- Tumor Etiology and Screening Department of Cancer Institute and General Surgery, The First Affiliated Hospital of China Medical University, Key Laboratory of Cancer Etiology and Prevention, China Medical University, Liaoning Provincial Education Department, Shenyang, China
Background: Here we carried out a panoramic analysis of the expression and prognosis of HSP110, HSP90, HSP70, and HSP60 families in 33 types of cancer, with the aim of deepening the systematic understanding of heat shock proteins (HSPs) in cancer.
Materials and Methods: Next-generation sequencing data of multiple tumors were downloaded from TCGA, CCLE and Oncomine databases. RStudio 3.6.1 was used to analyze HSP110, HSP90, HSP70 and HSP60 families based on their expression in 33 types of cancer. The validations in vivo (stomach adenocarcinoma and colon adenocarcinoma tissues) were performed by qRT-PCR.
Results: HSPs were differentially expressed in different cancers. The results revealed mainly positive correlations among the expressions of HSPs in different cancers. Expressions of HSP family members were generally associated with poor prognosis in respiratory, digestive, urinary and reproductive system tumors and associated with good prognosis in cholangiocarcinoma, pheochromocytoma and paraganglioma. TCGA mutation analysis showed that HSP gene mutation rate in cancers was 0–23%. CCLE mutation analysis indicated that HSP gene mutation rate in 828 cell lines from 15 tumors was 0–17%. CNV analysis revealed that HSPs have different degrees of gene amplifications and deletions in cancers. Gene mutations of 15 HSPs influenced their protein expressions in different cancers. Copy number amplifications and deletions of 22 HSPs also impacted protein expression levels in pan-cancer. HSP gene mutation was generally a poor prognosis factor in cancers, except for uterine corpus endometrial carcinoma. CNVs in 14 HSPs showed varying influences on survival status in different cancers. HSPs may be involved in the activation and inhibition of multiple cancer-related pathways. HSP expressions were closely correlated with 22 immune cell infiltrations in different cancers. The qRT-PCR validation results in vivo showed that HSPA2 was down-regulated in stomach adenocarcinoma and colon adenocarcinoma; HSPA7 and HSPA1A also were down-regulated in colon adenocarcinoma. HSPA2-HSPA7 (r = 0.031, p = 0.009) and HSPA1A-HSPA7 (r = 0.516, p < 0.001) were positive correlation in colon adenocarcinoma.
Conclusion: These analysis and validation results show that HSP families play an important role in the occurrence and development of various tumors and are potential tumor diagnostic and prognostic biomarkers as well as anti-cancer therapeutic targets.
Introduction
The global cancer incidence and mortality rates have risen rapidly in the 21st century, and cancer has become the main cause of death in many countries around the world (Bray et al., 2018). The main causes of the high mortality rate of cancer lie in the unknown pathogenic mechanisms and the lack of effective treatment protocols. Therefore, exploring the pathogenic mechanism of cancer is critical to develop strategies for cancer treatment.
Heat shock proteins (HSPs) are a class of molecular chaperones that help protein folding and maintain the normal structures and functions of proteins (Wu et al., 2017). HSPs protect cells from physical and chemical stimuli and stress, such as ischemia, hypoxia, high temperature, metabolic factors, alcohol and drugs, thus maintaining cell homeostasis (Tsukahara et al., 2000; Macario and Conway de Macario, 2007). On the basis of their molecular weight, HSPs are divided into different families, including the HSP110(HSPH) family, HSP90 family, HSP70 (HSPA) family, HSP60 family, HSP40 (DNAJ) family and small heat shock proteins (Kampinga et al., 2009; Wu et al., 2017). Some research has suggested that different families not only independently regulate the structures and functions of proteins, but also collaborate with each other. For instance, protein folding and degradation are synergistically regulated by the HSP70 and HSP90 families (Kravats et al., 2018; Bhattacharya et al., 2020). The HSP60 precursor is transformed into mature HSP60 complexes under the cooperating of HSP70 and HSP10 (Böttinger et al., 2015). HSP family functions and jointly role was critical to sustain homeostasis.
Multiple studies have indicated that HSPs are involved in the occurrence and development of tumors. HSPs modulate cell proliferation, angiogenesis, and the migration, invasion and metastasis of tumor cells, as well as the resistance of tumors to anti-cancer drugs (Xu et al., 2006; Wong et al., 2008; Tsai et al., 2009; Lin et al., 2010; Gibert et al., 2013; Yun et al., 2019). Several studies have also suggested HSPs as potential diagnostic and prognostic biomarkers as well as therapeutic targets of tumors (Xu et al., 2006; Wong et al., 2008; Tsai et al., 2009; Lin et al., 2010; Gibert et al., 2013; Yun et al., 2019). In addition, mutations in HSPs may have an influence on cancer risk and prognosis (Medhi et al., 2013; He et al., 2014; Zou et al., 2020). In conclusion, HSPs play an important role in tumor progression when the body is in an unbalanced state of tumorigenesis.
At present, no study has performed a panoramic analysis of HSPs in 33 types of cancer. In this study, we used The Cancer Genome Atlas (TCGA), Cancer Cell Line Encyclopedia (CCLE), Oncomine and The Human Protein Atlas (THPA) databases to analyze the expressions, mutations, copy number variations (CNVs) and prognosis profiles of HSP110, HSP90, HSP70 and HSP60 families in cancer. We investigated the potential correlation of HSP expressions with cancer-related pathways, immune cell infiltration, and prognosis in pan-cancer, as well as the relationship between HSP mutations and CNVs with HSP expressions. Stomach adenocarcinoma and colon adenocarcinoma tissues were used to validate the differentially expressed of HSPs in vivo by quantitative real-time polymerase chain reaction (qRT-PCR). Our study aims include analyzing HSP profiles in different cancers, deepening HSP systematic recognitions, finding potential diagnostic and prognostic biomarkers as well as anti-cancer therapeutic targets and providing important clues for further mechanism research.
Materials and Methods
Data Collection at Tissue, Protein and Cell Levels
The HSP expressions, mutations and CNV data in 33 types of cancer were downloaded from TCGA database (http://cancergenome.nih.gov/). Clinical information, such as survival status and survival period, was downloaded from UCSC XENA (https://xenabrowser.net/). The details of 33 types of cancer collected from TCGA were as follows: ACC, Adrenocortical carcinoma; BLCA, Bladder Urothelial Carcinoma; BRCA, Breast invasive carcinoma; CESC, Cervical squamous cell carcinoma and endocervical adenocarcinoma; CHOL, Cholangiocarcinoma; COAD, Colon adenocarcinoma; DLBC, Lymphoid Neoplasm Diffuse Large B-cell Lymphoma; ESCA, Esophageal carcinoma; GBM, Glioblastoma multiforme; HNSC, Head and Neck squamous cell carcinoma; KICH, Kidney Chromophobe; KIRC, Kidney renal clear cell carcinoma; KIRP, Kidney renal papillary cell carcinoma; LAML, Acute Myeloid Leukemia; LGG, Brain Lower Grade Glioma; LIHC, Liver hepatocellular carcinoma; LUAD, Lung adenocarcinoma; LUSC, Lung squamous cell carcinoma; MESO, Mesothelioma; OV, Ovarian serous cystadenocarcinoma; PAAD, Pancreatic adenocarcinoma; PCPG, Pheochromocytoma, and Paraganglioma; PRAD, Prostate adenocarcinoma; READ, Rectum adenocarcinoma; SARC, Sarcoma; SKCM, Skin Cutaneous Melanoma; STAD, Stomach adenocarcinoma; TGCT, Testicular Germ Cell Tumors; THCA, Thyroid carcinoma; THYM, Thymoma; UCEC, Uterine Corpus Endometrial Carcinoma; UCS, Uterine Carcinosarcoma; UVM, Uveal Melanoma. In addition, expression data of HSP mRNA levels in different cancers were downloaded from the Oncomine database (Rhodes et al., 2007). Immunohistochemistry data of HSPs in 16 tumors were collected from THPA database (https://www.proteinatlas.org/). The HSP expressions and mutations data of 828 cell lines from 15 tumors were downloaded from the CCLE database (https://portals.broadinstitute.org/ccle) (Ghandi et al., 2019).
Comprehensive Analysis of HSP Expressions in Pan-Cancer
Analysis of HSP mRNA Levels in Pan-Cancer Tissues and Cells
Deseq2 R package was used to analyze expression data in TCGA to identify differentially expressed HSP family members in pan-cancer tissues. p < 0.05 was considered statistically significant. The Oncomine database was applied to verify the differentially expressed HSP mRNAs in different cancer tissues. The cut-off criteria were p < 0.05, |log2 (fold change) | ≥ 2 and top 10% gene rank.
We further examined the expression profiles of HSPs in different tumor cell lines using the CCLE database. Kruskal–Wallis rank test was used to compare expressions of HSPs in pan-cancer cell lines. p < 0.05 was considered statistically significant.
Analysis of HSP Protein Levels in Pan-Cancer Tissues
Immunohistochemistry data of HSPs were collected from THPA database to evaluate their expression profiles at protein level in pan-cancer. The cancer types were as follows: glioma, lung cancer, colorectal cancer, testis cancer, renal cancer, head and neck cancer, stomach cancer, pancreatic cancer, lymphoma, ovarian cancer, skin cancer, breast cancer, liver cancer, endometrial cancer, melanoma, thyroid cancer.
Correlation Analysis Between HSP Expressions and Cancer-Related Pathways
In this study, gene set variation analysis (GSVA), an algorithm of starting from gene expression and multiple pathway information, was used to estimate the changes in pathway activity of different samples in an unsupervised manner (Hänzelmann et al., 2013; Kim et al., 2018; Zhang et al., 2020a). Univariate Pearson correlation analysis was used to calculate and determine the correlation of HSPs expression with the activation or inhibition of pathways (Zhang et al., 2020a). | r | ≥ 0.2 and p < 0.05 were regarded as cut-off criteria and Cytoscape 3.7.1 was applied for visualization. Further, the same method was used to analyze the correlation of HSPs expression with pathway activation in single common types of cancer, including stomach adenocarcinoma, lung adenocarcinoma, lung squamous cell carcinoma, colon adenocarcinoma, liver hepatocellular carcinoma and rectum adenocarcinoma.
Correlation Analysis Between HSP Expressions and Immune Cell Infiltration in Pan-Cancer
CIBERSORT and Spearman correlation analysis was performed to evaluate the relationships of HSP family expression with infiltration of 22 immune cell types (Diboun et al., 2006; Newman et al., 2015; Miao et al., 2020). The cut-off value was |r | ≥ 0.3 and p < 0.05. RStudio 3.6.1 was used for data analysis.
Correlation and Interaction of HSP Expressions in Pan-Cancer
HSP family members often cooperate to exert their cellular functions (Böttinger et al., 2015; Genest et al., 2019). We determined Pearson’s correlation coefficient of HSP expressions in different cancers using TCGA data. Ggcorrplot and ggthemes R package were used to perform detailed analysis and visualization. Search Tool for the Retrieval of Interacting Genes (STRING, https://string-db.org/) was applied to predict the network to explore the potential interaction relationships of HSP family members. Cytoscape 3.7.2 was used to perform analysis and visualization. Degree score was determined to evaluate interaction strength among HSPs.
Correlation Analysis Between HSP Family Expression and Prognosis in Pan-Cancer
We analyzed the correlation of HSPs expressions with prognosis using gene expression and clinical information in TCGA. Patients were divided into two groups according to the median expression of HSPs and log-rank test was performed. p < 0.05 was considered to be statistically significant.
