- 1Department of Pathophysiology, Guangdong Provincial Key Laboratory of Brain Function and Disease, Zhongshan School of Medicine, Sun Yat-sen University, Guangzhou, China
- 2Department of Optical Neural and Molecular Physiology, Graduate School of Biostudies, Kyoto University, Kyoto, Japan
- 3Precursory Research for Embryonic Science and Technology (PRESTO), Japan Science and Technology Agency, Kyoto, Japan
Membrane potential is the critical parameter that reflects the excitability of a neuron, and it is usually measured by electrophysiological recordings with electrodes. However, this is an invasive approach that is constrained by the problems of lacking spatial resolution and genetic specificity. Recently, the development of a variety of fluorescent probes has made it possible to measure the activity of individual cells with high spatiotemporal resolution. The adaptation of this technique to image electrical activity in neurons has become an informative method to study neural circuits. Genetically encoded voltage indicators (GEVIs) can be used with superior performance to accurately target specific genetic populations and reveal neuronal dynamics on a millisecond scale. Microbial rhodopsins are commonly used as optogenetic actuators to manipulate neuronal activities and to explore the circuit mechanisms of brain function, but they also can be used as fluorescent voltage indicators. In this review, we summarize recent advances in the design and the application of rhodopsin-based GEVIs.
Introduction
Probing functional neural circuits at high spatiotemporal resolution is crucial for understanding how neuronal populations work together to generate behavior. To do this, it is necessary to measure neural activity from multiple neurons simultaneously. Electrophysiological approaches are used to measure membrane potential as the gold standard. However, the results acquired by recording with electrodes lack spatial resolution and genetic specificity. Optical imaging with genetically encoded indicators can overcome these drawbacks and monitor the activity of large numbers of neurons simultaneously.
Since somatic calcium influx is coupled with action potentials (APs), the activity of large numbers of neurons can be monitored simultaneously using calcium imaging as an indirect measurement of neuronal firing with an excellent signal-to-noise ratio (SNR) (Yuste and Katz, 1991; Grienberger and Konnerth, 2012). Genetically encoded calcium indicators (GECIs) are the most widely used to monitor neural activity in vitro and in vivo (Nakai et al., 2001; Tian et al., 2009; Zhao et al., 2011; Akerboom et al., 2012; Ohkura et al., 2012; Chen et al., 2013; Inoue et al., 2015, 2019; Dana et al., 2019). With calcium imaging, it is possible to measure spiking activity from thousands of neurons in neural circuits with single-cell resolution in behaving animals (Ziv et al., 2013; Sofroniew et al., 2016; Stirman et al., 2016; Ota et al., 2021). Furthermore, in addition to measuring the activity in the somata, the activity in other subcellular domains like dendritic spines and axonal boutons can be measured in vivo (Chen et al., 2013; Broussard et al., 2018; Inoue et al., 2019).
However, calcium dynamics revealed by fluorescent calcium indicators are not a direct measurement of membrane potential. Thus, calcium imaging is limited in its ability to provide a complete description of neuronal activity. First, somatic calcium imaging readouts only APs (Smetters et al., 1999). Subthreshold excitatory or inhibitory synaptic inputs are practically invisible in somatic calcium signals, making it difficult to monitor the relationship between the synaptic inputs and outputs. Second, due to biophysical constraints, calcium dynamics are significantly slower than the timescale of membrane potential dynamics. Therefore, when neurons fire a burst of spikes at > 40 Hz, it is difficult to assess the number of spikes and spike timimgs quantitatively with population calcium imaging (Smetters et al., 1999). Third, calcium dynamics are shaped by complicated interactions between ionic diffusion and extrusion, and they can be significantly altered by intrinsic and extrinsic calcium buffers and the expression of calcium indicators themselves (Neher, 1998). Calcium imaging is not an ideal method to measure neural activity for these reasons.
Voltage imaging, on the other hand, can directly monitor the electrical activity of each neuron, including subthreshold events (Peterka et al., 2011; Storace et al., 2016). Intensive efforts have been made to develop genetically encoded voltage indicators (GEVIs) (Akemann et al., 2010; Jin et al., 2012; Tsutsui et al., 2013; St-Pierre et al., 2014; Piao et al., 2015; Inagaki et al., 2017). These genetic indicators can target and measure specific cell types or subcellular compartments (Kwon et al., 2017). Newer GEVIs can detect subthreshold activity that is not detectable with calcium imaging both in vitro and in vivo (Bando et al., 2019; Villette et al., 2019), making it possible to generate more accurate decoding of brain functions. Therefore, voltage imaging using GEVIs appears to be a powerful tool that can supersede calcium imaging.
Microbial rhodopsins were initially used for optogenetic control of membrane potential (Boyden et al., 2005; Han and Boyden, 2007; Chow et al., 2010). It turned out that these rhodopsins also show a membrane voltage-dependent fluorescent change that is derived from the retinal chromophore (Kralj et al., 2011a, 2011b; Kojima et al., 2020). Compared with other types of GEVIs (ion channel-based or voltage-sensitive domain (VSD)-based), rhodopsin-based GEVIs display higher sensitivity and faster kinetics, and the use of this type of sensor has become widespread (Flytzanis et al., 2014; Hochbaum et al., 2014; Piatkevich et al., 2018; Adam et al., 2019; Chien et al., 2021). In this review, we will introduce recent advances in the design and the application of rhodopsin-based GEVIs. We hope that this review will enable the readers to choose the optimal GEVIs for their specific application and inspire the development and improvements of GEVIs.
Mechanism of Microbial Rhodopsins as a Voltage Indicator
Due to the low quantum yield of the retinal chromophore, little attention has been given to the fluorescence of rhodopsin. Kralj and colleagues developed a new type of GEVI based on microbial rhodopsins and their fluorescence. They found that proteorhodopsin, a light-driven proton pump discovered from uncultivated marine γ-proteobacteria, can detect the electrical activity in bacteria (Kralj et al., 2011b). By exploiting its properties, they developed a proteorhodopsin optical proton sensor (PROPS), and the authors measured membrane voltage fluctuations in E. Coli (Kralj et al., 2011b). However, PROPS does not localize to the plasma membranes of eukaryotic cells efficiently. They further screened other microbial rhodopsins and found Archaerhodopsin-3 (Arch) from Halorubrum sodomense reliably expressed and trafficked to the plasma membrane well in mammalian and successfully reported membrane potentials in neurons (Kralj et al., 2011a).
