- 1Department of Medical Oncology, Guangxi Medical University First Affiliated Hospital, Nanning, China
- 2Emergency Department, Guangxi Medical University First Affiliated Hospital, Nanning, China
- 3College of Oncology, Guangxi Medical University, Nanning, China
Immune-related genes (IRGs) are closely related to tumor progression and the immune microenvironment. Few studies have investigated the effect of tumor immune microenvironment on the survival and response to immune checkpoint inhibitors of patients with bladder urothelial carcinoma (BLCA). We constructed two IRG-related prognostic signatures based on gene–immune interaction for predicting risk stratification and immunotherapeutic responses. We also verified their predictive ability on internal and overall data sets. Patients with BLCA were divided into high- and low-risk groups. The high-risk group had poor survival, enriched innate immune-related cell subtypes, low tumor mutation burden, and poor response to anti-PD-L1 therapy. Our prognostic signatures can be used as reliable prognostic biomarkers, which may be helpful to screen the people who will benefit from immunotherapy and guide the clinical decision-making of patients with BLCA.
Introduction
Bladder cancer is the 10th most common cancer worldwide. In 2017, 474,000 new cases of bladder cancer were diagnosed and 197,000 deaths from the disease were reported worldwide (Fitzmaurice et al., 2019). Bladder urothelial carcinoma (BLCA) accounts for about 80–90% of all pathological types (Felsenstein and Theodorescu, 2018). About 25% of patients have muscle-invasive or metastatic lesions at the time of onset (Kamat et al., 2016). Patients have only 12–15 months of overall survival (OS) after being diagnosed before 2016 because of the lack of substantial progress in BLCA treatment; this finding has been maintained for the past 30 years (Bellmunt et al., 2017; Vlachostergios and Faltas, 2018). However, since 2016, several studies on immune checkpoint inhibitors (ICIs) have changed the treatment paradigm for metastatic urothelial cancer (mUC) and outlined a future therapeutic landscape (Rosenberg et al., 2016; Sharma et al., 2016; Apolo et al., 2017; Powles et al., 2017).
Considering that bladder cancer has high tumor mutation burden (TMB) and many mutations may be antigenic (Felsenstein and Theodorescu, 2018), traditional immunotherapy using Bacillus Calmette-Guérin has been successful for the treatment of early diagnosed bladder cancer (Kamat et al., 2015). Compared with previous first-line or second-line chemotherapy for mUC, new immunotherapy is undoubtedly an exciting breakthrough. However, the response rate of PD-1/PD-L1 inhibitors is only 20–24% (Kamat et al., 2015). PD-L1 status cannot highly predict treatment response; in this regard, more accurate predictive biomarkers should be identified to prescreen appropriate patients for immunotherapy and design personalized treatment. Existing immune and inflammatory markers in tumor may be the best biomarkers for evaluating the potential response of ICIs (Felsenstein and Theodorescu, 2018).
Studies have found that bladder cancer, similar to breast cancer, can be divided into different subtypes based on gene expression diversity and histological characteristics; this classification may be conducive to hierarchical management and precise treatment (Groenendijk et al., 2016; The Cancer Genome Atlas Research Network, 2014). Epigenetic regulatory mechanisms, such as DNA methylation, histone modification, and ncRNA expression, change with the development of bladder cancer and therefore may be used as potential biomarkers and therapeutic targets (Dudziec et al., 2011; Schulz and Goering, 2016; Peng et al., 2018). Additionally, differences in the molecular and genetic characteristics of tumor cells (such as gene mutation and copy number alteration) and the tumor microenvironment have a high impact on tumor invasiveness and sensitivity to treatment. The molecular profiles of immune components in the tumor microenvironment have great value as prognostic biomarkers. Immune-related genes (IRGs) can be quantified from a variety of cell types in a sample and have a predictive value for BLCA prognosis; these genes may be suitable biomarkers (Sweis et al., 2016; Li et al., 2020; Xu et al., 2020).
In this study, we downloaded the expression profiles and clinical information of patients from The Cancer Genome Atlas (TCGA)-BLCA cohort, analyzed the resulting differentially expressed genes (DEGs), and intersected the DEGs with the IRG sets downloaded from the ImmPort database (https://www.immport.org/home) to obtain differentially expressed immune-related genes (DE-IRGs). The DE-IRGs associated with prognosis were further analyzed to construct prognostic models. Furthermore, we assessed the differences in the immune cell infiltration, TMB, and response to ICI treatment between the high- and low-risk groups. The robust prognostic model may improve risk stratification and provide a more accurate assessment for the clinical management of BLCA.
Materials and Methods
Data Acquisition
The latest RNA-Seq expression profile data and clinical information of patients with BLCA were downloaded from TCGA (https://www.nature.com/articles/ng.2764, 2013), and data were also obtained from the Genotype-Tissue Expression (GTEx, https://www.gtexportal.org/home/) project. The lists of 456 IRGs and 318 transcription factors were derived from the ImmPort database (Sweis et al., 2016; Li et al., 2020; Xu et al., 2020) and Cistrome Cancer database (Mei et al., 2017), respectively, for subsequent analysis. The detailed clinicopathological and sequencing information of patients with advanced urothelial cancer treated with anti-PD-L1 agents (IMvigor210 cohort) were downloaded from the “IMvigor210CoreBiologies” R package (Mariathasan et al., 2018).
DE-IRG Identification
The expression profiles from TCGA and GTEx portal were integrated using the “limma” R package (Ritchie et al., 2015) to calculate the DEGs between BLCA tumor tissues and normal tissues (FDR <0.05 and |log2FC| > 1). All DEGs and IRGS were crossed to obtain 172 DE-IRGs. GO and KEGG pathway enrichment analyses of the DE-IRGs were conducted using the “clusterProfiler” R package (v3.16.1) (Yu et al., 2012).
Construction and Validation of OS and Disease-Free Survival Prognostic Signatures for Bladder Urothelial Carcinoma
Univariate Cox regression analysis was conducted to screen for DE-IRGs that are significantly associated with prognosis (p < 0.05). Least absolute shrinkage and selection operator (LASSO) Cox regression model (Tibshirani, 1996; Goeman, 2010) was used to construct an immune-related risk model using the “glmnet” R package as follows (Simon et al., 2011): Risk score = (level of gene a × coefficient a) + (level of gene b × coefficient b) + (level of gene c × coefficient c) + … + (level of gene n × coefficient n) (Yang et al., 2019). LASSO regression model is a compression estimation method, which constructs a penalty function to obtain a more refined model. This approach is a common method for biased data estimation with multicollinearity and has the advantage of preserving subset contraction. All samples were substituted into the formula to calculate the risk score value, which was then converted into Z-score using Z-score standardization. The samples with Z > 0 were included the high-risk group, and those with Z < 0 were included into the low-risk group. Kaplan–Meier survival curve analysis, log-rank test, and time-dependent Receiver operating characteristic (ROC) curve analysis were used to evaluate the predictive capability of the immune signatures.
Functional Enrichment Analysis of Gene Set
The “limma” R package (v3.36.5) (Yang et al., 2019) was used to calculate the differences in gene expression between the high- and low-risk groups. Gene set enrichment analysis (GSEA) (Hänzelmann et al., 2013) was used to analyze the KEGG pathway enrichment for the gene set sorted by log2 fold change value.
Estimation of the Tumor Microenvironment
CIBERSORT is a deconvolution analysis tool based on the principle of linear support vector regression for the expression matrix of human immune cell subtypes, which can infer the constituent ratio of 22 immune cell subtypes in complex tissues (Hänzelmann et al., 2013). The CIBERSORT computational tool (Chen et al., 2018) was used to determine the relative abundance of tumor-infiltrating immune cells.
Evaluation of Tumor Mutation Load
The TCGA database was mined for TMB to analyze and visualize the mutation spectrum by “maftools” in R (Mayakonda et al., 2018). The mutation load score of each sample was calculated to compare the TMB difference between the two groups (calculation formula:
Statistical Analysis
R software (v3.5.2) was used for statistical analysis. Differences between variables were analyzed by Chi-square test for categorical and continuous variables. Kaplan–Meier survival curve was constructed to compare survival across groups. ROC curve was used to determine the accuracy of the model. Statistical significance was set as p < 0.05.
Results
Clinical Information and Identification of Differentially Expressed Immune-Related Genes
After the RNA-seq data from TCGA-BLCA and GTEx was preprocessed, 407 tumor samples with OS information and 394 tumor samples with DFS information were obtained. The statistical results are shown in Supplementary Table S1. The patients with complete prognosis information were divided into training group (OS: n = 305, DFS: n = 295), testing group (OS: n = 102, DFS: n = 99), and entire group (OS: n = 407, DFS: n = 394) according to the ratio, 2:1:3. Chi-square test (p > 0.1) showed no difference in the distribution of clinical information between the testing and training groups (Table 1).
A total of 1,854 upregulated genes and 3,145 downregulated genes were identified between tumor and normal tissues (Supplementary Figure S1). The intersection of the predicted DEGs with the IRGs in BLCA yielded 62 upregulated and 109 downregulated DE-IRGs (Figure 1).
