- 1Sanders Brown Center on Aging, University of Kentucky, Lexington, KY, United States
- 2Spinal Cord and Brain Injury Research Center, University of Kentucky, Lexington, KY, United States
- 3Pathology & Laboratory Medicine, University of Kentucky, Lexington, KY, United States
- 4Neuroscience, University of Kentucky, Lexington, KY, United States
- 5Biostatistics, University of Kentucky, Lexington, KY, United States
- 6Anesthesiology Critical Care Medicine, University of Kentucky, Lexington, KY, United States
Approximately one-third of aneurysmal subarachnoid hemorrhage (aSAH) patients develop delayed cerebral vasospasm (DCV) 3–10 days after aneurysm rupture resulting in additional, permanent neurologic disability. Currently, no validated biomarker is available to determine the risk of DCV in aSAH patients. MicroRNAs (miRNAs) have been implicated in virtually all human diseases, including aSAH, and are found in extracellular biofluids including plasma and cerebrospinal fluid (CSF). We used a custom designed TaqMan Low Density Array miRNA panel to examine the levels of 47 selected brain and vasculature injury related miRNAs in CSF and plasma specimens collected from 31 patients with or without DCV at 3 and 7 days after aSAH, as well as from eight healthy controls. The analysis of the first 18-patient cohort revealed a striking differential expression pattern of the selected miRNAs in CSF and plasma of aSAH patients with DCV from those without DCV. Importantly, this differential expression was observed at the early time point (3 days after aSAH), before DCV event occurs. Seven miRNAs were identified as reliable DCV risk predictors along with a prediction model constructed based on an array of additional 19 miRNAs on the panel. These chosen miRNAs were then used to predict the risk of DCV in a separate, testing cohort of 15 patients. The accuracy of DCV risk prediction in the testing cohort reached 87%. The study demonstrates that our novel designed miRNA panel is an effective predictor of DCV risk and has strong applications in clinical management of aSAH patients.
Introduction
Aneurysmal subarachnoid hemorrhage (aSAH) is a catastrophic event that occurs when an intracranial arterial aneurysm ruptures, resulting in the release of oxygenated blood into the subarachnoid space (Dority and Oldham, 2016). Delayed cerebral vasospasm (DCV) has long been considered the most devastating acute complication following aSAH (Janjua and Mayer, 2003; Al-Mufti et al., 2017; Brami et al., 2020). Approximately 30% of aSAH patients develop DCV (Janjua and Mayer, 2003), which commonly occurs during 3–10 days after aneurysm rupture, requiring frequent neurologic monitoring and rapid, intensive interventions to minimize further injury and disability. The pathological events contributing to DCV are poorly understood but appear to include calcium dependent and independent vasoconstriction, oxidative stress, endothelial dysfunction, inflammatory responses, apoptosis, autophagy, and altered gene expression (Macdonald and Weir, 1991; Dietrich and Dacey, 2000; Kolias et al., 2009; Carr et al., 2013; Eisenhut, 2014; Miller et al., 2014; Chou, 2018). Predicting DCV risk before it becomes clinically symptomatic is crucial to limiting further injury. Unfortunately, no effective biomarker exists to predict DCV at a time point when neurologic injury can be prevented (Mocco et al., 2006; Etminan et al., 2011). At this time, clinical management includes a “wait and see” approach. Therefore, there is an urgent need to identify biomarkers that determine DCV risk/susceptibility in order to implement preventive or management strategies.
MicroRNAs (miRNAs) are small, non-coding, regulatory RNAs (Bartel, 2004; Huntzinger and Izaurralde, 2011). MiRNAs regulate all aspects of cellular function, and disruption of miRNA activity contributes to many disease states including neurological diseases such as Alzheimer’s disease, stroke, and traumatic brain injury (Wang et al., 2008, 2010, 2015; Liu and Xu, 2011; Tan et al., 2011; Koutsis et al., 2013; Hill and Lukiw, 2016). Several studies have demonstrated changes in miRNA levels in cerebral arteries following aSAH and DCV (Vikman et al., 2006; Muller et al., 2015; Li et al., 2018). For example, over 150 miRNAs were differentially expressed in the aneurysmal tissue when compared to normal arteries (Liu et al., 2014). MiRNAs are also recognized as powerful regulators of CNS inflammatory responses (O’connell et al., 2012), including that occurs following stroke and aSAH (Khoshnam et al., 2017; Lopes et al., 2018). Their functional role and the fact that miRNA can be secreted into extracellular fluids make them attractive candidates as biomarkers. Moreover, miRNAs in biofluids are highly stable at room temperature, resistant to RNase activity (Mraz et al., 2009) and relatively easy to detect and quantify (Etheridge et al., 2011). In this study, we explore the potential of using cerebral spinal fluid (CSF) and plasma miRNAs as biomarkers to predict DCV events in aSAH patients.
Materials and Methods
Patient Cohorts
All procedures and protocols related to the human participants and collections of specimens were approved by the University of Kentucky Institutional Review Board (IRB) that are in compliance with the 1964 Helsinki declaration and its later amendments. Following the approved IRB protocol (# 55914), all patients admitted to the University of Kentucky Chandler Medical Center (UKCMC) were screened for inclusion in this study. Inclusion criteria included being 18 years of age or older, radiographically evidence of aSAH, a Modified Fischer Score > 3 (Frontera et al., 2006), and a ventriculostomy (EVD: external ventricular drain) being placed prior to post-bleed-day 3 (PBD3). Patients were excluded if they arrived at the hospital in a non-survivable condition or were likely to die prior to PBD14 as determined by the attending physicians. Patients also were excluded if they had a history of systemic inflammatory disease or chronically dosed with a biologic inflammatory modulator. Finally, patients were excluded if a legally authorized representative (LAR) could not be located by PBD3 or who refused (or their LAR refused) consent.