Arch serves as a light-driven outward proton pump, and it is utilized as an inhibitory optogenetic actuator that is activated by green light (Chow et al., 2010). Retinal is bound to a specific lysine residue (K226) in the seventh helix of apoprotein (opsin) via a Schiff base (Figure 1A). When absorbing light, rhodopsin molecules in the ground state lead to the Frank-Condon state and then form the reactive state (S1r) or nonreactive S1 states (S1nr) within several tens of femtoseconds (Figure 1B). When the rhodopsin molecule in S1nr is illuminated, the excess energy is released as fluorescence, and the molecule returns to the ground state. This spontaneous emission is a common property of microbial rhodopsins (Figure 1B) (Nakamura et al., 2008; Kojima et al., 2020). In the reactive state (S1r), on the other hand, the retinal is isomerized from the all-trans to the 13-cis form. This light-induced isomerization triggers further distinctive photointermediates such as the K-, the L-, the M-, the N-, and the O-states, followed by returning to the ground state (Figure 1B). It also forms a Q-intermediate state when absorbing light in the N-intermediate state (Figure 1B) (Ohtani et al., 1992; Maclaurin et al., 2013). The Q-intermediate state emits fluorescence that is about 100 times larger than that of spontaneous emission. By comparing these different fluorescence intensities in mammalian neurons, rhodopsin fluorescence in Arch was derived from the Q-intermediate state (Kojima et al., 2020). Also, such photointermediate fluorescence arises from a sequential three-photon process. Photon 1 initiates the photocycle that Schiff base is protonated, and Arch transits from the ground state to N-intermediate state. Photon 2 further generates a Q-intermediate state, and photon 3 enables yield fluorescence (Figure 1B) (Maclaurin et al., 2013).
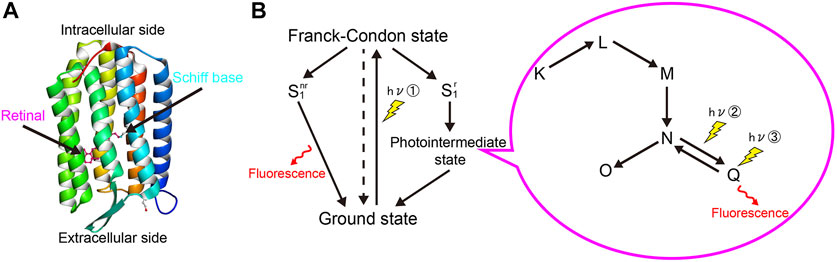
FIGURE 1. Fluorescence mechanism of microbial rhodopsins. (A) Crystal structure of Arch (PDB code: 6GUZ). Rhodopsin is a membrane protein with a seven-fold transmembrane alpha-helix structure and consists of a protein moiety called opsin and a retinal chromophore that is covalently bound to the apoprotein via a Schiff base. (B) Photoreaction scheme of microbial rhodopsins. The spontaneous emission (left) occurs from the nonreactive S1 state. The photointermediate fluorescence (right) is from the Q-intermediate state produced by a photon absorption of the N-intermediate in its photocycle. Photointermediate fluorescence arises through the sequential action of three photons (① - ③). The dashed line represents the non-radiative relaxation process.
Microbial Rhodopsin-Based Voltage Indicators
Arch can effectively reflect membrane potentials with extremely high temporal resolution. For voltage imaging, Arch and its mutants are excited by red light (640 nm) and emit in the infrared wavelength (peak at 715 nm) (Figure 2A) (Kralj et al., 2011a). Such voltage sensitivity arises through protonation of the Schiff in the photointermediate state, not the ground state. However, their practical applications are limited by their weak fluorescence (equal to 1/500–1/50 of EGFP) and insufficient SNR (Kralj et al., 2011a; Maclaurin et al., 2013). To overcome these problems, efforts were devoted to improving Arch performance (Figure 2B, Table 1). Mutating residues related to the photocycle or around the retinal Schiff base could significantly modify Arch’s brightness and SNR. Consequently, several GEVIs available in vitro and in vivo were developed (Kralj et al., 2011a; Gong et al., 2013; Flytzanis et al., 2014; Hochbaum et al., 2014; Piatkevich et al., 2018; Adam et al., 2019).
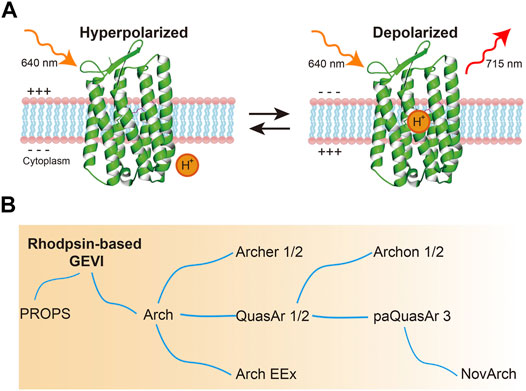
FIGURE 2. Microbial rhodopsin-based GEVIs. (A) Voltage sensing mechanism of microbial rhodopsin-based GEVIs. Rhodopsin-based GEVIs report voltage changes through the fluorescence intensity changes of retinal chromophore caused by protonation of the Schiff base in the photointermediate state, not in the ground state. (B) Evolution of microbial rhodopsin-based GEVIs.
Wild-type Arch generates hyperpolarizing photocurrents upon exposure to an imaging laser. By changing the residue of the first position of proton translocation in the photocycle (D95), the photocurrent could be significantly eliminated; however, this mutation also made the rise time of Arch ∼45 ms slower (Table 1) (Kralj et al., 2011a; Gong et al., 2013). D106 is the primary conduit for protons to protonate and deprotonate the voltage-sensitive Schiff base during modulations of membrane voltage. Gong and colleagues combined the mutation D95N/Q with D106E and generated the GEVIs called Arch-EEN and Arch-EEQ, and these showed a faster response (∼5–15 ms rise time) to APs when compared to Arch-D95N (Gong et al., 2013). Archer1 and 2 were generated based on the spectral shifts mutation of D95E, T99C, and A225M that was reported in Gloeobacter violaceus rhodopsin (GR), resulting in higher brightness and SNR (Engqvist et al., 2015). Archer1 also worked as a bi-functional tool, detecting membrane potential with red light illumination and inhibiting neural activity with green light illumination (Flytzanis et al., 2014). Furthermore, Venkatachalam and colleagues developed methods for light-gated photochemical voltage recording by modulating the photophysical properties of Arch. By illuminating a neural circuit during a user-defined “write” interval, a photochemical imprint was formed within each cell of the amount of electrical activity during the write interval. This fluorescence can be probed later (Venkatachalam et al., 2014).