Analysis of HSP Gene Mutations and CNVs in Pan-Cancer Tissues and Cells
HSP Gene Mutations in Pan-Cancer Tissues and Cells
Mutation data were downloaded from TCGA and CCLE databases. RStudio 3.6.1 was used to calculate the gene mutation frequency of HSP molecules in tissues and cell lines. The mutation frequency was defined as mutation proportion in each type of cancer.
HSP Gene CNVs in Pan-Cancer Tissues
CNV data were downloaded from TCGA database. RStudio 3.6.1 was used to calculate the copy number amplification and deletion frequency in tissues. The CNV frequency was defined as CNV proportion in each type of cancer.
Correlation Analysis Between HSP Gene Mutations and CNVs With Expressions in Pan-Cancer
Mann–Whitney U test was applied to statistically identify the influence of gene mutations and CNVs on HSP expressions in pan-cancer. p < 0.05 was considered to be statistically significant. All analyses were performed by RStudio 3.6.1.
Correlation Analysis Between HSP Gene Mutations and CNVs With Prognosis in Pan-Cancer
Patients were divided into two groups according to the median variation frequency of HSPs to identify the influence of mutations and CNVs on overall survival in pan-cancer. Log-rank test was performed by RStudio 3.6.1. p < 0.05 was considered to be statistically significant.
qRT-PCR Validations In Vivo
In vivo validation, we employed 53 pairs stomach adenocarcinoma tissues and 42 pairs colon adenocarcinoma tissues collected from the First Affiliated Hospital of China medical university. Our research was implemented according to the Declaration of Helsinki and supported by the research ethics committee of the First Affiliated Hospital of China medical university. The written informed consents of our study were signed by all patients before the samples were collected. qRT-PCR was performed by SuperReal PreMix Plus (SYBR Green, TIANGEN). The measurements were normalized using the β-actin. The primers sequences were listed in Supplementary Table S1. SPSSv25.0 (IBM, SPSS, and Chicago, IL, United States) and GraphPad Prism V8.0 (GraphPad software, United States) were utilized to perform data analysis, and 2−ΔCt was used to calculate relative expression. The differential expression profiles were assessed by Student’s t-test for normally distributed data while rank sum test for skewed distribution data. Spearman correlation analysis was used to analyze the association among genes. A Chi-square test was utilized to analyze the association of HSPs expression with clinicopathological parameters. p < 0.05 has statistically significant.
Results
HSP Expression Profiles in Pan-Cancer
HSP mRNA Expressions in Pan-Cancer
On the basis of a literature review, 22 HSPs among HSP110, HSP90, HSP70 and HSP60 families were selected for analysis (Table 1) (Kampinga et al., 2009). The sample size selected per cancer type was listed in Supplementary Table S2. All results were obtained from specific TCGA platform instead of other databases in TCGA website. We examined the mRNA expression of these 22 HSPs in cancer and non-tumor tissues in TCGA and found that mRNA levels of 10 HSPs were differentially expressed in 33 types of cancer (p < 0.05) (Figure 1A). HSPA2 mRNA was down-regulated in stomach adenocarcinoma, colon adenocarcinoma, bladder urothelial carcinoma, kidney renal clear cell carcinoma, kidney renal papillary cell carcinoma and kidney chromophobe (Figure 1B). The mRNA expression level of HSPA7 was decreased in colon adenocarcinoma but increased in lung adenocarcinoma, kidney renal clear cell carcinoma and kidney renal papillary cell carcinoma. HSPA1A mRNA was down-regulated in colon adenocarcinoma. HSPA6 mRNA was up-regulated in breast cancer, kidney renal clear cell carcinoma and kidney renal papillary cell carcinoma. HSPA4L mRNA expression was increased in lung squamous cell carcinoma and breast cancer and decreased in prostate adenocarcinoma. HSPA1L mRNA was down-regulated in uterine corpus endometrial carcinoma. HSPA12A mRNA was down-regulated in bladder urothelial carcinoma, breast cancer, uterine corpus endometrial carcinoma and kidney renal papillary cell carcinoma. HSPA12B mRNA was down-regulated in lung squamous cell carcinoma, lung adenocarcinoma, breast cancer, uterine corpus endometrial carcinoma and kidney renal papillary cell carcinoma. The mRNA expression levels of TRAP1 and HSPD1 were increased in lung squamous cell carcinoma.
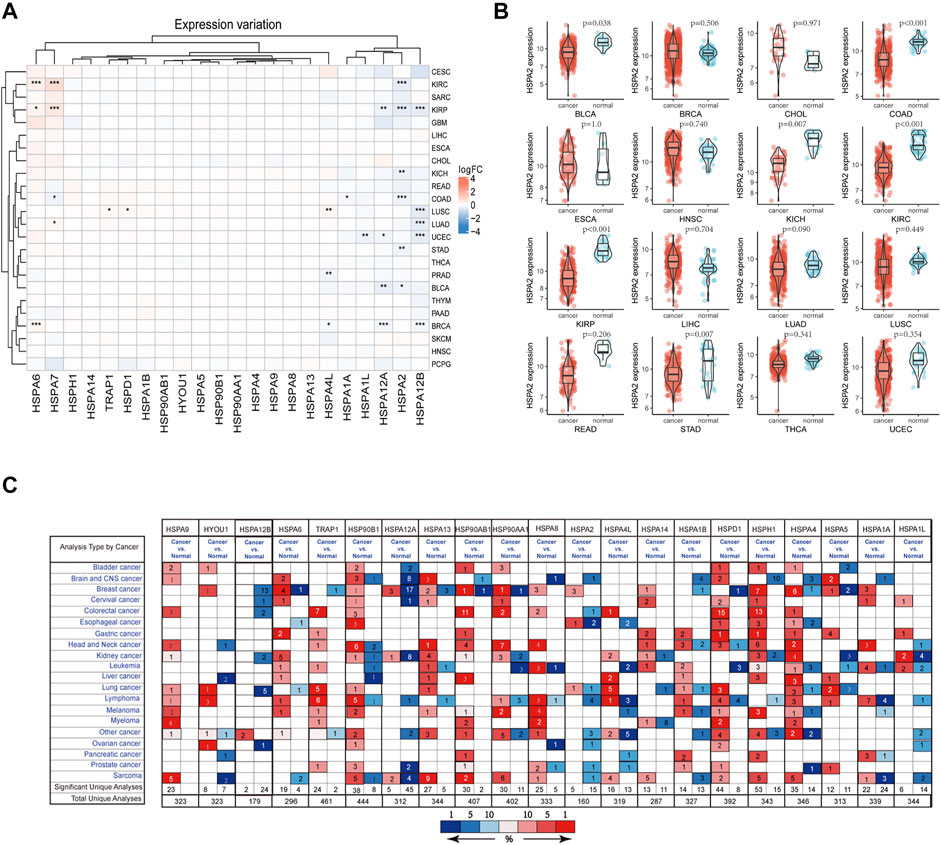
FIGURE 1. HSP expressions at mRNA level. (A) The differentially expressed of heat shock proteins in pan-cancer. The color in heat map represents the log2 fold change value between cancer and normal. Red color represents up-regulated and blue color represents down-regulated. *p < 0.05, **p < 0.01, ***p < 0.001. (B) HSPA2 expression in 16 types of cancers between cancer and normal tissues. (C) Expression profile of HSPs in different human cancer from Oncomine database. Red color represents up-regulated and blue color represents down-regulated. Cell color is determined by the best gene rank percentile for the analyses within the cell. HSP, heat shock protein.
We used the Oncomine database to validate the above findings. The results suggested that HSPs show varying degrees of differential expression in multiple tumors (Figure 1C).
HSP Protein Expressions in Pan-Cancer
We next used the THPA site to examine the expression profiles of HSP proteins in 16 tumors. Immunohistochemistry results indicated that HSP proteins showed different expression intensities in 16 tumors. The expression intensities of HSPs in 10 common tumors including lung cancer, liver cancer, colorectal cancer, pancreatic cancer, renal cancer, prostate cancer, breast cancer, endometrial cancer, ovarian cancer and melanoma were shown in Figure 2A. For example, HSP90B1, HSPA9, TRAP1, HSPH1 and HSPD1 showed high levels of immunostaining in 10 common tumors. HSPA6 showed moderate expression in lung cancer, breast cancer, endometrial cancer, ovarian cancer and melanoma and negative expression in endometrial cancer, renal cancer and liver cancer. HSPA8 showed moderate expression in other cancers. The immunostaining of HSPA9 is shown in Figure 2B.
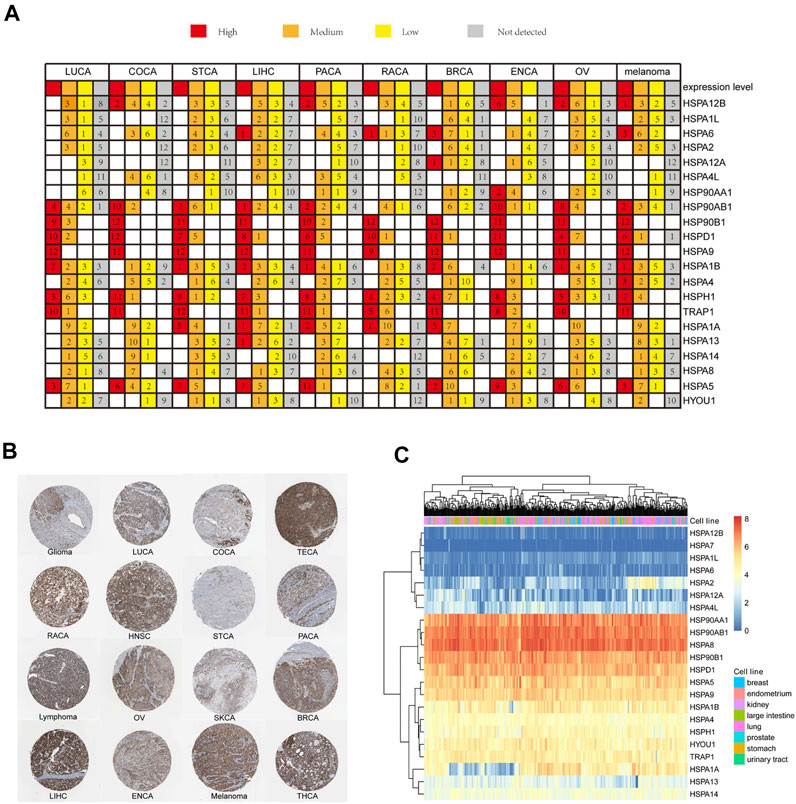
FIGURE 2. HSP expressions at protein and cell level. (A) HSP family members protein expression in 10 types of cancers. Each gene expression in one cancer was divided into four groups of high expression (red color), medium expression (orange color), low expression (yellow color) and not detected (grey color). (B) The immunohischemistry staining results of HSPA9 protein in 16 types of cancers in The Human Protein Atlas database. (C) Expression profile of HSPs family in cell lines in the CCLE database. Expression increased gradually from blue color to red color. CCLE, Cancer Cell Line Encyclopedia; LUCA, lung cancer; COCA, colorectal cancer; TECA, testis cancer; RACA, renal cancer; HNSC, head and neck cancer; STCA, stomach cancer; PACA; pancreatic cancer; OV, ovarian cancer; SKCA, skin cancer; BRCA, breast cancer; LIHC, liver cancer; ENCA, endometrial cancer; THCA, thyroid cancer.