During hospitalization, all patients were treated according to local standard of care. Patients were considered to have DCV if, during their hospitalization, the daily transcranial Doppler (TCD) monitoring demonstrated a mean flow velocity > 120 cm/sec in either middle cerebral artery (MCA) and a calculated Lindegaard ratio >3.0 (Kirsch et al., 2013).
Cell-Free CSF and Plasma Sample Collection
After written consent was obtained, CSF and plasma were collected at PBD 3, 5, 7, and 10 using a standard protocol. CSF was collected directly from the EVD drainage tubing. Collected samples were centrifuged at 3,000 × g for 5 min and the supernatant aliquoted into 250-μl aliquots and frozen at -80 C until further analysis. The miRNA analysis was performed only from the specimens collected at PBD3 and PBD7.
In addition, frozen plasma and CSF samples from several de-identified cases were gifted from the University of Pennsylvania (UPENN). This data set and biofluids had already been collected and no information on data collection or sample preparation were available. Therefore, they only were used in testing prediction model.
Finally, CSF and plasma specimens from eight healthy persons who had no clinically manifest neurological disease/diagnosis were procured from the University of Kentucky Alzheimer’s Disease Center (UK-ADC) biobank.
Experimental Design
A total of 39 patients and controls were analyzed in three separated groups according to the availability of the specimens at the time of analysis (Figure 1). Group A was analyzed in June 2019 and included eighteen aSAH patients (10 DCV-, 8 DCV+) from UKCMC and 8 healthy controls (HCs). Group B was analyzed in September 2019 and included nine aSAH patients including two experimental duplicates from Group A, three additional cases from UKCMC, and four cases from UPENN. Group C was analyzed in January 2020 and included six aSAH patients from UKCMC. The data from Group A was used as a training set to build a risk prediction model for testing the discrimination ability of the miRNA panel in Group B and Group C. Differences in demographics and clinical observations were evaluated using Fisher’s exact test.
A blinding procedure was implemented in this project. The physicians who diagnosed and treated the patient, and those that collected and aliquoted the specimens did not perform the miRNA analysis. Likewise, miRNA analyses were performed by personnel blinded to patient data and clinical outcome. Clinical and laboratory data were only unblinded after all data were acquired. The statisticians who constructed the prediction models were not involved in either the sample collection or miRNA analysis.
Design of a Customized Brain and Vasculature Injury Related miRNA Panel
A customized panel containing miRNAs associated with brain and vasculature injury was designed as described previously (Wang et al., 2017) and used to generate a TaqMan® miRNA RT-qPCR low density array (TLDA, Thermo Fisher Scientific, Waltham, MA, United States) that can simultaneously analyze 48 miRNAs in 8 independent samples.
RNA Isolation From CSF and Plasma
CSF and plasma total RNA were isolated using miRNeasy Serum/Plasma Advanced Kit (Cat #: 217204, Qiagen, Hilden, Germany) following manufacturer’s protocol. RNA was eluted with 30 μl of nuclease-free water containing 0.5 U/μl RNAsin (RNase inhibitor, Cat #: N2115, Promega, Madison, WI, United States).
RT-qPCR Using Customized TaqMan® Low-Density Array (TLDA)
MiRNA reverse transcription and real-time PCR detection procedures were performed following the manufacturer’s instruction and are also described in detail in prior publications (Wang et al., 2014, 2017).
TLDA Data Processing
Quantitative real-time PCR run files were imported to and analyzed using Thermo Fisher Cloud Software—Relative Quantification. The relative threshold cycle (Crt) method (Applied Biosystems, 2016) was applied to acquire the quantitative Crt values from all runs in each experiment. Crt values that failed software-defined QC and/or values equal to or more than 35 were considered as undetectable and excluded from further analysis. Furthermore, a miRNA would be removed from analysis if it was not detected in one third of tested samples. The TLDA consisted of specific selected miRNAs and an internal normalizer could not be identified. Therefore, the Global Mean Normalization method (Mestdagh et al., 2009) was used to normalize the TLDA data. Raw Crt values and the geometric mean of a given sample was used to obtain ΔCrt values calculated as ΔCrt = Crt–target - Crt–geomean for each miRNA. The time for processing 2–8 samples from RNA isolation to data analysis is around 6–8 h (Supplementary Figure 1).
Statistical Analysis
GraphPad Prism 8.0.2 (San Diego, CA, United States) and R (version 4.0.2) (R Core Team, 2020) were used for statistical analyses. Student’s t-tests (2-tailed) were used to evaluate mean differences for individual miRNAs. A 95% confidence interval with p < 0.05 being considered statistically significant. The receiver operating characteristics (ROC) were generated to evaluate DCV discrimination ability of individual miRNAs.