Random mutagenesis was another widely used approach to optimize Arch-based voltage indicators, which significantly advanced their kinetics and fluorescence (Table 1). QuasAr1 contains the mutation of P60S, T80S, D95H, D106H, and F161V, and QuasAr2 was generated by changing H95Q in the QuasAr1. Both of these indicators were characterized by significant improvements in brightness and sensitivity. Notably, QuasAr2 showed an approximately 90% ΔF/F to 100 mV membrane voltage change in HEK293T cells and resolved APs in organotypic slice culture (Hochbaum et al., 2014). A newly developed QuasAr3 (K171R to QuasAr2) further improved the expression level of the indicator and had an excellent membrane trafficking property, allowing one to detecting voltage dynamics in vivo (Adam et al., 2019). A point mutation (V59A) in QuasAr3 enhanced the population of the fluorescent from the Q-intermediate state. Thus, QuasAr3 (V59A) resulted in a “photoactivated QuasAr3” (paQuasAr3) that enhanced the baseline fluorescence 2-3-fold upon blue light illumination in HEK293T cells (Adam et al., 2019). NovArch, which introduced mutations of V209I and I213T to paQuasAr3, emits enhanced infrared fluorescence with additional weak two-photon catalytically excitation with any light other than blue light (Chien et al., 2021). Simultaneous one-photon and two-photon excitation of NovArch resolved single cells in an acute brain slice at depth up to ∼220 μm where conventional one-photon excitation wide-field and confocal approaches could not. Additionally, NovArch was able to detect back-propagating APs of dendrites in acute brain slices (Chien et al., 2021). Meanwhile, Piatkevich and colleagues developed a computer-vision-guided high-throughput screening system to optimize GEVI’s brightness and membrane localization. By screening QuasAr2 mutant libraries that were generated by error-prone PCR and site-directed mutagenesis, the authors identified multiple residues of QuasAr2 that yield better performance in brightness, membrane localization, and voltage sensitivity (Piatkevich et al., 2018). Compared to QuasAr2, Archon1 has the additional mutations of T20S, G41A, V44E, S80P, D88N, A137T, T184I, L199I, and G242Q. Archon2 has the additional mutations of T56P, S80P, T100C, T118I, T184I, L199I, and A226C. Archon1 is more resistant to photobleaching, retaining ∼95% of its baseline fluorescence after exposure to intensive light (800 mW/mm2) for 15 min, while other Arch-based indicators lost at least 25% fluorescence (Piatkevich et al., 2018). Archon1 had a high performance in its ability to detect neural activity in mouse brain slices (Piatkevich et al., 2018). Compared to Archon1, Archon2 had faster kinetics but had lower sensitivity. Also, the targeting of Archon1 to the soma (SomArchon) by adding a trafficking motif from the Kv2.1 potassium channel improved its SNR and sensitivity (Piatkevich et al., 2019).
Arch-based voltage indicators can be applied in conjunction with spectrally orthogonal optogenetic actuators. This simultaneous stimulation and the corresponding readout of membrane potential via light is called “all-optical electrophysiology” or “Optopatch.” Hochbaum and colleagues generated a blue-shifted channelrhodopsin actuator (CheRiff) to use in combination with QuasAr2. Notably, intense stimulation of QuasAr2 with a red laser did not induce any currents when this new actuator was used in cultured neurons and brain slices (Hochbaum et al., 2014). Later this combination was successfully applied for high-throughput screening of a Nav1.7-specific blocker from a library of candidates (Zhang et al., 2016). Moreover, the Cre-dependent transgenic mouse line “Floxopatch,” which expresses the combination of QuasAr2 and CheRiff, enabled the characterization of neural activity in genetically specified cell types in intact tissue (Lou et al., 2016). Another combination of using high-photocurrent channelrhodopsin from Chloromonas oogama (CoChR) and Archon1/2 also showed excellent performance (Piatkevich et al., 2018). This strategy has been applied successfully in vivo (Piatkevich et al., 2019; Fan et al., 2020).
In addition to optogenetic actuators, these microbial rhodopsin-based GEVIs work together with other sensors, such as calcium indicators and pH sensors. For example, a study imaged the changes in voltage with QuasAr2 and the changes in calcium with GECIs simultaneously to explore the correlations between the voltage variations and APs in neurons (Fan et al., 2018). A similar approach was used in a cardiology study to screen for the protective effect of cardioprotective drug candidates by tracking calcium, membrane voltage, and motion path in human induced pluripotent stem cell-derived cardiomyocytes (Nguyen et al., 2019). In addition, Werley and colleagues developed a technique called MOSAIC (Multiplexed Optical Sensors in Arrayed Islands of Cells) that introduced GEVIs and ∼20 other sensors to various kinds of cultured cells. These multiplexed data collected from multiple recordings allow further exploration of complex physiological responses in multiple cell types (Werley et al., 2020).
eFRET-Based Voltage Indicators
Despite the intensive engineering of Arch variants, the brightness is still lower than that of widely-used fluorescent proteins. To overcome the low fluorescent limitation of rhodopsins, an eFRET (electrochromic Förster resonance energy transfer) strategy was wisely developed. Microbial rhodopsins have absorption spectrum that overlaps with the emission spectrum of widely-used fluorescent proteins. Therefore, fluorescent proteins and other chemical fluorophores can serve as FRET donors, while rhodopsin molecules can serve as FRET acceptors (Bayraktar et al., 2012). eFRET sensors measure the absorption change of rhodopsin through the quenching of an attached fluorescent protein. When neurons depolarize, the fluorescent protein intensity is decreased by FRET from the fluorescent protein to the rhodopsin (Figure 3A) (Gong et al., 2014, 2015; Zou et al., 2014). Thus, these FRET-opsin sensors detect voltage depolarization by the decrease in emission intensity from the fluorescence donor. The rhodopsins utilized in this type of GEVIs were not limited to Arch (Zou et al., 2014). Mac (bacteriorhodopsin from Leptosphaeria maculans) (Gong et al., 2014) and Ace2 (bacteriorhodopsin from Acetabularia acetabulum) (Gong et al., 2015; Kannan et al., 2018; Beck and Gong, 2019) were also successfully used to generate new indicators that can detect spikes in neurons with fast kinetics and high SNR (Figure 3B, Table1). Microbial rhodopsins have a broad absorption spectrum (Kojima et al., 2020), so various fluorescent proteins with different colors can be utilized as donors (Gong et al., 2014, 2015; Zou et al., 2014; Kannan et al., 2018; Beck and Gong, 2019).