It was found that different HSPs could be differentially expressed in 11 of 33 types of tumors at the mRNA level and 10 of 16 types of cancers at the protein level. Further, the correlation between mRNA and protein expression pattern of HSPs were concluded. The result indicated that the expression trends of partial HSPs were consistent at mRNA level and protein level in 5 types of cancer, such as TRAP1, HSPD1 and HSPA12B in lung cancer; HSPA6, HSPA12A, HSPA4L and HSPA12B in breast cancer; HSPA1L in endometrial cancer; HSPA2 and HSPA1A in colorectal cancer; HSPA12A, HSPA12B and HSPA2 in renal carcinoma (Table 2).
HSP Expressions in Pan-Cancer Cell Lines
CCLE data revealed different expression levels of HSPs in 425 cell lines of 8 tumors, including breast cancer, endometrium cancer, kidney cancer, large intestine cancer, lung cancer, prostate cancer, stomach cancer and urinary tract cancer (Figure 2C). The following HSPs were expressed in cell lines in all eight tumors: HSP90AA1, HSP90AB1, HSPA8, HSP90B1, HSPD1, HSPA5, HSPA9, HSPA1B, HSPA4, HSPH1, HYOU1 and TRAP1. HSPA2 and HSPA1A were mainly expressed in lung cancer and breast cancer cell lines. HSPA12A was mainly expressed in lung cancer and kidney cancer cell lines. HSPA13 was mainly expressed in lung cancer, stomach cancer and endometrium cancer cell lines. HSPA14 and HSPA4L were mainly expressed in lung cancer and large intestine cancer cell lines. HSPA7, HSPA1L, HSPA12B and HSPA6 were rarely expressed in the cell lines examined.
Relationships of HSP Expressions and Interactions in Pan-Cancer
Previous studies showed that HSPs cooperate with each other to exert critical cellular functions. Therefore, we used TCGA data to analyze the correlations of HSPs expressions in 9 tumors including stomach adenocarcinoma, breast cancer, lung adenocarcinoma, lung squamous cell carcinoma, bladder urothelial carcinoma, colon adenocarcinoma, uterine corpus endometrial carcinoma, kidney renal clear cell carcinoma and kidney renal papillary cell carcinoma. The results revealed mostly positive correlations among the expressions of HSP families in different cancers (Figure 3A), such as HSPA2-HSPA7 (r = 0.4, p < 0.001) and HSPA1A-HSPA7 (r = 0.397, p < 0.001) in colon adenocarcinoma.
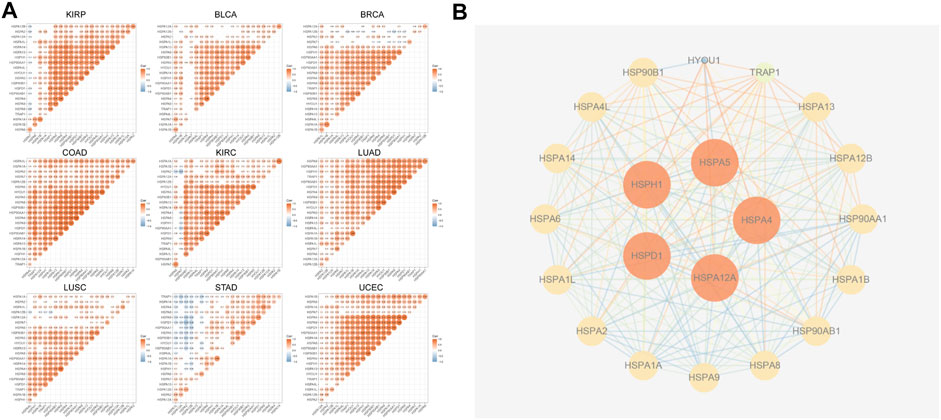
FIGURE 3. The correlation analysis and protein-protein interactions among HSPs. (A) The correlations among HSP expressions. Red color represents positive correlation and blue color represents negative color (p < 0.05). CCLE, Cancer Cell Line Encyclopedia. HSP, heat shock protein. (B) Protein-protein interaction network among HSP family. The higher the degree score, the larger the size.
We next used the STRING site to predict the potential PPI network of HSP families. The results showed that there were interactions among HSPs. HSPA4, HSPA5, HSPH1, HSPA12A and HSPD1 showed the highest degree score (Figure 3B).
Relationships Between HSP Expressions and Cancer-Related Pathways
The association of HSP expressions with cancer-related pathways was analyzed. The results demonstrated that HSP family proteins mainly participate in the fatty acid metabolism pathway, oxidative phosphorylation pathway, G2M checkpoint pathway, MTORC1 signaling pathway, mitotic spindle pathway, unfolded protein response pathway, protein secretion, reactive oxygen species pathway, E2F target pathway, MYC target pathway, UV response pathway and xenobiotic metabolism pathway (Figure 4A). We counted the numbers of pathways affected by each HSP; the results suggested that HSPA1L, HSPA12B, HSPA6, HSPA7 and HSPA12A may influence the activity of most cancer-related pathways and thus potently affect tumor development (Figure 4B). In addition, the numbers of activated pathways were more than inactivated pathways affected by HSPs, indicating a cancer promotion role of these genes (Figure 4B).
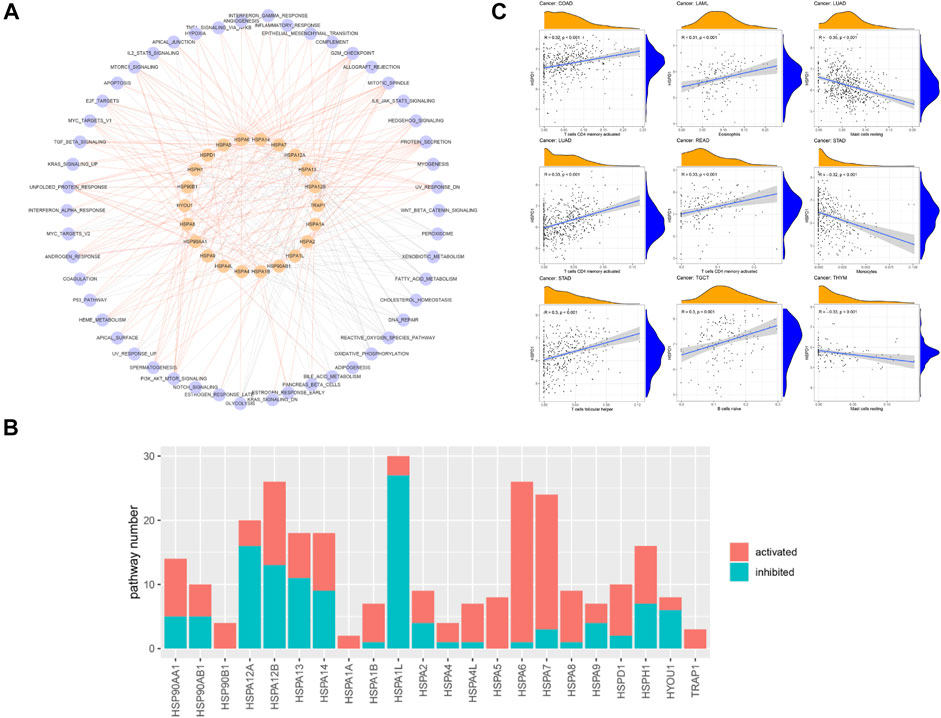
FIGURE 4. The association of HSP expressions with cancer-related pathway and immune cell infiltration. (A) Network displaying the correlation between HSP expressions and cancer-related pathways. Pink color represents HSPs and blue color represents pathways. The red edges represent activated pathways and grey edges represent inhibited pathways. (B) Number of activated and inhibited pathways of each heat shock protein. Pink color represents activated pathways activity and blue color represents inhibited pathways activity. (C) Association of HSPD1 with different immune cell infiltration across different cancer types. HSP, heat shock protein.
Further, we analyzed the correlation of HSPs expression with pathway activation in single common types of cancer, including stomach adenocarcinoma, lung adenocarcinoma, lung squamous cell carcinoma, colon adenocarcinoma, liver hepatocellular carcinoma and rectum adenocarcinoma. The result suggested that multiple HSPs were related to the change of pathway activation in different cancers (Supplementary Table S3). In addition, we found that most HSPs have consistent conclusions in the overall and individual analyses.
Relationships Between HSP Expressions and Immune Cell Infiltration in Pan-Cancer
Previous studies reported that HSPs participate in immune cell reactions (Todryk et al., 1950; Yamazaki et al., 2002). We next used Spearman correlation analysis to calculate the association of HSP expressions with infiltration of 22 immune cell types. HSP expressions were closely related to immune cell infiltration in the examined cancers (|r | ≥ 0.3, p < 0.05), except for uterine corpus endometrial carcinoma, cervical squamous cell carcinoma and endocervical adenocarcinoma, bladder urothelial carcinoma, lung squamous cell carcinoma and ovarian serous cystadenocarcinoma. The immune cell types included M1 macrophages, resting mast cells, M0 macrophages, M2 macrophages and CD4 memory activated T cells (Table 3 and Supplementary Table S4). For example, HSPD1 was positively associated with CD4 memory activated T cells and follicular helper T cells but negatively associated with monocytes in stomach adenocarcinoma, while HSPD1 was positively associated with CD4 memory activated T cells and negatively associated with resting mast cells in lung adenocarcinoma (Figure 4C).
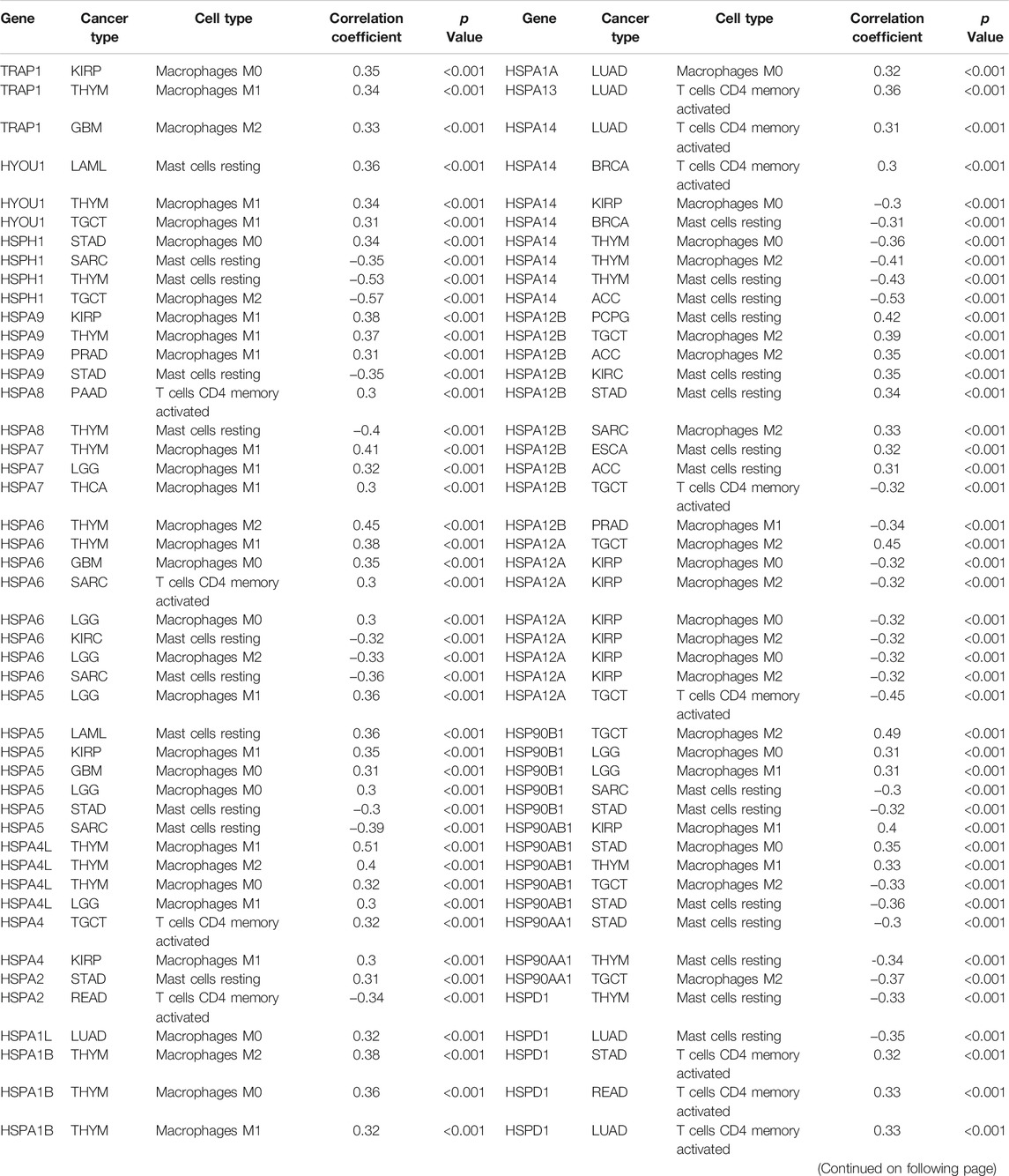
TABLE 3. The relationships between heat shock protein expressions and immune cell infiltration in pan-cancer.