Construction of DCV Prediction Model
A DCV prediction model was constructed using the Group A CSF/PBD3 dataset. The model used the 26 miRNAs (“variables,” Supplementary File 1) that did not have any missing data across all 3 patient groups. Among the 26 miRNAs, the expression levels of 7 miRNAs exhibited perfect discrimination between DCV+ and DCV- patient groups in the Group A dataset (Supplementary Figure 2 and Supplementary File 1). These seven miRNAs each served as individual predictors in a consensus predictive tool. The remaining 19 miRNAs were used to generate a decision tree using the Recursive partitioning and regression trees (Therneau and Atkinson, 2019) (Rpart) algorithm in R.
Results
Demographic and Pathological Data of Study Cohorts
From July 2018 to December 2019, 77 patients were admitted to UKCMC with aSAH, of these, 27 were included in this study. There were no significant differences between the patients with DCV (DCV+) and patients without DCV (DCV-) based on age, gender, height, weight, body mass index, ethnic origin (Table 1). Furthermore, no significant differences were observed between the two study groups in the classification of the severity of subarachnoid hemorrhage (Hunt Hess score, WFNS score, and modified Fischer score), location of the aneurysm, or the type of aneurysm obliteration. The average age of the HC group was older than that of aSAH patients, however, we were primarily interested in the comparison between DCV+ and DCV- patients and the HC group served as a secondary reference. Therefore, the age difference was not considered a confounding factor.
Design and Performance of the Customized Brain and Vasculature Injury Related miRNA Panel
We developed a customized miRNA panel consisting of 47 miRNAs relevant to brain and vasculature injury events (Table 2). MiRNAs that met any of following criteria were selected: (1) strong association with CNS injury or cerebrovascular damage, either identified in our prior studies (Wang et al., 2015, 2017) or reported in the literature; (2) implication in previous biomarker studies related to CNS injury (TBI, SCI, or neurodegeneration) or stroke; (3) potential data normalizer; (4) is detectable in CSF and plasma. The panel contained one additional rodent specific miRNA and the manufacturer’s mandatory control U6, both of which were excluded from analysis. CSF and plasma miRNA from a total of 31 aSAH patients (27 patients from UKCMC and 4 from UPENN) and 8 HCs were included in the TLDA analysis (Figure 1).
A miRNA was deemed detectable if it exhibited a Crt < 35 in at least two-thirds of the total specimens. Based on these criteria, six miRNAs from the CSF samples (miR-107, miR-144-3p, miR-153-3p, miR-15a-5p, miR-29b-3p, and miR-874-3p) and six miRNAs from the plasma samples (miR-124-3p, miR-144-3p, miR-1298-5p, miR-153-3p, miR-874-3p, and miR-9-5p) were removed from further analysis. Overall, a clear and distinct miRNA expression pattern was observed between the DCV+ and DCV- groups, aSAH and HC groups, and between sampling times (PBD3 vs. PBD7) in both biofluids (Figure 2).
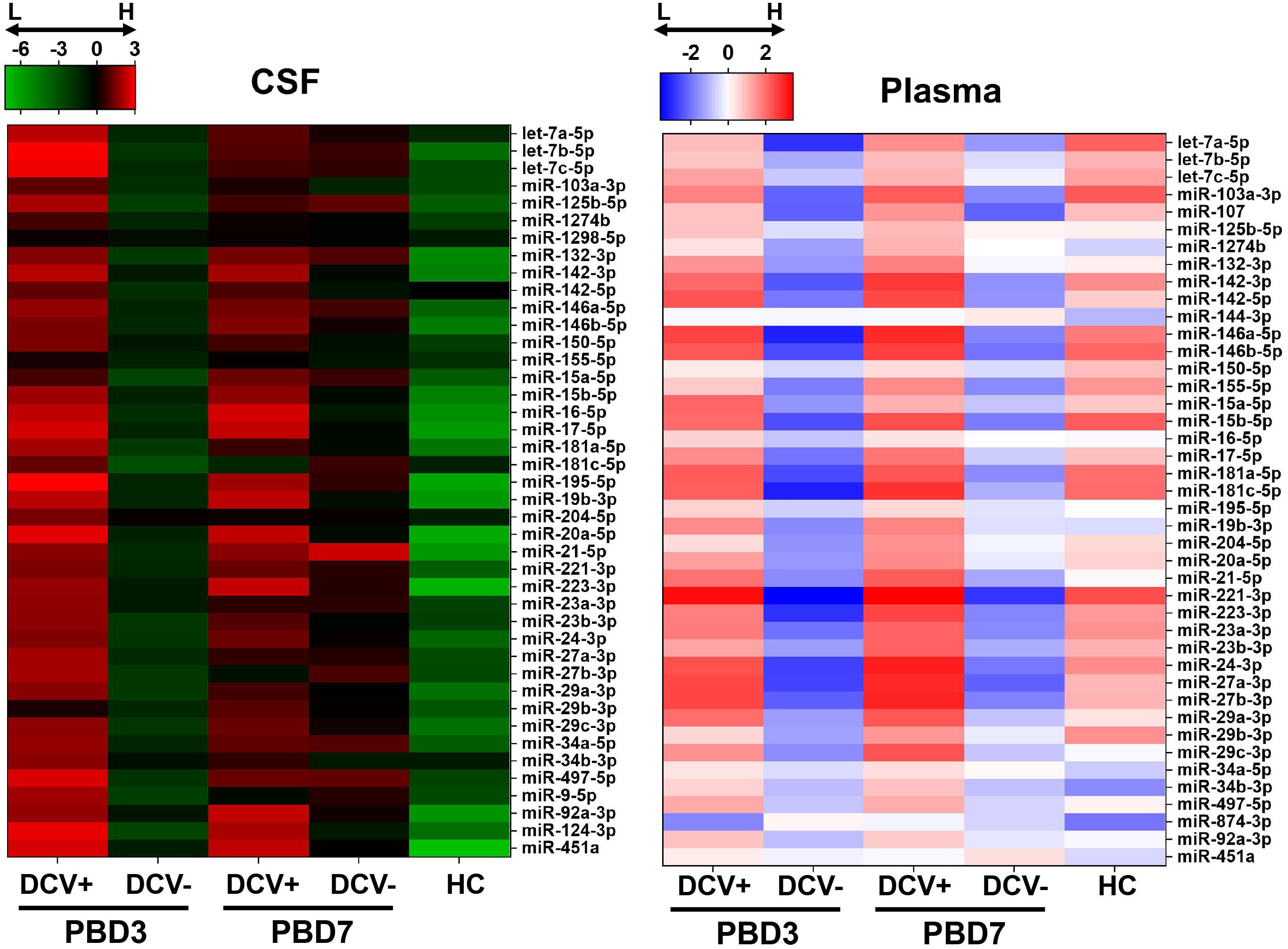
Figure 2. Heatmaps of overall miRNA expression in CSF and plasma specimens collected from aSAH patients and healthy controls (HC). Normalized –ΔCRT was used to generate the mean expression for each miRNA in each group.
