- Department of Plastic Surgery, The First Affiliated Hospital of China Medical University, Shenyang, China
Background: The purpose of our research was to establish a gene signature and determine the prognostic value of m6A methylation regulators in cutaneous melanoma and WTAP as a protective gene in cutaneous melanoma prognosis, we also evaluated gene mutations in cutaneous melanoma.
Methods: We downloaded the RNA-seq transcriptome data and the clinical information for cutaneous melanoma patients from the GTEx and TCGA databases. Consensus clustering analysis was applied to divide the samples into two groups. Then the least absolute shrinkage and selection operator (LASSO) analyses were conducted to construct a risk signature, and we use external and internal datasets to verify its predictive value. We further searched the cBioPortal tools to detect genomic alterations and WTAP mutations. Finally, WTAP was further identified as a prognostic factor, and the related mechanisms mediated by WTAP were predicted by gene set enrichment analysis (GSEA). Experimental validations and have been further carried out.
Results: Notably, m6A RNA methylation regulators play significant roles in tumorigenesis and development. In total, we selected three subtypes of cutaneous melanoma according to consensus clustering of the m6A RNA methylation regulators, and the stage of cutaneous melanoma was proven to be related to the subtypes. The Cox regression and LASSO analyses built a risk signature including ELF3, ZC3H13 and WTAP. The prognostic value of the risk signature in internal and external datasets have been proven then. The whole-genome and selected gene WTAP mutations were further explored. WTAP as a single prognostic factor was also explored and found to serve as an independent protective prognostic factor.
Conclusions: Our study constructed a stable risk signature composed of m6A RNA methylation regulators in cutaneous melanoma. Moreover, WTAP was identified as a valuable prognostic factor and potential molecular target for cutaneous melanoma treatment.
Introduction
As one of the deadliest forms of cancer, cutaneous melanoma is responsible for more than 75% of deaths among all cutaneous cancers and no less than 5% of cases of cutaneous cancer (Rebecca et al., 2020). Approximately 100,350 new cases were estimated in the US in 2020, associated with 6,850 deaths, and there is a 2.5–3.6% lifetime probability of developing the disease (Siegel et al., 2020). Cutaneous melanoma refers to the malignant transformation of melanocytes, a type of cell that produces melanin and also regulates the absorption of ultraviolet radiation (UVR) and skin pigmentation (Lin and Fisher, 2007). The malignant transformation of melanocytes has a high mutational burden and is linked with NRAS, HRAS, BRAF, neurofibromin 1 (NF1), KIT, GNAQ, and cyclin-dependent kinase inhibitor 2A (CDKN2A) mutations (Curtin et al., 2005; Bastian, 2014). Known for its high levels of aggressiveness and therapy resistance, cutaneous melanoma was considered to be untreatable before several checkpoint inhibitor therapies were approved, which later proved to show remarkable improvements. Nevertheless, approximately 70% of cutaneous melanoma patients and their attending physicians still face the challenges of immune checkpoint inhibitor (ICI) resistance, high mortality rates, recurrence, and dissemination (Jerby-Arnon et al., 2018). Consequently, attention to the underlying molecular mechanisms underlying this malignancy and the exploration of additional novel targets of cutaneous melanoma treatment is greatly needed.
In recent years, research on epigenetics in RNA has begun to increase in various fields. Posttranscriptional regulatory events such as RNA methylation have attracted increasing attention from researchers and have recently been identified as a mechanism for regulating tumorigenesis. The modification of RNA bases, especially RNA methylation, is being gradually understood for its effects in encouraging RNA translation, metabolism, splicing and stability (Lan et al., 2019). Among them, m6A RNA methylation has been a new research hotspot as the most frequent RNA modification in eukaryotes, Having been recognized as the most abundant and conserved internal transcriptional modification, N6-methyladenosine (m6A) has the ability to alter cancer cell initiation, tumor invasion, proliferation, differentiation inhibition, metastasis and therapy resistance (Wang et al., 2020). m6A refers to the methylation of the nitrogen atom (N) at the 6th position of adenine; enzymes can “write,” “erase” or “read” the methyl group and thus regulate the RNA. Methyltransferase complexes can be divided into three categories: “writers” refers to methyltransferases, mainly including METTL3, METTL14, WTAP, etc., which transfer the methyl to the nitrogen atom (N) at the sixth position of adenine; “erasers” refers to demethylases, mainly including FTO, ALKBH5, etc.; and “readers” refers to specific RNA binding proteins that can bind the DNA m6A modified RNA and have specific biological functions, including YTHDC1, YTHDC2, YTHDF1, YTHDF2, and so on. Therefore, the methylation of RNA in cells is reversible and dynamic. After methylation, RNA is transported out of the nucleus into the cytoplasm and is recognized and bound by downstream methylated reading proteins to regulate the function of the RNA (Nombela et al., 2021).
Recent studies have shown remarkable progress in linking m6A RNA demethylation with fat mass and obesity-associated protein (FTO) (Yang et al., 2019)and ALKBH5 (Li et al., 2020), which is strongly associated with cutaneous melanoma development and reactions to cancer therapy. Notably, other studies also revealed that m6A RNA methylation is significantly related to uveal cutaneous melanoma (UM) and conjunctival cutaneous melanoma (CM) progression and migration by promoting HINT-2 translation Jia et al. (2019) and the regulatory enzyme METTL3 targeting c-Met (Luo et al., 2020), thus demonstrating the close relationship between m6A RNA alterations and cutaneous melanoma. Wilms’ tumor 1-associating protein (WTAP) is widely expressed in the nucleus and functions as a putative splicing regulator. It was first recognized for its interaction with Wilms’ tumor 1 (WT1), which is quintessential in the urogenital system. Notably, WTAP is necessary for the cell cycle progress by stabilizing cyclin A2 mRNA and mammalian early embryo development Horiuchi et al. (2013), and it also has important functions in cell behaviors and cancer development (Yu et al., 2019). It was recently suggested that WTAP may be correlated with numerous types of cancers (Zhang et al., 2016), but there is little knowledge about the role of WTAP in cutaneous melanoma or its underlying mechanism. Our study is the first to investigate the difference in WTAP expression between cutaneous melanoma and normal tissue and its connection to clinicopathological characteristics, as well as to predict its mechanism of action. Our findings may provide strategies for exploring new therapeutic targets of cutaneous melanoma and may have significance for improving the clinical outcomes and prognosis of cutaneous melanoma (Figure 1).
Materials and Methods
Data Processing
The RNA transcription information of 471 cutaneous melanoma samples and 1 normal sample and the corresponding clinicopathological information (Table 1) of 470 cutaneous melanoma patients were downloaded from The Cancer Genome Atlas (TCGA) database (http://cancergenome.nih.gov/). To obtain more samples for the control group, that is, normal tissue, we downloaded the RNA-seq transcriptome data of 812 normal human cutaneous tissues from the GTEx database (https://www.gtexportal.org/home/datasets). The external validation dataset is obtained from the Gene Expression Omnibus (GEO) database (Lin et al., 2021). The RNA transcriptome data were normalized by fragment per kilobase of exon model per million (FPKM, mean fragment per kilobase million (Leek, 2014)). First, we transformed the probe IDs into official gene symbols on the basis of the platform. The probe with the higher expression level was selected when multiple probe IDs matched to one gene symbol, then log2 (x + 1) is used to normalize the original matrix data conversion. The genomic alterations of WTAP were extracted from cBioPortal (www.cbioportal.org). The single nucleotide polymorphism (SNP) data for 471 cutaneous melanoma patients were also extracted from TCGA database by VarScan2 Variant Aggregation and Macutaneousg.
Selection of m6A RNA Methylation Regulators and Correlation Detection
To make the research more comprehensive, we selected 17 classical genes (METTL3, METTL14, WTAP, RBM15, ZC3H13, YTHDC1, YTHDC2, YTHDF1, YTHDF2, HNRNPC, FTO, ALKBH5, KIAA1429, IGF2BP1, IGF2BP2, IGF2BP3, and ELF3) as m6A RNA methylation regulators extracted from previous studies (Luo et al., 2020; Tang et al., 2020). Gene expression were extracted from the TCGA-SCKM cohort and GTEx cutaneous group with corresponding clinical information. To better understand the differences in m6A RNA methylation regulators between cutaneous melanoma and normal samples, heatmaps and violin plots were made by using the “limma” R package (http://www.bioconductor.org/packages/release/bioc/html/limma.html). The screening conditions we used to define the differentially expressed genes were p < 0.05, p < 0.01 and p < 0.001 respectively. Additionally, “corrplot” R package was applied to draw the correlation between the 17 genes (https://cran.r-project.org/web/packages/corrplot/vignettes/corrplot-intro.html).
Consensus Clustering Analysis
To observe the role of 17 m6A RNA methylation regulatory factors in the cutaneous melanoma cohort, consensus clustering analysis was then conducted, with a cumulative distribution function (CDF) for k = 2–9. The “ConsensusClusterPlus” package (1,000 iterations and resample rate of 80%, https://bioconductor.org/packages/ConsensusClusterPlus/) assigned the patients into two categories with 1,000 permutations random sampling algorithm. Principal component analysis was performed to better identified the three groups. The overall survival between clusters was calculated by Kaplan–Meier analysis. The heatmap painted by “pheatmap” R package (https://CRAN.R-project.org/package=pheatmap) showed the relationship between grouping and clinicopathological factors.