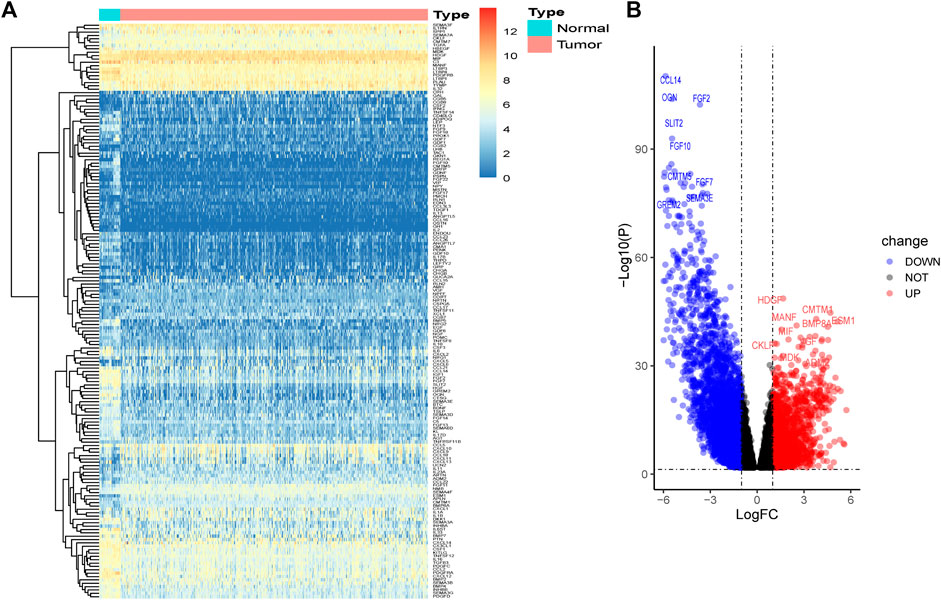
FIGURE 1. Identification of DE-IRGs between tumors and normal bladder tissues. (A) Heatmap of DE-IRGs. (B) Volcano plot of DE-IRGs.
Biological Characteristics of Differentially Expressed Immune-Related Genes
Pathway enrichment analysis was performed to interpret the biological function of the DE-IRGs. A total of 195 GO categories were found with an FDR of less than 0.05. The most significant terms are displayed in Figures 2A–C. The GO analysis results showed that the enriched biological process (BP) terms were muscle system process and axonogenesis. The enriched cellular component (CC) terms were related to collagen-containing extracellular matrix and synaptic membrane. Among molecular function (MF) terms, channel activity and passive transmembrane transporter activity were dominant. For the KEGG pathway analysis (23 terms, FDR <0.05), the top 20 enriched terms included neuroactive ligand–receptor interaction, calcium signaling pathway, and cAMP signaling pathway (Figure 2D). We further studied the relationship between target gene regulation and transcription factors. First, 97 differentially expressed transcription factor-related genes (DE-TFs) were obtained from the intersection of all DE-IRGs and transcription factor-related (TF) genes in BLCA. Univariate Cox proportional hazard regression analysis was conducted using the Coxph function in the “survival” R package, and p < 0.05 was selected as the threshold to filter data. Thirty-one OS-related and 27 DFS-related DE-IRGs were screened, and the regulatory network between these genes and DE-TFs (Supplementary Figure S2) was constructed using Cytoscape (v3.7.1) software (Cline et al., 2007).
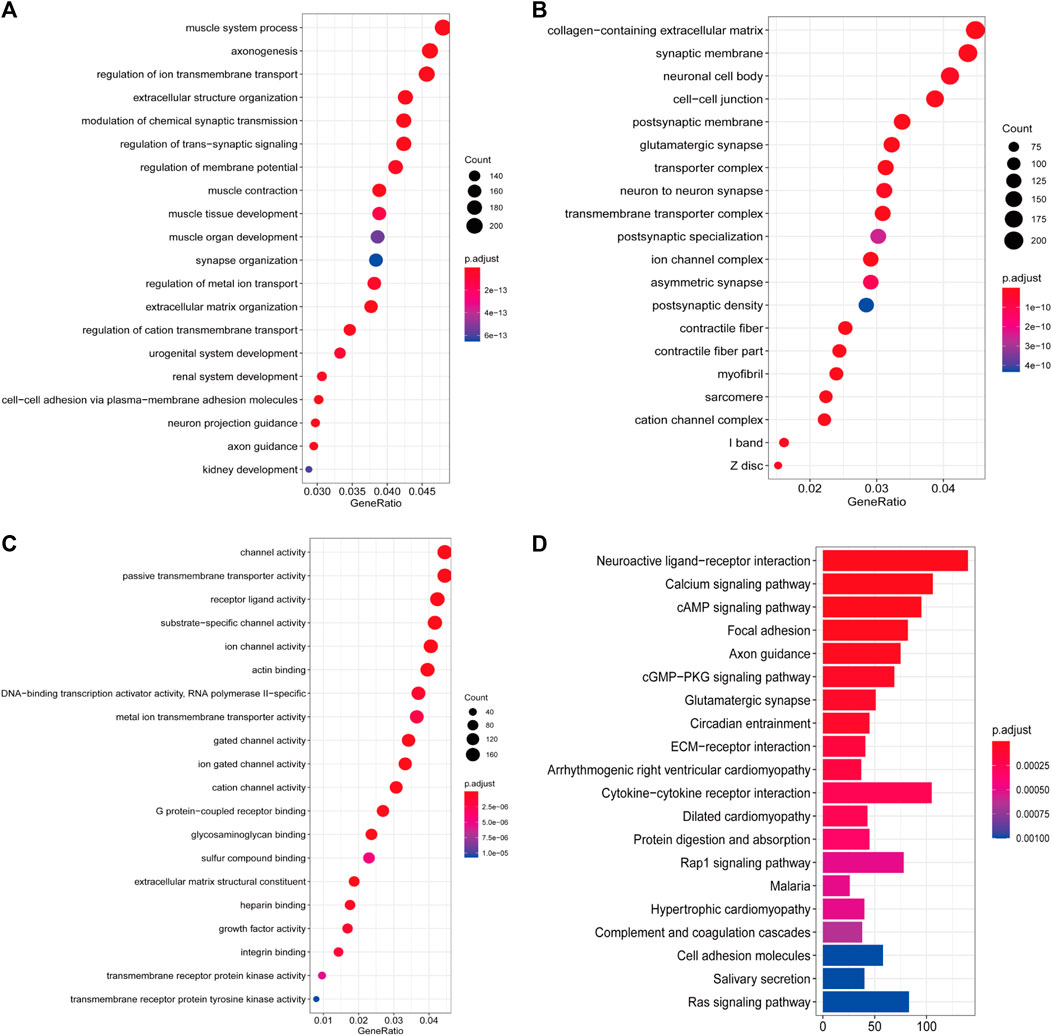
FIGURE 2. Enrichment analyses of DE-IRGs. (A) Top 20 most enriched BP categories. (B) Top 20 most enriched CC categories. (C) Top 20 most enriched MF categories. (D) Top 20 most enriched KEGG pathways.
Construction and Verification of Gene Immune Signatures in Bladder Urothelial Carcinoma
The training group was used to identify prognostic immune genes and construct prognostic risk models. The testing and entire groups were used to verify the predictive ability and robustness of the models. First, univariate Cox regression analysis was used to identify the candidate genes that are significantly associated with prognosis (p < 0.05). Sixteen genes were screened from 31 OS-related DE-IRGs, and 22 genes were screened from 27 DFS-related DE-IRGs. We then conducted LASSO Cox regression analysis to further compress the genes and found 13 OS-related IRGs and 15 DFS-related IRGs (Supplementary Figures S3, S4). Finally, multivariate Cox proportional hazard regression analysis was carried out, and two prediction models were established as follows:
For the 13 OS-IRGs: Risk score = (0.0252 × HGF) + (0.0014 × FGF9) + (0.1379 × INHBB) + (0.0791 × PLAU) + (0.0082 × IL17B) + (−0.0519 × CXCL5) + (0.0775 × SEMA4F) + (0.0446 × PDGFRB) + (0.0621 × LTBP1) + (−0.0545 × FGF18) + (−0.1726 × CCL17) + (0.0618 × CGB8) + (0.1069 × CCL26) + (0.0550 × EGF) + (−0.0196 × CXCL1).
For the 15 DFS-IRGs: Risk score = (0.0252 × HGF) + (0.0014 × FGF9) + (0.1379 × INHBB) + (0.0791 × PLAU) + (0.0082 × IL17B) + (−0.0519 × CXCL5) + (0.0775 × SEMA4F) + (0.0446 × PDGFRB) + (0.0621 × LTBP1) + (−0.0545 × FGF18) + (−0.1726 × CCL17) + (0.0618 × CGB8) + (0.1069 × CCL26) + (0.0550 × EGF) + (−0.0196 × CXCL1).
The risk score for each patient was calculated using these formulas. The patients with complete OS/DFS information were divided into the high-risk groups (OS: n = 294, DFS: n = 308) and low-risk groups (OS: n = 113, DFS: n = 86). The ROC analysis results revealed that the three groups had large areas under the curve (AUCs) for 1-, 3-, and 5-years survival (Figures 3A–C and Figures 4A–C). The risk score distribution maps (Figures 3D–F and Figures 4D–F) show a remarkable increase in the number of deaths in the high-risk group. This finding indicates that the samples with high-risk scores have a poor OS. In the training group, the Kaplan–Meier curves for OS and DFS consistently showed that patients in the low-risk group exhibited a better prognosis than patients in the high-risk group (Figure 3G and Figure 4G). The results were verified in the testing and entire groups (Figures 3H,I and Figures 4H,I). The results of the analyses of the three groups were consistent, which suggests the good predictive ability and robustness of the two models. In addition, the 13-IRG signature identified EGF, CCL26, FGF18, LTBP1, CXCL5, CGB5, IL17B, PDGFC, INHBB, MANF, and IGF1 as risk factors, and their high expression was associated with high risk. By contrast, RLN2 and NPFF were protective factors, and their high expression was associated with low risk. For the 15-IRG signature, 14 genes were protective factors (HGF, FGF9, INHBB, PLAU, IL17B, CXCL5, SEMA4F, PDGFRB, LTBP1, FGF18, CGB8, CCL26, EGF, and CXCL1), and one gene was identified to be a risk factor (CCL17).
