adenosine triphosphate (ATP pathways), and mitochondrial proteins synthesis, among others (Balfoussia et al., 2014; Hargreaves and Spriet, 2020). For instance, plasma proteome has been shown to correlate to the extreme level of physical stress experienced by athletes taking part into the Spartathlon race who exhibited differentially expressed proteins belonging to several cascades and networks, including inflammation, anti-oxidation, anti-coagulation and iron and vitamin D transport pathways (Balfoussia et al., 2014). Running as well as other exercises and physical activities may induce changes in the acetylome (Philp et al., 2014). Other biomarkers of cellular stress are given by biological processes and events such as protein sumoylation: conducting intense resistance exercises can result into an acute, transient nuclear translocation of Small Ubiquitin-Related Modifier (SUMO)-1 at the level of Human Myofibres types I and II (Gehlert et al., 2016). Proteomics can assess both from a qualitative and quantitative point of view the modifications induced by long-term moderate training, as well as the effects of acute swimming exercise and other sports disciplines (Ubaida-Mohien et al., 2019; Gomes et al., 2020). Magherini and collaborators (Magherini et al., 2013) explored protein expression and patterns and markers of oxidative stress in the Extensor Digitorum Longus and the Soleus. Authors found that the protein carbonylation and lipid peroxidation levels were reduced following acute swimming bursts. Also one-half minute of maximal exercise can induce changes in human urinary proteome, some of which are still visible after 2 hours, whilst prolonged strenuous exercise can cause proteinuria (haemoglobinuria or myoglobinuria), which reflects in larger proteomics alterations and biochemical/biophysical anomalies (Bellinghieri et al., 2008; Kohler et al., 2015).
Concerning sports injuries, Sejersen and others (Sejersen et al., 2015) reviewed the proteomics of rotator cuff pathologies and tendinopathies and found an increase of collagen I and III, several MMPs, including MMP-1, MMP-9, MMP-13, tissue inhibitor of MMP type 1 (TIMP-1), and VEGF, and a decrease in MMP-3. Daisy et al. (Daisy et al., 2021) recruited 47 concussion athletes from both contact and non-contact sports disciplines, who were age- and sex-matched with 48 controls. Authors identified IGF-1 and IGF binding protein 5 (IGFBP5) as urine proteomics biomarkers, highly correlating with single-task gait velocity and predictive of concussions at acute time-points.
Finally, Khoramipour and others (Khoramipour et al., 2021) were able to identify five major categories covered by sports and exercise metabolomics/metabonomics studies: namely, i) exercise metabolism; ii) exercise metabolism with focus on nutritional aspects; iii) sports metabolism; iv) exercise metabolism with an emphasis on clinical implications; and v) metabolome assessments and comparisons. Authors found that exercise metabolism was the research area most addressed by scholarly investigations, generally utilizing blood and urine samples but relying on untargeted, qualitative rather than targeted, quantitative platforms. Despite attracting increasing interest from the scientific community which reflects into a higher publishing trend, sports metabolomics/metabonomics still represents more an art than a science, warranting more high-quality studies, with samples drawn from larger cohorts and exploiting a longitudinal, randomized design.
Sports nutrigenomics is a young super-specialty, relatively under-explored in the existing scholarly literature (Bragazzi, 2013). It can potentially help i) devise and deliver target nutritional advice, ii) stratify and identify athletes’ metabotypes (Hillesheim et al., 2020). Few studies have attempted exploring this topic. Among the early pioneering contributions, we have to mention the study by Ribeiro et al. (Ribeiro et al., 2013), who explored the effects of erythropoietin (EPO T→G) and α-actinin-3 (ACTN3 R577X) SNPs on the response to the dietary ingestion of antioxidant supplementation based on pequi oil (Caryocar brasiliense Camb.) in a sample of runners. CYP1A2 genotype was found to modify the effects of caffeine uptake versus placebo on muscle strength in a sample of competitive male athletes (Wong et al., 2021).
The term “immunome” has been coined to indicate the incredibly diverse and vast individual’s repertoire of antibodies, B cell receptors and T cell receptors (TRs), that can be generally assessed from the blood, and consists of approximately 50,000–440,000 B lymphocytes and 600,000–3,500,000 T lymphocytes per ml in apparently healthy adults (Arnaout et al., 2021). Exercise can be conceived as a “molecular choreography,” that is to say, as a concerted series of biological, and cellular processes at the level of the cardiovascular, endocrine, and immunologic systems (Contrepois et al., 2020). Physical activity and sport are able to modulate the expression, production and release of cytokines, known as exerkines (Eaton et al., 2018; Sellami et al., 2021b; Magliulo et al., 2021).