Individual miRNA Performance in CSF and Plasma
The majority of the selected miRNAs in CSF and plasma showed significant differential expression between DCV+ and DCV- at PBD3, but was reduced by PBD7 (Table 3). The difference was especially robust in CSF specimens obtained at PBD3. Specifically, 37 miRNAs from the CSF demonstrated highly significant differences in samples collected from DCV+ relative to DCV- patients at PBD3. Several miRNAs including Let-7b-5p, miR-15b-5p, miR-17-5p, miR-19b-3p, miR-20a-5p, miR-24-3p, and miR-29a-3p exhibited remarkable differential levels between the DCV+ and DCV- groups at PBD3, as well as between the DCV+/PBD3 group and HCs (Figure 3 and Supplementary Figure 2). With respect to the plasma specimens, 29 miRNAs demonstrated significant differential expression between the DCV+ and DCV- groups at PBD3 (Table 3), with several miRNAs (Let-7a-5p, miR-146a-5p, miR-204-5p, miR-221-3p, miR-23a-3p, miR-497-5p) showing high differential expression patterns (Figure 4).
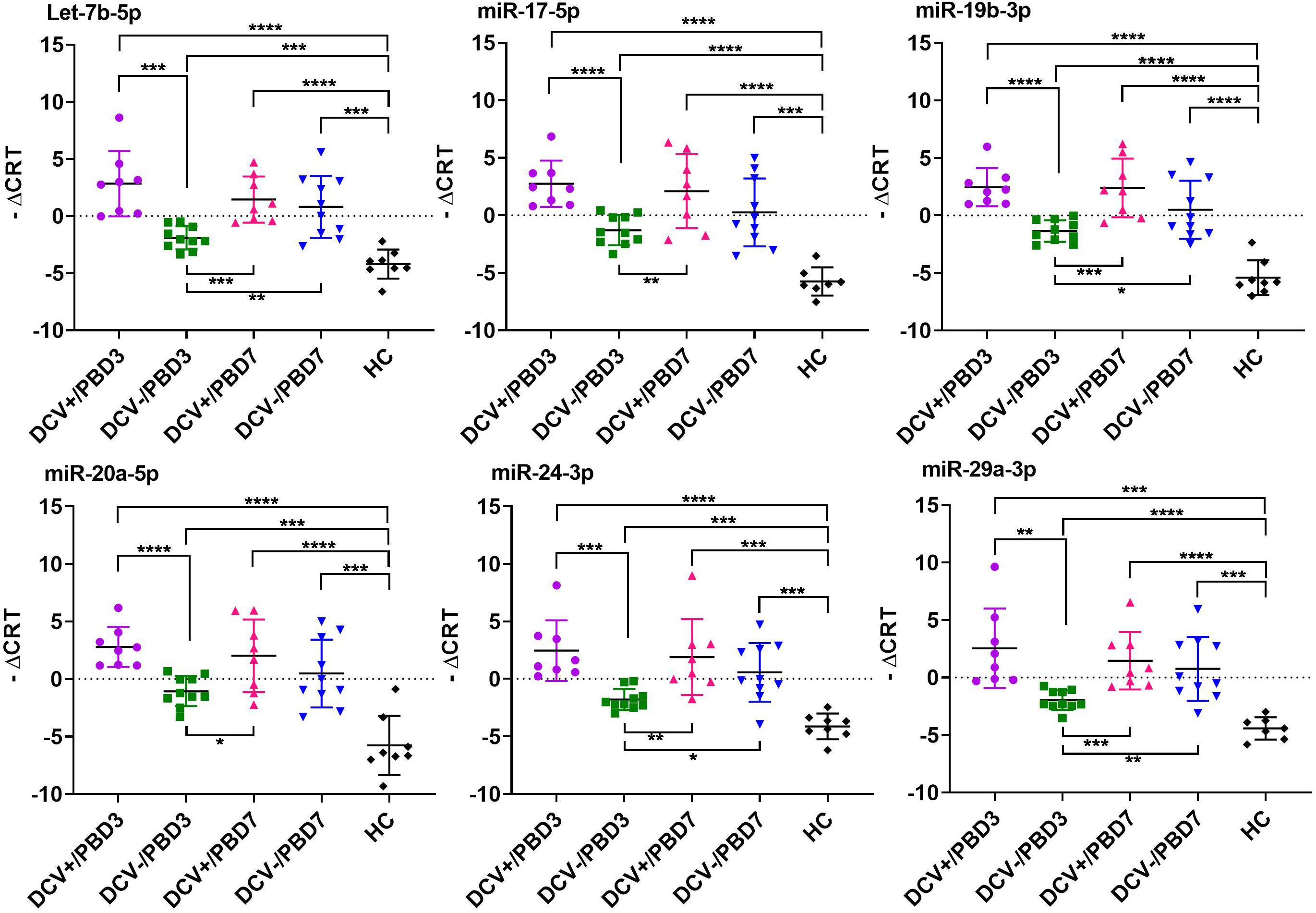
Figure 3. Individual CSF miRNAs that showed highly differential expression in DCV+, DCV-, and HC groups. The differential miRNA expression levels are expressed as –ΔCRT and a two tailed Student’s t-test was used to evaluate differences between groups. Significant levels expressed as: ****p < 0.0001; ***p = 0.0001–0.001; **p = 0.001–0.01; *p = 0.01–0.05.