Prognostic Signature Generation and Validation
Multivariate Cox regression was used to calculate the correlation of m6A RNA methylation regulator genes with overall survival in cutaneous melanoma. Risk ratios (HRs) were used as the risk factor evaluation criteria. When the HR is greater than 1, the gene is considered a risk factor. Otherwise, it is considered a protective factor. Meanwhile, the risk score was characterized by the coefficients on the basis of the result of the least absolute shrinkage and selection operator (LASSO) algorithm. Finally, based on the minimum criteria, three regulators along with their coefficients were chosen, the risk score was calculated by applying the following formula (Huang et al., 2020):
where Codfi is the coefficient and
Evaluating the Prognostic Value of the Signature in Internal and External Datasets
The overall survival between the high- and low-risk groups was estimated by Kaplan–Meier analysis with the log-rank test. Then the receiver operating characteristic (ROC) curve for patient survival was analyzed to assess the prognostic value. Similarly, the relationships among the clinical factors (age, sex, stage, T stage, N stage, M stage) was also visualized through a heatmap by the “pheatmap” R package. In order to further verify the validity of our signature, we validated using the GSE65904 with 214 melanoma samples dataset downloaded from the Gene Expression Omnibus (GEO) database. The signature calculated by the mentioned formula, and the Kaplan-Meier and ROC curve analysis were implemented in progress. Both univariate and multivariate Cox regression analyses were conducted to predict whether these factors can be served as independent prognostic factors in cutaneous melanoma patients.
Analysis of Genomic Alterations and Related Gene Enrichment
The genes with the top 30 mutation rates in cutaneous melanoma samples were visualized by twoR packages, “GenomeInfoDbData” and “GenVisR” (https://cran.r-project.org/web/packages/viridis/index.html.). According to these 30 mutant genes, we divided those samples into mutation group and normal group to calculate overall survival. The mutations and putative copy number alterations of WTAP in melanoma were got from the cBioPortal tool (http://cbioportal.org) (Gao et al., 2013).
Identifying the WTAP as an Independent Prognostic Factor
WTAP has been selected as an independent prognostic factor in cutaneous melanoma. First, “limma” R package was applied to visualize the WTAP expression differences in normal and tumor samples. According to the expression of WTAP, the data were divided into two groups and overall survival between two clusters were calculated. What is more, the Gene set enrichment analysis (GSEA) 4.1.0 was used to predict downstream access.
Patient Samples and Immunohistochemistry
A total of seven human cutaneous melanoma tissues and their adjacent tissues were collected for immunohistochemical technique (IHC) from The First Hospital of China Medical University. Pathologists assessed the histological features of the specimens by referring to the standard criteria. The research was authorized by the Ethics Commission of the First Hospital of China Medical University (No. 2016-2-3) and was executed based on the ethical principles of the World Medical Association Declaration of Helsinki. Expression of WTAP gene in cutaneous melanoma and their adjacent tissues was assessed by immunohistochemistry (Proteintech, B600010) following the manufacturer’s instructions. In order to quantify WTAP expression, we first evaluated the staining intensity according to the following criteria: negative (score 0), weak (score 1), moderate (score 2), and strong (score 3). Meanwhile, the staining extent was graded into five levels: negative (score 0), 0–25% (score 1), 26–50% (score 2), 51–75% (score 3) and 76–100% (score 4). The merged overall score calculated by the intensity score multiply by percentage score.
Cell Culture and Transfection
Human A375 cells were purchased from the Cell Bank of the Chinese Academy of Sciences (Shanghai, China) and cultured in DMEM medium (Gibico). 10% certified heat-inactivated fetal bovine serum (FBS; Gibico), penicillin (100 U/ mL), and streptomycin (100 mg/ ml) were supplied to culture cells. 37°C and a humidified 5% CO2 atmosphere were also apllied. Plasmids were constructed by Syngentech (Beijing China) based on the sequence of human WTAP in gene bank (NM_004906). The pZDonor-CMV-MCS-BGH_pA-hef1a-EGFP-P2A-neo vector was used for WTAP overexpression. Using Lipofectamine™ 3,000 Transfection Reagent (Thermo Fisher), cells were transfected with pc-WTAP (5 μg), or pc-Con (5 μg) as a negative control, based on the manufacturer’s instructions.
Isolation RNA and Quantitative Real-Time PCR
Total RNA was extracted by using RNAiso Plus (Takara), and cDNA was generated by the PrimeScript RT Reagent Kit (Takara). Quantitative real-time PCR using Powerup SYBR Green PCR Master Mix (Life Technologies) was performed using a real-time PCR system (Applied Biosystems).
Western Blotting
The cells were harvested and rinsed by PBS for three times after transfection for 48 h. Then the lysis buffer was used for the cell extracts and centrifuged at 13,000 xg for 30 min at 4°C. Sodium dodecyl sulfate–polyacrylamide gel electrophoresis (SDS-PAGE) was applied to separated protein samples then transferred to polyvinylidene fluoride membranes. 5% milk blocked samples for 1 h, then we used the primary antibody in 5% BSA overnight at 4°C to incubate. A secondary antibody labeled by horseradish peroxidase (Invitrogen) incubate the membrane after washing. Bio-Rad Imaging System for the visualization and quantification of the band signals.
Cell Proliferation Assay
A375 cells were seeded in 96-well plates with the intensity of 10,000 cells per well. CellTiter 96 AQueous One Solution Cell Proliferation Assay (Promega) was used to assessed according to the manufacturer’s protocol after 24, 48, and 72 h.
Flow Cytometry to Detect the Apoptosis and Cell Cycle
Apoptosis was assessed by the Annexin V:PE Apoptosis Detection Kit I (DOJINDO), following the manufacturer’s instructions. Then samples were detected by a flow cytometer (BD FACSCalibur).
For cell cycle analysis, cells were collected and pre-fixed in 75% cold ethanol and stored overnight at 4°C. After rinsing for 3 times in PBS and staining in propidium iodide (PI) for 30 min, cell cycle was detected by the flow cytometer (BD FACSCalibur).
Migration Assays
Transwell assay evaluated the migration of the A375 melanoma cells. First we collected and resuspended 10,000 cells for the migration assay, cells were seeded into the upper chamber with an 8.0 μm pore (Corning, United States). While DMEM medium containing 20% FBS in the lower chambers. After incubating cells for 24 h, we removed the upper surface and the lower cells fixed with 100% methanol then the hematoxylin staining was performed and the observed under microscope, the above experiment was carried out three times and the number of migrated cells was counted repeatedly.
Statistical Analysis
R software (Version 4.0.3) was utilized to calculate all statistical analyses, and the data presented as the means ± standard deviations. Differences between two groups analyzed by the Wilcoxon test, while the difference among multiple groups applied the one-way analysis of variance (ANOVA). The risk score was obtained by the coefficients which calculated by the LASSO algorithm. Moreover, Kaplan–Meier analysis and a log-rank test were used to calculated the differences in overall survival. Statistical significance was recognized at a P-value threshold of 0.05. In some cases, the false discovery rate was used to correct the p value and, items with corrected p-values of no more than 0.05 were taken.
Results
Differential Expression of m6A RNA Methylation Regulators in Normal Skin and Cutaneous Melanoma
It is noteworthy that the m6A RNA methylation regulators are necessary in the occurrence and development of cancer. To further understand the relationship, we systematically researched the transcription level of the 17 RNA regulators in TCGA datasets. To contribute to this discussion, a heatmap (Figure 2A) and a violin plot (Figure 2B) were used to show the difference. There was a significant difference in all 17 m6A RNA methylation regulators between normal skin and cutaneous melanoma, with all P values less than 0.001. As can be deduced from the picture, all 17 m6A RNA methylation regulators were split into two groups. One group (IGF2BP1, IGF2BP3, RBM15, ZC3H13, YTHDF1, IGF2BP2, YTHDF2, ALKBH5) were highly expressed in the cutaneous melanoma group, while the other group (KIAA1429, HNRNPC, ELF3, METTL14, YTHDC2, METTL3, WTAP, YTHDC1, and FTO) were highly expressed in the normal tissue.