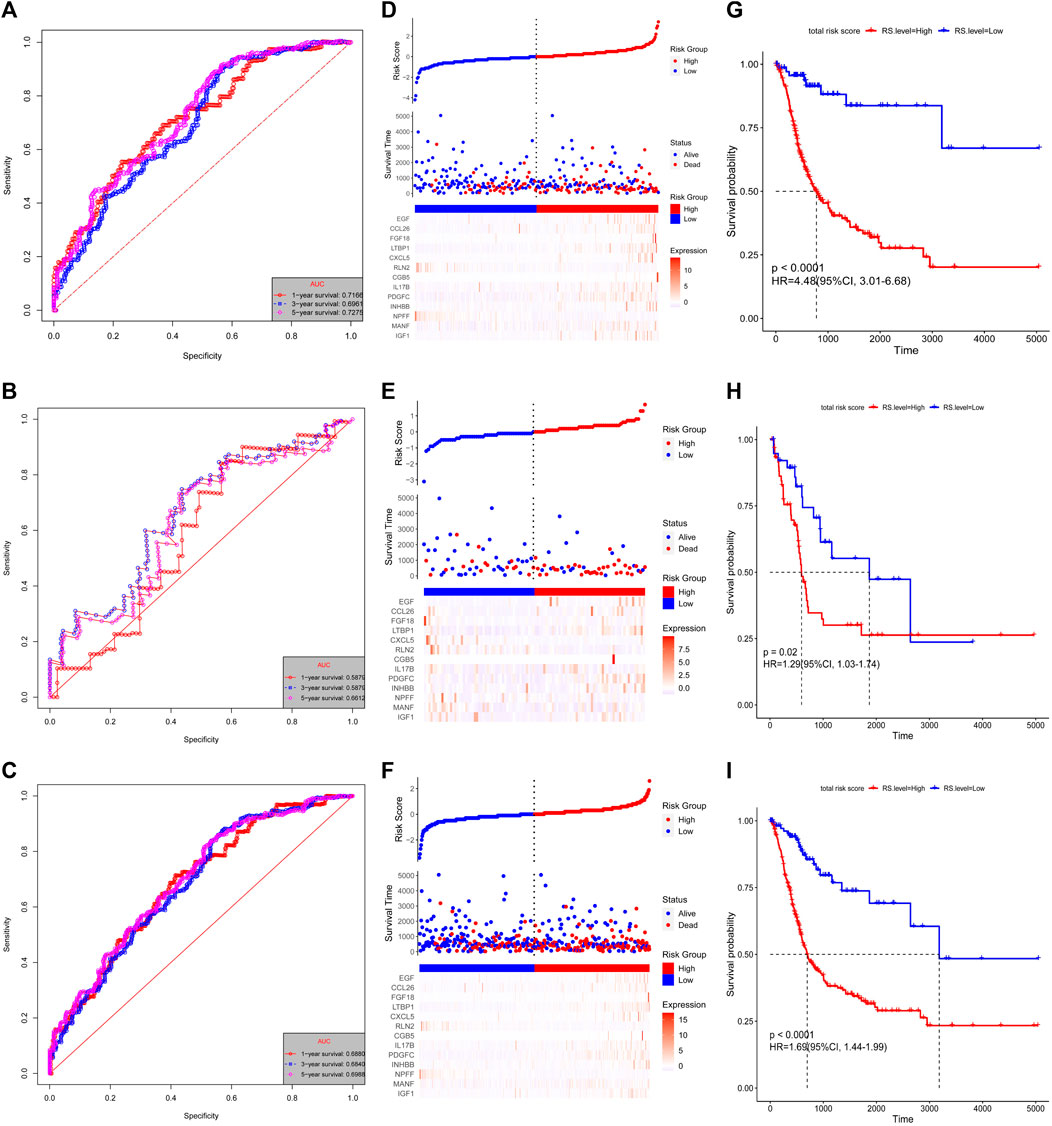
FIGURE 3. Validation of the 13-IRG signature. Kaplan–Meier estimates of the OS of the training group (A), testing group (B), and entire group (C). Risk score distributions and heatmaps of the mRNA expression of the 13-IRG signature in the training group (D), testing group (E), and entire group (F). Time-dependent ROC analyses of the 13-IRG signature in the training group (G), testing group (H), and entire group (I).
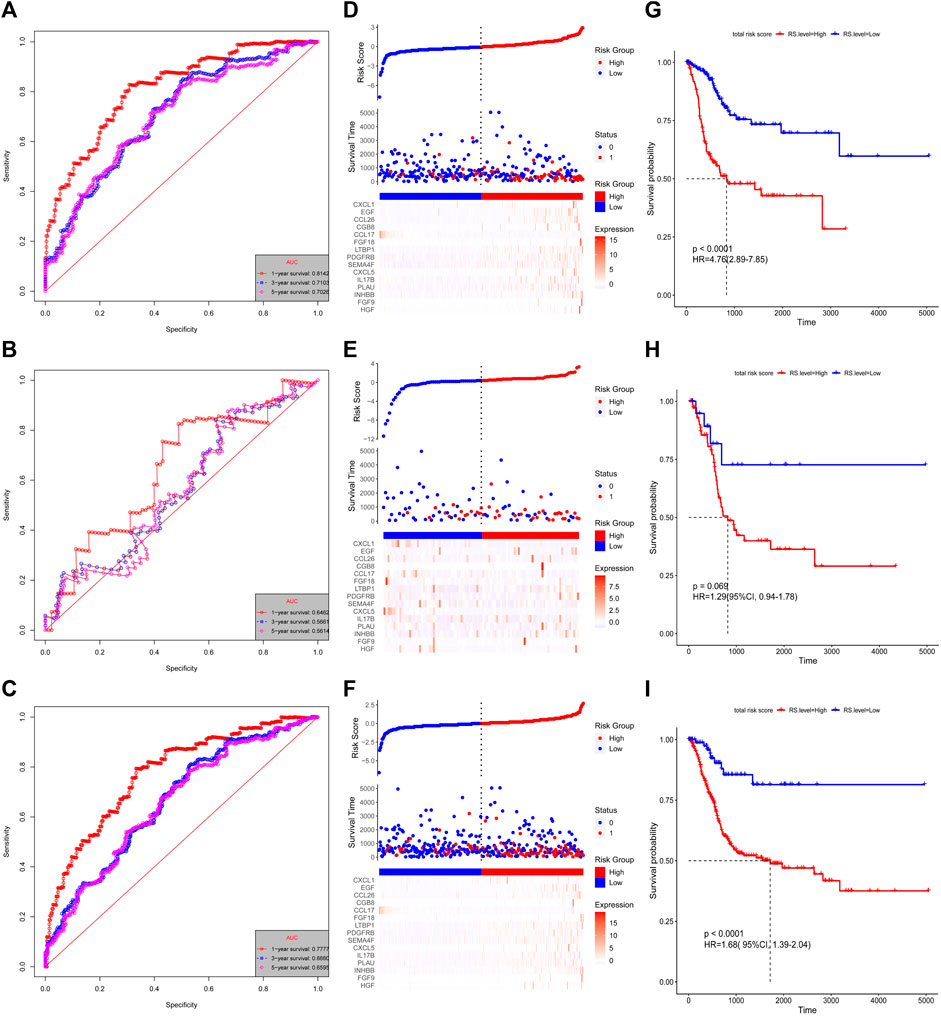
FIGURE 4. Validation of the 15-IRG signature. Kaplan–Meier estimates of the DFS of the training group (A), testing group (B), and entire group (C). Risk score distributions and heatmaps of the mRNA expression of the 15-IRG signature in the training group (D), testing group (E), and entire group (F). Time-dependent ROC analysis of the 15-IRG signature in the training group (G), testing group (H), and entire group (I).
Potential Biological Functions of Different Genes in High- and Low-Risk Groups
The KEGG pathway-based GSEA of the DEGs between the high- and low-risk groups revealed potential biological importance (the top 10 pathways are shown in Supplementary Figure S5). In OS and DFS models, “cell adhesion molecules cams,” “chemokine signaling pathway,” “cytokine–cytokine receptor interaction,” and “JAK–STAT signaling pathway” were enriched in both gene sets. Hence, the key DEGs may be involved in tumor metastasis and immunosuppression.
Prognostic Value of Immune Gene Signatures in Patients With Bladder Urothelial Carcinoma
Univariate and multivariate Cox regression analyses were conducted to systematically analyze the clinical information (including age, gender, T stage, N stage, M Stage, and smoking level) and risk score (high vs. low) of patients with BLCA in the training and entire groups (Table 2 and Supplementary Table S2). The univariate Cox regression analysis showed that a high-risk score was unfavorable for OS and DFS. The multivariate Cox regression analysis showed that the risk scores of the immune signatures were a remarkable predictor of survival. Therefore, our 13- and 15-IRG signatures had good predictive performance in the clinic.
A prognostic nomogram for OS/DFS with scales for three independent prognostic factors, including age, stage, and risk score, was constructed according to the results of the multivariate analysis. The nomogram displayed that risk score had the greatest impact on the prediction of survival rate (Figure 5A and Supplementary Figure S6A). This finding further confirmed that the immune signatures could predict prognosis. Calibration plots were used to visualize the predictive performance of the nomograms. Figure 5B shows that the calibration plots of 1-, 3-, and 5-years OS nomograms exhibited good performance and accurately estimated mortality. The DFS results are shown in Supplementary Figure S6B. The time-dependent ROC curves indicated the accuracy of the nomograms. The AUCs of 1-, 3-, and 5-years OS were 0.70, 0.653, and 0.723, respectively (Figure 5C), and the 1-, 3-, and 5-years DFS were 0.698, 0.751, and 0.70, respectively (Supplementary Figure S6C). In conclusion, risk score had better predictive ability compared with a single clinical factor. Moreover, the combined model of risk score and clinical factor showed the highest predictive accuracy.