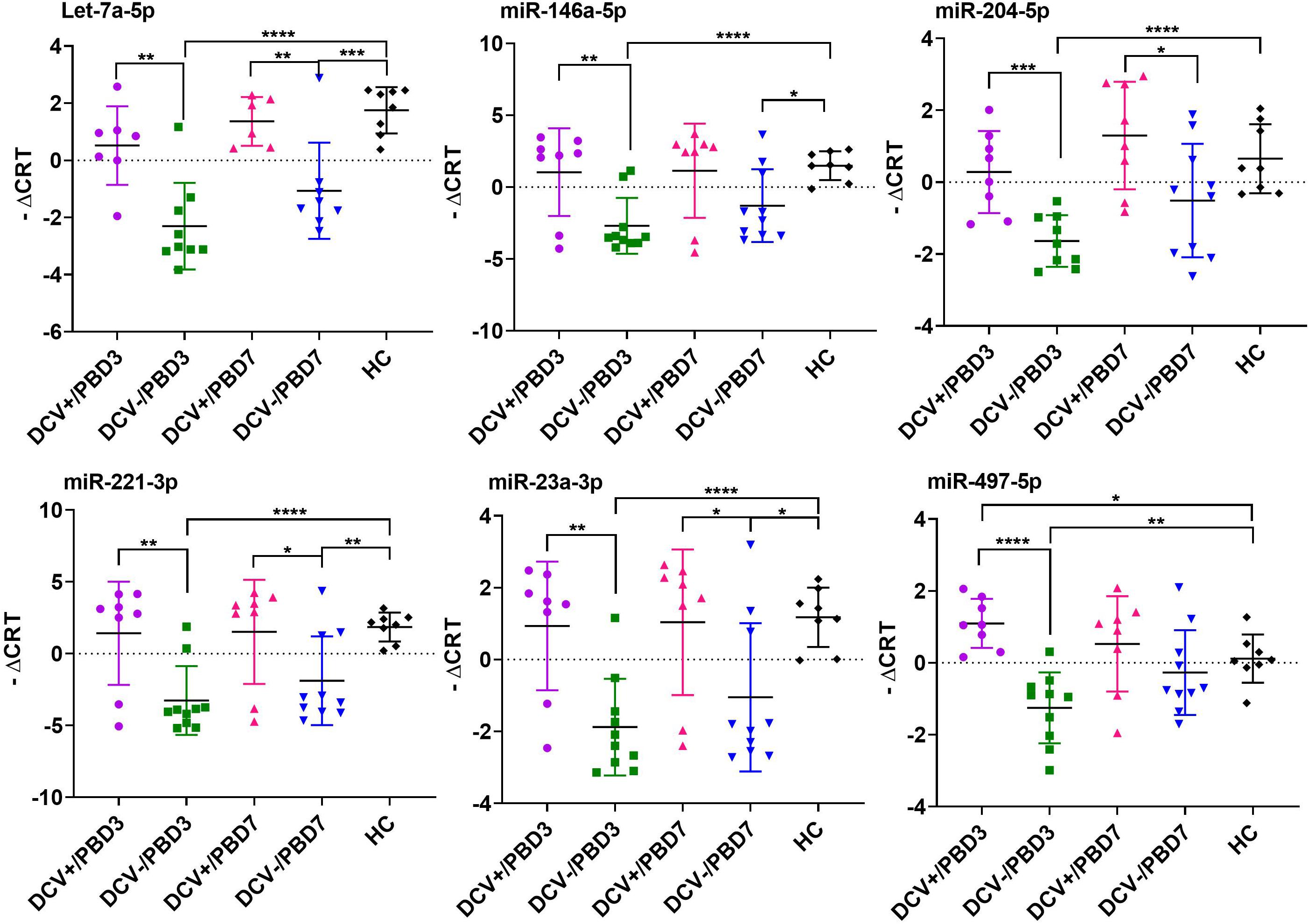
Figure 4. Individual plasma miRNAs that showed highly differential expression in DCV+, DCV–, and HC groups. The differential miRNA expression levels are expressed as –ΔCRT and a two tailed Student’s t-test was used to evaluate the difference between groups. Significant levels expressed as: ****p < 0.0001; ***p = 0.0001–0.001; **p = 0.001–0.01; *p = 0.01–0.05.