Relationships Between HSP Expressions and Prognosis in Pan-Cancer
We analyzed the correlation of HSP expressions with prognosis using gene expression and clinical information in TCGA. All specimens were divided into two groups according to the median expression of HSPs, and log-rank test was performed to evaluate the correlation of expression with prognosis. The expressions of single HSP showed different effects on prognosis in 25 types of cancer, except for rectum adenocarcinoma, stomach adenocarcinoma, thymoma, prostate adenocarcinoma, pancreatic adenocarcinoma, ovarian serous cystadenocarcinoma and lymphoid neoplasm diffuse large B-cell lymphoma (Figure 5). For instance, HSPA2 was related to poor prognosis of lung adenocarcinoma and thymoma. The expression of HSPA7 hinted poor prognosis of kidney renal clear cell carcinoma, acute myeloid leukemia, brain lower grade glioma and glioblastoma multiforme, while it predicted good prognosis in skin cutaneous melanoma. HSPA1A was associated with poor prognosis of colon adenocarcinoma, liver hepatocellular carcinoma and adrenocortical carcinoma and good prognosis of pheochromocytoma and paraganglioma.
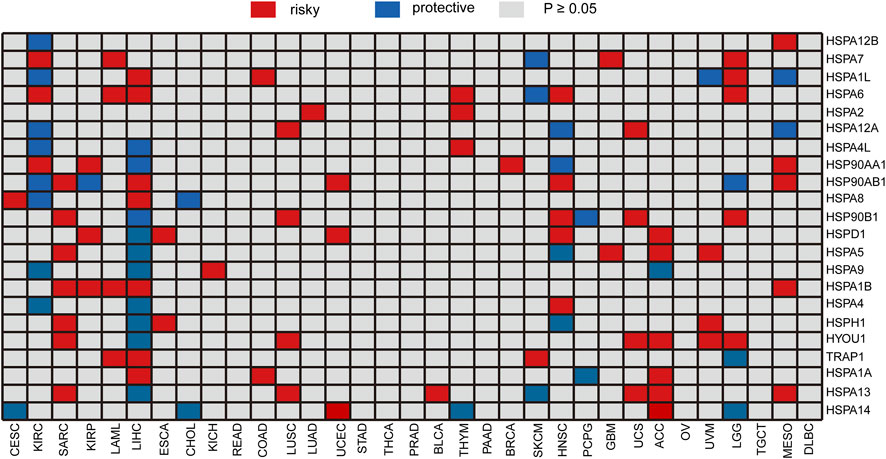
FIGURE 5. Heatmap of the relationship between individual HSP expression and overall survival. Red represents a worse prognosis, and blue represents a better prognosis. HSP, heat shock protein.
Mutation Profiles of HSPs in Pan-Cancer Tissues and Cell Lines
We next examined the mutation profiles of HSPs using TCGA data. The results showed that mutations of HSPs were mainly present in uterine corpus endometrial carcinoma, colon adenocarcinoma, stomach adenocarcinoma, rectum adenocarcinoma, lung squamous cell carcinoma and lung adenocarcinoma, with a mutation frequency of 0–23% (Figure 6A). A waterfall plot for the mutation details of HSPs in uterine corpus endometrial carcinoma is shown in Figure 6B. Furthermore, CCLE analysis indicated that the mutation frequency of HSPs in 828 cell lines of 15 tumors was 0–17% (Figure 6C).
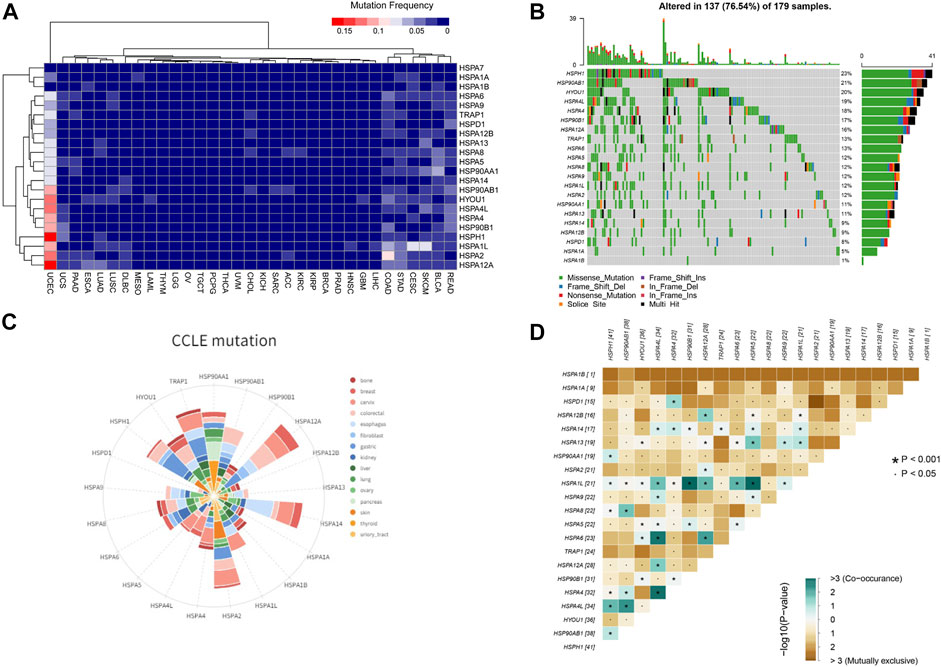
FIGURE 6. HSP mutations at tissue and cell level across different cancer types. (A) Mutation frequency of HSPs in tissues across different cancer types. (B) Oncoplot for HSPs in UCEC. HSPH1 has the most frequent mutation in UCEC. UCEC, uterine corpus endometrial carcinoma. (C) Mutation status of HSPs in different cancer cell lines from CCLE database. (D) Correlation among HSP mutations in UCEC. Blue color represents co-occurance mutation and brown color displayed mutually exclusive. The number represents sample sizes of mutation. UCEC, uterine corpus endometrial carcinoma. CCLE, Cancer Cell Line Encyclopedia. HSP, heat shock protein. UCEC, uterine corpus endometrial carcinoma.
Given that the HSPs often interact synergistically in regulating cellular functions, the mutation correlation among HSP genes were analyzed in uterine corpus endometrial carcinoma of the most common mutations. The results suggested that the mutation could be co-occurred or mutually exclusive among HSPs, but the co-occurrence mutation was more obvious (Figure 6D). The co-occurrence of mutations was frequently observed in HSPA1L-HSP90B1, HSPA1L-HSPA5, HSPA4L-HSPA4 and HSPA4L-HSPA6 pairs in uterine corpus endometrial carcinoma.
CNV analysis demonstrated that HSP family members showed different degrees of gene amplification and deletion in pan-cancer (Figure 7A). For instance, the gene amplification frequency of HSPA6 was 24% in bladder urothelial carcinoma and the frequency of HSP90AA1 gene deletion was 22% in cholangiocarcinoma.
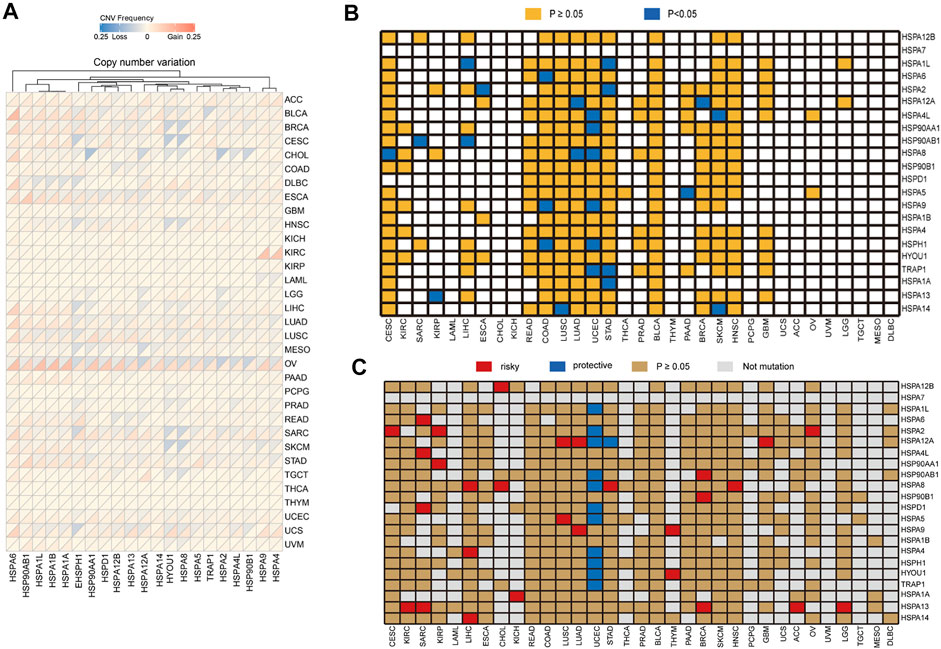
FIGURE 7. Copy number variation of HSPs and the correlation of their variation with expression and prognosis. (A) Copy number variation frequency of HSPs in across different cancer types. (B) Association of HSP mutations with expression. Orange color represents p ≥ 0.05, blue color represents p < 0.05. (C) Correlation between HSP mutations and prognosis in different cancer types. Red color represents a worse prognosis, blue color represents a better prognosis and brown color represents p ≥ 0.05. HSPs, heat shock proteins.
Relationships Between HSP Expressions and Gene Variations in Pan-Cancer
We then examined the correlations of HSP gene variations with expression in cancer. Our results showed that the mutation of 15 HSPs, not including HSPA7, HSPD1, HSPA4, HSPA1B, HYOU1, HSP90B1 and HSPA12B, affected their protein expression in different tumors (Table 4 and Figure 7B). Notably, the mutation of six HSPs, including HSPA4L, TRAP1, HSPH1, HSP90AA1, HSPA8 and HSPA9, was associated with their protein expression in uterine corpus endometrial carcinoma (p < 0.05).
