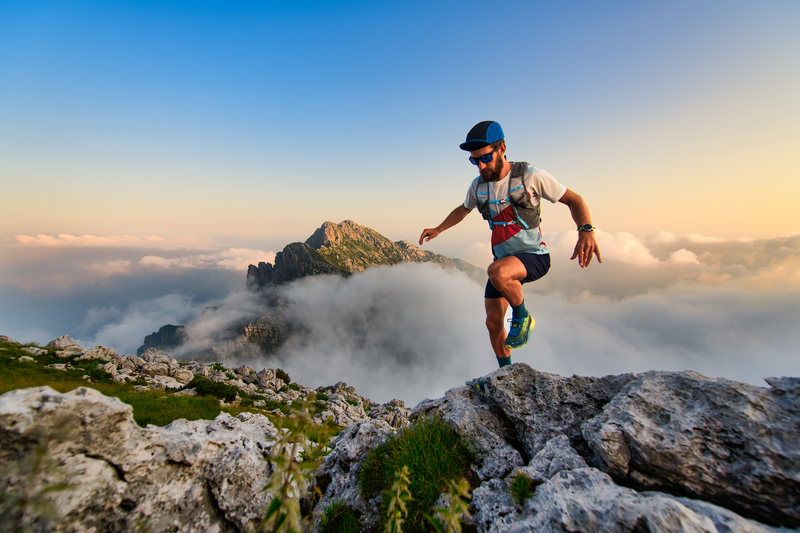
94% of researchers rate our articles as excellent or good
Learn more about the work of our research integrity team to safeguard the quality of each article we publish.
Find out more
ORIGINAL RESEARCH article
Front. Mol. Biosci. , 28 June 2021
Sec. Molecular Diagnostics and Therapeutics
Volume 8 - 2021 | https://doi.org/10.3389/fmolb.2021.692835
This article is part of the Research Topic Breast Cancer Resistance, Biomarkers and Therapeutics Development in the Era of Artificial Intelligence View all 12 articles
Pirin (PIR) protein is highly conserved in both prokaryotic and eukaryotic organisms. Recently, it has been identified that PIR positively regulates breast cancer cell proliferation, xenograft tumor formation, and metastasis, through an enforced transition of G1/S phase of the cell cycle by upregulation of E2F1 expression at the transcriptional level. Keeping in view the importance of PIR in many crucial cellular processes in humans, we used a variety of computational tools to identify non-synonymous single-nucleotide polymorphisms (SNPs) in the PIR gene that are highly deleterious for the structure and function of PIR protein. Out of 173 SNPs identified in the protein, 119 are non-synonymous, and by consensus, 24 mutations were confirmed to be deleterious in nature. Mutations such as V257A, I28T, and I264S were unveiled as highly destabilizing due to a significant stability fold change on the protein structure. This observation was further established through molecular dynamics (MD) simulation that demonstrated the role of the mutation in protein structure destability and affecting its internal dynamics. The findings of this study are believed to open doors to investigate the biological relevance of the mutations and drugability potential of the protein.
Pirin (PIR) protein is considered highly conserved in both prokaryotic and eukaryotic organisms; however, its biological functions are poorly described (Dunwell et al., 2001; Pang et al., 2004). Pirin is reported as a biomarker in breast cancer, which is abnormal and irregular proliferation of cells associated with inappropriate stimulation of pathways involved in signal transduction (Feitelson et al., 2015; Riaz et al., 2017; Chang et al., 2019). The crystal structure of the human PIR gene revealed its quercetinase (acts on quercetin flavonoid) and regulatory functions in many cellular pathways like an inhibitor of protein kinase, antioxidant as well as putative transcriptional co-factor (Chen et al., 2004; Wendler et al., 1997). Previous studies reported the overexpression of PIR in different neoplastic transformation and its role in the enhancement of tumor formation due to inducing the expression of Bcl3 by forming the ternary complex with proto-oncogenes Bcl3 and NF-kB (Zhu et al., 2003; Massoumi et al., 2009). Recently, it has been identified that PIR positively regulates breast cancer cell proliferation, xenograft tumor formation, and metastasis, through an enforced transition of G1/S phase of the cell cycle by upregulation of E2F1 expression at the transcriptional level (Suleman et al., 2019). It was a significant breakthrough in unveiling the hidden function of PIR in the field of cancer.
The most frequently occurring genetic variations are single-nucleotide polymorphisms (SNPs), which disturb both coding and non-coding regions of DNA. SNPs occur in every 200–300 bp in the human genome and consist of about 90% of the total genetic variations in the human genome. The nsSNPs (non-synonymous single-nucleotide polymorphisms) are the various mutations that occur in exonic regions and change the protein sequence, structure, and normal function by triggering modifications in the mechanism of transcription and translation.