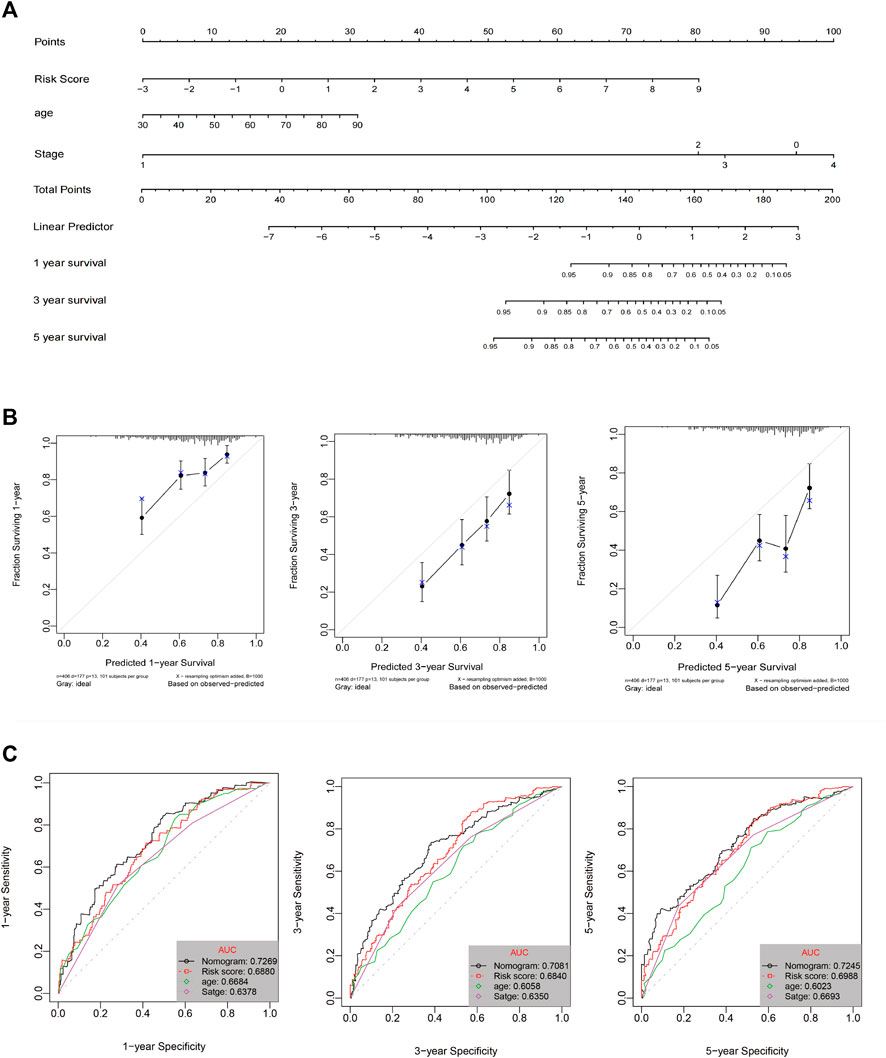
FIGURE 5. (A) Nomogram prediction of OS probability. (B) Calibration plots of the nomogram for 1-, 3-, and 5-years OS prediction. (C) Time-dependent ROC curves of 1-, 3-, and 5-years OS.
In addition, the relationship between the characteristics of the 13- and 15-IRG signatures and clinicopathological parameters was analyzed. The results suggest that the risk score of patients >60 years, female, with lymph node metastasis and/or distant metastasis, T3/T4, and stage III/IV is substantially increased (Figure 6).
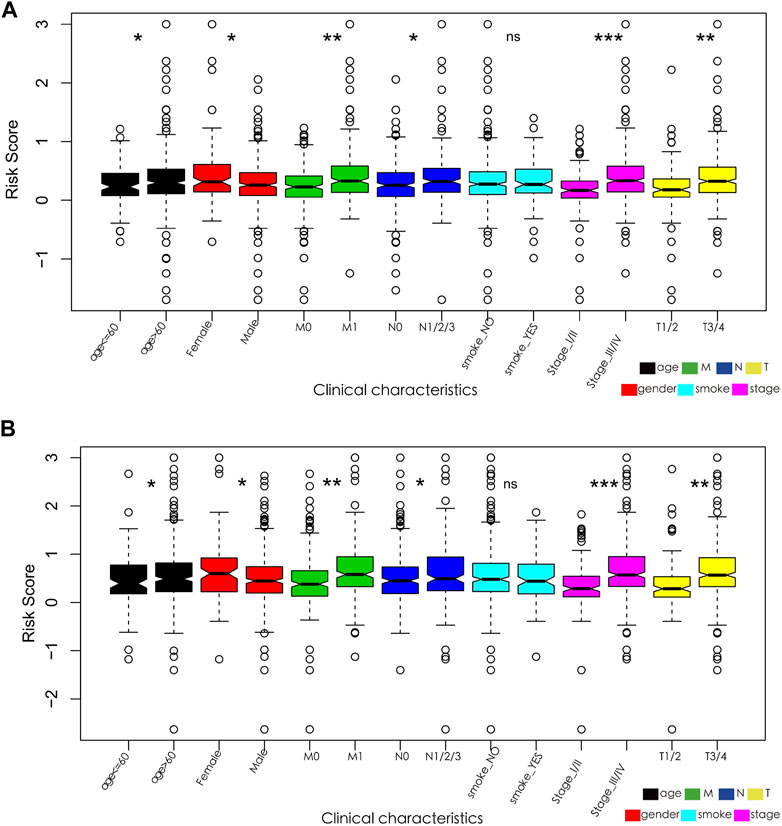
FIGURE 6. (A) Relationships between the 13-IRG signature and clinicopathological parameters. (B) Relationships between the 15-IRG signature and clinicopathological parameters.
Immune Gene Signatures and Tumor Immune Microenvironment
Our study revealed the possible interaction and correlation between these identified IRGs and the tumor immune microenvironment. Differences in immune cell infiltration were found between the high- and low-risk groups of the OS model (Figure 7). In the high-risk group, the proportions of naïve B cells, CD4 memory T cells, macrophage M0, macrophage M1, and neutrophils were considerably higher, whereas the abundance of memory B cells and T naïve CD4 cells increased substantially in the low-risk group. In the DFS model, CD4 memory T cells, macrophage M1, neutrophils, and memory B cells showed the same distribution characteristics (Supplementary Figure S7). Multiple innate immune-related cell types, including macrophage M0, macrophage M1, and neutrophils, were enriched in the high-risk group, which may indicate adverse clinical outcomes.
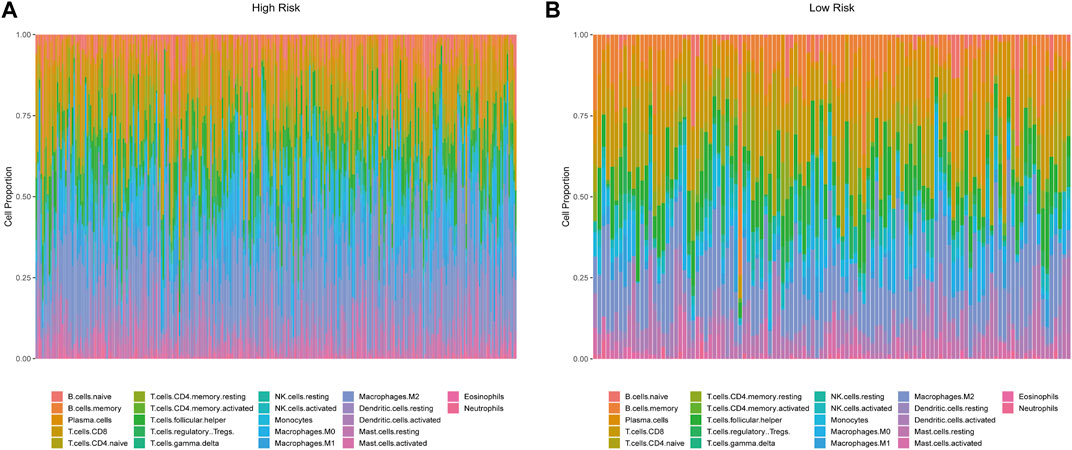
FIGURE 7. Characterization of immune cell infiltrate in high-risk (A) and low-risk groups (B) based on the 13-IRG signature.
Immune Gene Signatures and Tumor Mutation Burden
The frequency of mutation was high in all samples (>90%), and the main type of mutation was missense mutation. The mutation frequency of tumor suppressor gene, TP53 (tumor protein p53), was the highest in the high-risk group, whereas the mutation frequency of titin was the highest in the low-risk group (Figures 8A,B, Supplementary Figures S8A,B). The box plot of TMB scores shows that the low-risk group had higher TMB and longer OS than the high-risk group (Figure 8C), and the corresponding DFS model analysis did not indicate the same results (Supplementary Figure S8C). However, after stratification according to the TMB of the sample, the Kaplan–Meier survival curve demonstrated that the difference between the two groups was statistically significant (Figure 8D, Supplementary Figure S8D).
