- 1Department of Gastrointestinal Surgery, The Sixth Affiliated Hospital, Sun Yat-Sen University, Guangzhou, China
- 2Guangdong Provincial Key Laboratory of Colorectal and Pelvic Floor Diseases, Guangdong Institute of Gastroenterology, The Sixth Affiliated Hospital of Sun Yat-Sen University, Guangzhou, China
Purpose: Accumulating evidence indicates that N6-methyladenosine-related long non-coding RNAs (m6A-related lncRNAs) play a crucial role in the occurrence and development of several cancers. We aimed to explore the potential role of m6A-related lncRNA signatures in predicting prognosis for early-stage (stages I and II) colorectal cancer (CRC).
Methods: m6A-related lncRNA data were obtained from The Cancer Genome Atlas. Univariate Cox regression analysis was used to screen for prognostic m6A-related lncRNAs. Immune characteristics were analyzed in different subgroups created via unsupervised clustering analysis. Next, patients were randomly divided into training and test cohorts. In the training cohort, least absolute shrinkage and selection operator (LASSO) regression was performed to establish a prognostic model. The predictive value of the signature was evaluated in the training and test cohorts. Drug sensitivity was also examined.
Results: A total of 1,478 m6A-related lncRNAs were identified. Two subgroups were created based on the expression of seven prognostic m6A-related lncRNAs. Prognosis was worse for cluster 1 than for cluster 2, and cluster 1 was characterized by increased numbers of M2 macrophages, decreased numbers of memory B cells, and higher expression of checkpoint genes when compared with cluster 2. Five m6A-related lncRNAs were selected to establish a risk prediction signature via LASSO regression. The 3 years overall survival (OS) was higher in the low-risk group than in the high-risk group. The area under the curve at 1, 2, and 3 years was 0.929, 0.954, and 0.841 in the training cohort and 0.664, 0.760, and 0.754 in the test cohort, respectively. Multivariate Cox regression analysis suggests that the risk score was an independent predictor of OS in both the training and test cohorts. A prognostic nomogram based on the five m6A-related lncRNAs and their clinical features was built and verified. The high-risk group was more sensitive to chemotherapeutic drugs (camptothecin and cisplatin) than the low-risk group.
Conclusion: We identified two molecular subgroups of early-stage CRC with unique immune features based on seven prognostic m6A-related lncRNAs. Subsequent analyses demonstrated the usefulness of a five m6A-related lncRNA signature as a potential indicator of prognosis in patients with early-stage CRC.
Introduction
Colorectal cancer (CRC) is one of the most common gastrointestinal malignancies and is among the main causes of cancer-related deaths. Although the 5 years survival rate for early-stage CRC (stage I and stage II) can reach 90%, these patients are still at risk of recurrence, which is often fatal (Siegel et al., 2019; Walker et al., 2020). Whether chemotherapy is necessary for patients with early-stage CRC depends mainly on known clinical and pathological risk factors, such as microsatellite instability (MSI) status and bowel obstruction (Manfredi et al., 2006; Kannarkatt et al., 2017). However, these high-risk factors cannot distinguish between patients with poor prognosis and those who benefit from chemotherapy (Dienstmann et al., 2015; Kopetz et al., 2015). Therefore, a reliable molecular marker is urgently needed to identify high-risk groups of patients with early-stage CRC and to optimize treatment strategies.
N6-Methyladenosine (m6A)—which is present in messenger RNAs (mRNAs), non-coding RNAs (lncRNAs), and microRNAs (miRNAs) in most eukaryotes—is the most common epigenetic methylation modification of mammalian RNA (Desrosiers et al., 1974; Molinie and Giallourakis, 2017). M6A modification is regulated by a series of protein factors, including methyltransferases (writers), signal transducers (readers), and demethylases (erasers) (Yang et al., 2018a). Abnormal modification of m6A plays an important role in the occurrence and development of many tumors, such as hepatocellular carcinoma, breast cancer, glioblastoma, and lung cancer (Lin et al., 2016; Cui et al., 2017; Vu et al., 2017; Cai et al., 2018; Liu et al., 2019). Moreover, Zhang et al. demonstrated the effect of mutation of the m6A regulator on CRC prognosis (Zhang et al., 2020). Similar to mRNA, lncRNA is also regulated by m6A. Moreover, m6A-related lncRNAs also regulate a series of biological and pathological processes. Recent studies have demonstrated that m6A-related lncRNAs can reliably predict the prognosis of low-grade glioma, lung adenocarcinoma, and gastric cancer (Tu et al., 2020; Wang et al., 2021a; Wang et al., 2021b). Luo et al.(Zuo et al., 2021) further observed that m6A-related lncRNAs were associated with the occurrence and development of CRC, indicating that they may be an accurate prognostic factor for early-stage CRC.
The tumor microenvironment (TME) also plays an important role in tumor initiation and progression, and is comprised of tumor cells, stromal cells and innate and adaptive immune cells (Colangelo et al., 2017). Immunotherapy has quickly become the main treatment modality for CRC, such as programmed cell death1 (PD1)-blocking antibodies (Ganesh et al., 2019). Recently, more studies have focused on comprehensive analysis of specific m6A regulatory factors to strengthen the in-depth understanding of the heterogeneity and complexity of TME (Yang et al., 2019; Li et al., 2020). For example, Na Li et al. (Li et al., 2020) have found a deletion in Alkbh5 that sensitizes tumors to cancer immunotherapy by regulating myeloid-derived suppressor cells and suppressive lymphocyte Treg accumulation. However, at present, there are no studies on this aspect of early-stage CRC.
In the present study, we identified two molecular subgroups of early-stage CRC with unique immune characteristics and showed the potential role of m6A-related lncRNA signatures in predicting prognosis in patients with early-stage CRC.
Materials and Methods
Study Approval and Consent
This study was approved by the Medical Ethics Committee of the Sixth Affiliated Hospital of Sun Yat-sen University, Guangzhou, China (no. 2021ZSLYEC-006).
Data Acquisition and Preprocessing
Initially, we downloaded RNA sequencing data (FPKM value) related to gene expression and the corresponding clinical information for CRC from The Cancer Genome Atlas (TCGA) website (https://portal.gdc.cancer. gov). According to the 8th edition of the American Joint Committee on Cancer, we identified patients with early-stage CRC. The clinical information included age, sex, TNM stage, and survival status. In addition, patients with no survival information or a survival time of less than 3 months were excluded from further evaluation to reduce statistical bias. To distinguish the mRNAs and lncRNAs, we downloaded the GTF files from Ensembl (http://asia.ensembl.org) for further analysis. Data were normalized, processed, and analyzed using R software 4.0.3.
Identification of m6A-Related lncRNAs
We extracted expression data for 23 m6A-related genes identified in previous studies, including methyltransferases (METTL3, METTL14, METTL16, WTAP, VIRMA, ZC3H13, RBM15, and RBM15B), demethylases (ALKBH5 and FTO), and recognition proteins (YTHDC1, YTHDC2, YTHDF1, YTHDF2, YTHDF3, HNRNPC, FMR1, LRPPRC, HNRNPA2B1, IGFBP1, IGFBP2, IGFBP3, and RBMX) (Arguello et al., 2017; Huang et al., 2021a). Pearson correlation analysis was performed between the m6A genes and all lncRNAs. Absolute correlation coefficients >0.4 and p values <0.001 were used to define m6A-related lncRNAs. Univariate Cox regression analysis was used to screen for m6A-related lncRNAs significantly associated with overall survival (OS) (p < 0.01).
Unsupervised Clustering of Seven m6A-Related lncRNAs Associated With Prognosis
Unsupervised clustering analysis was used to classify patients with early-stage CRC into different subgroups based on the expression of the seven m6A-related lncRNAs associated with prognosis. The ConsensusClusterPlus package was used to perform the above steps and was repeated 1,000 times to ensure the stability of the classification (Wilkerson and Hayes, 2010). Then, we used the Euclicean disease to compute the similarity disease between patients with early-stage CRC. The optimal number of clusters was identified by CDF and consensus matrices.
GSEA (version 4.1.0) software was applied to determine the gene expression in cluster1 and cluster2 in the Molecular Signatures database (MSigDB) Collection (c2.cp.kegg. v7.2symbols.gmt) to further analyze the difference in KEGG pathway enrichment. The threshold of statistical significance was defined by a nominal p-value <0.05 and a false discovery rate (FDR) q value <0.25 (Subramanian et al., 2005).