Recently, various in silico computational tools, methods, and approaches were adopted to investigate the possible role of non-synonymous variation in protein structure and function efficiently and accurately (Kumar et al., 2009; Wadood et al., 2017; Muneer et al., 2019). These methods are of great interest to decipher important molecular mechanisms from protein–protein binding to drug development (Khan et al., 2020a; Khan et al., 2020b; Khan et al., 2020c; Khan et al., 2020d; Khan et al., 2021a; Khan et al., 2021b; Khan et al., 2021c). So far, a total of 173 SNPs comprising 119 missense mutations have been described in the human PIR gene and deposited to the database gnomAD (Karczewski et al., 2020).
The PIR gene is very polymorphic and is involved in tumorigenesis; however, at this stage, we are uncertain about the effects of the reported nsSNPs on protein structure and biological activities. Therefore, in the present study, with the help of various computational approaches, highly deleterious nsSNPs in the PIR gene will be identified, which profoundly affect the structure and function of PIR protein. This study is the first extensive in silico analysis of the PIR gene that can narrow down the candidate mutations for further validation and targeting for therapeutic purposes.
The online public resources were used to retrieve all the available data about the human PIR gene. All the experimentally reported single-nucleotide polymorphisms (SNPs) in the PIR gene were collected from an online database gnomAD (https://gnomad.broadinstitute.org/) (Karczewski et al., 2020), and the UniProt database (http://www.uniprot.org/) (Magrane, 2011) was used to retrieve the amino acid sequence (UniProt ID: O00625) that encodes for PIR protein. The already reported crystal structure (PDB ID: 6N0J) of PIR protein was obtained from the Protein Data Bank (http://www.rcsb.org/) (Rose et al., 2010).
Various online servers such as PredictSNP (Bendl et al., 2014), MAPP (Multivariate Analysis of Protein Polymorphism) (Chao et al., 2008), PhD-SNP (Predictor of human Deleterious Single Nucleotide Polymorphisms) (Capriotti and Fariselli, 2017), PolyPhen-2 (Polymorphism Phenotyping version 2) (Adzhubei et al., 2013), SIFT (Sorting Intolerant from Tolerant), SNAP (screening for non-acceptable polymorphisms) (Bromberg et al., 2008), and PANTHER (Protein ANalysis THrough Evolutionary Relationships) (Mi et al., 2019) were used to predict the functional effect of nsSNPs. The deleterious nsSNPs, as suggested by all servers, were selected for further analysis. PredictSNP (https://loschmidt.chemi.muni.cz/predictsnp1/) executes prediction with diverse tools and provides a more authentic and accurate substitute for the predictions provided by the individual integrated tool. The predictions by tools in the PredictSNP server are enhanced by experimental annotations from two databases (24). MAPP (http://mendel.stanford.edu/SidowLab/downloads/MAPP/) predicts the effect of all possible SNPs on the function of the protein by considering the physiochemical deviation present in a column of aligned protein sequence (Stone and Sidow, 2005). PhD-SNP (http://snps.biofold.org/phd- snp/phd-snp.html) predicts and divides nsSNPs into disease-related and neutral polymorphisms according to the score ranging from 0 to 1. This server considers SNPs as a disease associated with a score more than 0.5 by using a related program algorithm. The outputs of PhD-SNP depend on frequencies of wild and mutant residues, the conservation index of SNP position, and a number of sequences aligned (Capriotti et al., 2006). PolyPhen-2 (http://genetics.bwh.harvard.edu/pp2) predicts the effect of amino acid variation on protein structure and function. The PolyPhen output is represented with a score that ranges from 0 to 1. This online tool considers non-synonymous SNPs as deleterious, having a higher mutation score, while zero scores indicate no effect of amino acid substitution on protein function (Adzhubei et al., 2010). SIFT (http://sift.bii.a-star.edu.sg) is a program that predicts the effect of amino acid substitution on protein functions. The principles of SIFT predictions depend on the physicochemical properties of protein sequence and its homologies. SIFT classifies its output as deleterious or neutral according to the score ranging from 0 to 1 (0–0.05 as deleterious and 0.05–1 as neutral). (Sim et al., 2012). SNAP (https://rostlab.org/services/snap) is a neural network–based prediction server that identifies the functional effects of amino acid sequence variants. The prediction score ranges from -100 (strongly neutral prediction) to 100 (strong effect prediction), which reflects the likelihood of the single amino acid mutation that may alter the native protein function (Hecht et al., 2015). PANTHER-PSEP (http://www.pantherdb.org/tools/csnpScoreForm.jsp) is an advanced online tool that predicts the non-synonymous mutations that have an important role in human diseases. PANTHER-PSEP uses a correlated but distinctive metric-based evolutionary conservancy. Homologous proteins are used to reconstruct the likely sequences of ancestral proteins at nodes in a phylogenetic tree, and the history of each amino acid can be traced back in time from its current state to estimate how long that state has been preserved in its ancestors.
To analyze the effect of a mutation on protein stability, we used DynaMut (Rodrigues et al., 2020), a protein stability consensus predictor based on ENCoM’s predicted vibrational entropy changes and the stabilization changes predicted by an mCSM’s graph-based signature method. The degree of the evolutionary conservancy of protein sequence location correlates with the evolutionary degree, which is not the same for all amino acids in the corresponding protein. Positions of amino acids that change slowly are usually known to be conserved sites that are important for the structure and function of a protein.