Finally, sports microbiomics is a very recent and young super-specialty stemming from post-genomics specializations (Hughes, 2020; Marttinen et al., 2020). Athletes’ gut microbiome has been found to modulate the effects of nutrients uptake and dietary components and/or supplements, like probiotics, impacting as well on performance outcomes.
As previously mentioned, athletic phenotype is extremely complex and depends on the combination of a wide range of traits and characteristics: from genetic/genomics to post-genomics features, affecting the endocrine, immunologic systems. As such, it requires a “complex science,” like that of metadata and multi-OMICS profiles. Several ambitious multi-center trials and projects (like the previously mentioned ELITE, GAMES, Gene SMART, GENESIS, and POWERGENE) are aimed at discovering genomics-based biomarkers with an adequate predictive power.
Sportomics strategies have been so far applied to the investigation of effects of arginine, keto analogues, and other amino acids supplementation, or the determinants of performance outcomes in world-class canoeists or elite athletes (Gonçalves et al., 2012; Camerino et al., 2016; Coelho et al., 2016; Marttinen et al., 2020; Monnerat et al., 2020; Clauss et al., 2021).
The unique combination and convergence of Big Data derived from molecular techniques (OMICS), and data science offers unprecedented solutions to old problems as well as new opportunities The usage of more than one source/channel of data enables to have more realistic insights, given the complexity underlying physical performance, its drivers/predictors and its outcomes. Molecular Big Data are promising in that they are anticipated to enable a better understanding of the determinants of physical performance, shedding light on how optimizing and enhancing performance, identifying talents, predicting (nowcasting/forecasting) physiological outcomes, predicting injuries and identifying strategies for injury prevention, among others. However, the wealth of data is so huge and massive and heterogenous that new computational algorithms and protocols are needed, more computational power is required as well as new strategies for properly and effectively combining and integrating data.
MS, ME, and NB conceived and designed the study. MS, ME, LP, and NB drafted and critically revised the manuscript.
The authors declare that the research was conducted in the absence of any commercial or financial relationships that could be construed as a potential conflict of interest.
All claims expressed in this article are solely those of the authors and do not necessarily represent those of their affiliated organizations, or those of the publisher, the editors and the reviewers. Any product that may be evaluated in this article, or claim that may be made by its manufacturer, is not guaranteed or endorsed by the publisher.
Ackermann, P. W., and Renström, P. (2012). Tendinopathy in Sport. Sports Health 4 (3), 193–201. doi:10.1177/1941738112440957
Ahmetov, I. I., Egorova, E. S., Gabdrakhmanova, L. J., and Fedotovskaya, O. N. (2016). Genes and Athletic Performance: An Update. Med. Sport Sci. 61, 41–54. doi:10.1159/000445240
Ahmetov, I. I., and Fedotovskaya, O. N. (2015). Current Progress in Sports Genomics. Adv. Clin. Chem. 70, 247–314. doi:10.1016/bs.acc.2015.03.003
Akberdin, I. R., Kiselev, I. N., Pintus, S. S., Sharipov, R. N., Vertyshev, A. Y., Vinogradova, O. L., et al. (2021). A Modular Mathematical Model of Exercise-Induced Changes in Metabolism, Signaling, and Gene Expression in Human Skeletal Muscle. Ijms 22 (19), 10353. doi:10.3390/ijms221910353