Receiver Operating Characteristic (ROC) Curves of Individual miRNAs
The sensitivity and specificity of individual miRNAs as predictors of DCV were examined using the area under the ROC curve (AUC). Many miRNAs demonstrated an AUC greater than 0.9 in CSF collected at PBD3 indicating a highly significant predictive ability of these miRNAs (Figure 5A). Of note, the AUC of Let-7b-5p, miR-15b-5p, miR-17-5p, miR-19b-3p, miR-20a-5p, miR-24-3p, and miR-29a-3p reached 1 (AUC = 1), signifying a perfect discrimination between DCV+ and DCV- at a time point prior to DCV onset. Similarly, several plasma miRNAs achieved AUCs greater than 0.8 (Figure 5B), demonstrating a robust ability of selected miRNA for discriminating DCV in two biofluids.
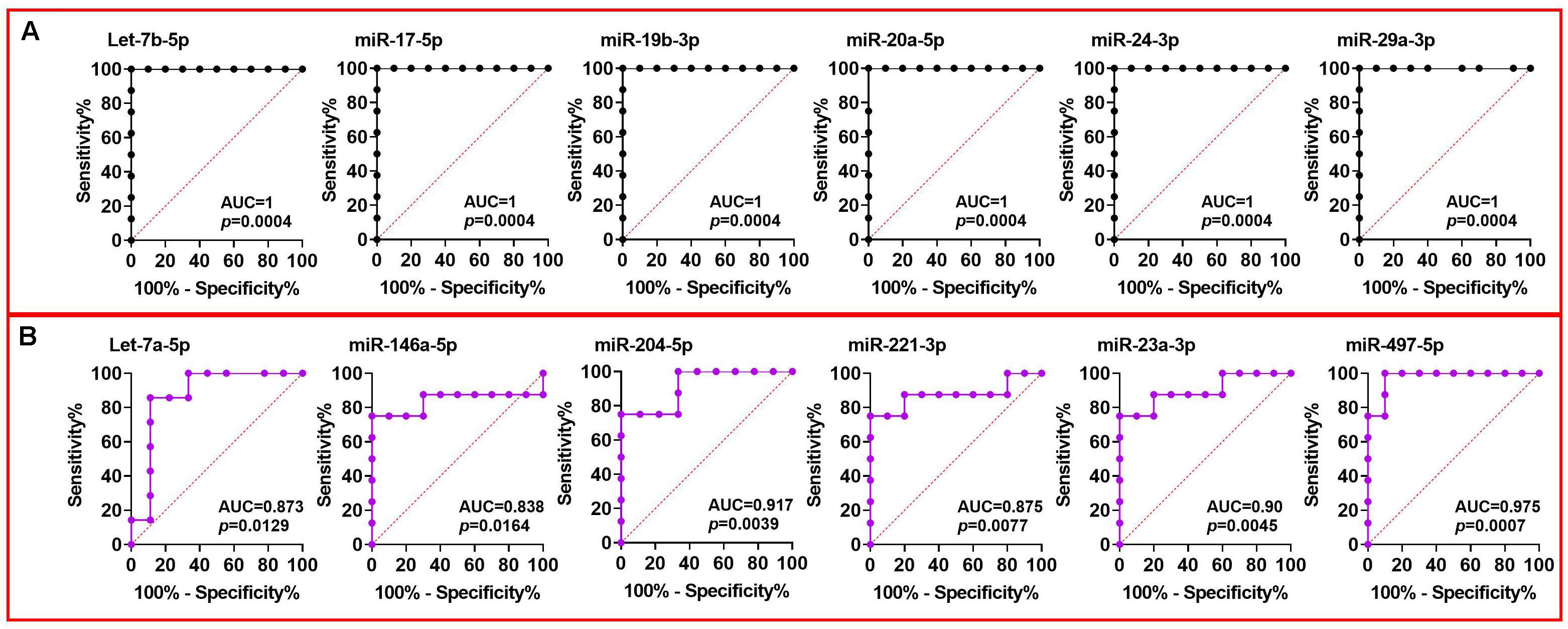
Figure 5. ROC analysis of miRNAs using Group A CSF and plasma data collected at PBD3 from aSAH patients. ROC curve constructed using (A) DCV+ and DCV– groups of CSF miRNA data; (B) DCV+ and DCV– groups of plasma miRNA data.
High AUC values were also achieved when comparing aSAH and HC using CSF/PBD3 data (Supplementary Figure 3), indicating that selected CSF miRNAs can also discriminate aSAH patients from HCs.
Prediction Accuracy of the Custom miRNA Panel on Group B and Group C
The CSF/PBD3 data from Group A was then used to build a predicting model, and the datasets from the remaining 15 cases from Group B (including 2 replicates from Group A) and Group C were used for retrospective risk prediction. A risk prediction accuracy of 93% was achieved with miR-19b-3p and miR-29a-3p using the corresponding cutoff values of -0.481 and 0.53, respectively (Table 4 and Supplementary File 1). In contrast, miR-24-3p had only 73% accuracy using the corresponding cutoff value of -0.011.