The crystal structure of the PIR protein was extracted from the PDB (Entry ID: 6N0J). The protein structure was minimized using Chimera software [(Webb and Sali, 2016),33]. Moreover, the wild type (WT) structure was mutated by each one of the three most deleterious mutants predicted in the previous sections. The three structures of mutant (MT) proteins, such as I28T, V257A, and I264S, were modeled by making a point mutation in the wild-type (WT) protein structure using Chimera software. The WT and three MT structures are shown in Figure 1.
FIGURE 1. Modeled 3D structures of the WT and I28T, V257A, and I264S mutants. The modeled mutations are encircled to show their position. The Fe ion binding is also shown.
The AMBER18 (Mermelstein et al., 2018) package was used for molecular dynamics simulation to investigate the stability of WT and mutants of pirin (PIR) using the ff14SB force field (Maier et al., 2015). Molecular dynamics (MD) simulation was performed for a total of four systems, including one wild type (WT) and three mutants I28T, V257A, and I264S. For the solvation of each system in a rectangular box water model, TIP3P was used. With the help of counterions, addition neutralization was achieved. A two-step energy minimization procedure: the steepest decent minimizations of 6,000 cycles and conjugate gradient minimization of 3,000 cycles, was applied for minimization of each neutralized system. After minimization, these complexes were heated up to 300 K for 0.2 ns, and then we equilibrated the system with weak restraint and without restraint for 2 ns at 300 K, respectively. The temperature was controlled with a Langevin thermostat (Adzhubei et al., 2010) (26), and the procedure was run for 100 ns. Each simulation was repeated three times. Long-range electrostatic interactions (Bromberg et al., 2008; Sim et al., 2012; Adzhubei et al., 2013; Hecht et al., 2015) were detected with the particle mesh Ewald method (Petersen, 1995) using a cut-off distance of 10.0 Å. The SHAKE method was applied for covalent bond treatment (Mi et al., 2019). The MD simulation production step was performed on the GPU-supported PMEMD code for each system (Glaser et al., 2003; Stone and Sidow, 2005), and the trajectories were analyzed on the CPPTRAJ package in Amber18.
Principal component analysis (PCA) was utilized for the calculation of high-amplitude fluctuations within the protein (Berezin et al., 2004; Capriotti et al., 2006). The CPPTRAJ package calculated the covariance matrix based on Cα coordinates. Eigenvectors and eigenvalues were calculated by diagonalizing the covariance matrix. 5,000 snapshots from the trajectory of each system were used to get PCA calculations. Eigenvectors and eigenvalues indicate the direction of motion and mean square fluctuation, respectively. PC1 and PC2 were used for plotting to monitor the motion. The lowest energy stable state was determined by the free energy landscape (FEL) and is indicated by deep valleys on plot, whereas the intermediate state is shown by boundaries between deep valleys (Xu et al., 2017; Adzhubei et al., 2010). In this study, FEL calculations based on PC1 and PC2 were obtained by
where the reaction coordinates are taken by PC1 and PC2, KB denotes the Boltzmann constant, and P (PC1, PC2) shows the probability distribution of the system along with the first two principal components.
The online public resources were used to retrieve all the available data of the human PIR gene. According to the information obtained from the online database gnomAD, there were a total of 173 SNPs in the PIR protein. Of those, 119 SNPs were identified as non-synonymous. These 119 SNPs were submitted to different online servers for identification of the deleterious mutations. First, the SNPs were submitted to PredictSNP and MAPP servers, and only 51 and 41 SNPs were found as deleterious, respectively. The nsSNPs were then submitted to PhD-SNP and SNAP online tools, and 63 and 55 SNPs were found as deleterious, respectively. The other online servers such as PolyPhen-1, PolyPhen-2, SIFT, and PANTHER analyzed the nsSNPs and predicted that, out of 119 SNPs, only 51, 46, 68, and 80 were deleterious, respectively. All the nsSNPs were selected for further analysis that were predicted as deleterious consistently by all the above online servers as shown in Table 1. The total number of predicted deleterious SNPs by each server is given in Figure 2.
TABLE 1. Processing of 119 missense variants by different servers predicted 24 mutations to be deleterious collectively. The predicted score by each server is also shown.
FIGURE 2. The total number of predicted deleterious SNPs by each server is shown as bars. Each bar represents a specific server, and its predicted deleterious mutations are given on the top.
To calculate the stability changes upon mutations, an online server mCSM was used, which reported the average changes ranging from 0.715 to −2.856 kcal/mol. Mutations, such as V257A with a stability fold change of -2.157 kcal/mol, I28T with a stability fold change of -2.374 kcal/mol, and I264S with a stability fold changes of -2.856 kcal/mol, were found to be highly destabilizing for the PIR protein structure. However, the mutation H81P with a stability fold change of 0.715 kcal/mol has the opposite effect (i.e., stability) and does not induce major changes in the protein structure (Table 2). The RMSDs between the WT and the three mutants are shown as a superimposed structure in Figure 3. The highly destabilizing mutations identified by mCSM were analyzed by DynaMut to check the effect of these mutations on the structure flexibility. As shown in Figure 4, the mutations I28T, V257A, and I264S produced higher flexibility in the protein structure. These results are clearly pointing out the importance of these three mutations. These changes in flexibility (red) and rigidity (blue) are mapped onto the corresponding protein structure and presented in Figure 4.