We further found that CNVs of HSP families impacted their expression levels in different tumors (p < 0.05) (Supplementary Table S5). For example, copy number amplification of HSPA2 was associated with its increased expression in breast cancer, glioblastoma multiforme, head and neck squamous carcinoma, lung squamous cell carcinoma, uterine corpus endometrial carcinoma and ovarian serous cystadenocarcinoma (Table 5). Increased copy number of HSPD1 was associated with increased expression in stomach adenocarcinoma and breast cancer, while its up-regulation was observed in pancreatic adenocarcinoma regardless of whether HSPD1 gene was amplified or deleted (Table 5).
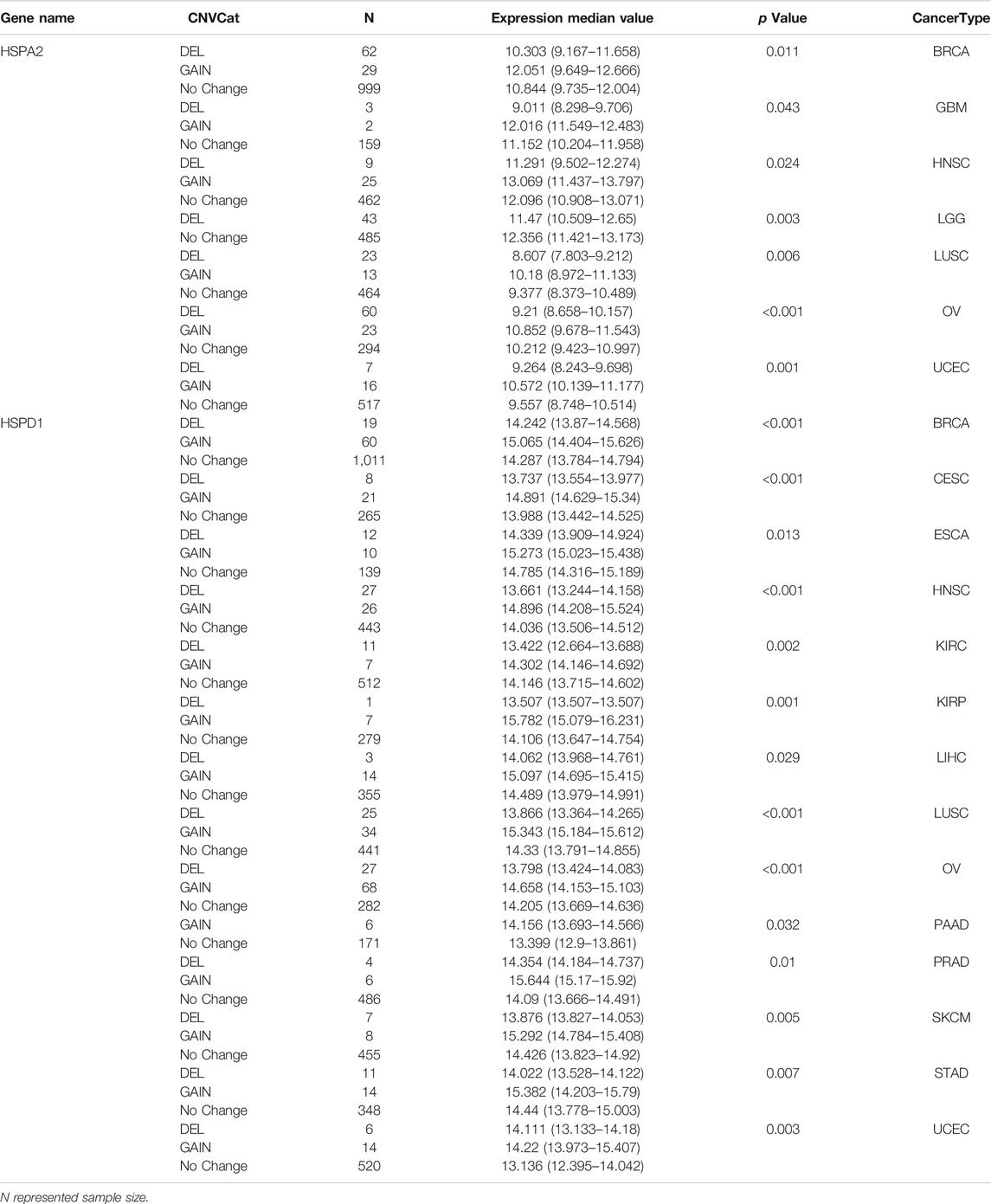
TABLE 5. Relationships between heat shock protein expressions and copy number variations in pan-cancer.
Relationships Between HSP Gene Variations and Prognosis in Pan-Cancer
We next examined the relationships of HSP gene variations with prognosis in pan-cancer using TCGA data. We found that mutations of HSP family genes were generally associated with good prognosis in uterine corpus endometrial carcinoma, while mutations in these genes were a poor prognostic factor in other tumors (Figure 7C). Mutations of 15 HSPs could lead to decrease or increase in expression and mutations of 20 HSPs were correlated with poor prognosis with different cancers (Table 4; Figures 7B,C). Further, we concluded and found that there were mutations of three HSPs that could cause the change of expression and were also related to prognosis (Table 4). For example, the expression of HSPA8 and HSPH1 could be increased and related to good prognosis of patients with their mutations in uterine corpus endometrial carcinoma; HSPA12A mutation in lung adenocarcinoma could down-regulate its expression and lead to poor prognosis (Table 4).
Furthermore, CNVs of HSPs, except for HSPA6, HSPA7, HSPD1, HSPA4L, HSPA14, HSPA12A, HSP90B1 and TRAP1, correlated with survival period in different cancers (Table 6). HSPA1A CNVs were correlated with shorter survival time in stomach adenocarcinoma (HR = 1.317, OR = 1.044–1.661, p = 0.02) and esophageal carcinoma (HR = 1.386, OR = 1.007–1.908, p = 0.045). HSP90AA1 CNVs was correlated with shorter survival rate in prostate adenocarcinoma (HR = 3.391, OR = 1.07–10.75, p = 0.038) (Table 6).
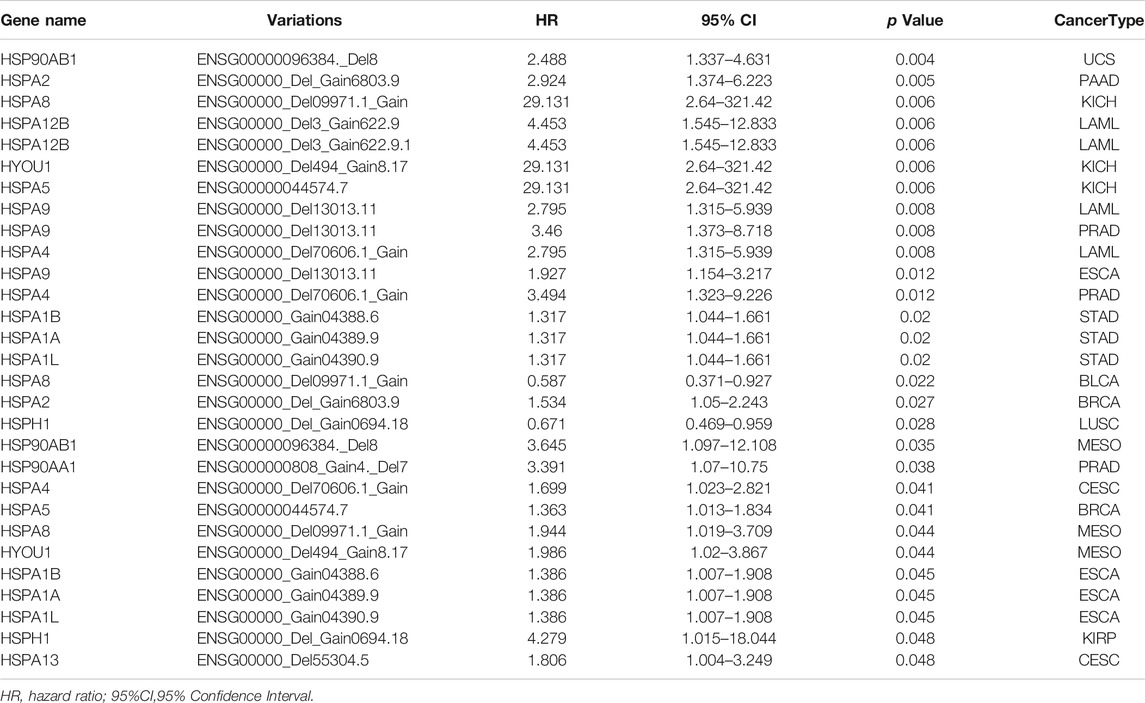
TABLE 6. Correlations between copy number variations and prognosis of heat shock proteins in pan-cancer.
qRT-PCR Validation and Clinicopathological Parameter Analysis of Differentially Expressed HSPs In Vivo
In this study, some tumors that differentially expressed HSPs, such as stomach adenocarcinoma and colon adenocarcinoma, were employed for validation in vivo by qRT-PCR. The result showed that HSPA2 was down-regulated in 53 pairs stomach adenocarcinoma tissues and 42 pairs colon adenocarcinoma tissues; HSPA7 and HSPA1A also were down-regulated in 42 pairs colon adenocarcinoma tissues (Figure 8). The correlation analysis also showed the significant association among genes in colon adenocarcinoma, such as HSPA2-HSPA7 (r = 0.031, p = 0.009), HSPA1A-HSPA7 (r = 0.516, p < 0.001) (Table 7). These results were consistent with above bioinformatic analysis.
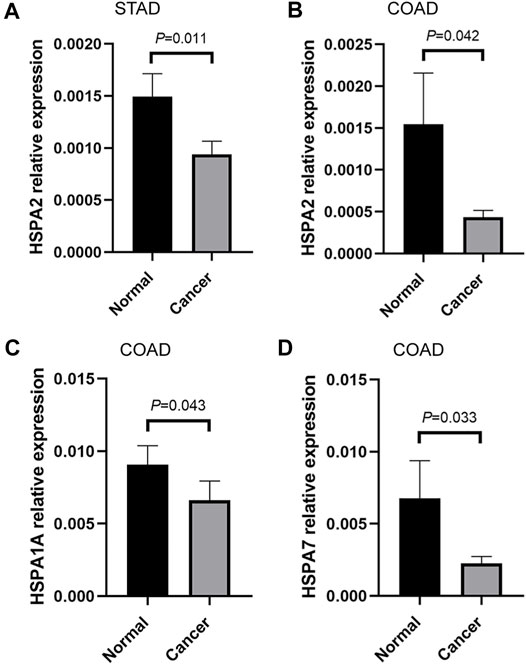
FIGURE 8. qRT-PCR validation of HSPs in vivo. (A) The mRNA relative expression of HSPA2 detected by qRT-PCR in STAD. (B) The mRNA relative expression of HSPA2 detected by qRT-PCR in COAD. (C) The mRNA relative expression of HSPA1A detected by qRT-PCR in COAD. (D) The mRNA relative expression of HSPA7 detected by qRT-PCR in COAD (Measurement data were expressed as mean ± SEM, p < 0.05). COAD, colon adenocarcinoma. HSPs, heat shock proteins. qRT-PCR, quantitative real-time polymerase chain reaction. STAD, stomach adenocarcinoma. SEM, standard error mean.