Immune Characteristics for Molecular Subtypes of Early-Stage CRC
The ESTIMATE algorithm was used to calculate the ESTIMATE score, stromal score, and immune score between cluster 1 and cluster 2 (Gentles et al., 2015). The CIBERSORT algorithm, which can sensitively and specifically distinguish 22 human immune cell phenotypes, was used to quantify the immune cells in early-stage CRC samples. The Wilcoxon signed-rank test was used to analyze the differences in immune infiltrating cells between the different molecular subtypes using the above method. In addition, levels of checkpoint gene expression were also compared. Furthermore, the relationship between PD1 and m6A-related lncRNAs associated with prognosis was quantified using Pearson correlation analysis. The thresholds were set as p-value < 0.05.
Establishment and Validation of the m6A-Related lncRNA Risk Prognosis Model
First, patients with early-stage CRC were randomly divided into training and test cohorts at a ratio of 1:1 using the caret package. Second, least absolute shrinkage and selection operator (LASSO) Cox regression analysis was performed to analyze the best candidates and multiple m6A-related lncRNA characteristics for constructing the prognostic signature. Ten-fold cross-validation was used to prevent overfitting. Based on the above model, the risk score for each patient was calculated as follows: risk score =
Construction of a Predictive Nomogram for OS in Patients With Early-Stage CRC
Nomograms are widely used to predict the outcomes of patients with cancer (Iasonos et al., 2008). Risk scores and clinical indicators were incorporated into a nomogram to evaluate the probability of 1, 2, and 3 years OS in patients with early-stage CRC using “rms” R package. The C-index, ROC curves, and calibration curves (by a bootstrap method with 1,000 peplicates) were used to evaluate the predictive ability and discriminative value of the nomogram.
Chemosensitivity Prediction
The Cancer Drug Sensitivity Genomics (GDSC) database (https://cancerrxgene.org) can be used for large-scale drug screening. Combined with genomic analysis, the response of tumors to chemotherapy drugs can be systematically identified. Using the GDSCdatabase, the half-maximal inhibitory concentration (IC50) of common chemotherapeutic drugs for gastrointestinal tumors (camptothecin, cisplatin, rapamycin, bryostatin, and methotrexate) was calculated to evaluate the clinical application of this model in the treatment of early-stage CRC. The R package used in the above operation is “pRRophetic”. Then, we compared the difference in the IC50 between the high-risk group and low-risk group using the Wilcoxon signed-rank test. To visualize the data, box drawings were created using “pRRophetic” and “ggplot2” in R (Slavin, 1989).
Statistical Analysis
R software (v 4.0.3) was used for statistical analysis (R packages: “limma,” “pheatmap,” “survival,” “survminer,” “glmnet,” “reshape2,” “ggpubr,” “ConsensusClusterPlus,” “ggplot2,” “corrplot,” “utils,” “vioplot,” “plyr,” “grid,” “gridExtra (multi-GSEA),” “pRRophetic,” “caret,” “glmnet,” “timeROC,” “ggExtra”, “survcomp”). In the above statistical tests, a two-sided p-value <0.05 was considered statistically significant.
Results
Identification of m6A-Related lncRNAs Associated With Prognosis in Early-Stage CRC
The bioinformatic analysis of this study was performed as depicted in Figure 1. First, a total of 14,081 lncRNA expression and 23 m6A-related gene expression profiles were obtained from 299 early-stage CRC and 31 normal samples. The corresponding clinical data of 251 patients were also extracted from TCGA (Table 1). Then, 1,478 m6A-related lncRNAs were identified and seven survival-associated m6A-related lncRNAs were obtained (Figure 2A). The expression levels of these lncRNAs differed significantly between normal and tumor tissues (p < 0.05, Figures 2B,C). Expression levels of the three m6A-related lncRNAs (LINC00562, AC007991.4, and AL121583.1) in tumor tissue were significantly higher than those in normal tissues, whereas the expression levels of the remaining four lncRNAs (EPS15-AS1, AC087277.2, AC008494.3, and AC244629.1) were higher in normal tissues.
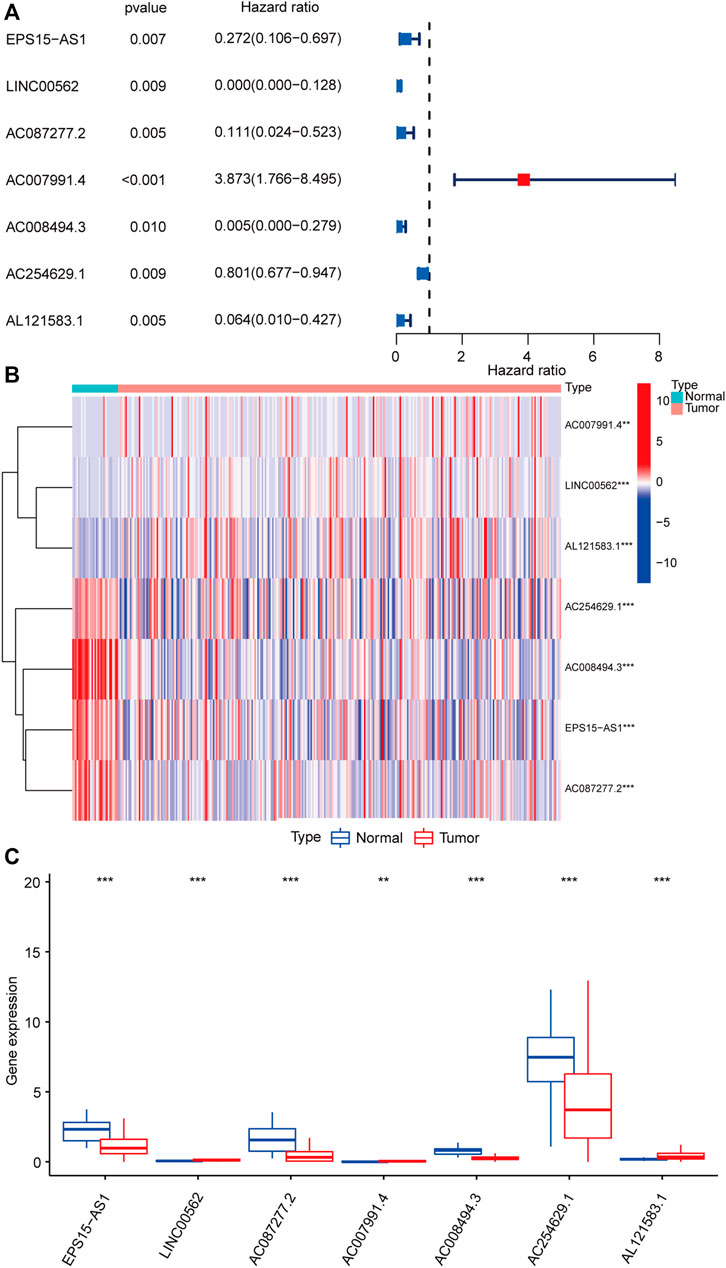
FIGURE 2. Identification of m6A-related lncRNAs associated with the prognosis of early-stage CRC. Forest plot of seven m6A-related lncRNAs associated with prognosis based on univariate Cox regression analysis. Red indicated that the m6a-related lncRNA is a risk factor for the prognosis of patients with early-stage CRC, and blue indicated that m6a-related lncRNA is a protective factor for the prognosis of patients with early-stage CRC (A). Heatmap of seven differentially expressed m6A-related lncRNAs between normal colorectal tissue (marked in green) and early-stage CRC (marked in red) (B). Expression patterns of seven m6A-related lncRNAs in normal tissue (marked in blue) and tumor tissue (marked in red) (C). *p < 0.05, **p < 0.01, and ***p < 0.001. Abbreviation: m6A-related lncRNAs, N6-methyladenosine-related long non-coding RNAs; CRC, colorectal cancer.
Construction of Two Molecular Subgroups of Early-Stage CRC Using Seven Survival-Associated m6A-Related lncRNAs
Unsupervised clustering identified two molecular subgroups as the optimal number of clusters, including 171 cases in cluster one and 80 cases in cluster two (Figure 3A, Supplementary Figure S1). Principal component analysis (PCA) was conducted based on the above classification methods, and there was a significant difference in the distributions of cluster one and cluster two (Figure 3B). The KEGG results revealed that “aminoacyl tRNA biosynthesis,” “antigen processing and presentation,” “cell cycle,” “cysteine and methionine metabolism,” “natural killer cell-mediated cytotoxicity,” “protein export,” and “ubiquitin-mediated proteolysis” were markedly enriched in cluster 1 (Figure 3C). Furthermore, the prognosis of cluster 1 was poorer than that of cluster 2 (Figure 3D). These results indicate that there are molecular subgroups of early-stage CRC with different characteristics.