TABLE 2. A list of 24 highly deleterious mutations was processed to identify the highly destabilizing mutations.
FIGURE 3. Superimposed structure of WT PIR protein (cyan) with mutants I28T (green), I264S (orange), and V257A (yellow). The RMSD of each superimposition was reported to be 0.333 Å (I28T) and 0.879 Å (I264S, V257A).
FIGURE 4. Effect of mutations on structural dynamics flexibility. Changes in flexibility (red) and rigidity (blue) mapped onto the corresponding protein structure are shown.
To estimate the impact of the specific mutant (I28T, V257A, and I264S) and WT, we calculated the RMSD (root mean square deviation) from the MD trajectory. We used 5,000 structures from the MD trajectory as a function of time. In the case of the WT, the RMSD remained stable during the 100 ns simulation time. No significant convergence was observed. The system reached the stability at 1.3 Å. The average RMSD was reported to be 1.25 Å. Overall, the system seems to be stable with no significant convergence during the 100 ns simulation. On the contrary, the I28T mutation showed significant convergence at different intervals. Initially, the structure continued to proceed stably until 20 ns, but after the system faced convergence, the RMSD increased from 1.5 to 2.0 Å.
Afterward, the RMSD decreased and remained uniform until 70 ns, but the structure faced significant perturbation and the RMSD increased again until 100 ns. The major convergence was observed specifically between 75 and 90 ns. The average RMSD (1.8 Å) also remained higher than that in the wild type. This shows that the I28T mutation has caused a significant structural stability shift and needs longer simulation to gain the equilibrium. Furthermore, the V257A mutation also induced significant structural stability changes. The RMSD remained higher during the 100 ns simulation. Initially, the RMSD increased until 1.25 Å and then continued to increase until 20 ns. Afterward, an abrupt decrease was observed at 22 ns, and then again, the RMSD increased. The RMSD between 60 and 80 ns significantly converged, and the average RMSD between 60 and 80 ns was observed to be 2.0 Å. The RMSD then decreased and remained uniform until 95 ns, but then again, the structure converged and the RMSD increased. Hence, the V257A mutation has caused significant structural perturbation, and the stability significantly shifted as compared to that of the wild type. I264S was reported to be the most destabilizing mutation among the list of 24 non-synonymous mutations reported to be deleterious. The results here are uniform with the mCSM server. The mutation has induced significant stability transition and perturbation. Initially until 20 ns, the RMSD remained uniform, but a sudden convergence increased the RMSD up to 2.5 Å. Later on, the RMSD decreased for a short period of time, and then significant convergence was observed between 35 and 40 ns. The RMSD then remained lower and uniform until 85 ns. The structure then faced significant perturbation, and the RMSD increased again up to 2.0 Å. The average RMSD for I264S was reported to be 2.2 Å. Thus, these results signify that the mutations have caused significant structural destability and internal dynamics of the protein. The RMSD graph of the WT and mutants (I28T, V257A, and I264S) is shown in Figure 5. The x-axis shows the time in nanoseconds, while the y-axis shows the RMSD in angstrom.
FIGURE 5. RMSD graph of the WT and mutants (I28T, V257A, and I264S). The x-axis shows the time in nanoseconds, while the y-axis shows the RMSD in angstrom. (A) Wild type; (B) I28T; (C) V257A; (D) I264S.
Furthermore, to estimate the impact of the mutation on the residual flexibility, we calculated the RMSF (root mean square fluctuation) as a function of residues. Overall, the residual flexibility showed more similar fluctuation except in few regions. In the case of V257A, the region between 15 and 25 showed higher fluctuation than the others. In addition, the region between 72 and 85 in the WT possesses higher fluctuation than the mutants. Thus, this shows that this region is significantly affected by the mutation induction. In the case of I264S, specifically the region between 140 and 150 showed higher fluctuation. Furthermore, this mutation also increased the fluctuation of the region between 250 and 280, thus causing significant internal dynamics fluctuation. These results show that the mutation has affected different regions of the protein to increase or decrease the flexibility. The RMSF graph of the WT and mutants (I28T, V257A, and I264S) is shown in Figure 6. The x-axis shows the number of residues, while the y-axis shows the RMSF in angstrom.
FIGURE 6. RMSF graph of the WT and mutants (I28T, V257A, and I264S). The x-axis shows the number of residues, while the y-axis shows the RMSF in angstrom.