Arnaout, R. A., Prak, E. T. L., Schwab, N., and Rubelt, F. (2021). The Future of Blood Testing Is the Immunome. Front. Immunol. 12, 626793. doi:10.3389/fimmu.2021.626793
Balfoussia, E., Skenderi, K., Tsironi, M., Anagnostopoulos, A. K., Parthimos, N., Vougas, K., et al. (2014). A Proteomic Study of Plasma Protein Changes under Extreme Physical Stress. J. Proteomics 98, 1–14. doi:10.1016/j.jprot.2013.12.004
Banitalebi, E., Ghahfarrokhi, M. M., and Dehghan, M. (2021). Effect of 12-weeks Elastic Band Resistance Training on MyomiRs and Osteoporosis Markers in Elderly Women with Osteosarcopenic Obesity: a Randomized Controlled Trial. BMC Geriatr. 21 (1), 433. doi:10.1186/s12877-021-02374-9
Bellinghieri, G., Savica, V., and Santoro, D. (2008). Renal Alterations during Exercise. J. Ren. Nutr. 18 (1), 158–164. doi:10.1053/j.jrn.2007.10.031
Ben-Zaken, S., Meckel, Y., Nemet, D., and Eliakim, A. (2015). Genetic Score of Power-Speed and Endurance Track and Field Athletes. Scand. J. Med. Sci. Sports 25 (2), 166–174. doi:10.1111/sms.12141
Blevins, F. T. (1997). Rotator Cuff Pathology in Athletes. Sports Med. 24 (3), 205–220. doi:10.2165/00007256-199724030-00009
Bouchard, C., Rankinen, T., and Timmons, J. A. (2011). Genomics and Genetics in the Biology of Adaptation to Exercise. Compr. Physiol. 1 (3), 1603–1648. doi:10.1002/cphy.c100059
Bragazzi, N. L. (2013). Situating Nutri-Ethics at the Junction of Nutrigenomics and Nutriproteomics in Postgenomics Medicine. Cppm 11 (2), 162–166. doi:10.2174/1875692111311020008
Bragazzi, N. L., Khoramipour, K., Chaouachi, A., and Chamari, K. (2020). Toward Sportomics: Shifting from Sport Genomics to Sport Postgenomics and Metabolomics Specialties. Promises, Challenges, and Future Perspectives. Int. J. Sports Physiol. Perform. 15, 1201–1202. doi:10.1123/ijspp.2020-0648
Bragazzi, N. L., Zhong, W., Shu, J., Abu Much, A., Lotan, D., Grupper, A., et al. (2021). Burden of Heart Failure and Underlying Causes in 195 Countries and Territories from 1990 to 2017. Eur. J. Prev. Cardiol., zwaa147. doi:10.1093/eurjpc/zwaa147
Camerino, S. R. A. e. S., Lima, R. C. P., França, T. C. L., Herculano, E. d. A., Rodrigues, D. S. A., Gouveia, M. G. d. S., et al. (2016). Keto Analogue and Amino Acid Supplementation and its Effects on Ammonemia and Performance under Thermoneutral Conditions. Food Funct. 7 (2), 872–880. doi:10.1039/c5fo01054c
Clauss, M., Gérard, P., Mosca, A., and Leclerc, M. (2021). Interplay between Exercise and Gut Microbiome in the Context of Human Health and Performance. Front. Nutr. 8, 637010. doi:10.3389/fnut.2021.637010
Coelho, W., Viveiros de Castro, L., Deane, E., Magno-França, A., Bassini, A., and Cameron, L.-C. (2016). Investigating the Cellular and Metabolic Responses of World-Class Canoeists Training: A Sportomics Approach. Nutrients 8 (11), 719. doi:10.3390/nu8110719
Cohen, C., Figueiredo, E. A., Belangero, P. S., Andreoli, C. V., Leal, M. F., and Ejnisman, B. (2020). Aspectos Genéticos Nas Afecções Do Ombro. Rev. Bras Ortop (Sao Paulo) 55 (5), 537–542. doi:10.1055/s-0040-1702955
Contrepois, K., Wu, S., Moneghetti, K. J., Hornburg, D., Ahadi, S., Tsai, M.-S., et al. (2020). Molecular Choreography of Acute Exercise. Cell 181 (5), 1112–1130. e16. doi:10.1016/j.cell.2020.04.043