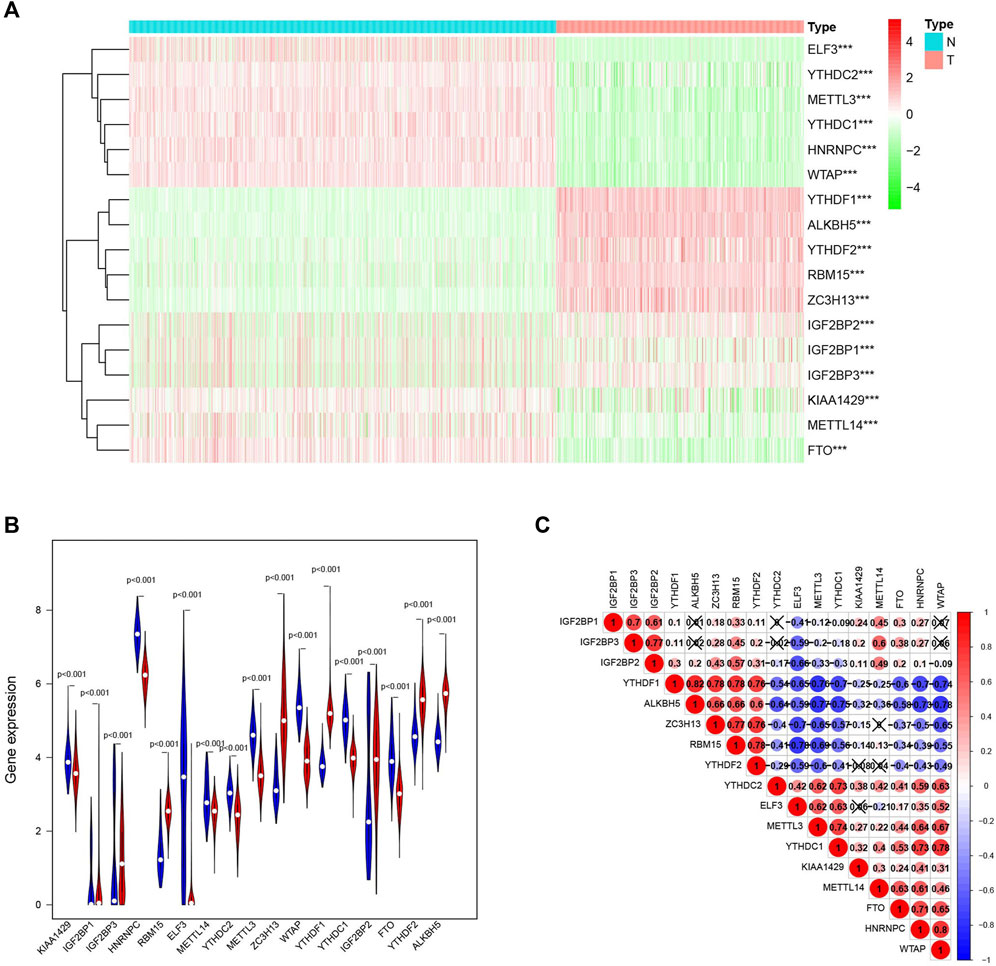
FIGURE 2. The expression characteristics and correlations of m6A RNA methylation regulators in melanoma. (A) Heatmaps presented the overall expression of 17 m6A RNA methylation regulators in melanoma tissues and normal tissues from The Cancer Genome Atlas (TCGA) and The Genotype-Tissue Expression (GTEx) datasets p < 0.05 (“*”), p < 0.01 (“**”), and p <0.001(“***”) (B) The differential expression of the m6A RNA methylation regulators was visualized by vioplot (blue means normal skin tissues, red means melanoma samples). (C) The interaction of the 17 m6A RNA methylation regulators.
To further explore the relationship among the 17 m6A RNA methylation regulators, a correlation between them was performed (Figure 2C). Among them, YTHDF1/ALKBH5 (r = 0.82) and WTAP/HNRNPC (r = 0.80) clearly had the most relevance.
Consensus Clustering of m6A RNA Methylation Regulators Identified Two Clusters of Cutaneous Melanoma and Correlation With Clinicopathological Features
To explore the connection between the m6A RNA methylation regulator expression profile and cutaneous melanoma prognosis, we grouped all 471 cutaneous melanoma cases in an unbiased way by using consensus clustering analysis. The stability of the clustering increased from k = 2 to 9, k means cluster count (Figures 3A,B), and K = 2 (Figure 3C) was selected as the most sensible choice. Moreover, we conducted principal component analysis (PCA) to further compare cluster 1 and cluster 2 (Figure 3D). However, there was no clear separation among the dots representing the two clusters or in the overall survival (Supplementary figure 1I) among the three clusters. We can thus conclude that there is no significant difference. As can be deduced from the heatmap (Figure 3E), only tumor stage was related to the clustering we established.
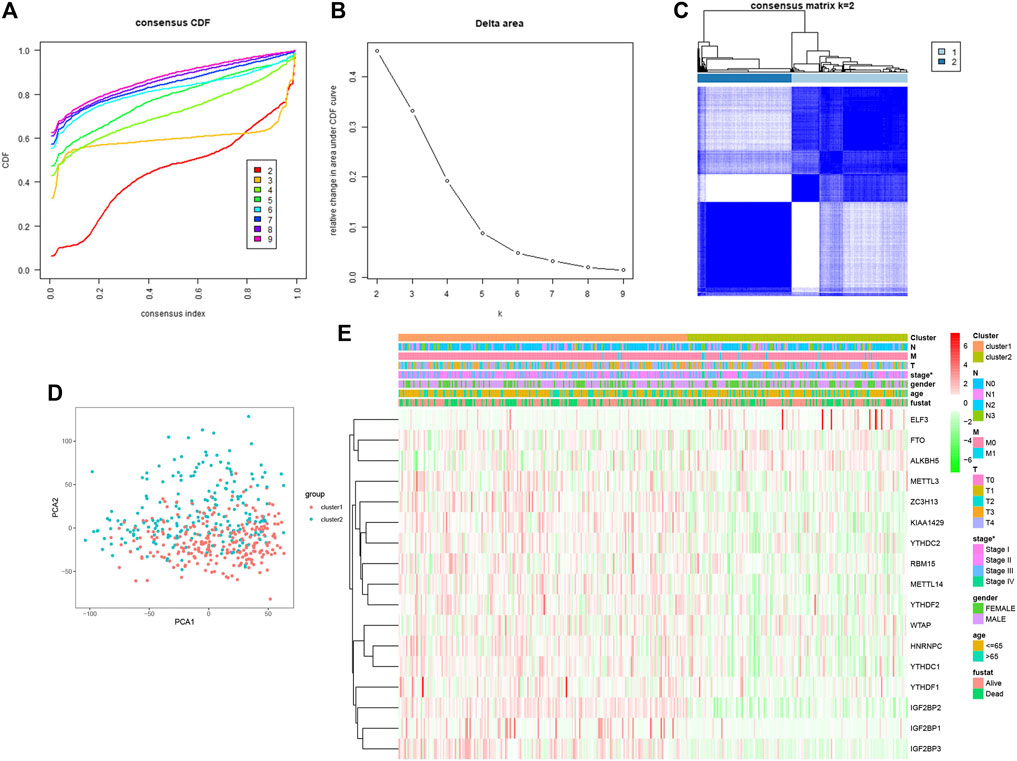
FIGURE 3. Association between the m6A RNA methylation regulators and clinicopathological and prognostic features of melanoma patients. (A) consensus clustering model with cumulation distribution function (CDF) for K = 2–9 (K means cluster count). (B) Relative change in area under the CDF curve for K = 2–9. (C) The Cancer G enome Atlas (TCGA) melanoma cohort was classified into three clusters with K = 2. (D) Principal component analysis of the total RNAexpression profile of cluster 1 (red), cluster 2 (blue). (E) The correlation of the two cluster with clinicopathologic features was visualized by heatmap. p < 0.05 (“*”).
The possible reasons for the absence of significant differences may be that the clustering algorithm is not as sensitive as we expected or the size of the sample needed to be larger than we expected.
Prognostic Value of the m6A RNA Methylation Regulators and the Construction of the Risk Model
As different expression levels of the m6A RNA methylation regulators were observed in cutaneous melanoma and normal tissue, the prognostic value of the factors should be further explored. The LASSO algorithm, a generalized linear model, was constructed for further analysis of prognostic factors based on the expression of m6A RNA methylation regulators the in the TCGA cohort (Figures 4A,B). From this, three important m6A RNA methylation regulators were selected, which included ELF3, ZC3H13, WTAP, multivariate Cox regression (Figure 4C) indicated that “ELF3” and “ZC3H13” served as risk factors that were closely related to poor survival, while “WTAP” was a protective factor among cutaneous melanoma patients. These three we selected had significant correlation to survival (p < 0.1). Meanwhile, receiver operating characteristic (ROC) (Figure 4D) analysis was used to examine whether the model according to the risk score was sensitive and specific for prognosis. The area under the curve (AUC) values were accumulated to reflect the specific size according to the ROC curve. We can summarize from Figure 4D that the one year has the best effect. Therefore, we chose one year to calculate the most suitable cutoff value, and the sample was split into two groups, a high-risk group and a low-risk group with a 0.946 treated as the cutoff point (Figure 4E).