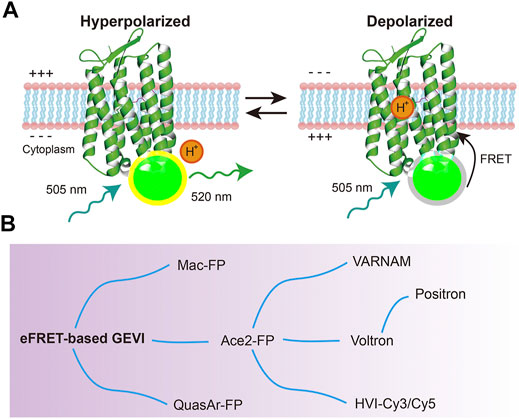
FIGURE 3. eFRET-based GEVIs. (A) Voltage sensing mechanism of eFRET-based GEVIs (Ace2N-mNeon). At a depolarized stage, the Schiff base of microbial rhodopsin is protonated, and the absorbance of rhodopsin changes. This absorption quenches the fluorescence of the appended fluorescent proteins or other bright fluorophores. (B) Evolution of eFRET-based GEVIs.
Synthetic fluorescent dyes are also available as FRET donors (Table 1). Voltron has a self-labelling tag domain (HaloTag, 34 kDa) to use Janelia Fluor dyes as the FRET donor (Abdelfattah et al., 2019). The synthetic dyes are more photostable and brighter than the fluorescent proteins, allowing for in vivo voltage imaging from large fields of view. Since the absorbance of rhodopsins increases in response to depolarization, eFRET-based GEVIs have negatively sloped fluorescence-voltage relationships. This means that the indicators become dimmer when the neurons depolarize (negative-going). Positron is a positive-going eFRET-based GEVI generated from Voltron, and it possesses identical kinetics and sensitivity as Voltron (Abdelfattah et al., 2020). Furthermore, Arch- and Mac-based positive-going eFRET GEVIs have been developed by modifying the natural proton transport pathway within microbial rhodopsins (Abdelfattah et al., 2020). In addition, other hybrid eFRET indicators, HVI-Cy3 and HVI-Cy5, were reported (Liu et al., 2021). In these constructs, the fluorophore was directly linked to a small peptide (1.6 kDa) inserted at the extracellular loop of the rhodopsin, resulting in high FRET efficiency.
In vivo Voltage Imaging with Rhodopsin-Based Indicators
The primary goal of voltage imaging is to visualize neuronal activity in vivo. The development of new probes and an imaging apparatus has shed light on the activity of neurons in behaving animals. The endoplasmic reticulum (ER) and the Golgi export trafficking signal (TS) could significantly improve membrane localization of microbial rhodopsin (Gradinaru et al., 2010). Moreover, a trafficking motif from the soma-localized Kv2.1 potassium channel could confine the GEVI expression to the soma (Baker et al., 2016), which dramatically decreased the background noise and further improve SNR in the living mammalian brain (Adam et al., 2019; Piatkevich et al., 2019). Combined with these motifs, Arch-based SomArchon and paQuasAr3-s successfully target somata and detect their fluorescence with cellular resolution in vivo (Adam et al., 2019; Piatkevich et al., 2019). These indicators also could record neuronal activity from multiple neurons simultaneously in the hippocampus, enabling the study of correlation and coherence of subthreshold activity between pairs of neurons (Adam et al., 2019; Piatkevich et al., 2019). Furthermore, paQuasAr3 enabled the detection of back-propagating APs from dendrites in the hippocampal CA1 region.
MacQ-mCitrine was the first indicator used to investigate neuron membrane voltage of mice and flies in vivo due to its bright fluorescence baseline as an eFRET based voltage indictor (Gong et al., 2014). Then, Ace2N-mNeon responded 5–6 times faster than MacQ-mCitrine and provided exquisite spike-timing accuracy. Moreover, Ace2N-mNeon and Voltron were used to measure spiking activity with precise orientation selectivity in the primary visual cortex during the presentation of drifting grating stimuli (Gong et al., 2015; Abdelfattah et al., 2019). Voltron, in particular, showed superior photostability and allowed for over 15 min of continuous imaging (Abdelfattah et al., 2019).
Voltage imaging is possible in freely moving animals using optical fibers. Mashall and colleagues developed a method named “trans-membrane electrical measurement performed optically (TEMPO)” to record the changes in the voltage dynamics by using fluctuations in the fluorescence of Mac-mCitrine or Ace2N-mNeon (Marshall et al., 2016). They succeeded in measuring the activity of D1-or D2-dopamine receptor-expressing striatal medium spiny neurons (Marshall et al., 2016). Also, using both an eFRET-based red indicator (VARNAM) in conjunction with TEMPO was able to accurately detect theta (6–10 Hz) and delta (0.5–4 Hz) oscillatory waves in the CA1 region of the hippocampus (Kannan et al., 2018).
The fluorescent fluctuations of rhodopsin-based GEVIs are barely detectable by two-photon microscopy, so the imaging is still restricted to conventional one-photon microscopy that lacks optical sections. To improve the imaging acquisition conditions, Adam and colleagues introduced a digital mirror device for targeted illumination and succeeded in improving the SNR (Adam et al., 2019). Further improvement of SNR was achieved by the use of a spatial light modulator to restrict the illumination area more precisely (Fan et al., 2020). In addition to the performance of GEVIs, optimization of the technology to analyze the data is also essential. Recently, two analysis pipelines (VolPy and SGPMD-NMF) were developed for the processing of voltage imaging data (Cai et al., 2021; Xie et al., 2021). Both pipelines could correct motion artifacts, denoise voltage signals, and extract APs and subthreshold signals from the raw imaging data recorded in mouse and zebrafish brains in vivo.
Conclusion
The development of microbial rhodopsin-based GEVIs helped significantly advance our ability to detect neuronal activity with high spatiotemporal resolution. These indicators bring hope for us to elucidate better how networks of synaptic connections in the brain work together precisely. Furthermore, all living cells have membrane potentials. Therefore, it is interesting to apply voltage imaging to cells other than neurons to elucidate biological phenomena.
However, the rhodopsin-based indicators have a significant downfall due to the fact that they yield a low amount of fluorescence. In addition, the ultra-intensive laser (∼500 W/cm2) quickly brings side effects, such as heat damage to the tissue. These problems may be addressed by modifying these sensors to improve their brightness and photostability. The alternative method is to develop synthetic retinal analogs with strong absolute fluorescence (Sineshchekov et al., 2012; Herwig et al., 2017; Hontani et al., 2018). Also, due to the three-photon state mechanism described above, two-photon excitation tends to lose voltage sensitivity even with eFRET-based GEVIs (Maclaurin et al., 2013; Chamberland et al., 2017; Bando et al., 2019). Solving this problem would be a significant advance toward deeper-tissue voltage imaging.