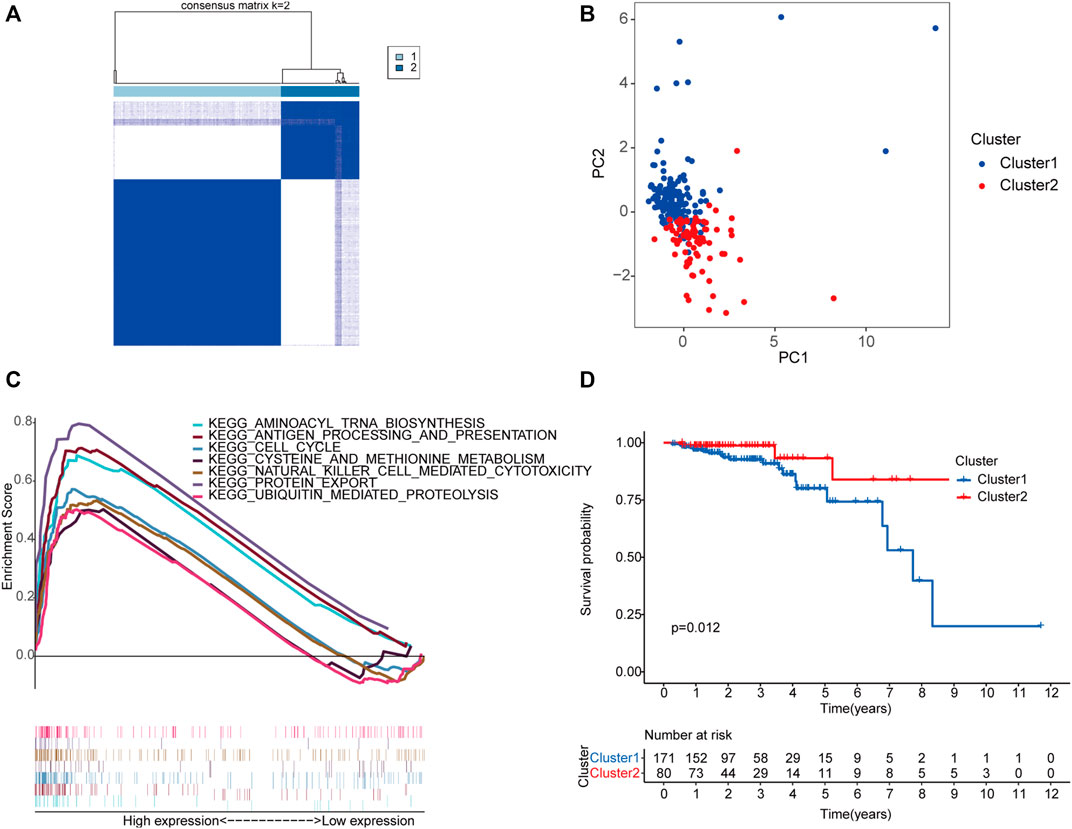
FIGURE 3. Construction of two molecular subgroups of early-stage CRC using seven m6A-related lncRNAs associated with prognosis. The consensus matrices of the TCGA database for k = 2 (A). PCA between cluster one (marked in blue) and cluster two (marked in red) (B). GSEA analysis of the different activation status of the biological pathways between cluster one and cluster 2 (C). Survival analysis of two subgroups (blue represented cluster 1, and red represented cluster 2) based on data from 251 patients with early-stage CRC in the TCGA cohort (D). Abbreviation: CRC, colorectal cancer; m6A-related lncRNAs, N6-methyladenosine-related long non-coding RNAs; PCA, principal component analysis; GSEA, gene set enrichment analysis; TCGA, The Cancer Genome Atlas.
Immune Characteristics of the Two Molecular Subgroups of Early-Stage CRC
In addition to tumor cells, the tumor microenvironment includes immune cells and stromal cells, which provide protection and support for the occurrence and development of tumors. As shown in Figure 4A, no significant differences in ESTIMATE or stromal scores were observed between cluster 1 and cluster 2; however, immune scores were higher in cluster 1 than in cluster two (p = 0.024), indicating that levels of immune cells differ based on molecular subgroup. Our analysis revealed enrichment of the proportion of M2 macrophages in cluster one, as well as a higher proportion of memory B cells in cluster two (Figure 4B). We also examined differences in the expression profiles of immune checkpoint genes between clusters 1 and 2. Levels of most immune checkpoint genes were higher in cluster 1 than in cluster two, suggesting that patients in cluster 1 were more likely to exhibit immune escape and poor prognosis (Figure 4C). We also observed that the expression of PD1 was positively correlated with AC087277.2 and AC007991.4 yet negatively correlated with AC254629.1 (Figure 4D).
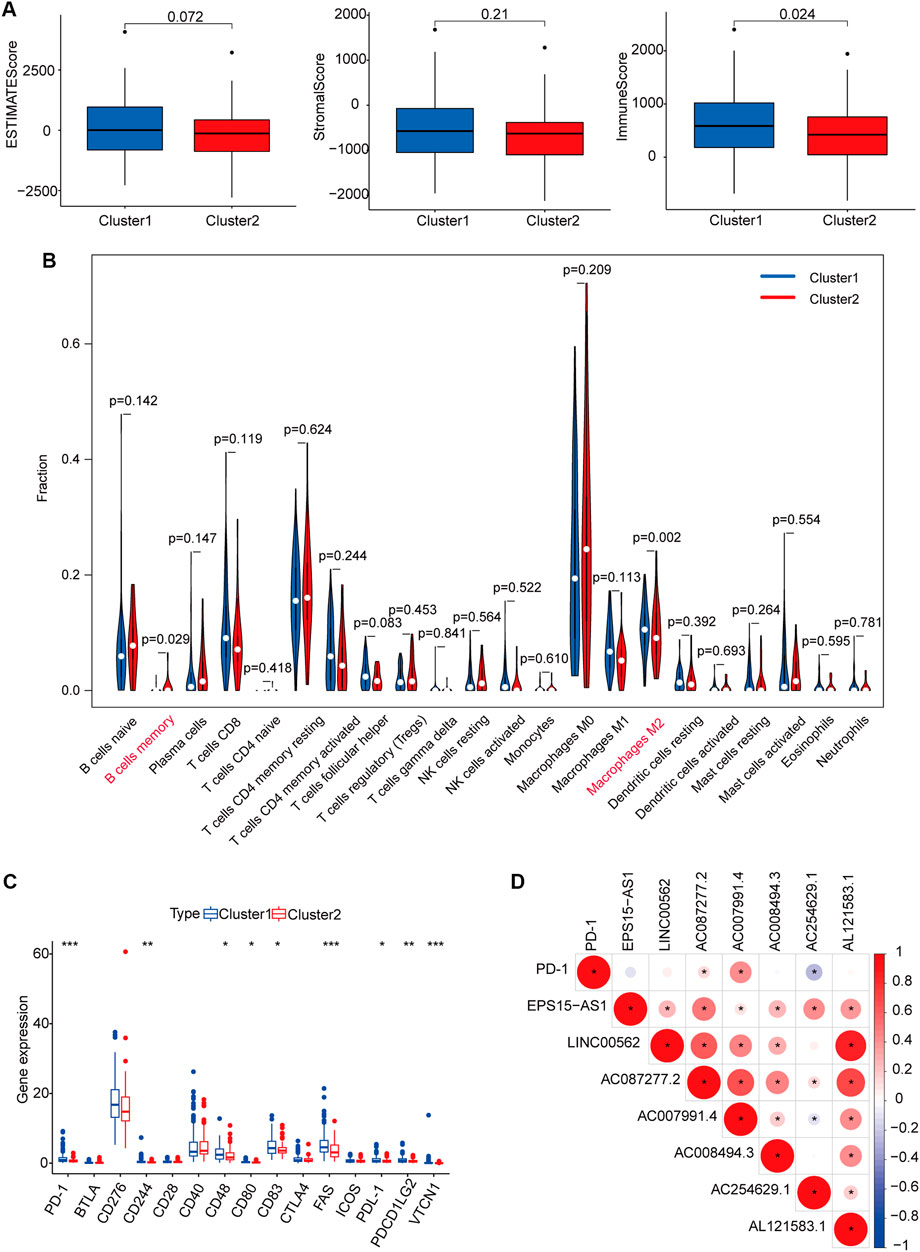
FIGURE 4. Immune cell infiltration and biological functions in the two subgroups. ESTIMATE, stromal, and immune scores in cluster 1 (marked in blue) and cluster 2 (marked in red) (A). Abundance of 22 immune cells in the two subgroups (blue represented cluster 1, and red represented cluster 2) (B). Expression of checkpoint genes in the two subgroups (Blue represented cluster 1, and red represented cluster 2). *p < 0.05, **p < 0.01, and ***p < 0.001 (C). Heatmap for the correlation between PDL1 gene and seven prognostic m6A-related lncRNAs. Blue represented a positive correlation between the PD-1 gene and the m6A-related lncRNA, and red represented a negative correlation between the PD-1 gene and the m6A-related lncRNA. The darker the color, the stronger the relevance (D). *p < 0.05. Abbreviation: m6A-related lncRNAs, N6-methyladenosine-related long non-coding RNAs.