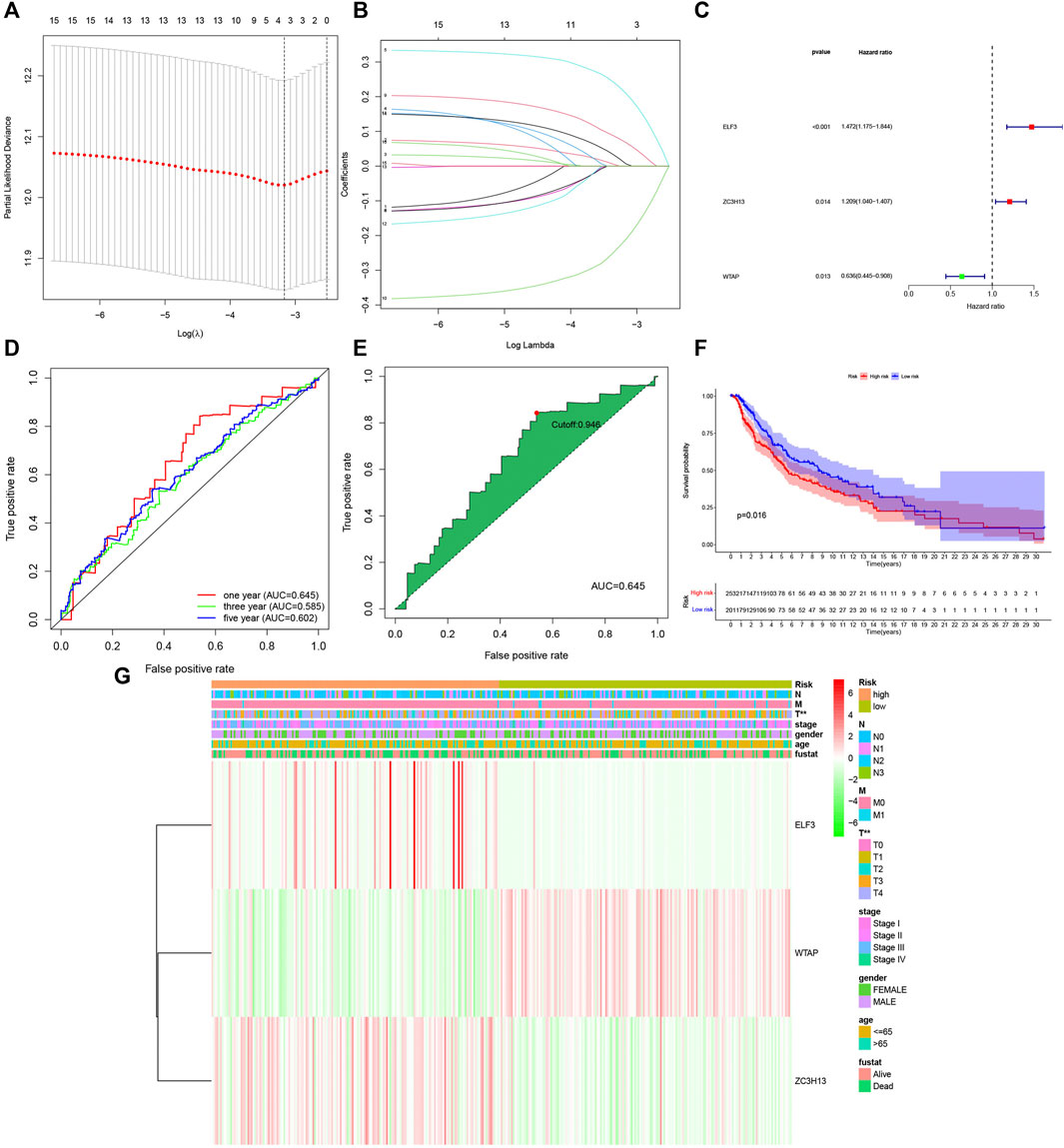
FIGURE 4. Construction of the three m6A RNA methylation regulators-based risk signature. (A–B) Determination of the number of factors by the LASSO analysis. (C) Multivariate analysis of the three-gene signature (ELF3,ZC3H13, AND WTAP). (D) Receiver operating characteristic (ROC) curves for 1-,3-,5-year prognosis. (E) 1 year was chosen to calculate the best cutoff value. (F) The kaplan-Meier curves of melanoma patients at the high-risk group and low-risk group in TCGA cohort. (G) The expression features of the three m6A RNA methylation regulators and the disteibution of clinicopathological features were compared between the low-and high group of The Cancer Genome Atlas (TCGA) melanoma datasets. p < 0.01 (“**”).
Meanwhile, we further estimated the overall survival (Figure 4F) between the two groups, and a significant difference was observed. A remarkable difference in overall survival was found between the high- and low-risk groups, with a 5-years survival rate of 86% in the low-risk group and 66% in the high-risk group, with a difference of 20%.
Hence, we compared the clinicopathological characteristics (including stage, sex, age, survival time, and TNM) of the groups with different risk scores. The heatmap (Figure 4G) clearly shows that there was a correction between the group assignment and clinicopathological factors (T and stage), with a P value less than 0.01.
To ensure the accuracy of the signature we constructed, we selected external data sets for verification. One GEO dataset (GSE65904) comprising 214 melanoma samples was used as a validation cohort. Consistent with our TCGA database results, the lower risk scores represented a higher possibility of survival in patients (Figure 5A). Then we calculate the cutoff value in GEO separately (Figures 5B,C), Similarly, the samples with low-risk scores showed longer overall survival times (p < 0.05), proved our model was feasible and accurate (Figure 5D).
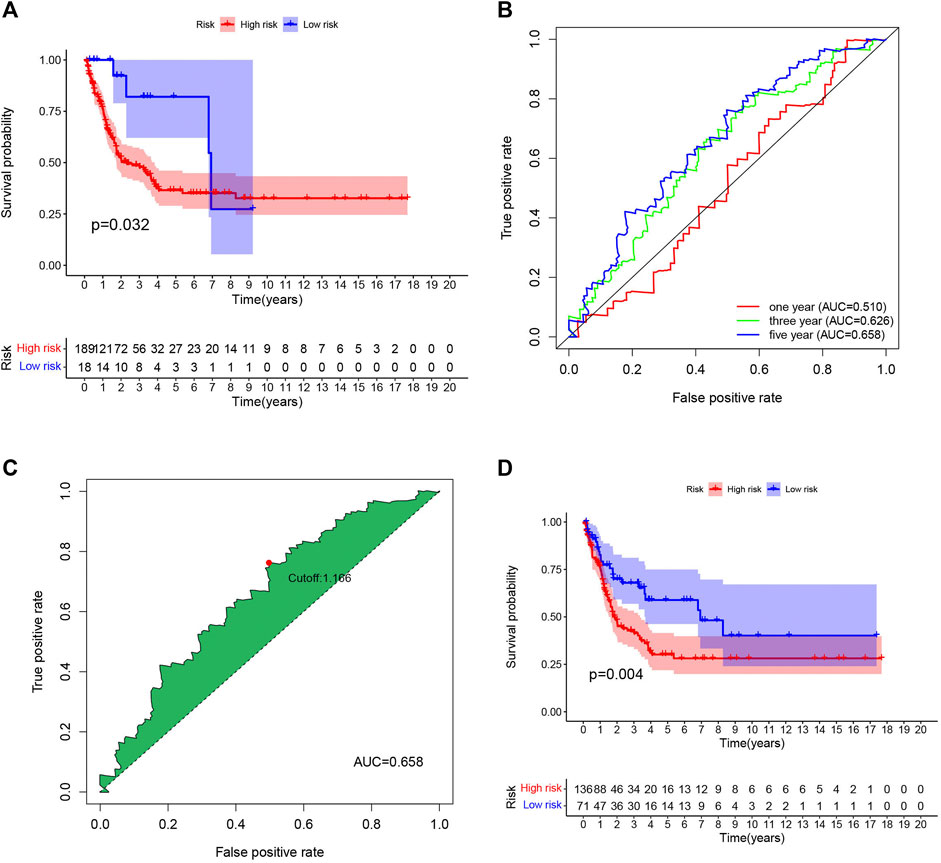
FIGURE 5. External validation of the risk signature. (A) The same cutoff value as TCGA was used to verify in GEO database. (B) Receiver operating characteristic (ROC) curves for 1-,3-,5-year prognosis in GEO database. (C) 5-year was chosen to calculated the brst cutoff value in GEO database. (D) The Kaplan–Meier curves of melanoma patients at the high group and low-risk group in GEO cohort.
Moreover, univariate (Figure 6A) and multivariate (Figure 6B) Cox analyses for overall survival were performed to estimate whether clinicopathological characteristics (including stage, sex, age, survival time, TNM and risk score) were independent prognostic factors. It has been proven that T stage, N stage, risk score, and age were all independent factors for poor prognosis in cutaneous melanoma patients. Multivariate analysis sharing the same variables with the univariate analysis which supported that T (p = 2.61E-05, 95% CI HR 1.215–1.707), N (p = 5.61E-05, 95% CI HR 1.289–2.084), risk score (p = 0.00019, 95% CI HR 1.640–4.890), age (p = 0.02298, 95% CI HR 1.002–1.024). They were also served as poor prognostic factors for cutaneous melanoma patients.