Correia, C. C. M., Rodrigues, L. F., de Avila Pelozin, B. R., Oliveira, E. M., and Fernandes, T. (2021). Long Non-coding RNAs in Cardiovascular Diseases: Potential Function as Biomarkers and Therapeutic Targets of Exercise Training. ncRNA 7 (4), 65. doi:10.3390/ncrna7040065
Dai, H., Alsalhe, T. A., Chalghaf, N., Riccò, M., Bragazzi, N. L., and Wu, J. (2020). The Global burden of Disease Attributable to High Body Mass index in 195 Countries and Territories, 1990-2017: An Analysis of the Global Burden of Disease Study. Plos Med. 17 (7), e1003198. doi:10.1371/journal.pmed.1003198
Dai, H., Bragazzi, N. L., Younis, A., Zhong, W., Liu, X., Wu, J., et al. (2021). Worldwide Trends in Prevalence, Mortality, and Disability-Adjusted Life Years for Hypertensive Heart Disease from 1990 to 2017. Hypertension 77 (4), 1223–1233. doi:10.1161/HYPERTENSIONAHA.120.16483
Dai, H., Lotan, D., Much, A. A., Younis, A., Lu, Y., Bragazzi, N. L., et al. (2021). Global, Regional, and National Burden of Myocarditis and Cardiomyopathy, 1990-2017. Front. Cardiovasc. Med. 8, 610989. doi:10.3389/fcvm.2021.610989
Dai, H., Much, A. A., Maor, E., Asher, E., Younis, A., Xu, Y., et al. (2020). Global, Regional, and National burden of Ischaemic Heart Disease and its Attributable Risk Factors, 1990-2017: Results from the Global Burden of Disease Study 2017. Eur. Heart J. Qual. Care Clin. Outcomes, qcaa076. doi:10.1093/ehjqcco/qcaa076
Dai, H., Zhang, Q., Much, A. A., Maor, E., Segev, A., Beinart, R., et al. (2020). Global, Regional, and National Prevalence, Incidence, Mortality, and Risk Factors for Atrial Fibrillation, 1990-2017: Results from the Global Burden of Disease Study 2017. Eur. Heart J. Qual. Care Clin. Outcomes 7, 574–582. doi:10.1093/ehjqcco/qcaa061
Daisy, C. C., Varinos, S., Howell, D. R., Kaplan, K., Mannix, R., Meehan, W. P., et al. (2021). Proteomic Discovery of Noninvasive Biomarkers Associated with Sport-Related Concussions. Neurology. doi:10.1212/WNL.0000000000013001
De Sanctis, P., Filardo, G., Abruzzo, P. M., Astolfi, A., Bolotta, A., Indio, V., et al. (2021). Non-Coding RNAs in the Transcriptional Network that Differentiates Skeletal Muscles of Sedentary from Long-Term Endurance- and Resistance-Trained Elderly. Ijms 22 (4), 1539. doi:10.3390/ijms22041539
Denham, J., Marques, F. Z., Bruns, E. L., O’Brien, B. J., and Charchar, F. J. (2016). Epigenetic Changes in Leukocytes after 8 Weeks of Resistance Exercise Training. Eur. J. Appl. Physiol. 116 (6), 1245–1253. doi:10.1007/s00421-016-3382-2
Domańska-Senderowska, D., Laguette, M.-J., Jegier, A., Cięszczyk, P., September, A., and Brzeziańska-Lasota, E. (2019). MicroRNA Profile and Adaptive Response to Exercise Training: A Review. Int. J. Sports Med. 40 (4), 227–235. doi:10.1055/a-0824-4813
Eaton, M., Granata, C., Barry, J., Safdar, A., Bishop, D., and Little, J. P. (2018). Impact of a Single Bout of High-Intensity Interval Exercise and Short-Term Interval Training on Interleukin-6, FNDC5, and METRNL mRNA Expression in Human Skeletal Muscle. J. Sport Health Sci. 7 (2), 191–196. doi:10.1016/j.jshs.2017.01.003
Fernandes, J., Arida, R. M., and Gomez-Pinilla, F. (2017). Physical Exercise as an Epigenetic Modulator of Brain Plasticity and Cognition. Neurosci. Biobehavioral Rev. 80, 443–456. doi:10.1016/j.neubiorev.2017.06.012
Fisher, A. G., Seaborne, R. A., Hughes, T. M., Gutteridge, A., Stewart, C., Coulson, J. M., et al. (2017). Transcriptomic and Epigenetic Regulation of Disuse Atrophy and the Return to Activity in Skeletal Muscle. FASEB j. 31 (12), 5268–5282. doi:10.1096/fj.201700089RR