Construction and Validation of the m6A-Related lncRNA Prognostic Signature
The 251 patients were randomly divided into a training cohort (n = 127) and a test cohort (n = 124) in a 1:1 ratio. To build the m6A-related lncRNA signature for forecasting the prognosis of patients with early-stage CRC, LASSO Cox analysis was performed based on the seven m6A-related lncRNAs in the training cohort. The m6A-related lncRNA signature included five m6A-related lncRNAs and the coefficients of each (Figures 5A,B). Based on the coefficients, the risk scores of each patient were calculated using the following formula: risk score = –0.828 × AC087277.2 + 0.989 × AC007991.4–5.396 × AC008494.3–0.151 × AC254629.1–1.435 × AL121583.1. Patients in the training cohort were grouped into low-risk and high-risk groups according to the median value of the risk scores. The Kaplan–Meier survival curve showed that patients with early-stage CRC with higher risk scores had poorer clinical outcomes than those with relatively lower risk scores (Figure 5C). The ROC curve showed that the m6A-related lncRNA signature had a good ability to predict OS in the training cohort (1 year AUC = 0.929, 2-years AUC = 0.954, 3-years AUC = 0.841; Figure 5E). Consistent with the results in the training cohort, higher risk scores were associated with shorter OS times and lower OS rates in the test cohort (Figure 5D). The ROC analysis also revealed that the m6A-related lncRNA signature had a strong prognostic value in the test cohort (1 year AUC = 0.664, 2 years AUC = 0.760, 3 years AUC = 0.754; Figure 5F). The distributions of risk, survival status, and expression of five m6A-related lncRNAs in the training and test cohorts are shown in Figure 6, which suggests that patients with higher risk scores exhibited shorter OS. Univariate and multivariate Cox regression analyses show that risk scores were significantly related to OS, independent of clinical parameters (Figures 7A–D).
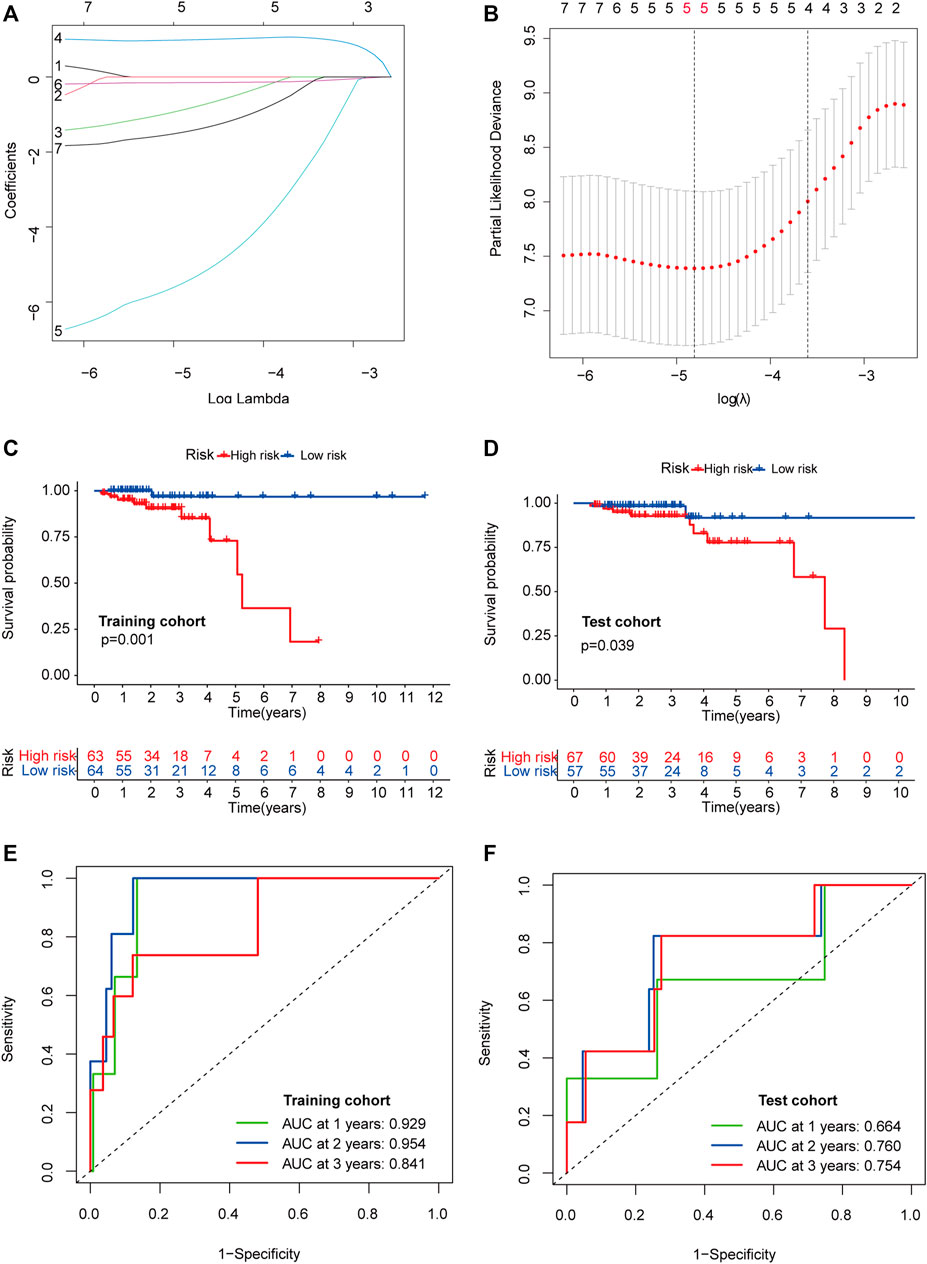
FIGURE 5. Construction and validation of the prognostic risk model. LASSO coefficient profiles of seven candidates in the training cohort (A). Selection of the optimal parameter (lambda) in the LASSO model (B). Survival analysis in the high- (marked in red) and low-risk groups (marked in blue) in the training cohort (C) and test cohort (D). Time-dependent ROC analysis for 1, 2, and 3-years OS prediction among patients with early-stage CRC in the training (E) and test cohorts (F). Abbreviation: LASSO, least absolute shrinkage and selection operator; ROC, receiver operating characteristic; OS, overall survival; CRC, colorectal cancer.