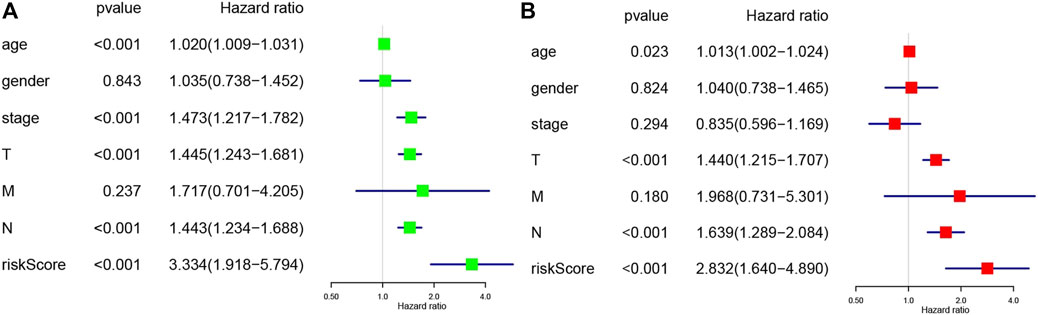
FIGURE 6. (A) Univariate analysis of the hazard ratios for risk score as independent prognostic elements to aniticipate the overall survival. (B) Multivariate analysis of the hazard ratios for risk score as independent prognostic elements to predict the overall survival.
Genomic Alterations and Clinical Implications in Cutaneous Melanoma
Then, we downloaded the SNP data from the 471 samples of cutaneous melanoma and executed mutation visualization analysis through the R package “GenomeInfoDbData” and “GenVisR”. From the waterfall chart (Figure 7A) we obtained; we identified the top 30 mutated genes in the 439 samples. The mutation rates of TTN and MUC16 were over 60%. Kaplan-Meier analysis was used to detect these top 30 mutant genes. Only MUC16(Figure 7B) and ADGRV1 (Figure 7C) showed significant differences (p < 0.05) in overall survival between the mutant and wild type.
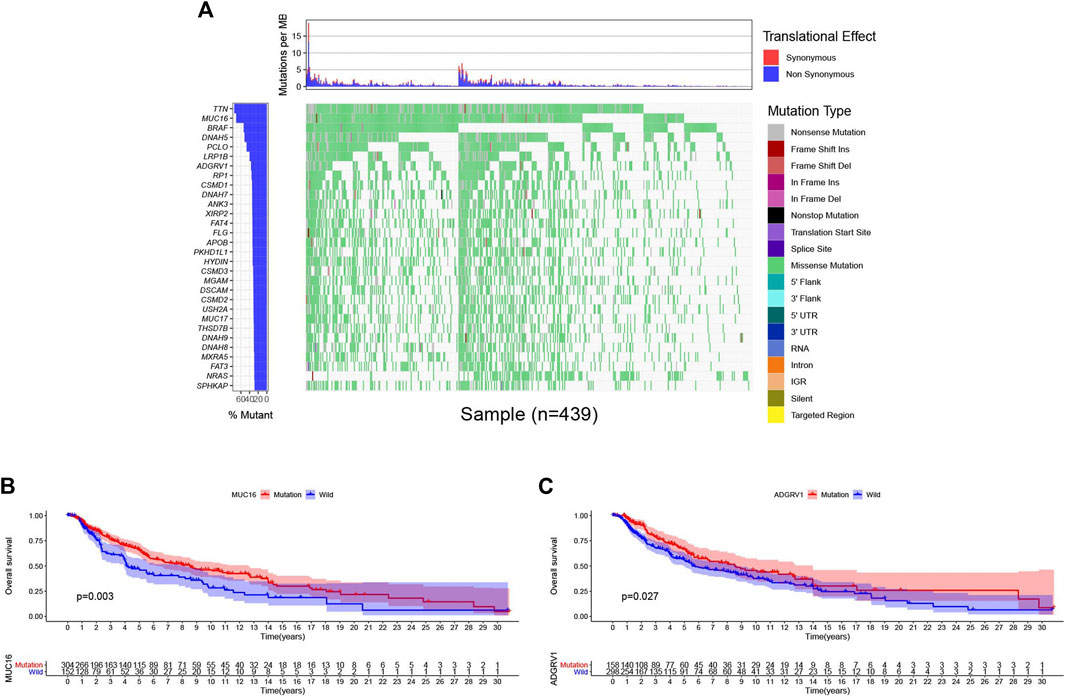
FIGURE 7. Genomic alterations and clincal implications in cutaneous melanoma. (A) The waterfall plots of top 30 mutated genes in TCGA database. (B–C) Kaplan-Meier survival analysis for MUC16 (B), ADGRV1 (C) mutated genes.
Given that the m6A RNA methylation regulator-based signature was closely related to tumor stage and T stage in TNM staging, we wanted to further evaluate those genes that can be treated as independent prognostic factors. Wilms’ tumor 1-associating protein (WTAP) functions as one of the main components of the mA methyltransferase complex in humans. Moreover, a complementary study (Ping et al., 2014) demonstrated that the RNA-binding capability of METTL3 is obviously inhibited when WTAP is absent. WTAP may regulate the activity of its m6A methyltransferase complex toward mRNA targets. Thus, we subsequently focused on the m6A writer WTAP. First, we used cBioPortal to identify the frequency and location of WTAP mutations. According to Oncoprint (Figure 8A), WTAP is altered in 11 (4%) of the examined patients, including cases of missense mutation, amplification, and deep deletion. Among them, deep deletion accounts for the vast majority of alterations. A lollipop diagram of WTAP was generated to show the locations of the gene mutations (Figure 8B). Next, we arranged the transcribed data into two groups, depending on the mutation data in cBioPortal. A total of 469 samples belonged to the wild-type group, while only two samples belonged to the mutation group. We screened the differential expression of the genes between the two groups through logFC > 1 and FDR < 0.05. Moreover, the heatmap (Supplementary figure 2A) and the volcano map (Supplementary figure 2B) may show the difference more obviously. Only red dots appear in the volcano map, which means that the WTAP alteration enhances the expression of those genes. To better understand the functions of the differentially expressed genes between the two groups, we performed Gene Ontology (GO) enrichment analysis. The results showed that the differentially expressed genes mainly function in the “immunoglobin complex,” “phagocytosis,” and “antigen binding” categories in the cellular component (CC) (Supplementary figure 2C), molecular function (MF) (Supplementary figure 2D), and biological process (BP) categories (Supplementary figure 2E), respectively. KEGG enrichment analysis (Supplementary figure 2F) revealed significant enrichment of genes in the “GABAergic synapse,” “morphine addiction,” “serotonergic synapse” and “retrograde endocannabinoid signaling” pathways. The protein-protein interaction (PPI) (Supplementary figure 2G) network analysis indicated that ALDH1A3 and ACSS2 had the most interactions of the studied genes.
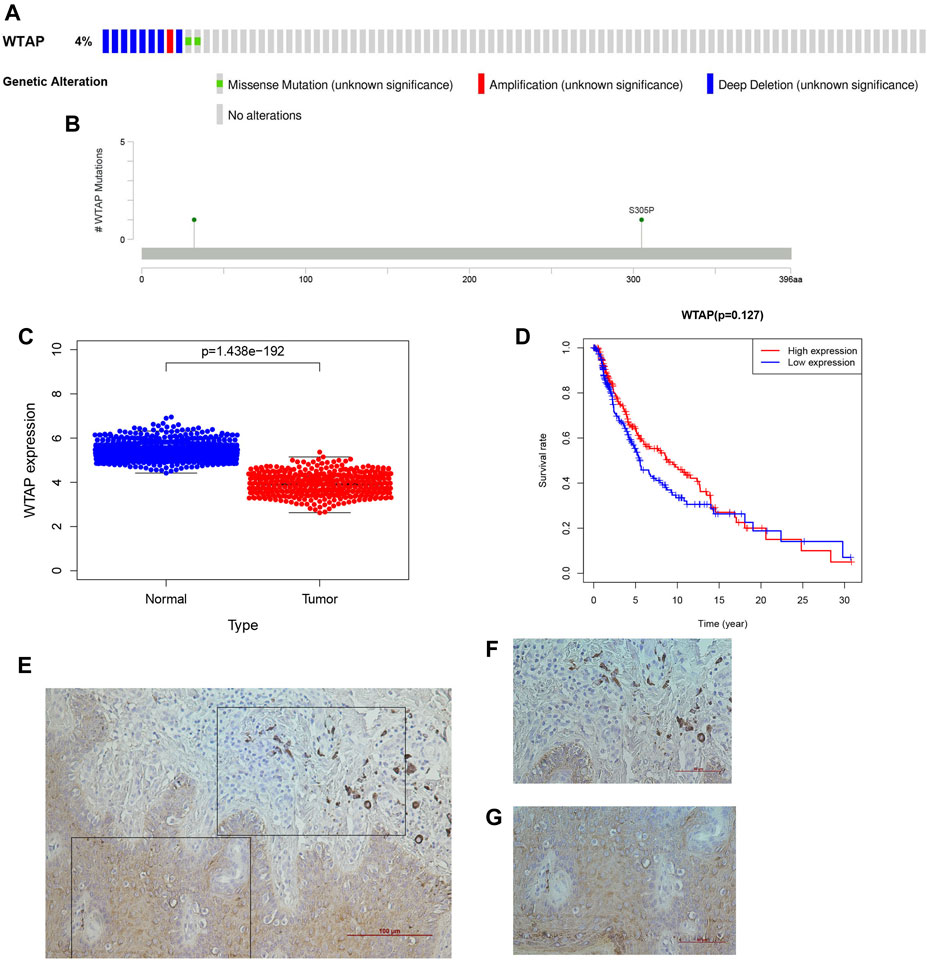
FIGURE 8. WTAP as a novel prognostic biomarker in cutaneous melanoma. (A) OncoPrint of WTAP altertions in melanoma cohort identified by cBioPortal. (B) lollipop of WTAP alteration in melanoma cohort identified. (C) The differential expression of WTAP in cutaneous melanoma. (D) The overall survival of cutaneous melanoma patients in the two clusters (high WTAP expression group and the low WTAP expreaaion group) was calculated by Kaplan-Meier curves. (E-G) The histological features of WTAP gene in cutaneous melanoma (F) and their adjacent tissuses (G).