In order to calculate the compactness of all the WT and mutant (I28T, V257A, and I264S) systems, Rg (radius of gyration) was calculated. The stability of the complexes formed also depended on the compactness of the structure. From Figure 7, it can be easily observed that the average Rg value for all the systems is between 19.0 and 19.4 Å. In the case of wild type, the Rg value remained uniform until 100 ns. The average value for the WT was observed to be 19.0 Å. In the case of I28T, the system remained relatively less compact than the wild type. The average Rg value was reported to be 19.0 Å for the first 52 ns, and then the Rg value continued to increase and reached 19.2 Å during the simulation time. In the case of V257A, the Rg value remained lower until 5 ns. The Rg then continued to increase until 100 ns. The Rg value for the rest of 95 ns remained 19.3 Å. The Rg for I264S started from 19.2 Å and continued to increase. After reaching 30 ns, the Rg value increased to 19.3 Å and increased further. After 70 ns, the Rg value further increased to 19.5 Å and continued until 100 ns. These results significantly justify that the mutation has different compactness than the WT during the simulation. The Rg graph of the WT and mutants (I28T, V257A, and I264S) is shown Figure 7. The x-axis shows the time in nanoseconds, while the y-axis shows the Rg in angstrom.
FIGURE 7. Rg graph of the WT and mutants (I28T, V257A, and I264S). The x-axis shows the time in nanoseconds, while the y-axis shows the RMSD in angstrom.
To describe the protein motion and clustering of the related structural frames, principal component analysis (PCA) was performed. PCA is a statistical approach that incorporates a smaller number of uncorrelated variables called principal components into several correlated variables. The eigenvectors were measured and are provided in Figure 8 to comprehensively explain the effect of the substitution on the protein motion. From the PCs, we can understand the overall and internal motions. In the wild type, the total contributed variance by the first three eigenvectors to the total motions was reported to be 47%, while in the case of I28T, the variance by the three eigenvectors was observed to be 38%, and for V257A, it was observed to be 39%. In the other mutation such as I264S, the variance by the first three eigenvectors was reported to be 35%.
FIGURE 8. Fractions of the first ten eigenvectors. Using the MD trajectory, the fraction of motions is calculated and given in percentage against the eigenvector numbers.
The other eigenvectors have shown localized or overall motions. Hence, it is confirmed that the mutations have impacted the total trajectory motion and, thus, internal dynamics behavior. To further gain convincible attributes, the first two PCs, i.e., PC1 and PC2, were drawn against each other. Different colors (red to blue) reflect the conformational transition from one to another. Each dot in Figure 9 depicts a single frame of the trajectory. As compared to the WT, the mutant complexes covered a lower region of the phase space except in V257A and I264S. Together, these observations suggest that mutations had a substantial influence on the structure that has contributed to pirin (PIR) destabilization.
FIGURE 9. Principal component analysis of all the systems, including the WT and the three mutants. The first two principal components (PC1 and PC2) are used for the projection of motion in the space phase at 300 K.
Conformational changes induced by a particular mutation during the MD simulation were explored through the FEL. PC1 and PC2 were used to map the energy minima and extract the variations due to a specific mutation. In the case of the wild type, the lowest energy minima were reached at 23 ns. Figure 10 shows that, during the simulation, no structural perturbation was experienced in the wild type. On the contrary, in the three mutations, destabilization of the Fe ion was observed. The energy minima separated by subspace in each mutant complex were reached at 49 ns (I28T), 67 ns (V257A), and 79 ns (I264S). In addition, the cavity surrounding the Fe ion also exhibits a dynamic structure in all the mutants. The beta sheet covering the Fe ion from the top and the loop on the alternate side changed their orientations, and an opening–closing switch-like pattern was observed. In addition, the flipping of beta sheets in the mutant complexes was most frequently observed in the mutant complexes. All the FEL graphs of the wild type, I28T, V257A, and I264S are given in Figure 10.
FIGURE 10. Conformational changes during the molecular dynamics simulation are represented through the FEL. PC1 and PC2 are used to map the energy minima and extract the variations due to a specific mutation.
PIR is an oxidative stress sensor from the functionally diverse superfamily of cupin. This protein is suggested to have biological relevance in cancer development and thus remains a novel research area. Being polymorphic, its oncogenic activity is a hot topic of discussion in the recent past. The work reported herein attempted to use an extensive computational framework to screen all potential mutations of the protein and identify deleterious mutants that could affect protein structure stability and ultimately functionality. The work predicted 119 missense variants by different servers and reported 24 deleterious mutations consistently reported by all available mutation predictor servers. Furthermore, it was highlighted that the three mutations I28T, V257A, and I264S are most destabilizing and confer structure flexibility to the PIR protein. To sum up, the study provides structural basis for each mutation-induced conformational change and disclosed a possible way for the mutations’ role in the progression of Breast Cancer (BC); thus, PIR acts a potential therapeutic target or a biomarker in the future ahead.
The datasets presented in this study can be found in online repositories. The names of the repository/repositories and accession number(s) can be found in the article/Supplementary Material.
MS, MT, and SS performed data retrieval. MS, MT, SS, SA, SSA, AA, FA, MW, and KA were responsible for data analysis. HK, FA, and WK were responsible for graphics and writing. AA, FA, MW, and KA have contributed to drafting and finalizing the manuscript.
The article-processing charges were funded by Qassim University, Saudi Arabia. We acknowledge their help.