Gehlert, S., Klinz, F., Willkomm, L., Schiffer, T., Suhr, F., and Bloch, W. (2016). Intense Resistance Exercise Promotes the Acute and Transient Nuclear Translocation of Small Ubiquitin-Related Modifier (SUMO)-1 in Human Myofibres. Ijms 17 (5), 646. doi:10.3390/ijms17050646
Gomes, C., Almeida, J. A., Franco, O. L., and Petriz, B. (2020). Omics and the Molecular Exercise Physiology. Adv. Clin. Chem. 96, 55–84. doi:10.1016/bs.acc.2019.11.003
Gonçalves, L. C., Bessa, A., Freitas-Dias, R., Luzes, R., Werneck-de-Castro, J. P. S., Bassini, A., et al. (2012). A Sportomics Strategy to Analyze the Ability of Arginine to Modulate Both Ammonia and Lymphocyte Levels in Blood after High-Intensity Exercise. J. Int. Soc. Sports Nutr. 9 (1), 30. doi:10.1186/1550-2783-9-30
Griswold, A. J., Correa, D., Kaplan, L. D., and Best, T. M. (2021). Using Genomic Techniques in Sports and Exercise Science: Current Status and Future Opportunities. Curr. Sports Med. Rep. 20 (11), 617–623. doi:10.1249/JSR.0000000000000908
Grzywacz, A., Chmielowiec, K., Boroń, A., Michałowska-Sawczyn, M., Chmielowiec, J., Trybek, G., et al. (2021). Influence of DAT1 Promotor Methylation on Sports Performance. Genes 12 (9), 1425. doi:10.3390/genes12091425
Guest, N. S., Horne, J., Vanderhout, S. M., and El-Sohemy, A. (2019). Sport Nutrigenomics: Personalized Nutrition for Athletic Performance. Front. Nutr. 6, 8. doi:10.3389/fnut.2019.00008
Hargreaves, M., and Spriet, L. L. (2020). Skeletal Muscle Energy Metabolism during Exercise. Nat. Metab. 2 (9), 817–828. doi:10.1038/s42255-020-0251-4
Hecksteden, A., Leidinger, P., Backes, C., Rheinheimer, S., Pfeiffer, M., Ferrauti, A., et al. (2016). miRNAs and Sports: Tracking Training Status and Potentially Confounding Diagnoses. J. Transl Med. 14 (1), 219. doi:10.1186/s12967-016-0974-x
Hillesheim, E., Ryan, M. F., Gibney, E., Roche, H. M., and Brennan, L. (2020). Optimisation of a Metabotype Approach to Deliver Targeted Dietary Advice. Nutr. Metab. (Lond) 17, 82. doi:10.1186/s12986-020-00499-z
Horak, M., Novak, J., and Bienertova-Vasku, J. (2016). Muscle-specific microRNAs in Skeletal Muscle Development. Dev. Biol. 410 (1), 1–13. doi:10.1016/j.ydbio.2015.12.013
Hughes, R. L. (2020). A Review of the Role of the Gut Microbiome in Personalized Sports Nutrition. Front. Nutr. 6, 191. doi:10.3389/fnut.2019.00191
Jacques, M., Hiam, D., Craig, J., Barrès, R., Eynon, N., and Voisin, S. (2019). Epigenetic Changes in Healthy Human Skeletal Muscle Following Exercise- a Systematic Review. Epigenetics 14 (7), 633–648. doi:10.1080/15592294.2019.1614416
Kakouris, N., Yener, N., and Fong, D. T. P. (2021). A Systematic Review of Running-Related Musculoskeletal Injuries in Runners. J. Sport Health Sci. 10 (5), 513–522. doi:10.1016/j.jshs.2021.04.001
Kapodistrias, N., Bobori, C., and Theocharopoulou, G. (2017). MiR-140-3p Downregulation in Association with PDL-1 Overexpression in Many Cancers: A Review from the Literature Using Predictive Bioinformatics Tools. Adv. Exp. Med. Biol. 988, 225–233. doi:10.1007/978-3-319-56246-9_18
Kashimoto, R. K., Toffoli, L. V., Manfredo, M. H. F., Volpini, V. L., Martins-Pinge, M. C., Pelosi, G. G., et al. (2016). Physical Exercise Affects the Epigenetic Programming of Rat Brain and Modulates the Adaptive Response Evoked by Repeated Restraint Stress. Behav. Brain Res. 296, 286–289. doi:10.1016/j.bbr.2015.08.038
Khoramipour, K., Sandbakk, Ø., Keshteli, A. H., Gaeini, A. A., Wishart, D. S., and Chamari, K. (2021). Metabolomics in Exercise and Sports: A Systematic Review. Sports Med. doi:10.1007/s40279-021-01582-y