WTAP in Cutaneous Melanoma and the Prediction of the Downstream Signaling Pathway
WTAP is recognized as an important writer of the m6A RNA methylation transferase complex. As deduced from the above, it is also a protective factor for the development of cutaneous melanoma. To better understand the influence of WTAP on clinically related factors in cutaneous melanoma and whether it can be treated as an independent prognostic factor to judge the prognosis of cutaneous melanoma, we performed a series of bioinformatic analyses. In the figure, we can see that there was an obvious difference in expression between the normal tissue and the tumor group (Figure 8C), and the observed attenuation of WTAP expression in the tumor group also proved its protective role. Then, two groups were formed through the expression level of WTAP in the sample, and Kaplan–Meier analysis with the log-rank test was also applied to judge the difference in overall survival (OS) between the high- and low-expression groups (Figure 8D). Here, there was a difference, but it was not statistically significant. We went a further step to verify the roles of WTAP in melanoma, we first examined the expression of WTAP in melanoma and adjacent tissues. It was noteworthy that the expression of the known m6A “writer” WTAP was significantly declined in cutaneous melanoma than the adjacent tissue (Figures 8E–G), which is consist with the analysis we performed.
GSEA was executed to predict the downstream pathway induced by WTAP, to help us to more comprehensively understand how WTAP works. FDR < 0.05 was used as the screening condition, and we obtained seven possible pathways. Among them, the pathways that were positively correlated with WTAP gene expression included “NOD-like receptor signaling pathway (Figure 9A), JAK-STAT signaling pathway (Figure 9B), T cell receptor signaling pathway (Figure 9C), Natural killer cell mediated cytotoxicity (Figure 9D), B cell receptor signaling pathway (Figure 9E), RNA polymerase (F), Glycosylphosphatidylinositol (GPI)-anchor Biosynthesis (Figure 9G)”. Through multiple GSEA (Figure 9H), we summarized the results into one graph.
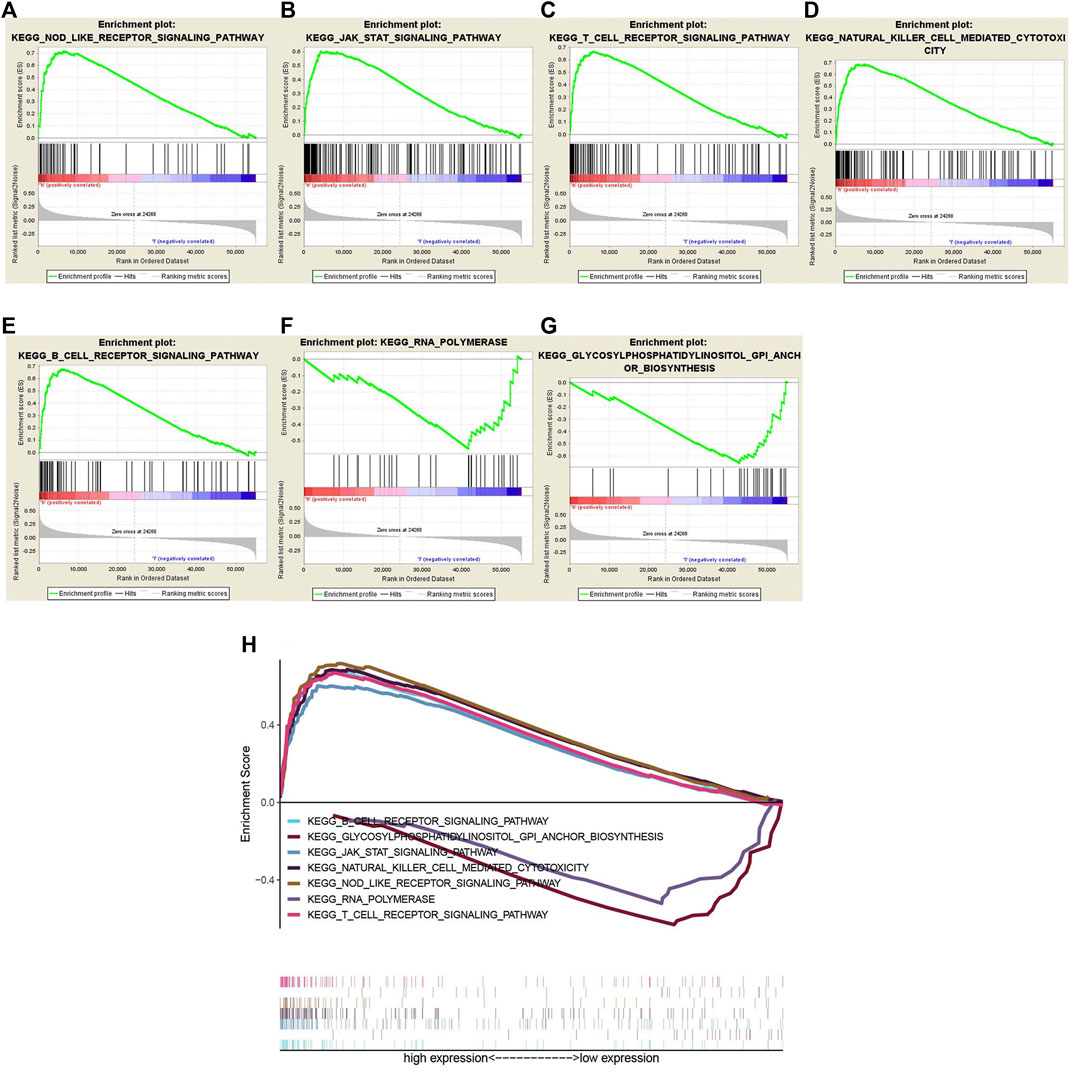
FIGURE 9. (A–G) Gene set enrichment analysis (GSEA) was conducted to predict the potential functions and pathways regulated by WTAP base on Kyoto Encyclopedia of Genes and Genomes (KEGG) datasets: NOD-like receptor signaling pathway (A), JAK-STAT signaling pathway (B), T cell receptor signaling pathway (C), Natural killer cell mediated cytotoxicity (D), B cell receptor signaling pathway (E), RNA polymerase (F), glycosylphosphatidylinositol (GPI)-anchor Biosynthesis (G). (H) Multiple Gene Set enrichment analysis (GSEA).
Validation the Effect of WTAP in Cutaneous Melanoma Tissues and Cells
To further validate the effect of WTAP in cutaneous melanoma, plasmids were transfected into melanoma cell line A375 cells to overexpress WTAP. Quantitative real-time PCR (Figure 10A) and Western Blotting (Figure 10B) proved the success of transfection. The proliferation of melanoma cells was significantly repressed in the overexpressed group (Figure 10C). Consistently, in apoptosis assay, the apoptotic rate of A375 cells was significantly increased while both early apoptosis and late apoptosis are promoted in WTAP overexpressed group (Figure 10D). In cell cycle assay, overexpression of WTAP upregulated the proportion of cells in G0-G1 stage, while downregulated the proportion of cells in S stage (Figure 10E). Furthermore, the migration assays indicated the migration was inhibited in the WTAP overexpressed group (Figure 10F). These results indicated that WTAP is a protective gene in melanoma.