The authors declare that the research was conducted in the absence of any commercial or financial relationships that could be construed as a potential conflict of interest.
The handling editor declared a past co-authorship with several of the authors (SS, SSA, and MS).
Adzhubei, I. A., Schmidt, S., Peshkin, L., Ramensky, V. E., Gerasimova, A., Bork, P., et al. (2010). A Method and Server for Predicting Damaging Missense Mutations. Nat. Methods 7 (4), 248–249. doi:10.1038/nmeth0410-248
Adzhubei, I., Jordan, D. M., and Sunyaev, S. R. (2013). Predicting Functional Effect of Human Missense Mutations Using PolyPhen‐2. Curr. Protoc. Hum. Genet. 76 (1), 7–20. doi:10.1002/0471142905.hg0720s76
Bendl, J., Stourac, J., Salanda, O., Pavelka, A., Wieben, E. D., Zendulka, J., et al. (2014). PredictSNP: Robust and Accurate Consensus Classifier for Prediction of Disease-Related Mutations. Plos Comput. Biol. 10 (1), e1003440. doi:10.1371/journal.pcbi.1003440
Berezin, C., Glaser, F., Rosenberg, J., Paz, I., Pupko, T., Fariselli, P., et al. (2004). ConSeq: the Identification of Functionally and Structurally Important Residues in Protein Sequences. Bioinformatics 20 (8), 1322–1324. doi:10.1093/bioinformatics/bth070
Bromberg, Y., Yachdav, G., and Rost, B. (2008). SNAP Predicts Effect of Mutations on Protein Function. Bioinformatics 24 (20), 2397–2398. doi:10.1093/bioinformatics/btn435
Capriotti, E., Calabrese, R., and Casadio, R. (2006). Predicting the Insurgence of Human Genetic Diseases Associated to Single point Protein Mutations with Support Vector Machines and Evolutionary Information. Bioinformatics 22 (22), 2729–2734. doi:10.1093/bioinformatics/btl423
Capriotti, E., and Fariselli, P. (2017). PhD-SNPg: a Webserver and Lightweight Tool for Scoring Single Nucleotide Variants. Nucleic Acids Res. 45 (W1), W247–W252. doi:10.1093/nar/gkx369
Chang, J.-W., Ding, Y., Tahir ul Qamar, M., Shen, Y., Gao, J., and Chen, L.-L. (2019). A Deep Learning Model Based on Sparse Auto-Encoder for Prioritizing Cancer-Related Genes and Drug Target Combinations. Carcinogenesis 40 (5), 624–632. doi:10.1093/carcin/bgz044
Chao, E. C., Velasquez, J. L., Witherspoon, M. S. L., Rozek, L. S., Peel, D., Ng, P., et al. (2008). Accurate Classification ofMLH1/MSH2missense Variants with Multivariate Analysis of Protein Polymorphisms-Mismatch Repair (MAPP-MMR). Hum. Mutat. 29 (6), 852–860. doi:10.1002/humu.20735
Chen, C. C., Chow, M. P., Huang, W. C., Lin, Y. C., and Chang, Y. J. (2004). Flavonoids Inhibit Tumor Necrosis Factor-Alpha-Induced Up-Regulation of Intercellular Adhesion Molecule-1 (ICAM-1) in Respiratory Epithelial Cells through Activator Protein-1 and Nuclear Factor-kappaB: Structure-Activity Relationships. Mol. Pharmacol. 66 (3), 683–693. doi:10.1124/mol.66.3
Dunwell, J. M., Culham, A., Carter, C. E., Sosa-Aguirre, C. R., and Goodenough, P. W. (2001). Evolution of Functional Diversity in the Cupin Superfamily. Trends Biochemical Sciences 26 (12), 740–746. doi:10.1016/s0968-0004(01)01981-8
Feitelson, M. A., Arzumanyan, A., Kulathinal, R. J., Blain, S. W., Holcombe, R. F., Mahajna, J., et al. (2015). Sustained Proliferation in Cancer: Mechanisms and Novel Therapeutic Targets. Semin Cancer Biol. 35, S25. doi:10.1016/j.semcancer.2015.02.006
Glaser, F., Pupko, T., Paz, I., Bell, R. E., Bechor-Shental, D., Martz, E., et al. (2003). ConSurf: Identification of Functional Regions in Proteins by Surface-Mapping of Phylogenetic Information. Bioinformatics 19 (1), 163–164. doi:10.1093/bioinformatics/19.1.163
Hecht, M., Bromberg, Y., and Rost, B. (2015). Better Prediction of Functional Effects for Sequence Variants. BMC genomics 16 (8), 1–12. doi:10.1186/1471-2164-16-s8-s1
Karczewski, K. J., Francioli, L. C., Francioli, L. C., Tiao, G., Cummings, B. B., Alföldi, J., et al. (2020). The Mutational Constraint Spectrum Quantified from Variation in 141,456 Humans. Nature 581 (7809), 434–443. doi:10.1038/s41586-020-2308-7