Further, the relationships of HSPs expression with clinicopathological parameter of gastrointestinal cancer patients were analyzed. The results showed that there is no significant association between HSPs expression and clinicopathological parameter in gastrointestinal cancer (Supplementary Tables S6, S7).
Discussion
HSPs play important roles in various biological processes in tumor cells including cell proliferation, invasion and migration and are potential clinical biomarkers and therapeutic targets (Kumar et al., 2016; Saini and Sharma, 2018). Systematic understanding of HSP profiles in pan-cancer is helpful for us to explore its pathogenic mechanism. In this study, we downloaded the expression, survival, mutation and CNVs data in various tumors from TCGA, Oncomine, CCLE and THPA databases, which were initially used to perform a joint analysis of different levels with HSP110, HSP90, HSP70 and HSP60 families. We comprehensively combed the expression profiles of HSPs at mRNA, protein and cell levels and investigated the associations among their expressions and the correlations of expressions with cancer-related pathways, immune cell infiltration and prognosis. qRT-PCR was used to validated the differentially expressed HSPs in stomach adenocarcinoma and colon adenocarcinoma tissues.
The expression profile analysis revealed that 11 HSPs were differentially expressed in 11 tumors at the mRNA level. Furthermore, 21 HSPs showed different expression patterns at the protein level in pan-cancer. Sixteen HSPs showed high expression levels in 425 cell lines from 8 tumors. qRT-PCR validation results verified that HSPA2 was down-regulated in stomach adenocarcinoma and colon adenocarcinoma, HSPA7 and HSPA1A also were down-regulated in colon adenocarcinoma tissues. Previous studies have shown that HSPs are differentially expressed in different cancers, including HSPA2 and HSPA1A (Melendez et al., 2006; Li et al., 2014; Cao et al., 2019; Lee and Kim, 2019; Zhang et al., 2020b). Our analysis and validations indicated that HSP family members have the potential to be diagnostic biomarkers of cancer.
We further analyzed the correlation and interaction relationship among HSP family members. The results revealed a mainly positive correlation among HSP expressions in different cancers, such as HSPA2-HSPA7 and HSPA1A-HSPA7 in colon adenocarcinoma colon adenocarcinoma. Our qRT-PCR result in vivo also verified the significant correlation in HSPA2-HSPA7 (r = 0.031, p = 0.009), HSPA1A-HSPA7 (r = 0.516, p < 0.001) in colon adenocarcinoma tissues. This study disclosed the correlation among HSPs for the first time, which contribute to the pathogenetic mechanism research. PPI network analysis indicated that there were generally interactions among HSPs. HSPH1 interacts with HSPA5 to promote endoplasmic reticulum stress–induced caspase-3 activation and subsequent apoptosis (Meares et al., 2008). The N-terminus and C-terminus of HSPA9 interact with HSP90B1 to regulate tumor cell functions (Takano et al., 2001). Other studies showed that HSPA9 and HSPA4 influence the biological behavior of tumor cells to promote cancer progression (Gu et al., 2019; Starenki et al., 2019). This study initially found and validated the correlation and interaction among HSPs. Therefore, we speculate that these HSPs may affect tumor development by cooperating with each other. Further study is needed to explore this possibility.
Our results indicate that HSPs may be involved in the activation and inhibition of different cancer-related pathways, such as the unfolded protein response pathway, mitotic spindle pathway and reactive oxygen species pathway, demonstrating that HSPs play different functions in tumor progression. A previous study reported that HSP90 is involved in activating the IL6/JAK/STAT3 signaling pathway to affect tumor progression (Lin et al., 2013; Kolosenko et al., 2014). HSP70 inhibits oxidative phosphorylation and compensate ATP balance through enhanced glycolytic activity in HeLa cells (Wang et al., 2012). HSP90 were related to the activation of the unfolded protein response pathway in myeloma plasma cells (Davenport et al., 2007). HSPA12B secreted by tumor-associated endothelial cells induces M2 polarization of macrophages by activating the PI3K/Akt/mTOR signaling pathway, thus promoting the formation of the immunosuppressive microenvironment within tumors (Zhou et al., 2020). Our results suggested that HSPA1L, HSPA12B, HSPA12A, HSPA7, HSPH1, HYOU1, HSPA14 and HSPA13 may inhibit the oxidative phosphorylation pathway. In addition, HSPA1L, HSPA12B, HSPA12A, HSP90AB1, HSPA2, HSPA14, HSPA13 and HSPH1 may inhibit the fatty acid metabolism pathway; HSPA6, HSPH1, HSP90AA1, HSPA14, HSPA5 and HSPA8 may activate the MTORC1 signaling pathway, while HSPA1L, HSPA12B and HSPA12A may inactivate the MTORC1 signaling pathway. Previous studies have demonstrated the close correlation of tumor progression with the above pathways (Li and Zhang, 2016; Kim et al., 2017; Yu et al., 2017). Our study provides the first evidence for the association of HSPs with these cancer-related pathways. These findings may provide new clues for expanding our understanding of cancer-related pathways and their specific functions in tumor cells.
Based on previous studies demonstrating an involvement of HSPs in the immune response, we examined the correlation of HSP with immune cell infiltration. The results showed that the HSPs was related to immune cell infiltration in 28 tumors. One study reported that HSPD1 regulates the antibacterial function of neutrophils (Osterloh et al., 2009). HSP70 released by heat-stressed tumor cells induced the production of tumor cell chemokines and activation of dendritic cells via the TLR4 pathway, thus initiating anti-cancer immunity (Chen et al., 2009). M2 macrophages could elevate HSPA5 expression to trigger an inflammatory response, and thus facilitating tumor metastasis. (Zhang et al., 2017). Our results showed that HSPs were closely related to the infiltration of immune cells, such as M1 macrophages, resting mast cells, M0 macrophages, M2 macrophages and CD4 memory activated T cells. These findings may provide new directions for researching immune-targeted therapy for cancer.
Our findings showed that HSPs expressions had various effects on prognosis in 25 tumors. HSPs expressions were related to poor prognosis in several cancers, such as sarcoma, lung squamous cell carcinoma and uterine corpus endometrial carcinoma, and were associated with good prognosis in cholangiocarcinoma, pheochromocytoma and paraganglioma. HSPs expressions were correlated with poor and good prognosis in other tumors, such as liver hepatocellular carcinoma and adrenocortical carcinoma. HSPD1 promotes cell invasion and migration, which contributes to the poor prognosis in oral squamous cell carcinoma (Kang et al., 2019). The expression of HSP90AB1 also caused the worse prognosis in lung adenocarcinoma (Wang et al., 2016). HSPA9 expression was positive associated with histological grade, pathological stage and lymphatic metastasis in breast cancer, and its expression was negative associated with shorter disease-free survival and overall survival (Jin et al., 2016). Our study demonstrated that HSP expressions showed different effects on the prognosis of tumors in the digestive, respiratory, urinary and reproductive system. These results indicate that HSPs have the potential to be prognostic biomarkers of different cancers. These findings may also help establish an important foundation for further mechanism studies on how HSPs affect prognosis.
In this study, HSP expression profiles, gene mutations and CNVs as well as the correlation of gene mutations and CNVs with expression and the impact of expression on prognosis were analyzed in pan-cancer. We found that HSP mutations were frequent in uterine corpus endometrial carcinoma, colon adenocarcinoma, stomach adenocarcinoma, rectum adenocarcinoma, lung squamous cell carcinoma and lung adenocarcinoma, with a mutation frequency of 0–23%. HSP mutations were the most common in endometrial cancer, while mutations rarely occurred in testicular germ cell tumors, uveal melanoma, thymoma and thymoma. The mutation frequency of HSP genes in different cell lines of 15 tumors was 0–17%. Previous analysis illustrated that frequent mutations of HSPH1, HSPD1, HSPA4 and HSP90AA1 were detected in head and neck cancer in head and neck squamous carcinoma (Fan et al., 2020). Somatic mutations and deletions of HSPA8 were observed in sporadic breast carcinoma (Bakkenist et al., 1999). The mutation frequency of HSP90AA1 in gestational trophoblastic neoplasia was 18.2% (Luo et al., 2020). Mutations in HSP genes may play an important role in the occurrence and development of tumors and might be targets of anti-cancer treatments. The mutation correlation among HSPs was analyzed in uterine corpus endometrial carcinoma. The results showed that the co-occurrence of mutations in HSPA1L-HSP90B1, HSPA1L-HSPA5, HSPA4L-HSPA4 and HSPA4L-HSPA6 pairs were frequently observed in uterine corpus endometrial carcinoma. Therefore, we predicted that the mutations of these HSPs were closely related and might affect tumor progression.
In this study, the mutation of 15 HSPs affected protein expressions in different cancers. HSP gene mutations were generally associated with good prognosis in uterine corpus endometrial carcinoma, while mutations were poor prognostic factors in other cancers. Previous studies reported frameshift mutations of HSPA4 in gastric and colorectal cancers with microsatellite instability (Jo et al., 2016). The HSPA4 frameshift mutation seems to reduce the survival activity of tumor cells, which may partially explain why patients of gastric and colorectal cancers with microsatellite instability have better prognosis than those with microsatellite stable cancer (Jo et al., 2016). One study demonstrated that mutant HSPH1 showed disrupted cellular localization and interaction with other HSPs, thus abolishing the chaperone activity and anti-apoptotic function of HSPH1 by a dominant-negative manner (Dorard et al., 2011). In addition, HSPH1 mutation enhanced chemosensitivity to drugs, such as oxaliplatin and 5-fluorouracil, and improved prognosis in colorectal cancers with microsatellite instability (Dorard et al., 2011).
CNV analysis indicated that amplification and deletion of HSP genes in pan-cancer. Our results also showed that CNVs impacted the expression level of HSPs in different tumors. CNVs of 14 HSPs were associated with good and poor prognosis in various tumors. Somatic CNVs in general can impact protein expression level and thus influence the occurrence and development of different tumors; these CNVs have potential to be diagnostic and prognostic biomarkers as well as anti-cancer therapeutic targets (Cheau-Feng Lin et al., 2014; Hieronymus et al., 2018; Shao et al., 2019). Mutations and CNVs are crucial for tumor progression and are closely related to the pathogenic mechanisms of tumors. In our study, mutations and CNVs of HSP genes were correlated with protein expression as well as prognosis in various cancers. These variations are potential immunotherapeutic targets, and further exploration of pathogenic mechanisms is required.
Conclusion
Our study analyzed the expression profiles of HSP110, HSP90, HSP70 and HSP60 families in pan-cancer, the relationships among their expression and the correlations of expression with cancer-related signal transduction pathways, immune cell infiltration and prognosis. We also examined the mutations and CNV profiles of HSPs as well as the association of expression with mutations and CNVs. We found that HSP family members are differently expressed in pan-cancer and are closely related to prognosis. Mutations and CNVs in HSPs exert various effects on expression and prognosis in different tumors. HSPs were closely related to immune cell infiltrations in different cancers. In this study, we comprehensively analyzed HSP110, HSP90, HSP70, and HSP60 families in cancer. These results expand our understanding of these proteins and clarified the potential roles for HSPs as diagnostic and prognostic biomarkers as well as anti-cancer therapeutic targets. Our findings provide promising clues for further research of the pathogenic mechanisms of tumors.
Data Availability Statement
The data that support the results of this article are available from the corresponding author upon reasonable request.
Ethics Statement
The studies involving human participants were reviewed and approved by The research ethics committee of the First Affiliated Hospital of China Medical University ([2018]2018-52-2 and [2021]94). The patients/participants provided their written informed consent to participate in this study.