One of the advantages of voltage imaging is to record neuronal activity from multiple neurons simultaneously. However, the time resolution and SNR of voltage imaging are still inferior compared to the patch-clamp recording. For practical use, voltage imaging needs to be able to measure subthreshold activity and decode absolute voltage from fluorescence changes. In fact, there have been several efforts to develop absolute voltage indicators based on microbial rhodopsins (Hou et al., 2014). Also, the fluorescent signal from population voltage imaging is correlated with local field potentials (Marshall et al., 2016; Bando et al., 2019; Piatkevich et al., 2019). Recently, implantable multi-electrode arrays (MEAs) are available for extracellular measurements of neural activity with high spatiotemporal resolution (Obien et al., 2014). Therefore, it is interesting to use MEAs for evaluating the performance of GEVIs.
The imaging apparatus and data processing play critical roles in voltage imaging. Membrane potential fluctuation occurs on a millisecond timescale. Thus, high-speed cameras with a large field of view are required to acquire images at a comparable frequency from multiple neurons. Also, an information processing system to handle big data is essential. For example, a size of 1-min voltage imaging by a sCMOS camera (512 pixels x 128 pixels, frame rate: 1 kHz) would be approximately 8 GB. Moreover, the signal of voltage imaging contains multiple waveforms. Data processing requires an accurate and coherent definition of APs, subthreshold activities, and background noise. Future directions need to focus on these aspects to improve the application of GEVIs with higher fidelity and reproducibility.
Despite these limitations, the development of GEVIs has allowed us to further investigate information dynamics and processes within neurons. Ultimately, voltage imaging will revolutionize the technology of imaging neural activity. It will make it possible to elucidate fundamental principles of how the brain functions, such as neuronal activity integration, information processing in micro-and long-range circuits, and the neuronal states.
Author Contributions
MS designed the concept. XMZ and MS searched the literature, wrote the manuscript, and made the figures and table. TY contributed to review of the manuscript. All authors reviewed and edited the final manuscript.
Funding
This work was supported in part by grants from Precursory Research for Embryonic Science and Technology (PRESTO)-JST (JPMJPR1906 to M.S.), AMED (JP20dm0207060, JP20dm0107159, JP21wm0525004 to M.S.), JSPS KAKENHI (JP20H04122, JP21K19429 to M.S.), Takeda Science Foundation (to M.S.), Uehara Memorial Foundation (to M.S.), Tokyo Biochemical Research Foundation (to M.S.), Research Foundation for Opto-Science and Technology (to M.S.), Lotte Foundation (to M.S.), The Konica Minolta Science and Technology Foundation (to M.S.), Brain Science Foundation (to M.S.), Nakatani Foundation (to M.S.).
Conflict of Interest
The authors declare that the research was conducted in the absence of any commercial or financial relationships that could be construed as a potential conflict of interest.
Publisher’s Note
All claims expressed in this article are solely those of the authors and do not necessarily represent those of their affiliated organizations, or those of the publisher, the editors, and the reviewers. Any product that may be evaluated in this article, or claim that may be made by its manufacturer, is not guaranteed or endorsed by the publisher.
Acknowledgments
We are grateful to Drs. Adam T Guy and Jennifer Day for carefully proofreading the manuscript. We also thank Drs. Yuki Sudo (Okayama University) and Haruhiko Bito (The University of Tokyo) for continuous collaboration and encouragement.
References
Abdelfattah, A. S., Kawashima, T., Singh, A., Novak, O., Liu, H., Shuai, Y., et al. (2019). Bright and Photostable Chemigenetic Indicators for Extended In Vivo Voltage Imaging. Science 365, 699–704. doi:10.1126/science.aav6416
Abdelfattah, A. S., Valenti, R., Zheng, J., Wong, A., Chuong, A. S., Hasseman, J. P., et al. (2020). A General Approach to Engineer Positive-Going eFRET Voltage Indicators. Nat. Commun. 11, 3444. doi:10.1038/s41467-020-17322-1
Adam, Y., Kim, J. J., Lou, S., Zhao, Y., Xie, M. E., Brinks, D., et al. (2019). Voltage Imaging and Optogenetics Reveal Behaviour-dependent Changes in Hippocampal Dynamics. Nature 569, 413–417. doi:10.1038/s41586-019-1166-7
Akemann, W., Mutoh, H., Perron, A., Rossier, J., and Knöpfel, T. (2010). Imaging Brain Electric Signals with Genetically Targeted Voltage-Sensitive Fluorescent Proteins. Nat. Methods. 7, 643–649. doi:10.1038/nmeth.1479
Akerboom, J., Chen, T.-W., Wardill, T. J., Tian, L., Marvin, J. S., Mutlu, S., et al. (2012). Optimization of a GCaMP Calcium Indicator for Neural Activity Imaging. J. Neurosci. 32, 13819–13840. doi:10.1523/JNEUROSCI.2601-12.2012
Baker, C. A., Elyada, Y. M., Parra, A., and Bolton, M. M. (2016). Cellular Resolution Circuit Mapping with Temporal-Focused Excitation of Soma-Targeted Channelrhodopsin. Elife 5, 1–15. doi:10.7554/eLife.14193
Bando, Y., Sakamoto, M., Kim, S., Ayzenshtat, I., and Yuste, R. (2019). Comparative Evaluation of Genetically Encoded Voltage Indicators. Cel. Rep. 26, 802–813. doi:10.1016/j.celrep.2018.12.088