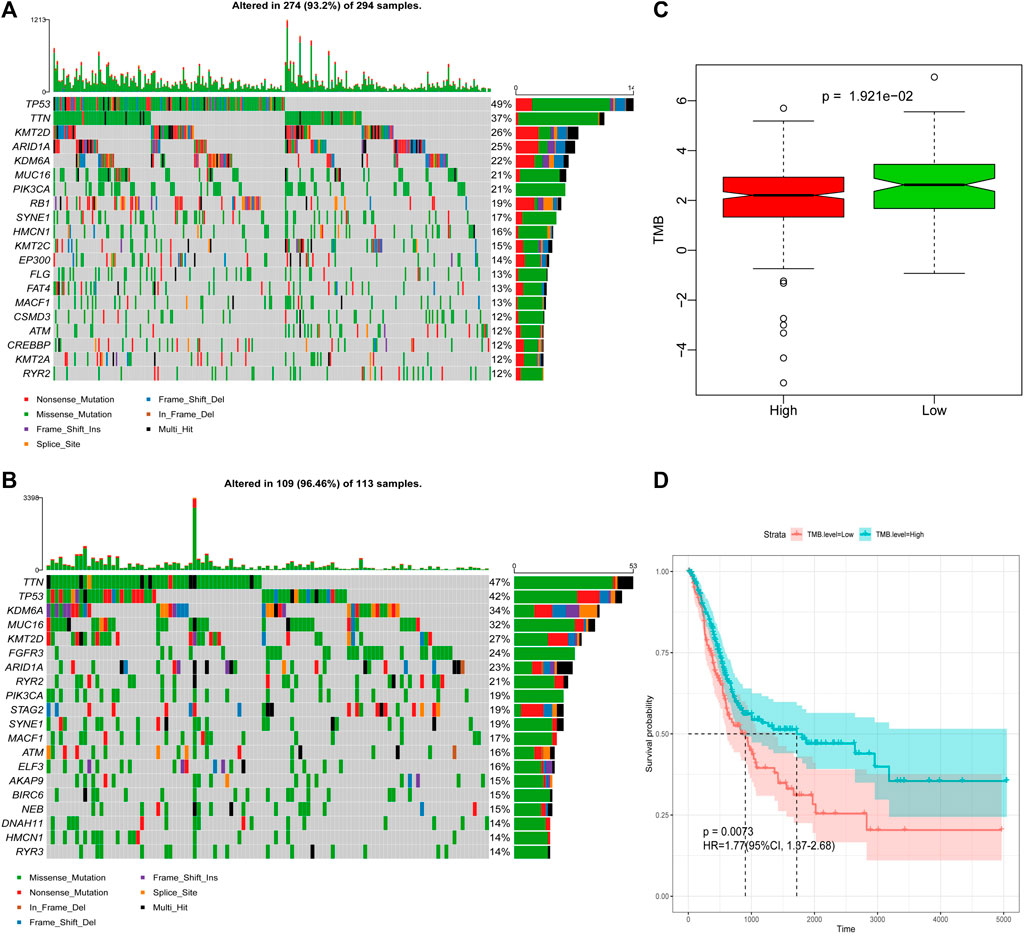
FIGURE 8. Differences in TMB between the high-risk and low-risk groups based on OS. (A) Mutation profile of high-risk group. (B) Mutation profile of low-risk group. (C) Box plot of TMB score in high-risk and low-risk groups. (D) Survival curves of high- and low-TMB score groups.
Prediction of Anti-PD-L1 Response With the Immune Gene Signatures
The analysis of the real ICI treatment cohort confirmed the predictive value of the immune signatures for checkpoint immunotherapy. We downloaded the gene expression profiles and clinical data of the IMvigor210 cohort. The IMvigor210 study is a single arm, multicenter, phase 2 clinical trial that investigated the clinical activity of PD-L1 blockade with atezolizumab in mUC. A total of 298 pre-treatment tumor samples were used for transcriptome RNA sequencing to evaluate the integrated biomarkers (Cline et al., 2007). Considering the absence of DFS data, we only explored the use of OS-related risk signature in predicting the benefit of anti-PD-L1 therapy for urothelial carcinoma. All samples were divided into high- and low-risk groups by the OS risk model. The risk score of patients with treatment response [complete response (CR) or partial response (PR)] was significantly lower than that of patients without treatment response [stable disease (SD) or progressive disease (PD); Wilcoxon, p = 2.076e-08; Figure 9A]. Moreover, the prognosis of the low-risk group was significantly better than that of the high-risk group (p = 0.0083, Figure 9B). After evaluating the distribution of CR/PR and SD/PD in the high- and low-risk groups, we found that patients with low-risk scores had better response to ICI treatment than patients with high-risk score (Figure 9C).
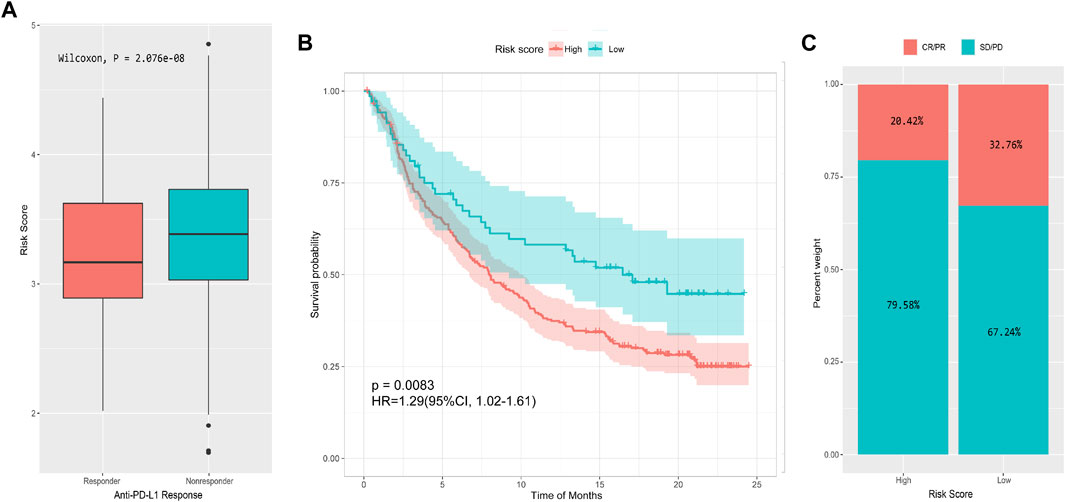
FIGURE 9. Performance of the 13-IRG signature in predicting response to anti-PD-L1 therapy in the IMvigor210 cohort. (A) Box plot of risk score and objective response to immunotherapy. (B) Survival curve of high- and low-risk groups. (C) Immunotherapy response rates of high- and low-risk groups.
Discussion
The main aim in this study was to construct a model using IRGs to predict the prognosis of patients BLCA, as well as the clinical benefit of immunotherapy. In addition, we performed GO and KEGG pathway enrichment analyses for the DE-IRGs and further screened the DE-IRGs associated with OS or DFS to construct network interaction relationships with DE-TFs to explore the possible biological mechanisms of DE-IRGs associated with prognosis. We constructed 13- and 15-IRG signatures using the RNA-Seq data of TCGA-BLCA and the immune-related gene set from the ImmPort database, respectively. The univariate Cox regression analysis showed that the risk scores of the immune signatures were an independent predictor of survival. We next constructed a prognostic nomogram of OS/DFS using three variables (age, stage, and risk score), which showed good discrimination and prediction ability. Besides, we also found that the low-risk score group had better response to ICI therapy than the high-risk score group, and the two groups showed differences in immune cell infiltration and TMB.
Previous studies indicated that IRGs are involved in shaping the immune landscape and influence the prognosis and response to immunotherapies of patients with tumor (Liu et al., 2020; Yi et al., 2021). In the present study, the IRGs identified were related to the progression of malignant tumors, and some of them can regulate the occurrence and development of cancer by simultaneously regulating the state of the tumor immune microenvironment and the malignant biological characteristics of tumor cells. Yeh et al. (2015) found that fibroblast ERα increased the expression of CCL cytokines and IL-6 in the tumor microenvironment and promoted bladder cancer invasion. CXCL5 upregulates the expression of MMP2/MMP9 by activating PI3K/AKT signal to promote the migration and invasion of bladder cancer cells (Gao et al., 2015). In addition, CXCL5 is involved in changing the tumor microenvironment of bladder cancer. The interaction between endothelial cells and bladder cancer cells potentiates the recruitment of vascular endothelial cells through the CXCL1/CXCL5/CXCL8–CXCR2 pathway, which leads to tumor progression (Gao et al., 2015). Furthermore, research had found that Salmonella local immune stimulation considerably enhanced the expression of complement component 5a, CXCL2, CXCL5, CCL5, and CCL8; thus, it recruits specific CD8 T cells and promotes bladder cancer progression (Domingos-Pereira et al., 2015). Similarly, based on the principle of gene–immune interaction, CXCL5 plays an important role in the progression of colon cancer, gastric cancer, liver cancer, and other tumors by recruiting or activating neutrophils. A large number of studies have reported that the abnormal expression of epidermal growth factor (EGF) and its receptor is involved in the invasion and metastasis of a variety of tumors (Ma et al., 2012; Perera and Bardeesy, 2012; Tomas et al., 2014; Chen et al., 2021), such as bladder cancer, gastric cancer, liver cancer, breast cancer, and melanoma. Heparin-binding EGF-like growth factor (HB-EGF) accumulates in the nucleus of invasive bladder transitional cell carcinoma, which can promote the autocrine cycle of cells, lead to the proliferation of cancer cells, and protect cancer cells from apoptosis (Kim et al., 2005). Moreover, as a powerful tumor growth and angiogenesis inducer, HB-EGF promotes the migration of bladder cancer cells by inducing MMP-9 and MMP-3 expression and activity (Ongusaha et al., 2004). Therefore, a prognosis model constructed using these genes would have relatively sufficient basis, and our follow-up study confirmed this hypothesis.