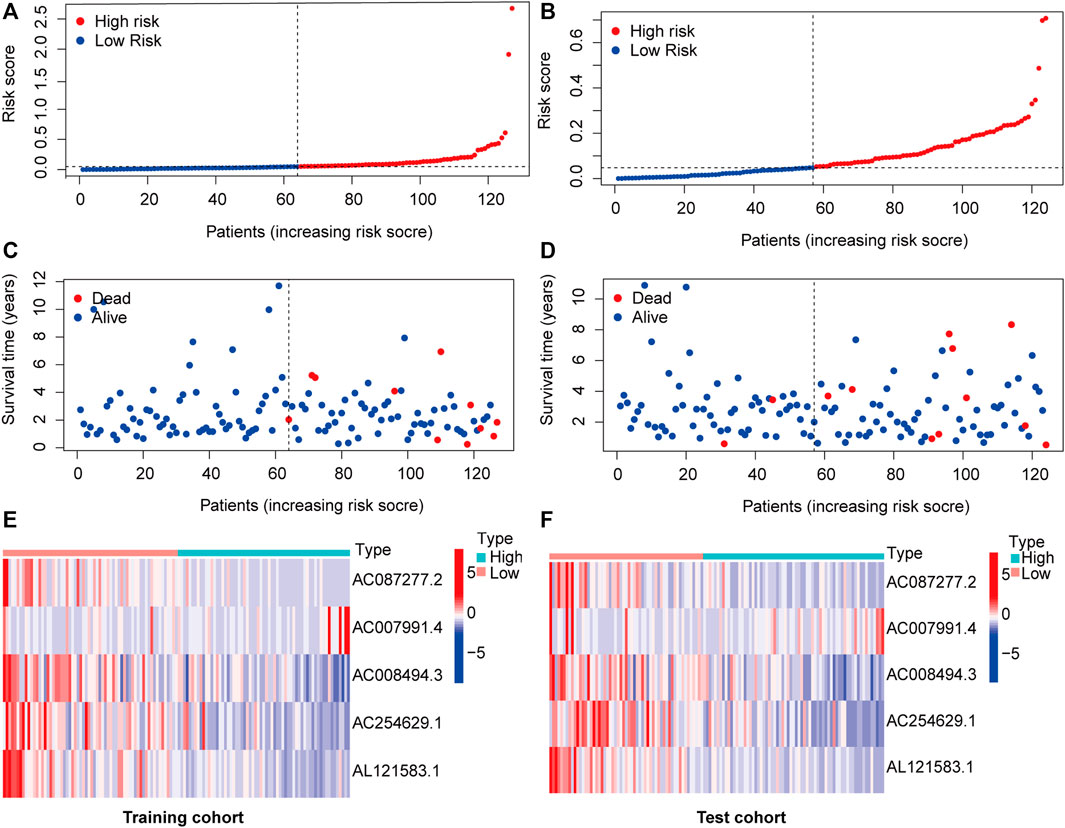
FIGURE 6. m6A-related lncRNA signature risk score analysis. Risk curves of each sample reordered by risk scores in the training cohort (A) and test cohort (B). Living status of patients with early-stage CRC in the training cohort (C) and test cohort (D). Heatmap of five m6A-related lncRNA expression profiles in the low- (marked in red) and high-risk groups (marked in green) in the training cohort (D) and test cohort (F). Abbreviation: m6A-related lncRNAs, N6-methyladenosine-related long non-coding RNAs; CRC, colorectal cancer.
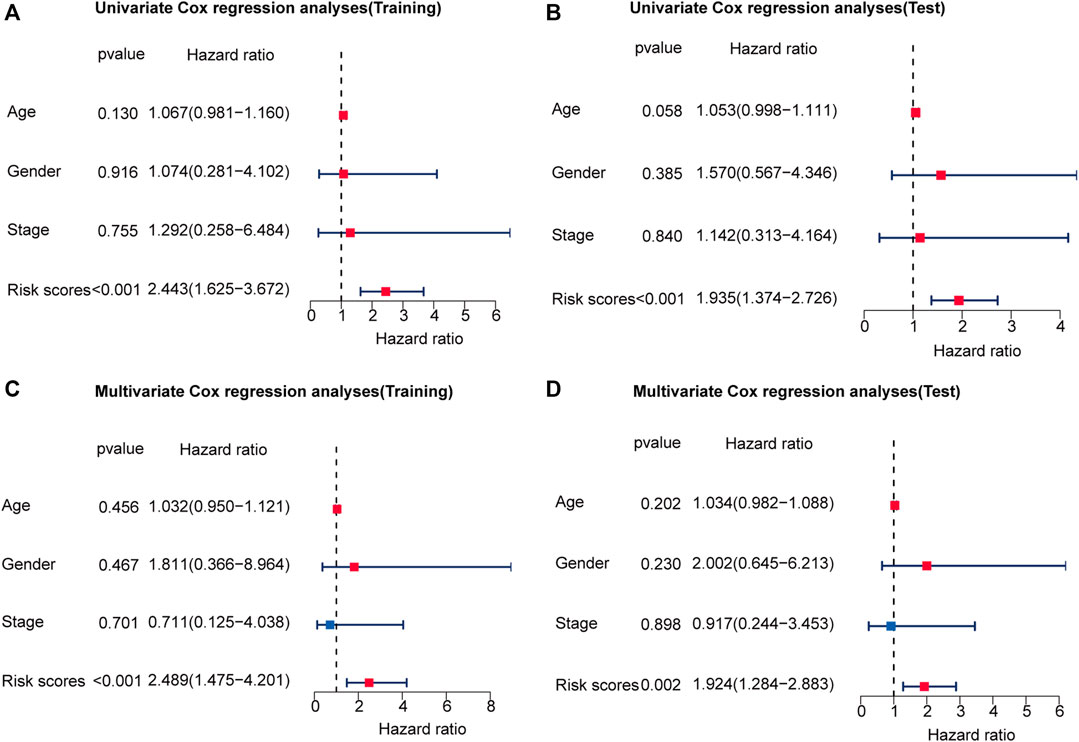
FIGURE 7. The risk score is an independent prognostic factor for early-stage CRC. Univariate regression analysis of early-stage CRC in the training cohort (A) and test cohort (B). Multivariate Cox regression analysis of early-stage CRC in the training cohort (C) and test cohort (D). Red indicated that the indicator is a risk factor for the prognosis of patients with early-stage CRC, and blue indicated that the indicator is a protective factor for patients with early-stage CRC. And p-value < 0.05 was considered statistically significant.
Construction of the Nomogram Based on the m6A-Related lncRNA Signature
To develop a clinically applicable method for predicting OS in patients with early-stage CRC, we built a nomogram using age, sex, stage, and risk scores (Figure 8A). The C-index of the nomogram for predicting the OS rate was 0.821. The nomogram had excellent accuracy regarding the 1, 2, and 3-years OS rates (AUC = 0.860, 0.873, and 0.842, respectively; Figure 8B). Moreover, the calibration curve demonstrated that the nomogram was suitable for clinical practice (Figure 8C).
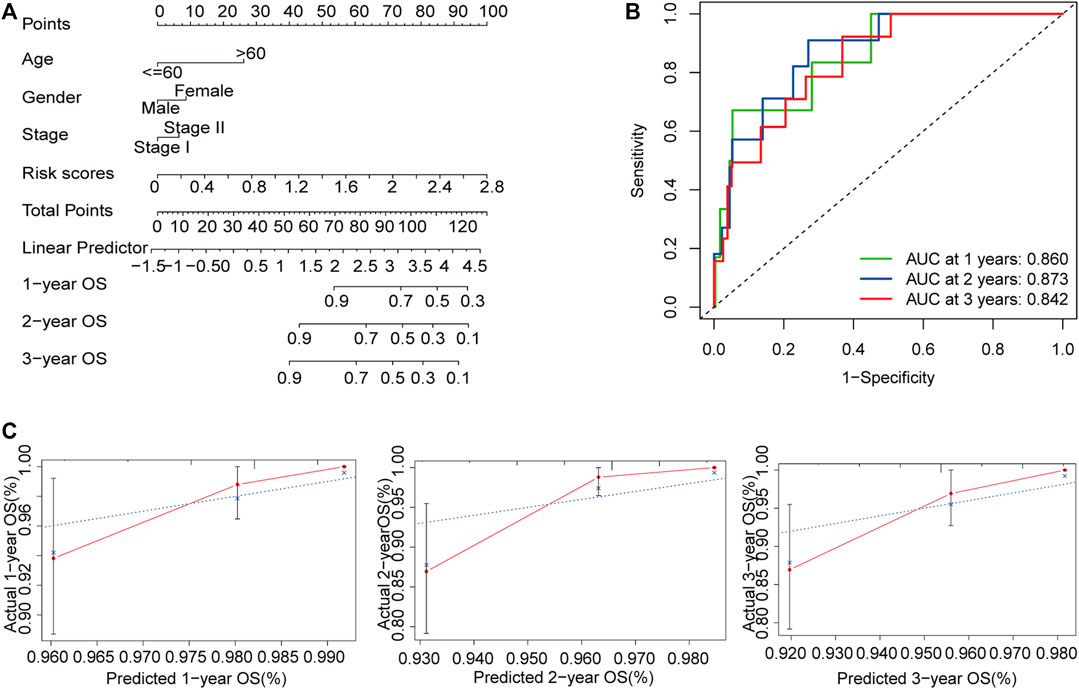
FIGURE 8. Construction of a nomogram for the prediction of outcomes in patients with early-stage CRC. The nomogram combining clinical features and the m6A-related lncRNA signature (A). ROC curves for the nomogram for predicting 1, 2, and 3-years OS (B). Calibration plot showing that the predicted probability and the actual probability were consistent (C). Abbreviation: m6A-related lncRNAs, N6-methyladenosine-related long non-coding RNAs; CRC, colorectal cancer; ROC, receiver operating characteristic; OS, overall survival.
Response to Chemotherapy in the High and Low-Risk Groups
To identify potential chemotherapeutic drugs targeting the m6A-related lncRNA signature for treating patients with early-stage CRC, the pRRophetic algorithm was used to estimate the therapeutic response based on the IC50 for each sample. We found that the high-risk group was more sensitive to camptothecin and cisplatin, which may provide insight into new treatment options for patients with early-stage CRC (Figures 9A–D).