Bayraktar, H., Fields, A. P., Kralj, J. M., Spudich, J. L., Rothschild, K. J., and Cohen, A. E. (2012). Ultrasensitive Measurements of Microbial Rhodopsin Photocycles Using Photochromic FRET. Photochem. Photobiol. 88 (1), 90–97. doi:10.1111/j.1751-1097.2011.01011.x
Beck, C., and Gong, Y. (2019). A High-Speed, Bright, Red Fluorescent Voltage Sensor to Detect Neural Activity. Sci. Rep. 9, 15878. doi:10.1038/s41598-019-52370-8
Boyden, E. S., Zhang, F., Bamberg, E., Nagel, G., and Deisseroth, K. (2005). Millisecond-timescale, Genetically Targeted Optical Control of Neural Activity. Nat. Neurosci. 8, 1263–1268. doi:10.1038/nn1525
Broussard, G. J., Liang, Y., Fridman, M., Unger, E. K., Meng, G., Xiao, X., et al. (2018). In Vivo measurement of Afferent Activity with Axon-specific Calcium Imaging. Nat. Neurosci. 21, 1272–1280. doi:10.1038/s41593-018-0211-4
Cai, C., Friedrich, J., Singh, A., Eybposh, M. H., Pnevmatikakis, E. A., Podgorski, K., et al. (2021). VolPy: Automated and Scalable Analysis Pipelines for Voltage Imaging Datasets. PLOS Comput. Biol. 17, e1008806. doi:10.1371/journal.pcbi.1008806
Chamberland, S., Yang, H. H., Pan, M. M., Evans, S. W., Guan, S., Chavarha, M., et al. (2017). Fast Two-Photon Imaging of Subcellular Voltage Dynamics in Neuronal Tissue with Genetically Encoded Indicators. Elife 6, e25690. doi:10.7554/eLife.25690
Chen, T.-W., Wardill, T. J., Sun, Y., Pulver, S. R., Renninger, S. L., Baohan, A., et al. (2013). Ultrasensitive Fluorescent Proteins for Imaging Neuronal Activity. Nature 499, 295–300. doi:10.1038/nature12354
Chien, M.-P., Brinks, D., Testa-Silva, G., Tian, H., Phil Brooks, F., Adam, Y., et al. (2021). Photoactivated Voltage Imaging in Tissue with an Archaerhodopsin-Derived Reporter. Sci. Adv. 7, eabe3216. doi:10.1126/sciadv.abe3216
Chow, B. Y., Han, X., Dobry, A. S., Qian, X., Chuong, A. S., Li, M., et al. (2010). High-performance Genetically Targetable Optical Neural Silencing by Light-Driven Proton Pumps. Nature 463, 98–102. doi:10.1038/nature08652
Dana, H., Sun, Y., Mohar, B., Hulse, B. K., Kerlin, A. M., Hasseman, J. P., et al. (2019). High-performance Calcium Sensors for Imaging Activity in Neuronal Populations and Microcompartments. Nat. Methods. 16, 649–657. doi:10.1038/s41592-019-0435-6
Engqvist, M. K. M., McIsaac, R. S., Dollinger, P., Flytzanis, N. C., Abrams, M., Schor, S., et al. (2015). Directed Evolution of Gloeobacter Violaceus Rhodopsin Spectral Properties. J. Mol. Biol. 427, 205–220. doi:10.1016/j.jmb.2014.06.015
Fan, L. Z., Kheifets, S., Böhm, U. L., Wu, H., Piatkevich, K. D., Xie, M. E., et al. (2020). All-Optical Electrophysiology Reveals the Role of Lateral Inhibition in Sensory Processing in Cortical Layer 1. Cell 180, 521–535. doi:10.1016/j.cell.2020.01.001
Fan, L. Z., Nehme, R., Adam, Y., Jung, E. S., Wu, H., Eggan, K., et al. (2018). All-optical Synaptic Electrophysiology Probes Mechanism of Ketamine-Induced Disinhibition. Nat. Methods 15, 823–831. doi:10.1038/s41592-018-0142-8
Flytzanis, N. C., Bedbrook, C. N., Chiu, H., Engqvist, M. K. M., Xiao, C., Chan, K. Y., et al. (2014). Archaerhodopsin Variants with Enhanced Voltage-Sensitive Fluorescence in Mammalian and Caenorhabditis elegans Neurons. Nat. Commun. 5. doi:10.1038/ncomms5894
Gong, Y., Huang, C., Li, J. Z., Grewe, B. F., Zhang, Y., Eismann, S., et al. (2015). High-speed Recording of Neural Spikes in Awake Mice and Flies with a Fluorescent Voltage Sensor. Science 350, 1361–1366. doi:10.1126/science.aab0810
Gong, Y., Li, J. Z., and Schnitzer, M. J. (2013). Enhanced Archaerhodopsin Fluorescent Protein Voltage Indicators. PLoS One 8, e66959. doi:10.1371/journal.pone.0066959
Gong, Y., Wagner, M. J., Zhong Li, J., and Schnitzer, M. J. (2014). Imaging Neural Spiking in Brain Tissue Using FRET-Opsin Protein Voltage Sensors. Nat. Commun. 5, 3674. doi:10.1038/ncomms4674
Gradinaru, V., Zhang, F., Ramakrishnan, C., Mattis, J., Prakash, R., Diester, I., et al. (2010). Molecular and Cellular Approaches for Diversifying and Extending Optogenetics. Cell 141, 154–165. doi:10.1016/j.cell.2010.02.037
Grienberger, C., and Konnerth, A. (2012). Imaging Calcium in Neurons. Neuron 73, 862–885. doi:10.1016/j.neuron.2012.02.011
Han, X., and Boyden, E. S. (2007). Multiple-Color Optical Activation, Silencing, and Desynchronization of Neural Activity, with Single-Spike Temporal Resolution. PLoS One 2, e299. doi:10.1371/journal.pone.0000299
Herwig, L., Rice, A. J., Bedbrook, C. N., Zhang, R. K., Lignell, A., Cahn, J. K. B., et al. (2017). Directed Evolution of a Bright Near-Infrared Fluorescent Rhodopsin Using a Synthetic Chromophore. Cel. Chem. Biol. 24 (3), 415–425. doi:10.1016/j.chembiol.2017.02.008
Hochbaum, D. R., Zhao, Y., Farhi, S. L., Klapoetke, N., Werley, C. A., Kapoor, V., et al. (2014). All-optical Electrophysiology in Mammalian Neurons Using Engineered Microbial Rhodopsins. Nat. Methods. 11, 825–833. doi:10.1038/nmeth.3000
Hontani, Y., Ganapathy, S., Frehan, S., Kloz, M., de Grip, W. J., and Kennis, J. T. M. (2018). Strong pH-dependent Near-Infrared Fluorescence in a Microbial Rhodopsin Reconstituted with a Red-Shifting Retinal Analogue. J. Phys. Chem. Lett. 9, 6469–6474. doi:10.1021/acs.jpclett.8b02780