Considering that studying the biological mechanism and function of gene sets involved in specific pathways is an effective method for cancer research (Ge et al., 2018; Liu et al., 2018), we conducted a functional enrichment analysis of the identified DE-IRGs in BLCA tumor tissues. The KEGG analysis indicated that DEGs in the high- and low-risk groups were enriched in immune-related pathways, such as “cell adhesion molecules,” “chemokine signaling pathway,” “cytokine–cytokine receptor interaction,” “JAK–STAT signaling pathway,” “leukocyte transendothelial migration,” and “natural killer cell-mediated cytotoxicity.” The results may indicate that related pathways are involved in the shaping of the immune landscape. These results provide new insights into the potential biological mechanism and function of IRGs.
Based on the principle of gene–immune interaction, we designed OS and DFS immune signatures to predict the prognosis and response to ICI of patients with BLCA. According to the univariate and multivariate Cox regression analyses, the risk scores of the immune signatures were an independent prognostic indicator of OS/DFS for patients with BLCA; that is, patients with low immune signature scores have a better prognosis. The nomogram and ROC analyses further verified the prediction performance of the IRG signatures. Risk score was also remarkably correlated with sex, age, and TNM stage; that is, patients with worse clinicopathological characteristics had higher risk scores. Through comprehensive analysis, we demonstrated that the IRG signatures might be a suitable guide for clinicians in conducting the risk stratification of patients with BLCA and could help to adopt appropriate treatment modes.
Tumor cells escape from immune surveillance by suppressing the effect of T cells through the immune checkpoint, which leads to a decrease in tumor surveillance and tumor recognition and the occurrence of immune escape (Gervois et al., 1996). Two key immune checkpoint receptors, namely, cytotoxic T lymphocyte antigen 4 (CTLA-4) and PD-1, have been widely used in emerging immunotherapy. The accurate mode of action and the determination of predictive markers are key research topics because of the minority of patients who appear to benefit from immunotherapy. Several biomarkers have been developed at the genome, transcriptome, and immunogenome levels (Havel et al., 2019). The expression of PD-L1 in tumor cells is a biomarker for predicting response to anti-PD-1 or anti-PD-L1 therapy, but data remain insufficient. Patients who tested negative for PD-L1 can still achieve an objective remission rate of 11–20% (Mahoney and Atkins, 2014; Rui et al., 2019). Tumor-infiltrating lymphocytes (TILs) (Mahoney and Atkins, 2014; Rui et al., 2019), TMB (Yarchoan et al., 2017; Wang et al., 2020), and microsatellite instability (Pećina-Šlaus et al., 2020) are related to therapeutic response to ICI treatment. Our study found that the IRG signatures were associated with immune cell infiltration and TMB, and their predictive effects were verified in the immunotherapy cohort.
The enrichment of TIL subsets associated with adaptive immunity decreased with tumor progression, whereas that associated with innate immunity increased (Charoentong et al., 2017). Using our analytical strategy, we found a similar evolving nature in infiltrating immune cell components during tumor progression; that is, TIL subpopulations related to innate immunity, such as macrophage M0, macrophage M1, neutrophils, and resting dendritic cells, were enriched in the high-risk group, whereas TILs related to adaptive immunity, such as memory B cells, naive CD4 T cells, and gamma delta T cells (Tγδ), were enriched in the low-risk group. Therefore, we speculate that the 13- and 15-IRG sets for model construction may change the biological behavior and therapeutic response of tumor cells by changing the tumor microenvironment. The predictive role of TMB in ICI treatment has been confirmed in many clinical studies (Davoli et al., 2017). Tumor cells with high TMB can produce more tumor-specific antigens and thus can be easily recognized and killed by immune cells (Kakoti et al., 2020). In our OS model, the TMB of the low-risk group was higher and the prognosis of the high-TMB group was remarkably better after the samples were grouped according to TMB. This finding suggests that a low-risk score predicts a good response rate to PD-1 inhibition and satisfactory clinical outcomes.
The IMvigor210 cohort study confirmed the above results. Compared with the PD-1 treatment nonresponse group, the risk score of the response group was considerably lower, and the low-risk group had a higher response to anti-PD-1 treatment and better prognosis. These data further supported that the IRG signatures may serve as a biomarker to predict the prognosis of patients with BLCA and their response to immunotherapy.
This study has some limitations. First, the 13- and 15-IRG signatures only used a series of immune genes, which are nonspecific to the specific immune microenvironment of patients with urothelial carcinoma. Second, the factors influencing tumor progression and immunotherapy are very complex, and the influence of immune genes may only be a part of them. Third, basic experiments and studies with larger sample sizes are needed to verify these associations. Despite these limitations, our analysis showed that the 13- and 15-IRG signatures can effectively predict the prognosis and response to ICI of patients with BLCA.
In summary, the 13- and 15-IRG signatures may be helpful to determine the prognosis of patients with BLCA and stratify those who will benefit from checkpoint blockade immunotherapy. This finding may contribute to cancer immunotherapy and promote the development of precise immune oncology.
Data Availability Statement
The datasets presented in this study can be found in online repositories. The names of the repository/repositories and accession number(s) can be found in the article/Supplementary Material.
Ethics Statement
The studies involving human participants were reviewed and approved by the Ethics and Human Subject Committee of Guangxi Medical University Cancer Hospital. Written informed consent for participation was not required for this study in accordance with the national legislation and the institutional requirements.
Author Contributions
FL and YX designed the study. FL and HZ carried out the data analysis. FL and YC interpreted the entire results and drafted the manuscript. YX, ZW, and TN helped carry out the data analysis. JZ reviewed the manuscript. All authors read and approved the final manuscript.
Funding
This study was funded by Guangxi Medical University First Affiliated Hospital.
Conflict of Interest
The authors declare that the research was conducted in the absence of any commercial or financial relationships that could be construed as a potential conflict of interest.
Publisher’s Note
All claims expressed in this article are solely those of the authors and do not necessarily represent those of their affiliated organizations, or those of the publisher, the editors and the reviewers. Any product that may be evaluated in this article, or claim that may be made by its manufacturer, is not guaranteed or endorsed by the publisher.
Acknowledgments
We sincerely acknowledge all the online databases for the availability of the data.
Supplementary Material
The Supplementary Material for this article can be found online at: https://www.frontiersin.org/articles/10.3389/fmolb.2021.673918/full#supplementary-material
Abbreviations
AUCs, areas under the curve; BLCA, bladder urothelial carcinoma; BP, biological process; CR, complete response; CTLA-4, cytotoxic T lymphocyte antigen 4; CC, cellular component; DEGs, differentially expressed genes; DE-IRGs, differentially expressed immune-related genes; DFS, disease-free survival; DE-TFs, differentially expressed transcription factor-related genes; EGF, epidermal growth factor; GSEA, gene set enrichment analysis; GTEx, Genotype-Tissue Expression; HB-EGF, Heparin-binding EGF; IRGs, immune-related genes; ICIs, immune checkpoint inhibitors; LASSO, least absolute shrinkage and selection operator; MF, molecular function; mUC, metastatic urothelial cancer; PR, partial response; PD, progressive disease; ROC, receiver operating characteristic; SD, stable disease; TMB, tumor mutation burden; TCGA, The Cancer Genome Atlas; TF, transcription factor-related; TILs, Tumor-infiltrating lymphocytes.