Kohler, M., Schänzer, W., and Thevis, M. (2015). Effects of Exercise on the Urinary Proteome. Adv. Exp. Med. Biol. 845, 121–131. doi:10.1007/978-94-017-9523-4_12
Kuji, T., Sugasawa, T., Fujita, S.-i., Ono, S., Kawakami, Y., and Takekoshi, K. (2021). A Pilot Study of miRNA Expression Profile as a Liquid Biopsy for Full-Marathon Participants. Sports 9 (10), 134. doi:10.3390/sports9100134
Lee, H. H., McGeary, J. E., Dunsiger, S., Baker, L., Balasubramanyam, A., Knowler, W. C., et al. (2021). The Moderating Effects of Genetic Variations on Changes in Physical Activity Level and Cardiorespiratory Fitness in Response to a Life-Style Intervention: A Randomized Controlled Trial. Psychosom Med. 83 (5), 440–448. doi:10.1097/PSY.0000000000000930
Magherini, F., Gamberi, T., Pietrovito, L., Fiaschi, T., Bini, L., Esposito, F., et al. (2013). Proteomic and Carbonylation Profile Analysis of Rat Skeletal Muscles Following Acute Swimming Exercise. PLoS One 8 (8), e71839. doi:10.1371/journal.pone.0071839
Magliulo, L., Bondi, D., Pini, N., Marramiero, L., and Di Filippo, E. S. (2021). The Wonder Exerkines-Novel Insights: a Critical State-Of-The-Art Review. Mol. Cel Biochem. doi:10.1007/s11010-021-04264-5
Makhnovskii, P. A., Bokov, R. O., Kolpakov, F. A., and Popov, D. V. (2021). Transcriptomic Signatures and Upstream Regulation in Human Skeletal Muscle Adapted to Disuse and Aerobic Exercise. Ijms 22 (3), 1208. doi:10.3390/ijms22031208
Marttinen, M., Ala-Jaakkola, R., Laitila, A., and Lehtinen, M. J. (2020). Gut Microbiota, Probiotics and Physical Performance in Athletes and Physically Active Individuals. Nutrients 12 (10), 2936. doi:10.3390/nu12102936
McAuley, A. B. T., Hughes, D. C., Tsaprouni, L. G., Varley, I., Suraci, B., Roos, T. R., et al. (2021). Genetic Association Research in Football: A Systematic Review. Eur. J. Sport Sci. 21 (5), 714–752. doi:10.1080/17461391.2020.1776401
McCarthy, J. J. (2011). The MyomiR Network in Skeletal Muscle Plasticity. Exerc. Sport Sci. Rev. 39 (3), 150–154. doi:10.1097/JES.0b013e31821c01e1
Mohr, A. E., Jäger, R., Carpenter, K. C., Kerksick, C. M., Purpura, M., Townsend, J. R., et al. (2020). The Athletic Gut Microbiota. J. Int. Soc. Sports Nutr. 17 (1), 24. doi:10.1186/s12970-020-00353-w
Monnerat, G., Sánchez, C. A. R., Santos, C. G. M., Paulucio, D., Velasque, R., Evaristo, G. P. C., et al. (2020). Different Signatures of High Cardiorespiratory Capacity Revealed with Metabolomic Profiling in Elite Athletes. Int. J. Sports Physiol. Perform. 15, 1156–1167. doi:10.1123/ijspp.2019-0267
Mytidou, C., Koutsoulidou, A., Katsioloudi, A., Prokopi, M., Kapnisis, K., Michailidou, K., et al. (2021). Muscle-derived Exosomes Encapsulate myomiRs and Are Involved in Local Skeletal Muscle Tissue Communication. FASEB J. 35 (2), e21279. doi:10.1096/fj.201902468RR
Nguyen, A., Duquette, N., Mamarbachi, M., and Thorin, E. (2016). Epigenetic Regulatory Effect of Exercise on Glutathione Peroxidase 1 Expression in the Skeletal Muscle of Severely Dyslipidemic Mice. PLoS One 11 (3), e0151526. doi:10.1371/journal.pone.0151526
Nieman, D. C., and Pence, B. D. (2020). Exercise Immunology: Future Directions. J. Sport Health Sci. 9 (5), 432–445. doi:10.1016/j.jshs.2019.12.003
Nieman, D. C., and Wentz, L. M. (2019). The Compelling Link between Physical Activity and the Body's Defense System. J. Sport Health Sci. 8 (3), 201–217. doi:10.1016/j.jshs.2018.09.009