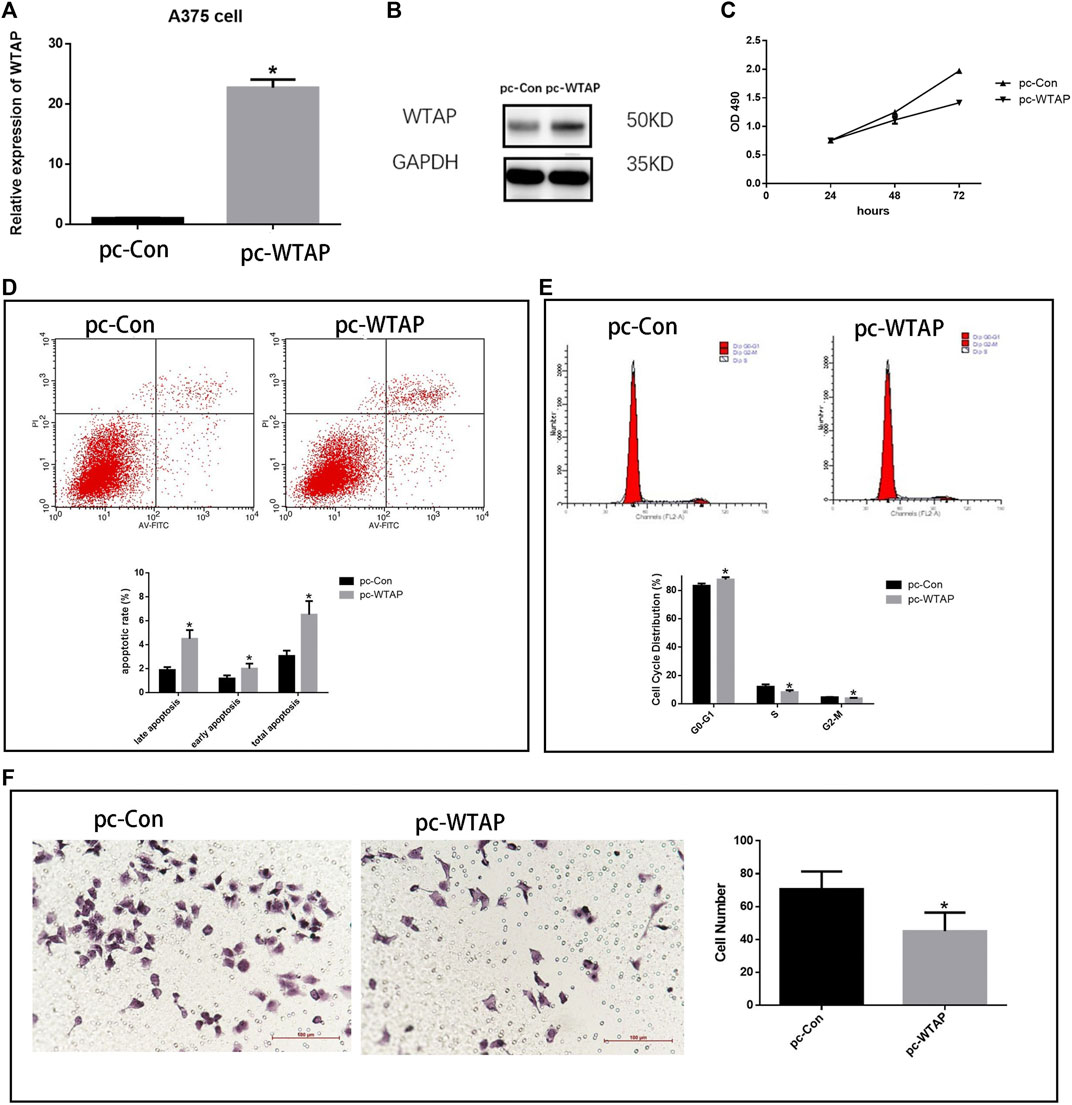
FIGURE 10. Overexpression WTAP inhibited the aggressive behaviors of melanoma cells. (A) Plasmids transfected into A375 cells to overexpress the WTAP expression. The mRNA level of WTAP in A375 cell lines was detected by qRT-PCR. (B) The expression of WTAP in A375 cell lines was detected by western blotting (C) The proliferation of cells was detected by cell counting Kit-8 (CCK-8). (D) The apoptosis of A375 cells with WTAP overexpression (E) cell cycle of A375 cells was evaluated by flow cytometry. (F) Migration of A375 cells were detected by transwell assay.
Discussion
In recent years, abundant genome-wide studies have proven that the majority of the genes are transcribed, thus forming an RNA network consisting of large and small RNAs in cells. However, only a small number of transcripts are translated to proteins (Pandiani et al., 2021). Posttranscriptional RNA modification is an essential process for translation. An estimated approximately 150 posttranscriptional RNA modifications have been proven in all species (Ogawa et al., 2021). Among those modifications, m6A is the most popular among eukaryotic mRNAs and long noncoding RNAs (Kwok et al., 2017). It has also been indicated that anomalous expression of m6A methylation regulators is closely related to the diverse processes in human cancer (Yang et al., 2019; Bian et al., 2020; Ji et al., 2020). Although there have been many studies on the function of m6A RNA methylation regulators in various types of cancer and the related pathways through which they exert their function, research in the field of cutaneous melanoma is quite limited, and only a few studies have studied uveal melanoma (Tang et al., 2020) and ocular melanoma (Jia et al., 2019).
Cutaneous melanoma is still the leading cause of cutaneous cancer-related deaths (Gupta et al., 2020). The treatment of cutaneous melanoma is complex and involves multidisciplinary methods, including surgery, adjuvant radiation and new systemic treatments, such as targeted agents and immunotherapy (Nenclares et al., 2020). Although its incidence rate is low, it is highly malignant (Ablain et al., 2018), metastasis occurs early, and its mortality is high, so it is necessary to study cutaneous melanoma systematically.
In our research, we examined the expression levels of 17 frequently mentioned m6A RNA methylation regulators in the TCGA-SKCM dataset. It was demonstrated that there is a large difference between normal and tumor tissue. We then separated the melanoma cohort into two clusters through consensus clustering analysis. The survival curve after clustering did not show significant statistical significance, which may be due to the insufficient number of samples or clustering needs to be adjusted.
According to the results of the multivariate Cox regression analysis, three of the seventeen m6A RNA methylation regulators were proved to be the potential prognostic factors of cutaneous melanoma, including ELF3, ZC3H13, and WTAP.
The LASSO algorithm was applied with the three mentioned genes to build a risk signature. The signature risk score was associated with different sizes of primary tumors, which could also be treated as an independent prognostic factor. Among the 17 m6A regulators, previous studies have reported that high WTAP expression in hepatocellular carcinoma was associated with poor prognosis; hence, WTAP promoted the growth and proliferation of HCC cells both in vitro and in vivo (Chen et al., 2019). Moreover, in bladder cancer patients, the expression level of WTAP is closely related to the risk of recurrence (Chen and Wang, 2018). In high-grade serous ovarian carcinoma, high expression of WTAP correlated with shorter overall survival, and low expression of WTAP reduced cancer cell proliferation and migration (Yu et al., 2019). Meanwhile, the reader ELF3 was reported to be closely related to poor survival in colorectal cancer (CRC) patients by driving β-catenin transactivation (Wang et al., 2014). Thus, m6A regulators are closely correlated with the prognosis of cancer. In our paper, we report further research on the methylation writer WTAP.
To analyze the mutation profile of cutaneous melanoma in the TCGA dataset, we identified the top 30 variant mutated genes. Difference analysis and functional enrichment analysis, as well as the construction of a PPI network, helped us to have a better understanding of its functions and GO annotations of genes involved in cutaneous melanoma. Then, we focused on WTAP in TCGA, and we performed a series of studies to detect the mutation probability and the survival rate of the mutation group and the normal group. The difference between the groups was not significant, possibly because the mutation rate was so low that we did not have enough samples in our analysis to detect a significant difference.
WTAP as a single factor was further analyzed in the tumor group and normal group, the relationship between high and low WTAP expression and clinicopathological factors, and whether it can be treated as an independent prognostic factor. Next, we tried to determine its potential mechanisms via bioinformatic prediction and molecular validation. The downstream pathways were further predicted by GSEA, with the corrected p value as the screening condition, and seven pathways were identified and selected for display. Further exploration with further experiments and analysis is needed.
Data Availability Statement
The datasets presented in this study can be found in online repositories. The names of the repository/repositories and accession number(s) can be found in the article/Supplementary Material.
Ethics Statement
The studies involving human participants were reviewed and approved by the Ethics Commission of the First Hospital of China Medical University. The patients/participants provided their written informed consent to participate in this study.
Author Contributions
Conceptualization: SG and ZF; original manuscript preparation: TW; draft correction, supervision and editing: SG and XS. All authors listed have made substantial contribution to the manuscript, which is acknowledged and confirmed by themselves. All authors have read and agreed on the final version of the manuscript.
Conflict of Interest
The authors declare that the research was conducted in the absence of any commercial or financial relationships that could be construed as a potential conflict of interest.
Acknowledgments
We would like to thank all the colleagues who have made contributions to this manuscript.