Hou, J. H., Venkatachalam, V., and Cohen, A. E. (2014). Temporal Dynamics of Microbial Rhodopsin Fluorescence Reports Absolute Membrane Voltage. Biophysical J. 106 (3), 639–648. doi:10.1016/j.bpj.2013.11.4493
Inagaki, S., Tsutsui, H., Suzuki, K., Agetsuma, M., Arai, Y., Jinno, Y., et al. (2017). Genetically Encoded Bioluminescent Voltage Indicator for Multi-Purpose Use in Wide Range of Bioimaging. Sci. Rep. 7, 42398. doi:10.1038/srep42398
Inoue, M., Takeuchi, A., Horigane, S.-i., Ohkura, M., Gengyo-Ando, K., Fujii, H., et al. (2015). Rational Design of a High-Affinity, Fast, Red Calcium Indicator R-CaMP2. Nat. Methods. 12, 64–70. doi:10.1038/nmeth.3185
Inoue, M., Takeuchi, A., Manita, S., Horigane, S.-i., Sakamoto, M., Kawakami, R., et al. (2019). Rational Engineering of XCaMPs, a Multicolor GECI Suite for In Vivo Imaging of Complex Brain Circuit Dynamics. Cell 177, 1346–1360. doi:10.1016/j.cell.2019.04.007
Jin, L., Han, Z., Platisa, J., Wooltorton, J. R. A., Cohen, L. B., and Pieribone, V. A. (2012). Single Action Potentials and Subthreshold Electrical Events Imaged in Neurons with a Fluorescent Protein Voltage Probe. Neuron 75, 779–785. doi:10.1016/j.neuron.2012.06.040
Kannan, M., Vasan, G., Huang, C., Haziza, S., Li, J. Z., Inan, H., et al. (2018). Fast, In Vivo Voltage Imaging Using a Red Fluorescent Indicator. Nat. Methods. 15, 1108–1116. doi:10.1038/s41592-018-0188-7
Kojima, K., Kurihara, R., Sakamoto, M., Takanashi, T., Kuramochi, H., Zhang, X. M., et al. (2020). Comparative Studies of the Fluorescence Properties of Microbial Rhodopsins: Spontaneous Emission versus Photointermediate Fluorescence. J. Phys. Chem. B. 124, 7361–7367. doi:10.1021/acs.jpcb.0c06560
Kralj, J. M., Douglass, A. D., Hochbaum, D. R., Maclaurin, D., and Cohen, A. E. (2011a). Optical Recording of Action Potentials in Mammalian Neurons Using a Microbial Rhodopsin. Nat. Methods. 9, 90–95. doi:10.1038/nmeth.1782
Kralj, J. M., Hochbaum, D. R., Douglass, A. D., and Cohen, A. E. (2011b). Electrical Spiking inEscherichia coliProbed with a Fluorescent Voltage-Indicating Protein. Science 333, 345–348. doi:10.1126/science.1204763
Kwon, T., Sakamoto, M., Peterka, D. S., and Yuste, R. (2017). Attenuation of Synaptic Potentials in Dendritic Spines. Cel. Rep. 20, 1100–1110. doi:10.1016/j.celrep.2017.07.012
Liu, S., Lin, C., Xu, Y., Luo, H., Peng, L., Zeng, X., et al. (2021). A Far-Red Hybrid Voltage Indicator Enabled by Bioorthogonal Engineering of Rhodopsin on Live Neurons. Nat. Chem. 13, 472–479. doi:10.1038/s41557-021-00641-1
Lou, S., Adam, Y., Weinstein, E. N., Williams, E., Williams, K., Parot, V., et al. (2016). Genetically Targeted All-Optical Electrophysiology with a Transgenic Cre-dependent Optopatch Mouse. J. Neurosci. 36, 11059–11073. doi:10.1523/JNEUROSCI.1582-16.2016
Maclaurin, D., Venkatachalam, V., Lee, H., and Cohen, A. E. (2013). Mechanism of Voltage-Sensitive Fluorescence in a Microbial Rhodopsin. Proc. Natl. Acad. Sci. 110, 5939–5944. doi:10.1073/pnas.1215595110
Marshall, J. D., Li, J. Z., Zhang, Y., Gong, Y., St-Pierre, F., Lin, M. Z., et al. (2016). Cell-Type-Specific Optical Recording of Membrane Voltage Dynamics in Freely Moving Mice. Cell 167, 1650–1662. doi:10.1016/j.cell.2016.11.021
Nakai, J., Ohkura, M., and Imoto, K. (2001). A High Signal-To-Noise Ca2+ Probe Composed of a Single green Fluorescent Protein. Nat. Biotechnol. 19, 137–141. doi:10.1038/84397
Nakamura, T., Takeuchi, S., Shibata, M., Demura, M., Kandori, H., and Tahara, T. (2008). Ultrafast Pump−Probe Study of the Primary Photoreaction Process in Pharaonis Halorhodopsin: Halide Ion Dependence and Isomerization Dynamics. J. Phys. Chem. B. 112, 12795–12800. doi:10.1021/jp803282s
Neher, E. (1998). Usefulness and Limitations of Linear Approximations to the Understanding of Ca++ Signals. Cell Calcium. 24, 345–357. doi:10.1016/S0143-4160(98)90058-6
Nguyen, C., Upadhyay, H., Murphy, M., Borja, G., Rozsahegyi, E. J., Barnett, A., et al. (2019). Simultaneous Voltage and Calcium Imaging and Optogenetic Stimulation with High Sensitivity and a Wide Field of View. Biomed. Opt. Express. 10, 789. doi:10.1364/BOE.10.000789
Obien, M. E. J., Deligkaris, K., Bullmann, T., Bakkum, D. J., and Frey, U. (2014). Revealing Neuronal Function through Microelectrode Array Recordings. Front. Neurosci. 8, 423. doi:10.3389/fnins.2014.00423
Ohkura, M., Sasaki, T., Sadakari, J., Gengyo-Ando, K., Kagawa-Nagamura, Y., Kobayashi, C., et al. (2012). Genetically Encoded Green Fluorescent Ca2+ Indicators with Improved Detectability for Neuronal Ca2+ Signals. PLoS One. 7, e51286–10. doi:10.1371/journal.pone.0051286
Ohtani, H., Itoh, H., and Shinmura, T. (1992). Time-resolved Fluorometry of Purple Membrane of Halobacterium Halobium O640 and an O-like Red-Shifted Intermediate Q. FEBS Lett. 305, 6–8. doi:10.1016/0014-5793(92)80643-u
Ota, K., Oisi, Y., Suzuki, T., Ikeda, M., Ito, Y., Ito, T., et al. (2021). Fast, Cell-Resolution, Contiguous-wide Two-Photon Imaging to Reveal Functional Network Architectures across Multi-Modal Cortical Areas. Neuron 109, 1810–1824. doi:10.1016/j.neuron.2021.03.032
Peterka, D. S., Takahashi, H., and Yuste, R. (2011). Imaging Voltage in Neurons. Neuron 69, 9–21. doi:10.1016/j.neuron.2010.12.010