References
Apolo, A. B., Infante, J. R., Balmanoukian, A., Patel, M. R., Wang, D., Kelly, K., et al. (2017). Avelumab, an Anti-programmed Death-Ligand 1 Antibody, in Patients with Refractory Metastatic Urothelial Carcinoma: Results from a Multicenter, Phase Ib Study. J. Clin. Oncol. 35, 2117–2124. doi:10.1200/JCO.2016.71.6795
Balar, A. V., Castellano, D., O'Donnell, P. H., Grivas, P., Vuky, J., Powles, T., et al. (2017a). First-line Pembrolizumab in Cisplatin-Ineligible Patients with Locally Advanced and Unresectable or Metastatic Urothelial Cancer (KEYNOTE-052): A Multicentre, Single-Arm. Lancet Oncol. (18), 1483–1492. doi:10.1016/s1470-2045(17)30616-2
Balar, A. V., Galsky, M. D., Rosenberg, J. E., Powles, T., Petrylak, D. P., Bellmunt, J., et al. (2017b). Atezolizumab as First-Line Treatment in Cisplatin-Ineligible Patients with Locally Advanced and Metastatic Urothelial Carcinoma: A Single-Arm. multicentre (389), 67–76. doi:10.1016/s0140-6736(16)32455-2
Bellmunt, J., de Wit, R., Vaughn, D. J., Fradet, Y., Lee, J.-L., Fong, L., et al. (2017). Pembrolizumab as Second-Line Therapy for Advanced Urothelial Carcinoma. N. Engl. J. Med. 376, 1015–1026. doi:10.1056/nejmoa1613683
Bellmunt, J., and Nadal, R. (2018). Changes in Expectations for Metastatic Urothelial Carcinoma. Nat. Rev. Clin. Oncol. 15 (2), 73–74. doi:10.1038/nrclinonc.2017.184
Bhattacharya, S., Andorf, S., Gomes, L., Dunn, P., Schaefer, H., Pontius, J., et al. (2014). ImmPort: Disseminating Data to the Public for the Future of Immunology. Immunol. Res. 58 (2-3), 234–239. doi:10.1007/s12026-014-8516-1
Charoentong, P., Finotello, F., Angelova, M., Mayer, C., Efremova, M., Rieder, D., et al. (2017). Pan-cancer Immunogenomic Analyses Reveal Genotype-Immunophenotype Relationships and Predictors of Response to Checkpoint Blockade. Cel Rep. 18 (1), 248–262. doi:10.1016/j.celrep.2016.12.019
Chen, B., Khodadoust, M. S., Liu, C. L., Newman, A. M., and Alizadeh, A. A. (2018). Profiling Tumor Infiltrating Immune Cells with CIBERSORT. Methods Mol. Biol. (Clifton, N.J.). 1711, 243–259. doi:10.1007/978-1-4939-7493-1_12
Chen, Y., Qian, B., Sun, X., Kang, Z., Huang, Z., Ding, Z., et al. (2021). Sox9/INHBB axis-mediated Crosstalk between the Hepatoma and Hepatic Stellate Cells Promotes the Metastasis of Hepatocellular Carcinoma. Cancer Lett. 499, 243–254. doi:10.1016/j.canlet.2020.11.025
Cline, M. S., Smoot, M., Cerami, E., Kuchinsky, A., Landys, N., Workman, C., et al. (2007). Integration of Biological Networks and Gene Expression Data Using Cytoscape. Nat. Protoc. 2 (10), 2366–2382. doi:10.1038/nprot.2007.324
Davoli, T., Uno, H., Wooten, E. C., and Elledge, S. J. (2017). Tumor Aneuploidy Correlates with Markers of Immune Evasion and with Reduced Response to Immunotherapy. Science 355 (6322). doi:10.1126/science.aaf8399
Domingos-Pereira, S., Hojeij, R., Reggi, E., Derré, L., Chevalier, M. F., Romero, P., et al. (2015). LocalSalmonellaimmunostimulation Recruits Vaccine-specific CD8 T Cells and Increases Regression of Bladder Tumor. Oncoimmunology 4 (7), e1016697. doi:10.1080/2162402x.2015.1016697
Dudziec, E., Goepel, J. R., and Catto, J. W. (2011). Global Epigenetic Profiling in Bladder Cancer. Epigenomics 3 (1), 35–45. doi:10.2217/epi.10.71
Felsenstein, K. M., and Theodorescu, D. (2018). Precision Medicine for Urothelial Bladder Cancer: Update on Tumour Genomics and Immunotherapy. Nat. Rev. Urol. 15 (2), 92–111. doi:10.1038/nrurol.2017.179
Fitzmaurice, C., Fitzmaurice, C., Abate, D., Abbasi, N., Abbastabar, H., Abd-Allah, F., et al. (2019). Global, Regional, and National Cancer Incidence, Mortality, Years of Life Lost, Years Lived with Disability, and Disability-Adjusted Life-Years for 29 Cancer Groups, 1990 to 2017: A Systematic Analysis for the Global burden of Disease Study. JAMA Oncol. 5 (12), 1749–1768. doi:10.1001/jamaoncol.2019.2996
Gao, Y., Guan, Z., Chen, J., Xie, H., Yang, Z., Fan, J., et al. (2015). CXCL5/CXCR2 axis Promotes Bladder Cancer Cell Migration and Invasion by Activating PI3K/AKT-Induced Upregulation of MMP2/MMP9. Int. J. Oncol. 47 (2), 690–700. doi:10.3892/ijo.2015.3041
Ge, Z., Leighton, J. S., Wang, Y., Peng, X., Chen, Z., Chen, H., et al. (2018). Integrated Genomic Analysis of the Ubiquitin Pathway across Cancer Types. Cell Rep 23 (1), 213–e3. doi:10.1016/j.celrep.2018.03.047
Gervois, N., Guilloux, Y., Diez, E., and Jotereau, F. (1996). Suboptimal Activation of Melanoma Infiltrating Lymphocytes (TIL) Due to Low Avidity of TCR/MHC-tumor Peptide Interactions. J. Exp. Med. 183 (5), 2403–2407. doi:10.1084/jem.183.5.2403
Goeman, J. J. (2010). L1 Penalized Estimation in the Cox Proportional Hazards Model. Biom J. 52, 70–84. doi:10.1084/jem.183.5.2403
Groenendijk, F. H., de Jong, J., Fransen van de Putte, E. E., Michaut, M., Schlicker, A., Peters, D., et al. (2016). ERBB2 Mutations Characterize a Subgroup of Muscle-Invasive Bladder Cancers with Excellent Response to Neoadjuvant Chemotherapy. Eur. Urol. 69 (3), 384–388. doi:10.1016/j.eururo.2015.01.014
Gros, A., Parkhurst, M. R., Tran, E., Pasetto, A., Robbins, P. F., Ilyas, S., et al. (2016). Prospective Identification of Neoantigen-specific Lymphocytes in the Peripheral Blood of Melanoma Patients. Nat. Med. 22 (4), 433–438. doi:10.1038/nm.4051
Hänzelmann, S., Castelo, R., and Guinney, J. (2013). GSVA: Gene Set Variation Analysis for Microarray and RNA-Seq Data. BMC Bioinformatics 14, 7. doi:10.1186/1471-2105-14-7
Havel, J. J., Chowell, D., and Chan, T. A. (2019). The Evolving Landscape of Biomarkers for Checkpoint Inhibitor Immunotherapy. Nat. Rev. Cancer 19 (3), 133–150. doi:10.1038/s41568-019-0116-x
Huang, Z., Zhang, M., Chen, G., Wang, W., Zhang, P., Yue, Y., et al. (2019). Bladder Cancer Cells Interact with Vascular Endothelial Cells Triggering EGFR Signals to Promote Tumor Progression. Int. J. Oncol. 54 (5), 1555–1566. doi:10.3892/ijo.2019.4729
Kakoti, S., Sato, H., Laskar, S., Yasuhara, T., and Shibata, A. (2020). DNA Repair and Signaling in Immune-Related Cancer Therapy. Front. Mol. Biosci. 7, 205. doi:10.3389/fmolb.2020.00205
Kamat, A. M., Flaig, T. W., Grossman, H. B., Konety, B., Lamm, D., O'Donnell, M. A., et al. (2015). Expert Consensus Document: Consensus Statement on Best Practice Management Regarding the Use of Intravesical Immunotherapy with BCG for Bladder Cancer. London, England: Reprinted, 225–235. doi:10.1038/nrurol.2015.58
Kamat, A. M., Hahn, N. M., Efstathiou, J. A., Lerner, S. P., Malmström, P.-U., Choi, W., et al. (2016). Bladder Cancer. The Lancet 388 (10061), 2796–2810. doi:10.1016/S0140-6736(16)30512-8
Kim, J., Adam, R. M., and Freeman, M. R. (2005). Trafficking of Nuclear Heparin-Binding Epidermal Growth Factor-like Growth Factor into an Epidermal Growth Factor Receptor-dependent Autocrine Loop in Response to Oxidative Stress. Cancer Res. 65 (18), 8242–8249. doi:10.1158/0008-5472.can-05-0942
Li, J., Lou, Y., Li, S., Sheng, F., Liu, S., Du, E., et al. (2020). Identification and Immunocorrelation of Prognosis-Related Genes Associated with Development of Muscle-Invasive Bladder Cancer. Front. Mol. Biosci. 7, 598599. doi:10.3389/fmolb.2020.598599
Li, X., Wang, M., Gong, T., Lei, X., Hu, T., Tian, M., et al. (2020). A S100A14-Ccl2/cxcl5 Signaling axis Drives Breast Cancer Metastasis. Theranostics 10 (13), 5687–5703. doi:10.7150/thno.42087
Lin, Y., Cheng, L., Liu, Y., Wang, Y., Wang, Q., Wang, H. L., et al. (2021). Intestinal Epithelium-Derived BATF3 Promotes Colitis-Associated colon Cancer through Facilitating CXCL5-Mediated Neutrophils Recruitment. Mucosal Immunol. 14 (1), 187–198. doi:10.1038/s41385-020-0297-3
Liu, C.-J., Hu, F.-F., Xia, M.-X., Han, L., Zhang, Q., and Guo, A.-Y. (2018). GSCALite: A Web Server for Gene Set Cancer Analysis. Bioinformatics (Oxford, England) 34 (21), 3771–3772. doi:10.1093/bioinformatics/bty411
Liu, J., Meng, H., Nie, S., Sun, Y., Jiang, P., Li, S., et al. (2020). Identification of a Prognostic Signature of Epithelial Ovarian Cancer Based on Tumor Immune Microenvironment Exploration. Genomics 112 (6), 4827–4841. doi:10.1016/j.ygeno.2020.08.027
Ma, L., Lan, F., Zheng, Z., Xie, F., Wang, L., Liu, W., et al. (2012). Epidermal Growth Factor (EGF) and Interleukin (IL)-1β Synergistically Promote ERK1/2-Mediated Invasive Breast Ductal Cancer Cell Migration and Invasion. Mol. Cancer 11, 79. doi:10.1186/1476-4598-11-79
Mahoney, K. M., and Atkins, M. B. (2014). Prognostic and Predictive Markers for the New Immunotherapies. Oncology (Williston Park) 28 Suppl 3 (Suppl. 3), 39–48.