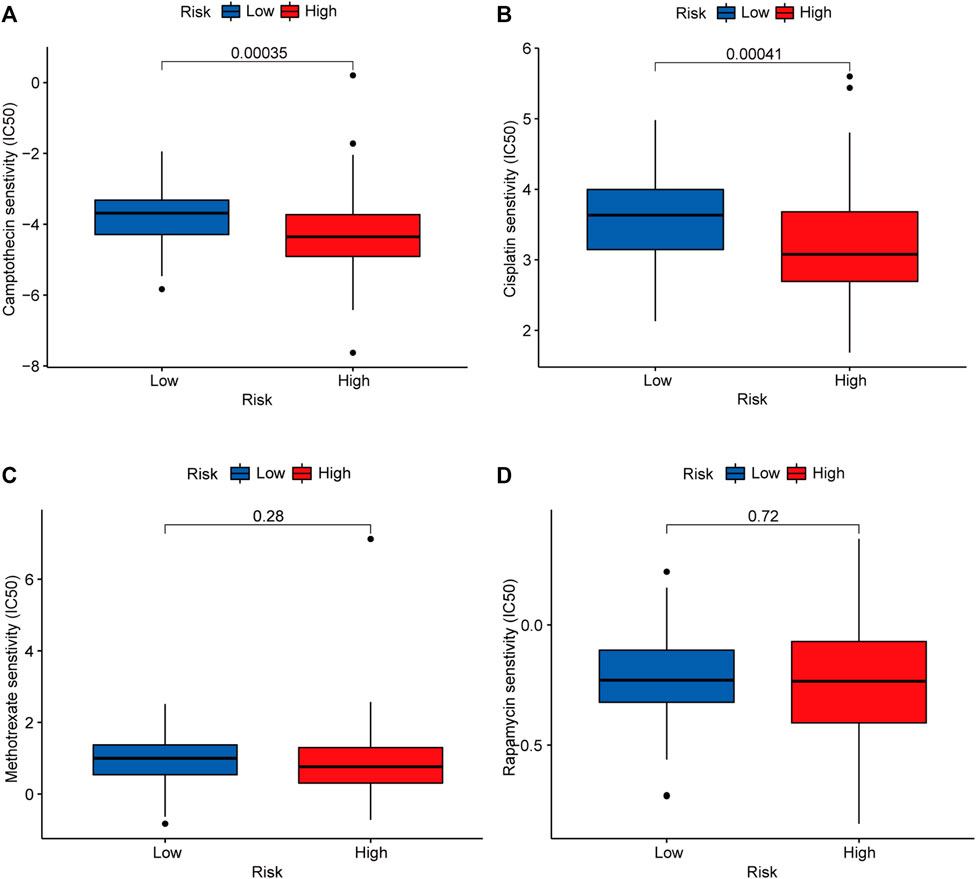
FIGURE 9. Candidate chemotherapeutic drugs targeting the m6A-related lncRNA signature. IC50s of camptothecin (A), cisplatin (B), methotrexate (C), and rapamycin (D) between the low- (marked in blue) and high-risk groups (marked in red). Abbreviation: m6A-related lncRNAs, N6-methyladenosine-related long non-coding RNAs.
Discussion
Even after radical surgical treatment, the risk of recurrence in patients with stage I and stage II CRC is still 5 and 20%, respectively, (Dotan and Cohen, 2011). Conventional high-risk characteristics, such as MSI status and bowel obstruction, cannot meet the demands for predicting prognosis in patients with early-stage CRC. However, numerous studies have highlighted the relevance of lncRNAs. Specific lncRNAs can be modified by m6A regulators to participate in tumorigenesis, development, and metastasis (Tu et al., 2020). In the present study, we extracted data regarding the expression of lncRNA and m6A in these patients from TCGA. Seven m6A-associated lncRNAs related to prognosis were identified using univariate Cox regression analysis. Based on these lncRNAs, we identified two molecular subgroups of early-stage CRC with unique immune characteristics. Furthermore, we constructed and validated a novel m6A-related lncRNA model for predicting OS in patients with early-stage CRC, following which we developed a nomogram for clinical application in this patient population. Finally, we assessed responses to common chemotherapeutic drugs in the high- and low-risk groups.
Here, based on seven m6A-related lncRNAs associated with prognosis, we identified two molecular subgroups of early-stage CRC with significantly distinct features. Prognosis was worse for cluster 1 than for cluster two, and cluster 1 was characterized by increased numbers of M2 macrophages, decreased numbers of memory B cells, and increased expression of checkpoint genes when compared with cluster two. Tumor-associated macrophages are comprised of M1 and M2 macrophages (Mantovani et al., 2017). It is well known that M1 (anti-tumor) and M2 (pro-tumor) phenotypes are related to different immunomodulatory functions (Yang et al., 2018b). In a previous study, Catherine et al. inferred that B lymphocytes may exert anti-tumor immune functions by collaborating with T lymphocytes (Sautès-Fridman et al., 2016). Edin et al. also found that tumor-infiltrating CD20+ B lymphocytes are associated with a favorable prognosis in CRC (Edin et al., 2019). Thus, cluster 1 may be associated with immunosuppression. Furthermore, expression levels of immune checkpoint genes were higher in cluster 1 than in cluster two, which is a characteristic of immunosuppression. This finding suggests that patients in cluster 1 were more likely to exhibit immune escape and poor prognosis than those in cluster two.
It has been reported that m6A-related lncRNAs are involved in the occurrence and development of a variety of tumors. For example, m6A on lncRNA NEAT1-1 may be a novel specific marker for bone metastasis and is correlated with poor prognosis (Wen et al., 2020). METL3 and METTL14 on LNCAROD have tumor-promoting functions in the development of head and neck squamous cell carcinoma (Ban et al., 2020). Moreover, m6A-related lncRNAs can reliably predict the prognosis in several tumors such as metastatic skin cutaneous melanoma and adrenocortical carcinoma (Huang et al., 2021b; Jin et al., 2021). Similar to these studies, we conducted a series of bioinformatics analyses to generate a new m6A-related lncRNA signature for early-stage CRC prognosis. The signature included AC087277.2, AC007991.4, AC008494.3, AC254629.1, and AL121583.1. The construction of a novel signature allowed us to distinguish samples at different risks. In the training cohort, the OS time of early-stage CRC was shorter in the high-risk group than in the low-risk group, and consistent results were obtained in the test cohort. In addition, univariate and multivariate Cox regression analyses confirmed that the risk score was an independent predictor of OS in patients with early-stage CRC. Taken together, these results highlight the ability of our m6A-related lncRNA prognostic signature to accurately predict OS in this population.
High-risk patients with early-stage CRC are usually treated via a combination of surgery and chemotherapy. Using the GDSC database, we found that high-risk patients were more sensitive to commonly used chemotherapy drugs (including camptothecin and cisplatin) than low-risk patients, which may provide new treatment options for patients with early-stage CRC. Camptothecin is an alkaloid extracted from Camptotheca acuminata that can inhibit the catalytic activity of topoisomerase (Hsiang et al., 1985; Mollica et al., 2012). In agreement with previous reports (Cross-Knorr et al., 2013), camptithecin can improve patients with early-stage CRC prognosis by inhibiting PKIP phosphorylation and STAT3 activation. Cisplatin is one of the main chemotherapy drugs used by oncologists to treat CRC (AlShamaileh et al., 2017). It has antitumor effects by binding to DNA and inducing DNA damage (Huang et al., 2019). More clinical trials are needed to explore the applicability of these two chemotherapeutic agents in patients with early-stage CRC.
However, our study had several limitations. First, this study was based on the TCGA database, and there was no external data set for verification. When searching for other data sets, such as the Gene Expression Omnibus, we were unable to locate one with both clinical information and the corresponding lncRNA and mRNA expression data. Future studies should use other data sets to verify the performance of our prognostic signature. Second, our findings must be verified in vivo and in vitro. Lastly, we did not explore the underlying mechanism of the studied m6A-related lncRNAs. Therefore, functional studies should examine these five lncRNAs individually and in combination to further verify the prediction accuracy of the signature and discover potential regulatory mechanisms. Despite these limitations, to the best of our knowledge, this is the first study to report an established external verification of m6A-related lncRNA signatures for early-stage CRC.
Conclusion
In summary, our analysis of seven m6A-related lncRNAs associated with prognosis identified two molecular subgroups of early-stage CRC with unique immune features. Subsequent analyses demonstrated the usefulness of a five m6A-related lncRNA signature as a potential indicator of prognosis in patients with early-stage CRC. Further studies are required to explore the mechanisms underlying the association between the significant m6A-related lncRNAs and CRC prognosis and to verify the applicability of our prognostic signature.