Khan, A., Ali, S. S., Khan, M. T., Saleem, S., Ali, A., Suleman, M., et al. (2020a). Combined Drug Repurposing and Virtual Screening Strategies with Molecular Dynamics Simulation Identified Potent Inhibitors for SARS-CoV-2 Main Protease (3CLpro). J. Biomol. Struct. Dyn. 38, 1–12. doi:10.1080/07391102.2020.1779128
Khan, A., Khan, M., Saleem, S., Babar, Z., Ali, A., Khan, A. A., et al. (2020b). Phylogenetic Analysis and Structural Perspectives of RNA-dependent RNA-Polymerase Inhibition from SARs-CoV-2 with Natural Products. Interdiscip. Sci. Comput. Life Sci. 12 (3), 335–348. doi:10.1007/s12539-020-00381-9
Khan, A., Khan, M. T., Saleem, S., Junaid, M., Ali, A., Ali, S. S., et al. (2020c). Structural Insights into the Mechanism of RNA Recognition by the N-Terminal RNA-Binding Domain of the SARS-CoV-2 Nucleocapsid Phosphoprotein. Comput. Struct. Biotechnol. J. 18, 2174. doi:10.1016/j.csbj.2020.08.006
Khan, A., Rehman, Z., Hashmi, H. F., Khan, A. A., Junaid, M., Sayaf, A. M., et al. (2020d). An Integrated Systems Biology and Network-Based Approaches to Identify Novel Biomarkers in Breast Cancer Cell Lines Using Gene Expression Data. Interdiscip. Sci. 12, 155–168. doi:10.1007/s12539-020-00360-0
Khan, A., Heng, W., Wang, Y., Qiu, J., Wei, X., Peng, S., et al. (2021a). In Silico and In Vitro Evaluation of Kaempferol as a Potential Inhibitor of the SARS-CoV-2 Main Protease (3CLpro). Phytotherapy Res. PTR doi:10.1002/ptr.6998
Khan, A., Khan, M., Ullah, S., and Wei, D.-Q. (2021b). Hantavirus: The Next Pandemic We Are Waiting for?. Interdiscip. Sci. Comput. Life Sci. 1-6, 335–348. doi:10.1007/s12539-020-00381-9
Khan, A., Zia, T., Suleman, M., Khan, T., Ali, S. S., Abbasi, A. A., et al. (2021c). Higher Infectivity of the SARS‐CoV‐2 New Variants Is Associated with K417N/T, E484K, and N501Y Mutants: An Insight from Structural Data. J. Cel Physiol. 236, 1–13. doi:10.1002/jcp.30367
Kumar, P., Henikoff, S., and Ng, P. C. (2009). Predicting the Effects of Coding Non-synonymous Variants on Protein Function Using the SIFT Algorithm. Nat. Protoc. 4 (7), 1073–1081. doi:10.1038/nprot.2009.86
Maier, J. A., Martinez, C., Kasavajhala, K., Wickstrom, L., Hauser, K. E., and Simmerling, C. (2015). ff14SB: Improving the Accuracy of Protein Side Chain and Backbone Parameters from ff99SB. J. Chem. Theor. Comput. 11 (8), 3696–3713. doi:10.1021/acs.jctc.5b00255
Massoumi, R., Kuphal, S., Hellerbrand, C., Haas, B., Wild, P., Spruss, T., et al. (2009). Down-regulation of CYLD Expression by Snail Promotes Tumor Progression in Malignant Melanoma. J. Exp. Med. 206 (1), 221–232. doi:10.1084/jem.20082044
Mermelstein, D. J., Lin, C., Nelson, G., Kretsch, R., McCammon, J. A., and Walker, R. C. (2018). Fast and Flexible Gpu Accelerated Binding Free Energy Calculations within the Amber Molecular Dynamics Package. J Comput Chem 39, 1354. doi:10.1002/jcc.25187
Mi, H., Muruganujan, A., Ebert, D., Huang, X., and Thomas, P. D. (2019). PANTHER Version 14: More Genomes, a New PANTHER GO-Slim and Improvements in Enrichment Analysis Tools. Nucleic Acids Res. 47 (D1), D419–D426. doi:10.1093/nar/gky1038
Muneer, I., ul Qamar, M. T., Tusleem, K., Hussain, S. H. M. J., and Siddiqi, A. R. (2019). Discovery of Selective Inhibitors for Cyclic AMP Response Element-Binding Protein. Anti-cancer drugs 30 (4), 363–373. doi:10.1097/cad.0000000000000727
Pang, H., Bartlam, M., Zeng, Q., Miyatake, H., Hisano, T., Miki, K., et al. (2004). Crystal Structure of Human Pirin. J. Biol. Chem. 279 (2), 1491–1498. doi:10.1074/jbc.m310022200
Petersen, H. G. (1995). Accuracy and Efficiency of the Particle Mesh Ewald Method. J. Chem. Phys. 103 (9), 3668–3679. doi:10.1063/1.470043
Riaz, M., Ashfaq, U. A., Qasim, M., Yasmeen, E., Ul Qamar, M. T., and Anwar, F. (2017). Screening of Medicinal Plant Phytochemicals as Natural Antagonists of P53-MDM2 Interaction to Reactivate P53 Functioning. Anti-cancer drugs 28 (9), 1032–1038. doi:10.1097/cad.0000000000000548