Philp, A., Rowland, T., Perez-Schindler, J., and Schenk, S. (2014). Understanding the Acetylome: Translating Targeted Proteomics into Meaningful Physiology. Am. J. Physiology-Cell Physiol. 307 (9), C763–C773. doi:10.1152/ajpcell.00399.2013
Pillon, N. J., Gabriel, B. M., Dollet, L., Smith, J. A. B., Sardón Puig, L., Botella, J., et al. (2020). Transcriptomic Profiling of Skeletal Muscle Adaptations to Exercise and Inactivity. Nat. Commun. 11 (1), 470. doi:10.1038/s41467-019-13869-w
Pintus, R., Bongiovanni, T., Corbu, S., Francavilla, V. C., Dessì, A., Noto, A., et al. (2021). Sportomics in Professional Soccer Players: Metabolomics Results during Preseason. J. Sports Med. Phys. Fitness 61 (2), 324–330. doi:10.23736/S0022-4707.20.11200-3
Pitsiladis, Y. P., Tanaka, M., Eynon, N., Bouchard, C., North, K. N., Williams, A. G., et al. (2016). Athlome Project Consortium: a Concerted Effort to Discover Genomic and Other "omic" Markers of Athletic Performance. Physiol. Genomics 48 (3), 183–190. doi:10.1152/physiolgenomics.00105.2015
Rahim, M., El Khoury, L. Y., Raleigh, S. M., Ribbans, W. J., Posthumus, M., Collins, M., et al. (2016). Human Genetic Variation, Sport and Exercise Medicine, and Achilles Tendinopathy: Role for Angiogenesis-Associated Genes. OMICS: A J. Integr. Biol. 20 (9), 520–527. doi:10.1089/omi.2016.0116
Ribeiro, I. F., Miranda-Vilela, A. L., Klautau-Guimarães, M. d. N., and Grisolia, C. K. (2013). The Influence of Erythropoietin (EPO T→G) and A-Actinin-3 (ACTN3 R577X) Polymorphisms on Runners' Responses to the Dietary Ingestion of Antioxidant Supplementation Based on Pequi Oil (Caryocar brasilienseCamb.): A Before-After Study. J. Nutrigenet Nutrigenomics 6 (6), 283–304. doi:10.1159/000357947
Ruiz, J. R., Gómez-Gallego, F., Santiago, C., González-Freire, M., Verde, Z., Foster, C., et al. (2009). Is There an Optimum Endurance Polygenic Profile? J. Physiol. 587 (Pt 7), 1527–1534. doi:10.1113/jphysiol.2008.166645
Seaborne, R. A., and Sharples, A. P. (2020). The Interplay between Exercise Metabolism, Epigenetics, and Skeletal Muscle Remodeling. Exerc. Sport Sci. Rev. 48 (4), 188–200. doi:10.1249/JES.0000000000000227
Sejersen, M. H. J., Frost, P., Hansen, T. B., Deutch, S. R., and Svendsen, S. W. (2015). Proteomics Perspectives in Rotator Cuff Research: a Systematic Review of Gene Expression and Protein Composition in Human Tendinopathy. PLoS One 10 (4), e0119974. doi:10.1371/journal.pone.0119974
Sellami, M., and Bragazzi, N. L. (2021). The Effect of Sport and Physical Activity on Transport Proteins: Implications for Cancer Prevention and Control. Adv. Protein Chem. Struct. Biol. 123, 17–26. doi:10.1016/bs.apcsb.2020.07.001
Sellami, M., Bragazzi, N. L., Aboghaba, B., and Elrayess, M. A. (2021). The Impact of Acute and Chronic Exercise on Immunoglobulins and Cytokines in Elderly: Insights from a Critical Review of the Literature. Front. Immunol. 12, 631873. doi:10.3389/fimmu.2021.631873
Sellami, M., Bragazzi, N., Prince, M. S., Denham, J., and Elrayess, M. (2021). Regular, Intense Exercise Training as a Healthy Aging Lifestyle Strategy: Preventing DNA Damage, Telomere Shortening and Adverse DNA Methylation Changes over a Lifetime. Front. Genet. 12, 652497. doi:10.3389/fgene.2021.652497
Sillanpää, E., Palviainen, T., Ripatti, S., Kujala, U. M., and Kaprio, J. (2021). Polygenic Score for Physical Activity Is Associated with Multiple Common Diseases. Med. Sci. Sports Exerc. Publish Ahead of Print. doi:10.1249/MSS.0000000000002788