Data Availability Statement
The original contributions presented in the study are included in the article/Supplementary Material further inquiries can be directed to the corresponding author.
Ethics Statement
The studies involving human participants were reviewed and approved by the Medical Ethics Committee of the Sixth Affiliated Hospital of Sun Yat-Sen University. The patients/participants provided their written informed consent to participate in this study.
Author Contributions
ZX analyzed the data and conducted the experiments, XL designed the study, and SY wrote the articles. MX conducted the experiments and critically revised the articles. CM, FZ, HC, and LJ analyzed the data and critically revised the articles. LL participated in the conception of the study, designed the study, and critically revised the articles All authors contributed to the articles and approved the submitted version.
Funding
This study was supported by the National Natural Science Foundation of China (grant nos. 81770557 and 82070684) and the Guangdong Natural Science Fund for Outstanding Youth Scholars (grant no. 2020B151502067).
Conflict of Interest
The authors declare that the research was conducted in the absence of any commercial or financial relationships that could be construed as a potential conflict of interest.
Publisher’s Note
All claims expressed in this article are solely those of the authors and do not necessarily represent those of their affiliated organizations, or those of the publisher, the editors and the reviewers. Any product that may be evaluated in this article, or claim that may be made by its manufacturer, is not guaranteed or endorsed by the publisher.
Acknowledgments
We thank all staff members of the Department of Colorectal Surgery at the Sixth Affiliated Hospital of Sun Yat-sen University.
Supplementary Material
The Supplementary Material for this article can be found online at: https://www.frontiersin.org/articles/10.3389/fmolb.2021.724889/full#supplementary-material
Abbreviations
CRC, colorectal cancer; GSEA, gene set enrichment analysis; IC50, half-maximal inhibitory concentration; LASSO, least absolute shrinkage and selection operator; lncRNA, non-coding RNA; m6Arelated lncRNAs, N6-methyladenosine-related long non-coding RNAs; m6A, N6-methyladenosine; miRNA, microRNAs; mRNAs, messenger RNAs; MSI, microsatellite instability; OS, overall survival; PD1, programmed cell death protein 1; ROC, receiver operating characteristic curve; TCGA, The Cancer Genome Atlas.
References
AlShamaileh, H., Wang, T., Xiang, D., Yin, W., Tran, P. H.-L., Barrero, R. A., et al. (2017). Aptamer-mediated Survivin RNAi Enables 5-fluorouracil to Eliminate Colorectal Cancer Stem Cells. Sci. Rep. 7 (1), 5898. doi:10.1038/s41598-017-05859-z
Arguello, A. E., DeLiberto, A. N., and Kleiner, R. E. (2017). RNA Chemical Proteomics Reveals the N6-Methyladenosine (m6A)-Regulated Protein-RNA Interactome. J. Am. Chem. Soc. 139 (48), 17249–17252. doi:10.1021/jacs.7b09213
Ban, Y., Tan, P., Cai, J., Li, J., Hu, M., Zhou, Y., et al. (2020). LNCAROD Is Stabilized by m6A Methylation and Promotes Cancer Progression via Forming a Ternary Complex with HSPA1A and YBX1 in Head and Neck Squamous Cell Carcinoma. Mol. Oncol. 14 (6), 1282–1296. doi:10.1002/1878-0261.12676
Cai, X., Wang, X., Cao, C., Gao, Y., Zhang, S., Yang, Z., et al. (2018). HBXIP-elevated Methyltransferase METTL3 Promotes the Progression of Breast Cancer via Inhibiting Tumor Suppressor Let-7g. Cancer Lett. 415, 11–19. doi:10.1016/j.canlet.2017.11.018
Colangelo, T., Polcaro, G., Muccillo, L., D'Agostino, G., Rosato, V., Ziccardi, P., et al. (2017). Friend or Foe? Biochim. Biophys. Acta (Bba) - Rev. Cancer 1867, 1–18. doi:10.1016/j.bbcan.2016.11.001
Cross-Knorr, S., Lu, S., Perez, K., Guevara, S., Brilliant, K., Pisano, C., et al. (2013). RKIP Phosphorylation and STAT3 Activation Is Inhibited by Oxaliplatin and Camptothecin and Are Associated with Poor Prognosis in Stage II colon Cancer Patients. BMC Cancer 13, 463. doi:10.1186/1471-2407-13-463
Cui, Q., Shi, H., Ye, P., Li, L., Qu, Q., Sun, G., et al. (2017). m 6 A RNA Methylation Regulates the Self-Renewal and Tumorigenesis of Glioblastoma Stem Cells. Cel Rep. 18 (11), 2622–2634. doi:10.1016/j.celrep.2017.02.059
Desrosiers, R., Friderici, K., and Rottman, F. (1974). Identification of Methylated Nucleosides in Messenger RNA from Novikoff Hepatoma Cells. Proc. Natl. Acad. Sci. 71 (10), 3971–3975. doi:10.1073/pnas.71.10.3971
Dienstmann, R., Salazar, R., and Tabernero, J. (2015). Personalizing colon Cancer Adjuvant Therapy: Selecting Optimal Treatments for Individual Patients. Jco 33 (16), 1787–1796. doi:10.1200/JCO.2014.60.0213
Dotan, E., and Cohen, S. J. (2011). Challenges in the Management of Stage II colon Cancer. Semin. Oncol. 38 (4), 511–520. doi:10.1053/j.seminoncol.2011.05.005
Edin, S., Kaprio, T., Hagström, J., Larsson, P., Mustonen, H., Böckelman, C., et al. (2019). The Prognostic Importance of CD20+ B Lymphocytes in Colorectal Cancer and the Relation to Other Immune Cell Subsets. Sci. Rep. 9 (1), 19997. doi:10.1038/s41598-019-56441-8
Ganesh, K., Stadler, Z. K., Cercek, A., Mendelsohn, R. B., Shia, J., Segal, N. H., et al. (2019). Immunotherapy in Colorectal Cancer: Rationale, Challenges and Potential. Nat. Rev. Gastroenterol. Hepatol. 16 (6), 361–375. doi:10.1038/s41575-019-0126-x
Gentles, A. J., Newman, A. M., Liu, C. L., Bratman, S. V., Feng, W., Kim, D., et al. (2015). The Prognostic Landscape of Genes and Infiltrating Immune Cells across Human Cancers. Nat. Med. 21 (8), 938–945. doi:10.1038/nm.3909
Hsiang, Y. H., Hertzberg, R., Hecht, S., and Liu, L. F. (1985). Camptothecin Induces Protein-Linked DNA Breaks via Mammalian DNA Topoisomerase I. J. Biol. Chem. 260 (27), 14873–14878. doi:10.1016/s0021-9258(17)38654-4
Huang, L., Zhu, J., Kong, W., Li, P., and Zhu, S. (2021). Expression and Prognostic Characteristics of m6A RNA Methylation Regulators in Colon Cancer. Ijms 22 (4), 2134. doi:10.3390/ijms22042134
Huang, S., Lyu, S., Gao, Z., Zha, W., Wang, P., Shan, Y., et al. (2021). m6A-Related lncRNAs Are Potential Biomarkers for the Prognosis of Metastatic Skin Cutaneous Melanoma. Front. Mol. Biosci. 8, 687760. doi:10.3389/fmolb.2021.687760
Huang, Z., Zhang, Y., Li, H., Zhou, Y., Zhang, Q., Chen, R., et al. (2019). Vitamin D Promotes the Cisplatin Sensitivity of Oral Squamous Cell Carcinoma by Inhibiting LCN2-Modulated NF-Κb Pathway Activation through RPS3. Cell Death Dis 10 (12), 936. doi:10.1038/s41419-019-2177-x
Iasonos, A., Schrag, D., Raj, G. V., and Panageas, K. S. (2008). How to Build and Interpret a Nomogram for Cancer Prognosis. Jco 26 (8), 1364–1370. doi:10.1200/JCO.2007.12.9791
Jin, Y., Wang, Z., He, D., Zhu, Y., Hu, X., Gong, L., et al. (2021). Analysis of m6A-Related Signatures in the Tumor Immune Microenvironment and Identification of Clinical Prognostic Regulators in Adrenocortical Carcinoma. Front. Immunol. 12, 637933. doi:10.3389/fimmu.2021.637933