Rodrigues, C. H. M., Pires, D. E. V., and Ascher, D. B. (2020). DynaMut2 : Assessing Changes in Stability and Flexibility upon Single and Multiple point Missense Mutations. Protein Sci. 30 (1), 60–69. doi:10.1002/pro.3942
Rose, P. W., Beran, B., Bi, C., Bluhm, W. F., Dimitropoulos, D., Goodsell, D. S., et al. (2010). The RCSB Protein Data Bank: Redesigned Web Site and Web Services. Nucleic Acids Res. 39 (Suppl. l_1), D392–D401. doi:10.1093/nar/gkq1021
Sim, N.-L., Kumar, P., Hu, J., Henikoff, S., Schneider, G., and Ng, P. C. (2012). SIFT Web Server: Predicting Effects of Amino Acid Substitutions on Proteins. Nucleic Acids Res. 40 (W1), W452–W457. doi:10.1093/nar/gks539
Stone, E. A., and Sidow, A. (2005). Physicochemical Constraint Violation by Missense Substitutions Mediates Impairment of Protein Function and Disease Severity. Genome Res. 15 (7), 978–986. doi:10.1101/gr.3804205
Suleman, M., Chen, A., Ma, H., Wen, S., Zhao, W., Lin, D., et al. (2019). PIR Promotes Tumorigenesis of Breast Cancer by Upregulating Cell Cycle Activator E2F1. Cell Cycle 18 (21), 2914–2927. doi:10.1080/15384101.2019.1662259
Wadood, A., Mehmood, A., Khan, H., Ilyas, M., Ahmad, A., Alarjah, M., et al. (2017). Epitopes Based Drug Design for Dengue Virus Envelope Protein: a Computational Approach. Comput. Biol. Chem. 71, 152–160. doi:10.1016/j.compbiolchem.2017.10.008
Webb, B., and Sali, A. (2016). Comparative Protein Structure Modeling Using MODELLER. Curr. Protoc. Bioinformatics 54 (1), 5–6. doi:10.1002/cpbi.3
Wendler, W. M. F., Kremmer, E., Förster, R., and Winnacker, E.-L. (1997). Identification of Pirin, a Novel Highly Conserved Nuclear Protein. J. Biol. Chem. 272 (13), 8482–8489. doi:10.1074/jbc.272.13.8482
Xu, S., Long, B. N., Boris, G. H., Chen, A., Ni, S., and Kennedy, M. A. (2017). Structural Insight into the Rearrangement of the Switch I Region in GTP-Bound G12A K-Ras. Acta Cryst. Sect D Struct. Biol. 73 (12), 970–984. doi:10.1107/s2059798317015418
Zhu, G., Reynolds, L., Crnogorac-Jurcevic, T., Gillett, C. E., Dublin, E. A., Marshall, J. F., et al. (2003). Combination of Microdissection and Microarray Analysis to Identify Gene Expression Changes between Differentially Located Tumour Cells in Breast Cancer. Oncogene 22 (24), 3742–3748. doi:10.1038/sj.onc.1206428
Keywords: nsSNPs, PIR protein, breast cancer, MD simulations, PIR
Citation: Suleman M, Tahir ul Qamar M, Saleem S, Ahmad S, Ali SS, Khan H, Akbar F, Khan W, Alblihy A, Alrumaihi F, Waseem M and Allemailem KS (2021) Mutational Landscape of Pirin and Elucidation of the Impact of Most Detrimental Missense Variants That Accelerate the Breast Cancer Pathways: A Computational Modelling Study. Front. Mol. Biosci. 8:692835. doi: 10.3389/fmolb.2021.692835
Received: 09 April 2021; Accepted: 07 June 2021;
Published: 28 June 2021.
Edited by:
Abbas Khan, Shanghai Jiao Tong University, ChinaReviewed by:
Mohammad Tariq, Catholic University of Louvain, BelgiumCopyright © 2021 Suleman, Tahir ul Qamar, Saleem, Ahmad, Ali, Khan, Akbar, Khan, Alblihy, Alrumaihi, Waseem and Allemailem. This is an open-access article distributed under the terms of the Creative Commons Attribution License (CC BY). The use, distribution or reproduction in other forums is permitted, provided the original author(s) and the copyright owner(s) are credited and that the original publication in this journal is cited, in accordance with accepted academic practice. No use, distribution or reproduction is permitted which does not comply with these terms.
*Correspondence: Khaled S. Allemailem, ay5hbGxlbWFpbGVtQHF1LmVkdS5zYQ==
Disclaimer: All claims expressed in this article are solely those of the authors and do not necessarily represent those of their affiliated organizations, or those of the publisher, the editors and the reviewers. Any product that may be evaluated in this article or claim that may be made by its manufacturer is not guaranteed or endorsed by the publisher.
Research integrity at Frontiers
Learn more about the work of our research integrity team to safeguard the quality of each article we publish.