Author Contributions
QX and YY conceived and designed this study. L-RY, S-XS, AW, H-XD, and Y-NL were responsible for the data analysis and performed data interpretation. L-RY wrote the paper. QX and YY revised the manuscript.
Funding
This work was supported by the National Key R and D Program of China (2017YFC0907402).
Conflict of Interest
The authors declare that the research was conducted in the absence of any commercial or financial relationships that could be construed as a potential conflict of interest.
Publisher’s Note
All claims expressed in this article are solely those of the authors and do not necessarily represent those of their affiliated organizations, or those of the publisher, the editors and the reviewers. Any product that may be evaluated in this article, or claim that may be made by its manufacturer, is not guaranteed or endorsed by the publisher.
Supplementary Material
The Supplementary Material for this article can be found online at: https://www.frontiersin.org/articles/10.3389/fmolb.2021.726244/full#supplementary-material
References
Bakkenist, C. J., Koreth, J., Williams, C. S., Hunt, N. C., and McGee, J. O. (1999). Heat Shock Cognate 70 Mutations in Sporadic Breast Carcinoma. Cancer Res. 59 (17), 4219–4221.
Bhattacharya, K., Weidenauer, L., Luengo, T. M., Pieters, E. C., Echeverría, P. C., Bernasconi, L., et al. (2020). The Hsp70-Hsp90 Co-chaperone Hop/Stip1 Shifts the Proteostatic Balance from Folding towards Degradation. Nat. Commun. 11 (1), 5975. doi:10.1038/s41467-020-19783-w
Böttinger, L., Oeljeklaus, S., Guiard, B., Rospert, S., Warscheid, B., and Becker, T. (2015). Mitochondrial Heat Shock Protein (Hsp) 70 and Hsp10 Cooperate in the Formation of Hsp60 Complexes. J. Biol. Chem. 290 (18), 11611–11622. doi:10.1074/jbc.m115.642017
Bray, F., Ferlay, J., Soerjomataram, I., Siegel, R. L., Torre, L. A., and Jemal, A. (2018). Global Cancer Statistics 2018: GLOBOCAN Estimates of Incidence and Mortality Worldwide for 36 Cancers in 185 Countries. CA: a Cancer J. clinicians 68 (6), 394–424. doi:10.3322/caac.21492
Cao, L., Yuan, X., Bao, F., Lv, W., He, Z., Tang, J., et al. (2019). Downregulation of HSPA2 Inhibits Proliferation via ERK1/2 Pathway and Endoplasmic Reticular Stress in Lung Adenocarcinoma. Ann. Transl. Med. 7 (20), 540. doi:10.21037/atm.2019.10.16
Cheau-Feng Lin, F., Jeng, Y.-C., Huang, T.-Y., Chi, C.-S., Chou, M.-C., and Chin-Shaw Tsai, S. (2014). Mitochondrial DNA Copy Number Is Associated with Diagnosis and Prognosis of Head and Neck Cancer. Biomarkers 19 (4), 269–274. doi:10.3109/1354750x.2014.902101
Chen, T., Guo, J., Han, C., Yang, M., and Cao, X. (2009). Heat Shock Protein 70, Released from Heat-Stressed Tumor Cells, Initiates Antitumor Immunity by Inducing Tumor Cell Chemokine Production and Activating Dendritic Cells via TLR4 Pathway. J. Immunol. 182 (3), 1449–1459. doi:10.4049/jimmunol.182.3.1449
Davenport, E. L., Moore, H. E., Dunlop, A. S., Sharp, S. Y., Workman, P., Morgan, G. J., et al. (2007). Heat Shock Protein Inhibition Is Associated with Activation of the Unfolded Protein Response Pathway in Myeloma Plasma Cells. Blood 110 (7), 2641–2649. doi:10.1182/blood-2006-11-053728
Diboun, I., Wernisch, L., Orengo, C. A., and Koltzenburg, M. (2006). Microarray Analysis after RNA Amplification Can Detect Pronounced Differences in Gene Expression Using Limma. BMC genomics 7, 252. doi:10.1186/1471-2164-7-252
Dorard, C., de Thonel, A., Collura, A., Marisa, L., Svrcek, M., Lagrange, A., et al. (2011). Expression of a Mutant HSP110 Sensitizes Colorectal Cancer Cells to Chemotherapy and Improves Disease Prognosis. Nat. Med. 17 (10), 1283–1289. doi:10.1038/nm.2457
Fan, G., Tu, Y., Wu, N., and Xiao, H. (2020). The Expression Profiles and Prognostic Values of HSPs Family Members in Head and Neck Cancer. Cancer Cel Int 20, 220. doi:10.1186/s12935-020-01296-7
Genest, O., Wickner, S., and Doyle, S. M. (2019). Hsp90 and Hsp70 Chaperones: Collaborators in Protein Remodeling. J. Biol. Chem. 294 (6), 2109–2120. doi:10.1074/jbc.rev118.002806
Ghandi, M., Huang, F. W., Jané-Valbuena, J., Kryukov, G. V., Lo, C. C., McDonald, E. R., et al. (2019). Next-generation Characterization of the Cancer Cell Line Encyclopedia. Nature 569 (7757), 503–508. doi:10.1038/s41586-019-1186-3
Gibert, B., Simon, S., Dimitrova, V., Diaz-Latoud, C., and Arrigo, A.-P. (2013). Peptide Aptamers: Tools to Negatively or Positively Modulate HSPB1(27) Function. Phil. Trans. R. Soc. B 368 (1617), 20120075. doi:10.1098/rstb.2012.0075
Gu, Y., Liu, Y., Fu, L., Zhai, L., Zhu, J., Han, Y., et al. (2019). Tumor-educated B Cells Selectively Promote Breast Cancer Lymph Node Metastasis by HSPA4-Targeting IgG. Nat. Med. 25 (2), 312–322. doi:10.1038/s41591-018-0309-y
Hänzelmann, S., Castelo, R., and Guinney, J. (2013). GSVA: Gene Set Variation Analysis for Microarray and RNA-Seq Data. BMC bioinformatics 14, 7. doi:10.1186/1471-2105-14-7
He, L., Deng, T., and Luo, H. S. (2014). Heat Shock Protein 70 Gene Polymorphisms and Cancer Risk: a Meta-Analysis. ScientificWorldJournal 2014, 540309. doi:10.1155/2014/540309
Hieronymus, H., Murali, R., Tin, A., Yadav, K., Abida, W., Moller, H., et al. (2018). Tumor Copy Number Alteration burden Is a Pan-Cancer Prognostic Factor Associated with Recurrence and Death. eLife 7, e37294. doi:10.7554/eLife.37294
Jin, H., Ji, M., Chen, L., Liu, Q., Che, S., Xu, M., et al. (2016). The Clinicopathological Significance of Mortalin Overexpression in Invasive Ductal Carcinoma of Breast. J. Exp. Clin. Cancer Res. 35, 42. doi:10.1186/s13046-016-0316-0
Jo, Y. S., Choi, M. R., Song, S. Y., Kim, M. S., Yoo, N. J., and Lee, S. H. (2016). Frameshift Mutations of HSPA4 and MED13 in Gastric and Colorectal Cancers. Pathol. Oncol. Res. 22 (4), 769–772. doi:10.1007/s12253-016-0070-9
Kampinga, H. H., Hageman, J., Vos, M. J., Kubota, H., Tanguay, R. M., Bruford, E. A., et al. (2009). Guidelines for the Nomenclature of the Human Heat Shock Proteins. Cell Stress and Chaperones 14 (1), 105–111. doi:10.1007/s12192-008-0068-7
Kang, B.-H., Shu, C.-W., Chao, J.-K., Lee, C.-H., Fu, T.-Y., Liou, H.-H., et al. (2019). HSPD1 Repressed E-Cadherin Expression to Promote Cell Invasion and Migration for Poor Prognosis in Oral Squamous Cell Carcinoma. Sci. Rep. 9 (1), 8932. doi:10.1038/s41598-019-45489-1
Kim, C., Gao, R., Sei, E., Brandt, R., Hartman, J., Hatschek, T., et al. (2018). Chemoresistance Evolution in Triple-Negative Breast Cancer Delineated by Single-Cell Sequencing. Cell 173 (4), 879–893. e13. doi:10.1016/j.cell.2018.03.041
Kim, L. C., Cook, R. S., and Chen, J. (2017). mTORC1 and mTORC2 in Cancer and the Tumor Microenvironment. Oncogene 36 (16), 2191–2201. doi:10.1038/onc.2016.363
Kolosenko, I., Grander, D., and Tamm, K. P. (2014). IL-6 Activated JAK/STAT3 Pathway and Sensitivity to Hsp90 Inhibitors in Multiple Myeloma. Cmc 21 (26), 3042–3047. doi:10.2174/0929867321666140414100831
Kravats, A. N., Hoskins, J. R., Reidy, M., Johnson, J. L., Doyle, S. M., Genest, O., et al. (2018). Functional and Physical Interaction between Yeast Hsp90 and Hsp70. Proc. Natl. Acad. Sci. USA 115 (10), E2210–E2219. doi:10.1073/pnas.1719969115
Kumar, S., Stokes, J., Singh, U. P., Scissum Gunn, K., Acharya, A., Manne, U., et al. (2016). Targeting Hsp70: A Possible Therapy for Cancer. Cancer Lett. 374 (1), 156–166. doi:10.1016/j.canlet.2016.01.056
Lee, H. W., and Kim, K. M. (2019). Clinical Significance of Heat Shock Protein 90α Expression as a Biomarker of Prognosis in Patients with Gastric Cancer. Niger. J. Clin. Pract. 22 (12), 1698–1705. doi:10.4103/njcp.njcp_68_19
Li, X.-s., Xu, Q., Fu, X.-y., and Luo, W.-s. (2014). Heat Shock Protein 60 Overexpression Is Associated with the Progression and Prognosis in Gastric Cancer. PloS one 9 (9), e107507. doi:10.1371/journal.pone.0107507
Li, Z., and Zhang, H. (2016). Reprogramming of Glucose, Fatty Acid and Amino Acid Metabolism for Cancer Progression. Cell. Mol. Life Sci. 73 (2), 377–392. doi:10.1007/s00018-015-2070-4
Lin, C.-S., He, P.-J., Hsu, W.-T., Wu, M.-S., Wu, C.-J., Shen, H.-W., et al. (2010). Helicobacter Pylori-Derived Heat Shock Protein 60 Enhances Angiogenesis via a CXCR2-Mediated Signaling Pathway. Biochem. biophysical Res. Commun. 397 (2), 283–289. doi:10.1016/j.bbrc.2010.05.101
Lin, H., Kolosenko, I., Björklund, A.-C., Protsyuk, D., Österborg, A., Grandér, D., et al. (2013). An Activated JAK/STAT3 Pathway and CD45 Expression Are Associated with Sensitivity to Hsp90 Inhibitors in Multiple Myeloma. Exp. Cel. Res. 319 (5), 600–611. doi:10.1016/j.yexcr.2012.12.006
Luo, L., Lin, L., Zhang, X., Cai, Q., Zhao, H., Xu, C., et al. (2020). Next-Generation Sequencing Panel Analysis of Clinically Relevant Mutations in Circulating Cell-free DNA from Patients with Gestational Trophoblastic Neoplasia: A Pilot Study. Biomed. Res. Int. 2020, 1314967. doi:10.1155/2020/1314967
Macario, A. J. L., and Conway de Macario, E. (2007). Molecular Chaperones: Multiple Functions, Pathologies, and Potential Applications. Front. Biosci. 12, 2588–2600. doi:10.2741/2257