Piao, H. H., Rajakumar, D., Kang, B. E., Kim, E. H., and Baker, B. J. (2015). Combinatorial Mutagenesis of the Voltage-Sensing Domain Enables the Optical Resolution of Action Potentials Firing at 60 Hz by a Genetically Encoded Fluorescent Sensor of Membrane Potential. J. Neurosci. 35, 372–385. doi:10.1523/JNEUROSCI.3008-14.2015
Piatkevich, K. D., Bensussen, S., Tseng, H.-A., Shroff, S. N., Lopez-Huerta, V. G., Park, D., et al. (2019). Population Imaging of Neural Activity in Awake Behaving Mice. Nature 574, 413–417. doi:10.1038/s41586-019-1641-1
Piatkevich, K. D., Jung, E. E., Straub, C., Linghu, C., Park, D., Suk, H.-J., et al. (2018). A Robotic Multidimensional Directed Evolution Approach Applied to Fluorescent Voltage Reporters. Nat. Chem. Biol. 14, 352–360. doi:10.1038/s41589-018-0004-9
Sineshchekov, O. A., Govorunova, E. G., Wang, J., and Spudich, J. L. (2012). Enhancement of the Long-Wavelength Sensitivity of Optogenetic Microbial Rhodopsins by 3,4-dehydroretinal. Biochemistry 51, 4499–4506. doi:10.1021/bi2018859
Smetters, D., Majewska, A., and Yuste, R. (1999). Detecting Action Potentials in Neuronal Populations with Calcium Imaging. Methods 18, 215–221. doi:10.1006/meth.1999.0774
Sofroniew, N. J., Flickinger, D., King, J., and Svoboda, K. (2016). A Large Field of View Two-Photon Mesoscope with Subcellular Resolution for In Vivo Imaging. Elife 5, 1–20. doi:10.7554/eLife.14472
St-Pierre, F., Marshall, J. D., Yang, Y., Gong, Y., Schnitzer, M. J., and Lin, M. Z. (2014). High-fidelity Optical Reporting of Neuronal Electrical Activity with an Ultrafast Fluorescent Voltage Sensor. Nat. Neurosci. 17, 884–889. doi:10.1038/nn.3709
Stirman, J. N., Smith, I. T., Kudenov, M. W., and Smith, S. L. (2016). Wide Field-Of-View, Multi-Region, Two-Photon Imaging of Neuronal Activity in the Mammalian Brain. Nat. Biotechnol. 34, 857–862. doi:10.1038/nbt.3594
Storace, D., Sepehri Rad, M., Kang, B., Cohen, L. B., Hughes, T., and Baker, B. J. (2016). Toward Better Genetically Encoded Sensors of Membrane Potential. Trends Neurosciences 39, 277–289. doi:10.1016/j.tins.2016.02.005
Tian, L., Hires, S. A., Mao, T., Huber, D., Chiappe, M. E., Chalasani, S. H., et al. (2009). Imaging Neural Activity in Worms, Flies and Mice with Improved GCaMP Calcium Indicators. Nat. Methods 6, 875–881. doi:10.1038/nmeth.1398
Tsutsui, H., Jinno, Y., Tomita, A., Niino, Y., Yamada, Y., Mikoshiba, K., et al. (2013). Improved Detection of Electrical Activity with a Voltage Probe Based on a Voltage-Sensing Phosphatase. J. Physiol. 591, 4427–4437. doi:10.1113/jphysiol.2013.257048
Venkatachalam, V., Brinks, D., Maclaurin, D., Hochbaum, D., Kralj, J., and Cohen, A. E. (2014). Flash Memory: Photochemical Imprinting of Neuronal Action Potentials onto a Microbial Rhodopsin. J. Am. Chem. Soc. 136, 2529–2537. doi:10.1021/ja411338t
Villette, V., Chavarha, M., Dimov, I. K., Bradley, J., Pradhan, L., Mathieu, B., et al. (2019). Ultrafast Two-Photon Imaging of a High-Gain Voltage Indicator in Awake Behaving Mice. Cell 179, 1590–1608. doi:10.1016/j.cell.2019.11.004
Werley, C. A., Boccardo, S., Rigamonti, A., Hansson, E. M., and Cohen, A. E. (2020). Multiplexed Optical Sensors in Arrayed Islands of Cells for Multimodal Recordings of Cellular Physiology. Nat. Commun. 11, 3881. doi:10.1038/s41467-020-17607-5
Xie, M. E., Adam, Y., Fan, L. Z., Böhm, U. L., Kinsella, I., Zhou, D., et al. (2021). High-fidelity Estimates of Spikes and Subthreshold Waveforms from 1-photon Voltage Imaging In Vivo. Cel Rep. 35, 108954. doi:10.1016/j.celrep.2021.108954
Yuste, R., and Katz, L. C. (1991). Control of Postsynaptic Ca2+ Influx in Developing Neocortex by Excitatory and Inhibitory Neurotransmitters. Neuron 6, 333–344. doi:10.1016/0896-6273(91)90243-S
Zhang, H., Reichert, E., and Cohen, A. E. (2016). Optical Electrophysiology for Probing Function and Pharmacology of Voltage-Gated Ion Channels. Elife 5, 1–20. doi:10.7554/eLife.15202
Zhao, Y., Araki, S., Wu, J., Teramoto, T., Chang, Y.-F., Nakano, M., et al. (2011). An Expanded Palette of Genetically Encoded Ca2+ Indicators. Science 333, 1888–1891. doi:10.1126/science.1208592
Ziv, Y., Burns, L. D., Cocker, E. D., Hamel, E. O., Ghosh, K. K., Kitch, L. J., et al. (2013). Long-term Dynamics of CA1 Hippocampal Place Codes. Nat. Neurosci. 16, 264–266. doi:10.1038/nn.3329
Keywords: voltage imaging, microbial rhodopsins, photocycle, FRET (förster resonance energy transfer), optogenetics, in vivo imaging
Citation: Zhang XM, Yokoyama T and Sakamoto M (2021) Imaging Voltage with Microbial Rhodopsins. Front. Mol. Biosci. 8:738829. doi: 10.3389/fmolb.2021.738829
Received: 09 July 2021; Accepted: 11 August 2021;
Published: 25 August 2021.
Edited by:
Leonid S. Brown, University of Guelph, CanadaReviewed by:
Joel Kralj, University of Colorado Boulder, United StatesAdam E. Cohen, Harvard University, United States
Copyright © 2021 Zhang, Yokoyama and Sakamoto. This is an open-access article distributed under the terms of the Creative Commons Attribution License (CC BY). The use, distribution or reproduction in other forums is permitted, provided the original author(s) and the copyright owner(s) are credited and that the original publication in this journal is cited, in accordance with accepted academic practice. No use, distribution or reproduction is permitted which does not comply with these terms.
*Correspondence: Masayuki Sakamoto, c2FrYW1vdG8ubWFzYXl1a2kuMmVAa3lvdG8tdS5hYy5qcA==