Kannarkatt, J., Joseph, J., Kurniali, P. C., Al-Janadi, A., and Hrinczenko, B. (2017). Adjuvant Chemotherapy for Stage II Colon Cancer: A Clinical Dilemma. Jop 13 (4), 233–241. doi:10.1200/JOP.2016.017210
Kopetz, S., Tabernero, J., Rosenberg, R., Jiang, Z. Q., Moreno, V., Bachleitner‐Hofmann, T., et al. (2015). Genomic Classifier ColoPrint Predicts Recurrence in Stage II Colorectal Cancer Patients More Accurately Than Clinical Factors. The Oncologist 20 (2), 127–133. doi:10.1634/theoncologist.2014-0325
Li, N., Kang, Y., Wang, L., Huff, S., Tang, R., Hui, H., et al. (2020). ALKBH5 Regulates Anti-PD-1 Therapy Response by Modulating Lactate and Suppressive Immune Cell Accumulation in Tumor Microenvironment. Proc. Natl. Acad. Sci. USA 117 (33), 20159–20170. doi:10.1073/pnas.1918986117
Lin, S., Choe, J., Du, P., Triboulet, R., and Gregory, R. I. (2016). The M 6 A Methyltransferase METTL3 Promotes Translation in Human Cancer Cells. Mol. Cel 62 (3), 335–345. doi:10.1016/j.molcel.2016.03.021
Liu, S., Lin, H., Wang, D., Li, Q., Luo, H., Li, G., et al. (2019). PCDH17 Increases the Sensitivity of Colorectal Cancer to 5-fluorouracil Treatment by Inducing Apoptosis and Autophagic Cell Death. Sig Transduct Target. Ther. 4, 53. doi:10.1038/s41392-019-0087-0
Manfredi, S., Bouvier, A. M., Lepage, C., Hatem, C., Dancourt, V., and Faivre, J. (2006). Incidence and Patterns of Recurrence after Resection for Cure of Colonic Cancer in a Well Defined Population. Br. J. Surg. 93 (9), 1115–1122. doi:10.1002/bjs.5349
Mantovani, A., Marchesi, F., Malesci, A., Laghi, L., and Allavena, P. (2017). Tumour-associated Macrophages as Treatment Targets in Oncology. Nat. Rev. Clin. Oncol. 14 (7), 399–416. doi:10.1038/nrclinonc.2016.217
Molinie, B., and Giallourakis, C. C. (2017). Genome-Wide Location Analyses of N6-Methyladenosine Modifications (m6A-Seq). Methods Mol. Biol. 1562, 45–53. doi:10.1007/978-1-4939-6807-7_4
Mollica, A., Stefanucci, A., Feliciani, F., Cacciatore, I., Cornacchia, C., and Pinnen, F. (2012). Delivery Methods of Camptothecin and its Hydrosoluble Analogue Irinotecan for Treatment of Colorectal Cancer. Cdd 9 (2), 122–131. doi:10.2174/156720112800234558
Sautès-Fridman, C., Lawand, M., Giraldo, N. A., Kaplon, H., Germain, C., Fridman, W. H., et al. (2016). Tertiary Lymphoid Structures in Cancers: Prognostic Value, Regulation, and Manipulation for Therapeutic Intervention. Front. Immunol. 7, 407. doi:10.3389/fimmu.2016.00407
Siegel, R. L., Miller, K. D., and Jemal, A. (2019). Cancer Statistics, 2019. CA A. Cancer J. Clin. 69 (1), 7–34. doi:10.3322/caac.21551
Slavin, M. L. (1989). Metastatic Malignant Meningioma. J. Clin. Neuroophthalmol 9 (1), 55–59. doi:10.3109/01658108909019508
Subramanian, A., Tamayo, P., Mootha, V. K., Mukherjee, S., Ebert, B. L., Gillette, M. A., et al. (2005). Gene Set Enrichment Analysis: a Knowledge-Based Approach for Interpreting Genome-wide Expression Profiles. Proc. Natl. Acad. Sci. 102 (43), 15545–15550. doi:10.1073/pnas.0506580102
Tu, Z., Wu, L., Wang, P., Hu, Q., Tao, C., Li, K., et al. (2020). N6-Methylandenosine-Related lncRNAs Are Potential Biomarkers for Predicting the Overall Survival of Lower-Grade Glioma Patients. Front. Cel Dev. Biol. 8, 642. doi:10.3389/fcell.2020.00642
Vu, L. P., Pickering, B. F., Cheng, Y., Zaccara, S., Nguyen, D., Minuesa, G., et al. (2017). The N6-Methyladenosine (m6A)-Forming Enzyme METTL3 Controls Myeloid Differentiation of normal Hematopoietic and Leukemia Cells. Nat. Med. 23 (11), 1369–1376. doi:10.1038/nm.4416
Walker, B. S., Zarour, L. R., Wieghard, N., Gallagher, A. C., Swain, J. R., Weinmann, S., et al. (2020). Stem Cell Marker Expression in Early Stage Colorectal Cancer Is Associated with Recurrent Intestinal Neoplasia. World J. Surg. 44 (10), 3501–3509. doi:10.1007/s00268-020-05586-z
Wang, H., Meng, Q., and Ma, B. (2021). Characterization of the Prognostic m6A-Related lncRNA Signature in Gastric Cancer. Front. Oncol. 11, 630260. doi:10.3389/fonc.2021.630260
Wang, W., Li, J., Lin, F., Guo, J., and Zhao, J. (2021). Identification of N6-Methyladenosine-Related lncRNAs for Patients with Primary Glioblastoma. Neurosurg. Rev. 44 (1), 463–470. doi:10.1007/s10143-020-01238-x
Wen, S., Wei, Y., Zen, C., Xiong, W., Niu, Y., and Zhao, Y. (2020). Long Non-coding RNA NEAT1 Promotes Bone Metastasis of Prostate Cancer through N6-Methyladenosine. Mol. Cancer 19 (1), 171. doi:10.1186/s12943-020-01293-4
Wilkerson, M. D., and Hayes, D. N. (2010). ConsensusClusterPlus: a Class Discovery Tool with Confidence Assessments and Item Tracking. Bioinformatics 26 (12), 1572–1573. doi:10.1093/bioinformatics/btq170
Yang, M., McKay, D., Pollard, J. W., and Lewis, C. E. (2018). Diverse Functions of Macrophages in Different Tumor Microenvironments. Cancer Res. 78 (19), 5492–5503. doi:10.1158/0008-5472.CAN-18-1367
Yang, S., Wei, J., Cui, Y.-H., Park, G., Shah, P., Deng, Y., et al. (2019). m6A mRNA Demethylase FTO Regulates Melanoma Tumorigenicity and Response to Anti-PD-1 Blockade. Nat. Commun. 10 (1), 2782. doi:10.1038/s41467-019-10669-0
Yang, Y., Hsu, P. J., Chen, Y.-S., and Yang, Y.-G. (2018). Dynamic Transcriptomic m6A Decoration: Writers, Erasers, Readers and Functions in RNA Metabolism. Cell Res 28 (6), 616–624. doi:10.1038/s41422-018-0040-8
Zhang, Q., Cai, Y., Kurbatov, V., Khan, S. A., Lu, L., Zhang, Y., et al. (2020). Gene Alterations of N6-Methyladenosine (m6A) Regulators in Colorectal Cancer: A TCGA Database Study. Biomed. Res. Int. 2020, 1–13. doi:10.1155/2020/8826456
Keywords: early-stage colorectal cancer, long non-coding RNA, N6-methyladenosine, nomogram, prognosis
Citation: Xiong Z, Li X, Yin S, Xie M, Mao C, Zhang F, Chen H, Jin L and Lian L (2021) Prognostic Value of N6-Methyladenosine-Related lncRNAs in Early-Stage Colorectal Cancer: Association With Immune Cell Infiltration and Chemotherapeutic Drug Sensitivity. Front. Mol. Biosci. 8:724889. doi: 10.3389/fmolb.2021.724889
Received: 14 June 2021; Accepted: 28 September 2021;
Published: 12 October 2021.
Edited by:
Ismail Hosen, University of Dhaka, BangladeshReviewed by:
Siva Koganti, Stony Brook Medicine, United StatesTabish H. Khan, University of Virginia, United States
Copyright © 2021 Xiong, Li, Yin, Xie, Mao, Zhang, Chen, Jin and Lian. This is an open-access article distributed under the terms of the Creative Commons Attribution License (CC BY). The use, distribution or reproduction in other forums is permitted, provided the original author(s) and the copyright owner(s) are credited and that the original publication in this journal is cited, in accordance with accepted academic practice. No use, distribution or reproduction is permitted which does not comply with these terms.
*Correspondence: Lei Lian, bGlhbmxlaTJAbWFpbC5zeXN1LmVkdS5jbg==
†These authors contributed equally to this work