Decision Tree (DT) Results
MiRNAs with an AUC less than 1 were used to generate a decision tree model (Figure 6). Out of the 19 miRNAs entered into the model, miR-142-3p and miR-1274b were identified as key variables in the prediction process. This model correctly predicted 100% of the training dataset (Group A, Figure 6), and 87% of the test dataset (Group B + Group C, Table 4).
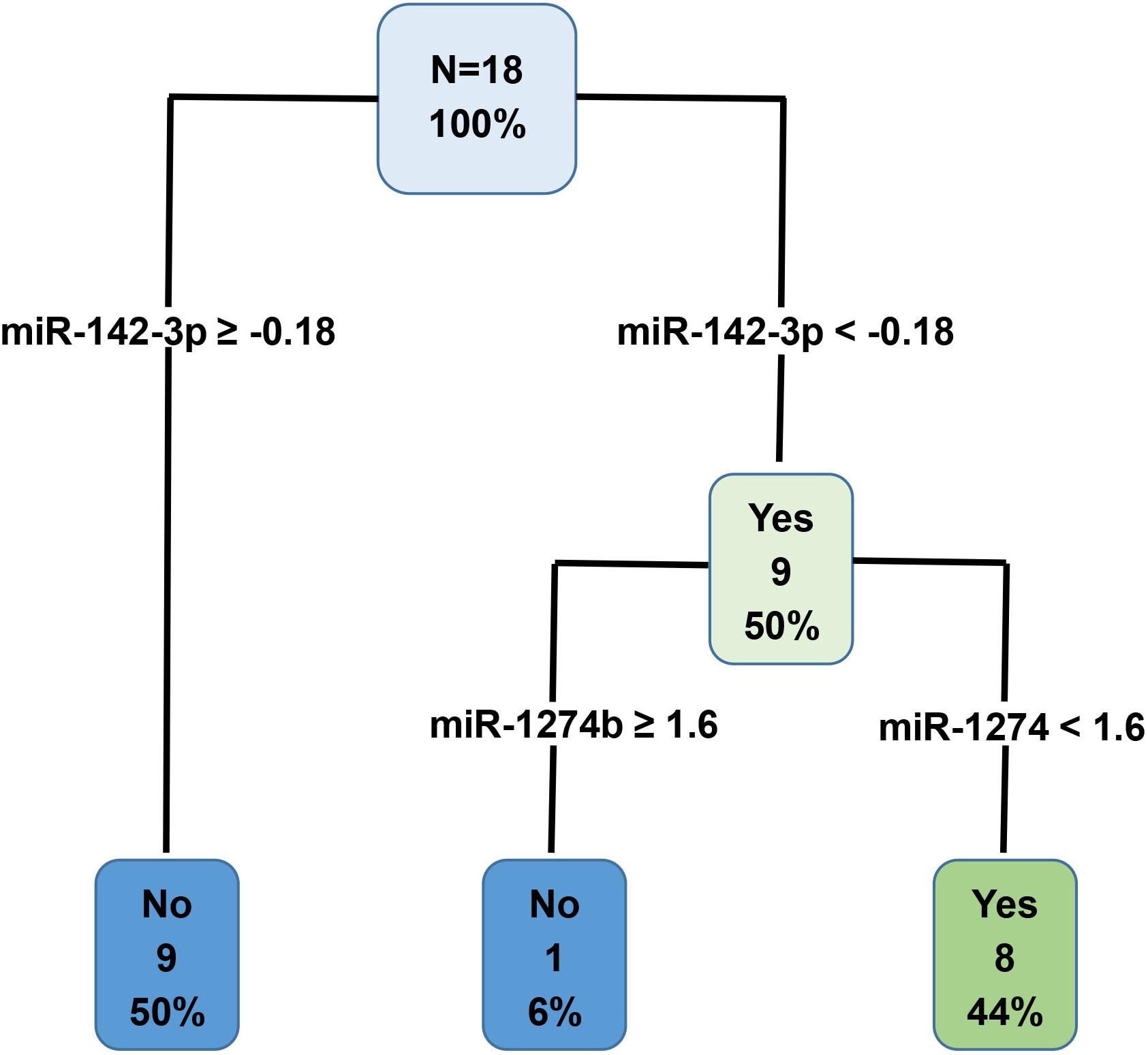
Figure 6. Decision tree prediction model was generated using the Group A training dataset. Out of the 19 variables entered into the model, miR-142-3p and miR-1274b were identified as key variables in the prediction process. Specifically, these two miRNAs correctly predicted 100% of the Group A training dataset.
Overall Prediction of the miRNA Panel
Table 4 presents a consensus prediction table incorporating 7 miRNAs with a perfect AUC of 1 and additional 19 miRNAs from the DT. The prediction of each testing subject was determined when at least five of the eight criteria were met for either DCV+ or DCV-. Notably, the majority of the predictions were uniformly accurate across the test dataset with exceptions in only two out of 15 test cases. Case 4 had only false positive predictions, while case 15 resulted in 5 out of 8 false positive predictions. Despite the two false positive predictions, the overall performance of the custom panel in predicting DCV risk was 87% accurate.