Mao, Z., Zhang, J., Shi, Y., Li, W., Shi, H., Ji, R., et al. (2020). CXCL5 Promotes Gastric Cancer Metastasis by Inducing Epithelial-Mesenchymal Transition and Activating Neutrophils. Oncogenesis 9 (7), 63. doi:10.1038/s41389-020-00249-z
Mariathasan, S., Turley, S. J., Nickles, D., Castiglioni, A., Yuen, K., Wang, Y., et al. (2018). TGFβ Attenuates Tumour Response to PD-L1 Blockade by Contributing to Exclusion of T Cells. Nature 554 (7693), 544–548. doi:10.1038/nature25501
Mayakonda, A., Lin, D.-C., Assenov, Y., Plass, C., and Koeffler, H. P. (2018). Maftools: Efficient and Comprehensive Analysis of Somatic Variants in Cancer. Genome Res. 28 (11), 1747–1756. doi:10.1101/gr.239244.118
Mei, S., Meyer, C. A., Zheng, R., Qin, Q., Wu, Q., Jiang, P., et al. (2017). Cistrome Cancer: A Web Resource for Integrative Gene Regulation Modeling in Cancer. Cancer Res. 77 (21), e19–e22. doi:10.1158/0008-5472.CAN-17-0327
Network, T. C. G. A. (2014). Comprehensive Molecular Characterization of Urothelial Bladder Carcinoma. Nature 507 (7492), 315–322. doi:10.1038/nature12965
Newman, A. M., Liu, C. L., Green, M. R., Gentles, A. J., Feng, W., Xu, Y., et al. (2015). Robust Enumeration of Cell Subsets from Tissue Expression Profiles. Nat. Methods 12 (5), 453–457. doi:10.1038/nmeth.3337
Ongusaha, P. P., Kwak, J. C., Zwible, A. J., Macip, S., Higashiyama, S., Taniguchi, N., et al. (2004). HB-EGF Is a Potent Inducer of Tumor Growth and Angiogenesis. Cancer Res. 64 (15), 5283–5290. doi:10.1158/0008-5472.can-04-0925
Pećina-Šlaus, N., Kafka, A., Salamon, I., and Bukovac, A. (2020). Mismatch Repair Pathway, Genome Stability and Cancer. Front. Mol. Biosci. 7, 122. doi:10.3389/fmolb.2020.00122
Peng, D., Ge, G., Xu, Z., Ma, Q., Shi, Y., Zhou, Y., et al. (2018). Diagnostic and Prognostic Biomarkers of Common Urological Cancers Based on Aberrant DNA Methylation. Epigenomics 10 (9), 1189–1199. doi:10.2217/epi-2018-0017
Perera, R. M., and Bardeesy, N. (2012). Ready, Set, GoThe EGF Receptor at the Pancreatic Cancer Starting Line, 281–282. doi:10.1016/j.ccr.2012.08.019
Petrylak, D. P., de Wit, R., Chi, K. N., Drakaki, A., Sternberg, C. N., Nishiyama, H., et al. (2020). Ramucirumab Plus Docetaxel versus Placebo Plus Docetaxel in Patients with Locally Advanced or Metastatic Urothelial Carcinoma after Platinum-Based Therapy (RANGE): Overall Survival and Updated Results of a Randomised, Double-Blind, 105–120. (Reprinted.
Powles, T., O’Donnell, P. H., Massard, C., Arkenau, H.-T., Friedlander, T. W., Hoimes, C. J., et al. (2017). Efficacy and Safety of Durvalumab in Locally Advanced or Metastatic Urothelial Carcinoma. JAMA Oncol. 3, e172411. doi:10.1001/jamaoncol.2017.2411
Ritchie, M. E., Phipson, B., Wu, D., Hu, Y., Law, C. W., Shi, W., et al. (2015). Limma powers Differential Expression Analyses for RNA-Sequencing and Microarray Studies. Nucleic Acids Res. 43 (7), e47. doi:10.1093/nar/gkv007
Rosenberg, J. E., Hoffman-Censits, J., Powles, T., van der Heijden, M. S., Balar, A. V., Necchi, A., et al. (2016). Atezolizumab in Patients with Locally Advanced and Metastatic Urothelial Carcinoma Who Have Progressed Following Treatment with Platinum-Based Chemotherapy: A Single-ArmMulticentre, Phase 2 Trial, 1909–1920. (Reprinted. doi:10.1016/s0140-6736(16)00561-4
Rui, X., Gu, T.-T., Pan, H.-F., and Zhang, H.-Z. (2019). Evaluation of PD-L1 Biomarker for Immune Checkpoint Inhibitor (PD-1/pd-L1 Inhibitors) Treatments for Urothelial Carcinoma Patients: A Meta-Analysis. Int. Immunopharmacology 67, 378–385. doi:10.1016/j.intimp.2018.12.018
Schulz, W. A., and Goering, W. (2016). DNA Methylation in Urothelial Carcinoma. Epigenomics 8 (10), 1415–1428. doi:10.2217/epi-2016-0064
Sharma, P., Callahan, M. K., Bono, P., Kim, J., Spiliopoulou, P., Calvo, E., et al. (2016). Nivolumab Monotherapy in Recurrent Metastatic Urothelial Carcinoma (CheckMate 032): A Multicentre, Open-Label, Two-Stage, Multi-Arm, Phase 1/2 Trial, 1590–1598. doi:10.1016/s1470-2045(16)30496-x
Simon, N., Friedman, J., Hastie, T., and Tibshirani, R. (2011). Regularization Paths for Cox's Proportional Hazards Model via Coordinate Descent. J. Stat. Softw. 39 (5), 1–13. doi:10.18637/jss.v039.i05
Sweis, R. F., Spranger, S., Bao, R., Paner, G. P., Stadler, W. M., Steinberg, G., et al. (2016). Molecular Drivers of the Non-T-cell-inflamed Tumor Microenvironment in Urothelial Bladder Cancer. Cancer Immunol. Res. 4 (7), 563–568. doi:10.1158/2326-6066.CIR-15-0274
Tibshirani, R. (1996). Regression Shrinkage and Selection via the Laaso. J. R. Stat. Soc. Ser. B (Methodological) 58 (No. 1), 267–288. doi:10.1111/j.2517-6161.1996.tb02080.x
Tomas, A., Futter, C. E., and Eden, E. R. (2014). EGF Receptor Trafficking: Consequences for Signaling and Cancer. Trends Cel Biol. 24 (1), 26–34. doi:10.1016/j.tcb.2013.11.002
Tumeh, P. C., Harview, C. L., Yearley, J. H., Shintaku, I. P., Taylor, E. J. M., Robert, L., et al. (2014). PD-1 Blockade Induces Responses by Inhibiting Adaptive Immune Resistance. Nature 515, 568–571. doi:10.1038/nature13954
Vlachostergios, P. J., and Faltas, B. M. (2018). Treatment Resistance in Urothelial Carcinoma: An Evolutionary Perspective. Nat. Rev. Clin. Oncol. 15 (8), 495–509. doi:10.1038/s41571-018-0026-y
Wang, Y., Chen, L., Ju, L., Xiao, Y., and Wang, X. (2020). Tumor Mutational burden Related Classifier Is Predictive of Response to PD-L1 Blockade in Locally Advanced and Metastatic Urothelial Carcinoma. Int. Immunopharmacology 87, 106818. doi:10.1016/j.intimp.2020.106818
Xia, J., Xu, X., Huang, P., He, M., and Wang, X. (2015). The Potential of CXCL5 as a Target for Liver Cancer - what Do We Know So Far. Expert Opin. Ther. Targets 19, 141–146. doi:10.1517/14728222.2014.993317
Xu, N., Ke, Z.-B., Lin, X.-D., Chen, Y.-H., Wu, Y.-P., Chen, Y., et al. (2020). Development and Validation of a Molecular Prognostic index of Bladder Cancer Based on Immunogenomic Landscape Analysis. Cancer Cel Int 20, 302. doi:10.1186/s12935-020-01343-3
Yang, S., Wu, Y., Deng, Y., Zhou, L., Yang, P., Zheng, Y., et al. (2019). Identification of a Prognostic Immune Signature for Cervical Cancer to Predict Survival and Response to Immune Checkpoint Inhibitors. Oncoimmunology 8 (12), e1659094. doi:10.1080/2162402X.2019.1659094
Yarchoan, M., Hopkins, A., and Jaffee, E. M. (2017). Tumor Mutational Burden and Response Rate to PD-1 Inhibition. N. Engl. J. Med. 377, 2500–2501. doi:10.1056/nejmc1713444
Yeh, C. R., Hsu, I., Song, W., Chang, H., Miyamoto, H., Xiao, G. Q., et al. (2015). Fibroblast ERα Promotes Bladder Cancer Invasion via Increasing the CCL1 and IL-6 Signals in the Tumor Microenvironment. Am. J. Cancer Res. 5 (3), 1146–1157.
Yi, M., Li, A., Zhou, L., Chu, Q., Luo, S., and Wu, K. (2021). Immune Signature-Based Risk Stratification and Prediction of Immune Checkpoint Inhibitor's Efficacy for Lung Adenocarcinoma. Cancer Immunol. Immunother. 70 (6), 1705–1719. doi:10.1007/s00262-020-02817-z
Keywords: bladder urothelial carcinoma, immune-related genes, tumor immune microenvironment, immune checkpoint inhibitor, immunotherapy, prognostic model
Citation: Liang F, Xu Y, Chen Y, Zhong H, Wang Z, Nong T and Zhong J (2021) Immune Signature-Based Risk Stratification and Prediction of Immunotherapy Efficacy for Bladder Urothelial Carcinoma. Front. Mol. Biosci. 8:673918. doi: 10.3389/fmolb.2021.673918
Received: 28 February 2021; Accepted: 15 November 2021;
Published: 24 December 2021.
Edited by:
Shengtao Zhou, Sichuan University, ChinaReviewed by:
Sanjay Mishra, The Ohio State University, United StatesPaula Dobosz, MNM Diagnostics Sp. z o. o., Poland
Copyright © 2021 Liang, Xu, Chen, Zhong, Wang, Nong and Zhong. This is an open-access article distributed under the terms of the Creative Commons Attribution License (CC BY). The use, distribution or reproduction in other forums is permitted, provided the original author(s) and the copyright owner(s) are credited and that the original publication in this journal is cited, in accordance with accepted academic practice. No use, distribution or reproduction is permitted which does not comply with these terms.
*Correspondence: Jincai Zhong, MTM5MDc3MTk4NjNAMTYzLmNvbQ==
†These authors have contributed equally to this work and share first authorship