Sun, X., Haider Ali, M. S. S., and Moran, M. (2017). The Role of Interactions of Long Non-coding RNAs and Heterogeneous Nuclear Ribonucleoproteins in Regulating Cellular Functions. Biochem. J. 474 (17), 2925–2935. doi:10.1042/BCJ20170280
Tanisawa, K., Wang, G., Seto, J., Verdouka, I., Twycross-Lewis, R., Karanikolou, A., et al. (2020). Sport and Exercise Genomics: the FIMS 2019 Consensus Statement Update. Br. J. Sports Med. 54 (16), 969–975. doi:10.1136/bjsports-2019-101532
Tarnowski, M., Kopytko, P., and Piotrowska, K. (2021). Epigenetic Regulation of Inflammatory Responses in the Context of Physical Activity. Genes 12 (9), 1313. doi:10.3390/genes12091313
Tashjian, R. Z., Kim, S. K., Roche, M. D., Jones, K. B., and Teerlink, C. C. (2021). Genetic Variants Associated with Rotator Cuff Tearing Utilizing Multiple Population-Based Genetic Resources. J. Shoulder Elbow Surg. 30 (3), 520–531. doi:10.1016/j.jse.2020.06.036
Ubaida-Mohien, C., Gonzalez-Freire, M., Lyashkov, A., Moaddel, R., Chia, C. W., Simonsick, E. M., et al. (2019). Physical Activity Associated Proteomics of Skeletal Muscle: Being Physically Active in Daily Life May Protect Skeletal Muscle from Aging. Front. Physiol. 10, 312. doi:10.3389/fphys.2019.00312
Wong, O., Marshall, K., Sicova, M., Guest, N. S., García-Bailo, B., and El-Sohemy, A. (2021). CYP1A2 Genotype Modifies the Effects of Caffeine Compared with Placebo on Muscle Strength in Competitive Male Athletes. Int. J. Sport Nutr. Exerc. Metab. 31, 420–426. doi:10.1123/ijsnem.2020-0395
Xu, M., Zhu, J., Liu, X.-D., Luo, M.-Y., and Xu, N.-J. (2021). Roles of Physical Exercise in Neurodegeneration: Reversal of Epigenetic Clock. Transl Neurodegener 10 (1), 30. doi:10.1186/s40035-021-00254-1
Yao, R.-W., Wang, Y., and Chen, L.-L. (2019). Cellular Functions of Long Noncoding RNAs. Nat. Cel Biol 21 (5), 542–551. doi:10.1038/s41556-019-0311-8
Keywords: molecular big data, data science, sports medicine, sports sciences, exercise and physical activity, OMICS sports sciences
Citation: Sellami M, Elrayess MA, Puce L and Bragazzi NL (2022) Molecular Big Data in Sports Sciences: State-of-Art and Future Prospects of OMICS-Based Sports Sciences. Front. Mol. Biosci. 8:815410. doi: 10.3389/fmolb.2021.815410
Received: 15 November 2021; Accepted: 20 December 2021;
Published: 11 January 2022.
Edited by:
Luciana Hannibal, University of Freiburg Medical Center, GermanyReviewed by:
L. C. Cameron, Rio de Janeiro State Federal University, BrazilCopyright © 2022 Sellami, Elrayess, Puce and Bragazzi. This is an open-access article distributed under the terms of the Creative Commons Attribution License (CC BY). The use, distribution or reproduction in other forums is permitted, provided the original author(s) and the copyright owner(s) are credited and that the original publication in this journal is cited, in accordance with accepted academic practice. No use, distribution or reproduction is permitted which does not comply with these terms.
*Correspondence: Nicola Luigi Bragazzi, cm9iZXJ0b2JyYWdhenppQGdtYWlsLmNvbQ==
Disclaimer: All claims expressed in this article are solely those of the authors and do not necessarily represent those of their affiliated organizations, or those of the publisher, the editors and the reviewers. Any product that may be evaluated in this article or claim that may be made by its manufacturer is not guaranteed or endorsed by the publisher.
Research integrity at Frontiers
Learn more about the work of our research integrity team to safeguard the quality of each article we publish.