Supplementary Material
The Supplementary Material for this article can be found online at: https://www.frontiersin.org/articles/10.3389/fmolb.2021.665222/full#supplementary-material
References
Ablain, J., Xu, M., Rothschild, H., Jordan, R. C., Mito, J. K., Daniels, B. H., et al. (2018). Human Tumor Genomics and Zebrafish Modeling Identify SPRED1 Loss as a Driver of Mucosal Melanoma. Science 362 (6418), 1055–1060. doi:10.1126/science.aau6509
Bastian, B. C. (2014). The Molecular Pathology of Melanoma: an Integrated Taxonomy of Melanocytic Neoplasia. Annu. Rev. Pathol. Mech. Dis. 9, 239–271. doi:10.1146/annurev-pathol-012513-104658
Bian, S., Ni, W., Zhu, M., Song, Q., Zhang, J., Ni, R., et al. (2020). Identification and Validation of the N6-Methyladenosine RNA Methylation Regulator YTHDF1 as a Novel Prognostic Marker and Potential Target for Hepatocellular Carcinoma. Front. Mol. Biosciences 7, 604766. doi:10.3389/fmolb.2020.604766
Chen, L., and Wang, X. (2018). Relationship between the Genetic Expression of WTAP and Bladder Cancer and Patient Prognosis. Oncol. Lett. 16 (6), 6966–6970. doi:10.3892/ol.2018.9554
Chen, Y., Peng, C., Chen, J., Chen, D., Yang, B., He, B., et al. (2019). WTAP Facilitates Progression of Hepatocellular Carcinoma via m6A-HuR-dependent Epigenetic Silencing of ETS1. Mol. Cancer 18 (1), 127. doi:10.1186/s12943-019-1053-8
Curtin, J. A., Fridlyand, J., Kageshita, T., Patel, H. N., Busam, K. J., Kutzner, H., et al. (2005). Distinct Sets of Genetic Alterations in Melanoma. N. Engl. J. Med. 353 (20), 2135–2147. doi:10.1056/nejmoa050092
Gao, J., Aksoy, B. A., Dogrusoz, U., Dresdner, G., Gross, B., Sumer, S. O., et al. (2013). Integrative Analysis of Complex Cancer Genomics and Clinical Profiles Using the cBioPortal. Sci. Signal. 6 (269), pl1. doi:10.1126/scisignal.2004088
Gupta, R., Janostiak, R., and Wajapeyee, N. (2020). Transcriptional Regulators and Alterations that Drive Melanoma Initiation and Progression. Oncogene 39 (48), 7093–7105. doi:10.1038/s41388-020-01490-x
Horiuchi, K., Kawamura, T., Iwanari, H., Ohashi, R., Naito, M., Kodama, T., et al. (2013). Identification of Wilms' Tumor 1-associating Protein Complex and its Role in Alternative Splicing and the Cell Cycle. J. Biol. Chem. 288 (46), 33292–33302. doi:10.1074/jbc.m113.500397
Huang, R., Li, G., Wang, Z., Hu, H., Zeng, F., Zhang, K., et al. (2020). Identification of an ATP Metabolism‐related Signature Associated with Prognosis and Immune Microenvironment in Gliomas. Cancer Sci. 111 (7), 2325–2335. doi:10.1111/cas.14484
Jerby-Arnon, L., Shah, P., Cuoco, M. S., Rodman, C., Su, M.-J., Melms, J. C., et al. (2018). A Cancer Cell Program Promotes T Cell Exclusion and Resistance to Checkpoint Blockade. Cell 175 (4), 984–997. doi:10.1016/j.cell.2018.09.006
Ji, L., Chen, S., Gu, L., and Zhang, X. (2020). Exploration of Potential Roles of m6A Regulators in Colorectal Cancer Prognosis. Front. Oncol. 10, 768. doi:10.3389/fonc.2020.00768
Jia, R., Chai, P., Wang, S., Sun, B., Xu, Y., Yang, Y., et al. (2019). mA Modification Suppresses Ocular Melanoma through Modulating HINT2 mRNA Translation. Mol. Cancer 18 (1), 161. doi:10.1186/s12943-019-1088-x
Kwok, C., Marshall, A., Rasko, J., and Wong, J. (2017). Genetic Alterations of mA Regulators Predict Poorer Survival in Acute Myeloid Leukemia. J. Hematol. Oncol. 10 (1), 39. doi:10.1186/s13045-017-0410-6
Lan, Q., Liu, P. Y., Haase, J., Bell, J. L., Hüttelmaier, S., and Liu, T. (2019). The Critical Role of RNA m6A Methylation in Cancer. Cancer Res. 79 (7), 1285–1292. doi:10.1158/0008-5472.can-18-2965
Leek, J. T. (2014). Svaseq: Removing Batch Effects and Other Unwanted Noise from Sequencing Data. Nucleic Acids Res. 42 (21), e161. doi:10.1093/nar/gku864
Li, N., Kang, Y., Wang, L., Huff, S., Tang, R., Hui, H., et al. (2020). ALKBH5 Regulates Anti-PD-1 Therapy Response by Modulating Lactate and Suppressive Immune Cell Accumulation in Tumor Microenvironment. Proc. Natl. Acad. Sci. USA 117 (33), 20159–20170. doi:10.1073/pnas.1918986117
Lin, J. Y., and Fisher, D. E. (2007). Melanocyte Biology and Skin Pigmentation. Nature 445 (7130), 843–850. doi:10.1038/nature05660
Lin, Y., Wang, S., Liu, S., Lv, S., Wang, H., and Li, F. (2021). Identification and Verification of Molecular Subtypes with Enhanced Immune Infiltration Based on m6A Regulators in Cutaneous Melanoma. Biomed. Res. Int. 2021, 2769689. doi:10.1155/2021/2769689
Luo, G., Xu, W., Zhao, Y., Jin, S., Wang, S., Liu, Q., et al. (2020). RNA M 6 A Methylation Regulates Uveal Melanoma Cell Proliferation, Migration, and Invasion by Targeting c‐Met. J. Cel Physiol 235 (10), 7107–7119. doi:10.1002/jcp.29608
Nenclares, P., Ap Dafydd, D., Bagwan, I., Begg, D., Kerawala, C., King, E., et al. (2020). Head and Neck Mucosal Melanoma: The United Kingdom National Guidelines. Eur. J. Cancer 138, 11–18. doi:10.1016/j.ejca.2020.07.017
Nombela, P., Miguel-López, B., and Blanco, S. (2021). The Role of mA, mC and Ψ RNA Modifications in Cancer: Novel Therapeutic Opportunities. Mol. Cancer 20 (1), 18. doi:10.1186/s12943-020-01263-w
Ogawa, A., Nagiri, C., Shihoya, W., Inoue, A., Kawakami, K., Hiratsuka, S., et al. (2021). N-methyladenosine (mA) Is an Endogenous A3 Adenosine Receptor Ligand. Mol. Cel. 81, 659. doi:10.1016/j.molcel.2020.12.038
Pandiani, C., Strub, T., Nottet, N., Cheli, Y., Gambi, G., Bille, K., et al. (2021). Single-cell RNA Sequencing Reveals Intratumoral Heterogeneity in Primary Uveal Melanomas and Identifies HES6 as a Driver of the Metastatic Disease. Cel Death Differ. 28, 1990. doi:10.1038/s41418-020-00730-7
Ping, X.-L., Sun, B.-F., Wang, L., Xiao, W., Yang, X., Wang, W.-J., et al. (2014). Mammalian WTAP Is a Regulatory Subunit of the RNA N6-Methyladenosine Methyltransferase. Cell Res 24 (2), 177–189. doi:10.1038/cr.2014.3
Rebecca, V. W., Somasundaram, R., and Herlyn, M. (2020). Pre-clinical Modeling of Cutaneous Melanoma. Nat. Commun. 11 (1), 2858. doi:10.1038/s41467-020-15546-9
Siegel, R. L., Miller, K. D., and Jemal, A. (2020). Cancer Statistics, 2020. CA A. Cancer J. Clin. 70 (1), 7–30. doi:10.3322/caac.21590
Tang, J., Wan, Q., and Lu, J. (2020). The Prognostic Values of m6A RNA Methylation Regulators in Uveal Melanoma. BMC Cancer 20 (1), 674. doi:10.1186/s12885-020-07159-8
Wang, J.-L., Chen, Z.-F., Chen, H.-M., Wang, M.-Y., Kong, X., Wang, Y.-C., et al. (2014). Elf3 Drives β-catenin Transactivation and Associates with Poor Prognosis in Colorectal Cancer. Cell Death Dis 5, e1263. doi:10.1038/cddis.2014.206
Wang, T., Kong, S., Tao, M., and Ju, S. (2020). The Potential Role of RNA N6-Methyladenosine in Cancer Progression. Mol. Cancer 19 (1), 88. doi:10.1186/s12943-020-01204-7
Yang, S., Wei, J., Cui, Y. H., Park, G., Shah, P., Deng, Y., et al. (2019). m(6A mRNA Demethylase FTO Regulates Melanoma Tumorigenicity and Response to Anti-PD-1 Blockade. Nat. Commun. 10 (1), 2782. doi:10.1038/s41467-019-10669-0
Yu, H.-L., Ma, X.-D., Tong, J.-F., Li, J.-Q., Guan, X.-J., and Yang, J. (2019). WTAP Is a Prognostic Marker of High-Grade Serous Ovarian Cancer and Regulates the Progression of Ovarian Cancer Cells. Onco. Targets Ther. 12, 6191–6201. doi:10.2147/ott.s205730
Keywords: cutaneous melanoma, m6A regulators, WTAP, biomarker, survival analysis, genomic alterations
Citation: Feng Z-Y, Wang T, Su X and Guo S (2021) Identification of the m6A RNA Methylation Regulators WTAP as a Novel Prognostic Biomarker and Genomic Alterations in Cutaneous Melanoma. Front. Mol. Biosci. 8:665222. doi: 10.3389/fmolb.2021.665222
Received: 07 February 2021; Accepted: 22 June 2021;
Published: 05 July 2021.
Edited by:
Cristina Pellegrini, University of L’Aquila, ItalyReviewed by:
Wenjie Zheng, Affiliated Hospital of Nantong University, ChinaChu Ming Tao, Nanchang University, China
Copyright © 2021 Feng, Wang, Su and Guo. This is an open-access article distributed under the terms of the Creative Commons Attribution License (CC BY). The use, distribution or reproduction in other forums is permitted, provided the original author(s) and the copyright owner(s) are credited and that the original publication in this journal is cited, in accordance with accepted academic practice. No use, distribution or reproduction is permitted which does not comply with these terms.
*Correspondence: Shu Guo, c2d1b0BjbXUuZWR1LmNu