Meares, G. P., Zmijewska, A. A., and Jope, R. S. (2008). HSP105 Interacts with GRP78 and GSK3 and Promotes ER Stress-Induced Caspase-3 Activation. Cell Signal. 20 (2), 347–358. doi:10.1016/j.cellsig.2007.10.032
Medhi, S., Sarma, M. P., Asim, M., and Kar, P. (2013). Genetic Variants of Heat Shock Protein A1L2437 and A1B1267 as Possible Risk Factors for Hepatocellular Carcinoma in India. J. Viral Hepat. 20 (4), e141–e147. doi:10.1111/jvh.12021
Melendez, K., Wallen, E. S., Edwards, B. S., Mobarak, C. D., Bear, D. G., and Moseley, P. L. (2006). Heat Shock Protein 70 and Glycoprotein 96 Are Differentially Expressed on the Surface of Malignant and Nonmalignant Breast Cells. Cell Stress Chaper 11 (4), 334–342. doi:10.1379/csc-187.1
Miao, Y., Wang, J., Li, Q., Quan, W., Wang, Y., Li, C., et al. (2020). Prognostic Value and Immunological Role of PDCD1 Gene in Pan-Cancer. Int. immunopharmacology 89 (Pt B), 107080. doi:10.1016/j.intimp.2020.107080
Newman, A. M., Liu, C. L., Green, M. R., Gentles, A. J., Feng, W., Xu, Y., et al. (2015). Robust Enumeration of Cell Subsets from Tissue Expression Profiles. Nat. Methods 12 (5), 453–457. doi:10.1038/nmeth.3337
Osterloh, A., Geisinger, F., Piédavent, M., Fleischer, B., Brattig, N., and Breloer, M. (2009). Heat Shock Protein 60 (HSP60) Stimulates Neutrophil Effector Functions. J. Leukoc. Biol. 86 (2), 423–434. doi:10.1189/jlb.0109011
Rhodes, D. R., Kalyana-Sundaram, S., Mahavisno, V., Varambally, R., Yu, J., Briggs, B. B., et al. (2007). Oncomine 3.0: Genes, Pathways, and Networks in a Collection of 18,000 Cancer Gene Expression Profiles. Neoplasia 9 (2), 166–180. doi:10.1593/neo.07112
Saini, J., and Sharma, P. K. (2018). Clinical, Prognostic and Therapeutic Significance of Heat Shock Proteins in Cancer. Cdt 19 (13), 1478–1490. doi:10.2174/1389450118666170823121248
Shao, X., Lv, N., Liao, J., Long, J., Xue, R., Ai, N., et al. (2019). Copy Number Variation Is Highly Correlated with Differential Gene Expression: a Pan-Cancer Study. BMC Med. Genet. 20 (1), 175. doi:10.1186/s12881-019-0909-5
Starenki, D., Sosonkina, N., Hong, S. K., Lloyd, R. V., and Park, J. I. (2019). Mortalin (GRP75/HSPA9) Promotes Survival and Proliferation of Thyroid Carcinoma Cells. Int. J. Mol. Sci. 20 (9), 2069. doi:10.3390/ijms20092069
Takano, S., Wadhwa, R., Mitsui, Y., and Kaul, S. C. (2001). Identification and Characterization of Molecular Interactions between Glucose-Regulated Proteins (GRPs) mortalin/GRP75/peptide-Binding Protein 74 (PBP74) and GRP94. Biochem. J. 357 (Pt 2), 393–398. doi:10.1042/0264-6021:3570393
Todryk, S., Melcher, A. A., Hardwick, N., Linardakis, E., Bateman, A., Colombo, M. P., et al. (1950). Heat Shock Protein 70 Induced during Tumor Cell Killing Induces Th1 Cytokines and Targets Immature Dendritic Cell Precursors to Enhance Antigen Uptake. J. Immunol. 163 (3), 1398–1408.
Tsai, Y.-P., Yang, M.-H., Huang, C.-H., Chang, S.-Y., Chen, P.-M., Liu, C.-J., et al. (2009). Interaction between HSP60 and β-catenin Promotes Metastasis. Carcinogenesis 30 (6), 1049–1057. doi:10.1093/carcin/bgp087
Tsukahara, F., Yoshioka, T., and Muraki, T. (2000). Molecular and Functional Characterization of HSC54, a Novel Variant of Human Heat-Shock Cognate Protein 70. Mol. Pharmacol. 58 (6), 1257–1263. doi:10.1124/mol.58.6.1257
Wang, L., Schumann, U., Liu, Y., Prokopchuk, O., and Steinacker, J. M. (2012). Heat Shock Protein 70 (Hsp70) Inhibits Oxidative Phosphorylation and Compensates ATP Balance through Enhanced Glycolytic Activity. J. Appl. Physiol. 113 (11), 1669–1676. doi:10.1152/japplphysiol.00658.2012
Wang, M., Feng, L., Li, P., Han, N., Gao, Y., and Xiao, T. (2016). Hsp90AB1 Protein is Overexpressed in Non-small Cell Lung Cancer Tissues and Associated with Poor Prognosis in Lung Adenocarcinoma Patients. Zhongguo Fei Ai Za Zhi 19 (2), 64–69. doi:10.3779/j.issn.1009-3419.2016.02.02
Wong, C., Wong, V., Chan, C., Ma, B., Hui, E., Wong, M., et al. (2008). Identification of 5-fluorouracil Response Proteins in Colorectal Carcinoma Cell Line SW480 by Two-Dimensional Electrophoresis and MALDI-TOF Mass Spectrometry. Oncol. Rep. 20 (1), 89–98. doi:10.3892/or.20.1.89
Wu, J., Liu, T., Rios, Z., Mei, Q., Lin, X., and Cao, S. (2017). Heat Shock Proteins and Cancer. Trends Pharmacological Sciences 38 (3), 226–256. doi:10.1016/j.tips.2016.11.009
Xu, L., Chen, S., and Bergan, R. C. (2006). MAPKAPK2 and HSP27 Are Downstream Effectors of P38 MAP Kinase-Mediated Matrix Metalloproteinase Type 2 Activation and Cell Invasion in Human Prostate Cancer. Oncogene 25 (21), 2987–2998. doi:10.1038/sj.onc.1209337
Yamazaki, K., Ohsawa, Y., Tabeta, K., Ito, H., Ueki, K., Oda, T., et al. (2002). Accumulation of Human Heat Shock Protein 60-reactive T Cells in the Gingival Tissues of Periodontitis Patients. Infect. Immun. 70 (5), 2492–2501. doi:10.1128/iai.70.5.2492-2501.2002
Yu, L., Lu, M., Jia, D., Ma, J., Ben-Jacob, E., Levine, H., et al. (2017). Modeling the Genetic Regulation of Cancer Metabolism: Interplay between Glycolysis and Oxidative Phosphorylation. Cancer Res. 77 (7), 1564–1574. doi:10.1158/0008-5472.can-16-2074
Yun, C. W., Kim, H. J., Lim, J. H., and Lee, S. H. (2019). Heat Shock Proteins: Agents of Cancer Development and Therapeutic Targets in Anti-cancer Therapy. Cells 9 (1), 60. doi:10.3390/cells9010060
Zhang, L., Li, Z., Ding, G., La, X., Yang, P., and Li, Z. (2017). GRP78 Plays an Integral Role in Tumor Cell Inflammation-Related Migration Induced by M2 Macrophages. Cell Signal. 37, 136–148. doi:10.1016/j.cellsig.2017.06.008
Zhang, Q., Huang, R., Hu, H., Yu, L., Tang, Q., Tao, Y., et al. (2020). Integrative Analysis of Hypoxia-Associated Signature in Pan-Cancer. iScience 23 (9), 101460. doi:10.1016/j.isci.2020.101460
Zhang, S., Wang, B., Xiao, H., Dong, J., Li, Y., Zhu, C., et al. (2020). LncRNA HOTAIR Enhances Breast Cancer Radioresistance through Facilitating HSPA1A Expression via Sequestering miR ‐449b‐5p. Thorac. Cancer 11 (7), 1801–1816. doi:10.1111/1759-7714.13450
Zhou, J., Zhang, A., and Fan, L. (2020). HSPA12B Secreted by Tumor-Associated Endothelial Cells Might Induce M2 Polarization of Macrophages via Activating PI3K/Akt/mTOR Signaling. Ott 13, 9103–9111. doi:10.2147/ott.s254985
Zou, T., Liu, J.-Y., She, L., Yin, J.-Y., Li, X., Li, X.-P., et al. (2020). The Association between Heat-Shock Protein Polymorphisms and Prognosis in Lung Cancer Patients Treated with Platinum-Based Chemotherapy. Front. Pharmacol. 11, 1029. doi:10.3389/fphar.2020.01029
Glossary
ACC adrenocortical carcinoma
BLCA bladder urothelial carcinoma
BRCA breast invasive carcinoma
CCLE cancer cell line encyclopedia
CNVs copy number variations
CESC cervical squamous cell carcinoma and endocervical adenocarcinoma
CHOL cholangiocarcinoma
COAD colon adenocarcinoma
DLBC lymphoid neoplasm diffuse large B-cell lymphoma
ESCA esophageal carcinoma
GBM glioblastoma multiforme
HSPs heat shock proteins
HNSC head and neck squamous cell carcinoma
KICH kidney Chromophobe
KIRC kidney renal clear cell carcinoma
KIRP kidney renal papillary cell carcinoma
LAML acute myeloid leukemia
LGG brain lower grade glioma
LIHC liver hepatocellular carcinoma
LUAD lung adenocarcinoma
LUSC lung squamous cell carcinoma
MESO mesothelioma
OV ovarian serous cystadenocarcinoma
PAAD Pancreatic adenocarcinoma
PCPG pheochromocytoma, and paraganglioma
PPI protein-protein interaction
PRAD prostate adenocarcinoma
READ rectum adenocarcinoma
SARC sarcoma
SKCM skin cutaneous melanoma
STAD stomach adenocarcinoma
TGCT testicular germ cell tumors
THCA thyroid carcinoma
THYM thymoma
TCGA the cancer genome atlas
THPA the human protein atlas
UCEC uterine corpus endometrial carcinoma
UCS uterine carcinosarcoma
UVM uveal melanoma.
Keywords: heat shock proteins, expression, prognosis, mutation, pan-cancer
Citation: Yan L-r, Shen S-x, Wang A, Ding H-x, Liu Y-n, Yuan Y and Xu Q (2021) Comprehensive Pan-Cancer Analysis of Heat Shock Protein 110, 90, 70, and 60 Families. Front. Mol. Biosci. 8:726244. doi: 10.3389/fmolb.2021.726244
Received: 16 June 2021; Accepted: 27 September 2021;
Published: 12 October 2021.
Edited by:
Mahendra Pratap Kashyap, University of Alabama at Birmingham, United StatesReviewed by:
Sushmita Chatterjee, Tel Aviv University, IsraelPrihantono Prihantono, Hasanuddin University, Indonesia
Copyright © 2021 Yan, Shen, Wang, Ding, Liu, Yuan and Xu. This is an open-access article distributed under the terms of the Creative Commons Attribution License (CC BY). The use, distribution or reproduction in other forums is permitted, provided the original author(s) and the copyright owner(s) are credited and that the original publication in this journal is cited, in accordance with accepted academic practice. No use, distribution or reproduction is permitted which does not comply with these terms.
*Correspondence: Qian Xu, cXh1QGNtdS5lZHUuY24=; Yuan Yuan, eXVhbnl1YW5AY211LmVkdS5jbg==