Discussion
The potential of miRNAs serving as biomarkers for aSAH complications was recently explored in several reports (Su et al., 2015; Powers et al., 2016; Bache et al., 2017; Kikkawa et al., 2017; Lu et al., 2017; Stylli et al., 2017; Lopes et al., 2018; Pulcrano-Nicolas et al., 2018; Sheng et al., 2018a). These studies confirmed that dysregulated miRNA expression is associated with aSAH and its acute complications, which could be observed in extracellular fluids. However, there is no consensus in the limited literature for targeting biofluid miRNAs as a valid biomarkers for predicting DCV. This is due, in part, to significant variability across studies, including differences in sampling size (4–129 cases), type of biofluid used (whole blood, CSF, serum, plasma), collection time points (1–14 days), as well as miRNA analysis methodologies. Several studies utilized time-consuming and costly analytical methodologies such as next-generation sequencing and microarray (Su et al., 2015; Kikkawa et al., 2017; Pulcrano-Nicolas et al., 2018) that, while informative, may limit clinical applications.
The major difference between our study and other reports is that we employed a panel of selected miRNAs that is highly relevant to brain and vasculature injury events. This strategy directly targets a condition with a dedicated set of miRNAs involving related pathological processes. By doing so, the likelihood of identifying responsive biomarkers is much greater and may be achieved using a smaller patient cohort. Furthermore, multiple miRNAs can be used concomitantly in the overall assessment of DCV risk to increase prediction accuracy.
Our study revealed a robust differential expression of the selected miRNAs between DCV+ and DCV- patients, as well as between aSAH and HCs in both CSF and plasma, with the CSF samples showing the most pronounced difference. This differential pattern resulted in a high prediction accuracy of 87% in our independent cohort studies. It is important to point out that all of the predictor miRNAs falsely identified case 4 to be DCV positive (Table 4), and five of the eight predictors incorrectly identified case 15 to be DCV positive. It is not entirely clear why these two cases resulted in incorrect predictions, and it may be related, in part, with the complex clinical conditions or comorbidities of the individuals.
Several miRNAs in our panel were previously reported to link to aSAH related complications. Significantly increased levels of miR-21-5p and miR-221-3p in CSF were shown to associate with delayed cerebral ischemia (DCI) and miR-221-3p, miR-132-3p, and miR-19b-3p in CSF were associated with DCV (Bache et al., 2017). In addition, CSF expression levels of miR-27a-3p were differentially expressed between aSAH patients with or without DCV (Stylli et al., 2017). Finally, temporal changes in Let-7b-5p and miR-92a-3p in CSF, and miR-15a in both CSF and plasma of DCI patients have been reported (Powers et al., 2016; Kikkawa et al., 2017). In other studies, the levels of miR-146a-5p, miR-17-5p, and miR-451a in whole blood were significantly different in aSAH patients compared to the HCs (Lopes et al., 2018). Su et al. (2015) reported that miR-132-3p is differentially expressed in peripheral blood between DCI+ and DCI- patients. Our data were generally consistent with the findings of these prior studies. However, because the wide differences in the patient cohorts, specimens used, sampling/analysis time points, detection methodologies, and variations on study focus (e.g., aSAH vs. control, occurrence of DCV or DCI, etc.), it is difficult to make direct comparisons between our results and those reported in the previously published studies.
Another important advantage of our panel is that individual miRNAs are easily replaced if they do not perform well. Not all of the miRNAs on our current panel contributed to DCV prediction and several miRNAs were excluded from further analysis due to their low expression levels (e.g., miR-144-5p, miR-153-3p, and miR-874-3p). In addition, other miRNAs, such as miR-1298-5p in CSF and miR-16-5p in plasma, did not differentiate between DCV+ and DCV-. Therefore, replacing these miRNAs with other candidate miRNAs, for example, miR-502, miR-516a-5p, and miR-1297 (Lai et al., 2017; Stylli et al., 2017), that may prove more effective in DCV prediction will be considered in future studies.
We recognize that there are several limitations with our current study. First, the patient cohort was relatively small, and samples were limited to two institutions. This can be partially attributed to the rarity of aSAH. The incidence of aSAH is 6–16 cases per 100,000 persons, with approximately 30,000 cases occurring each year in the United States (de Rooij et al., 2007). To fully address this limitation and to validate the DCV miRNA panel, future studies will need to expand the patient cohort and include recruitment from various medical institutions. In addition, we are currently exploring the possibility of validating our data in aSAH animal models. Second, there were only two sampling time points. Prior studies reported that altered miRNA expression levels in biofluids following aSAH are temporally dynamic (Powers et al., 2016; Kikkawa et al., 2017; Sheng et al., 2018b; Bache et al., 2020). Therefore, it will be critical to identify a time point that shows the greatest differences in miRNA expression levels and, more importantly, provides the earliest prediction to allow clinicians time to make medical decisions. Third, the detection of DCV miRNAs was solely based on TaqMan miRNA assay technology. Although this technology has also been successfully applied in other aSAH miRNA studies (Bache et al., 2017, 2020), it will be important to validate this miRNA panel using a different detection method to avoid technological bias. Lastly, the current study did not correlate the expression of selected miRNAs with other aSAH complications, such as DCI or early brain injury, nor with the prognosis. Our focus was to examine DCV after aSAH and future studies are warranted to evaluate how this novel miRNA biomarker panel might be applied these other scenarios and how it might impact clinical practice.
Data Availability Statement
The data presented in the study are deposited in the National Center for Biotechnology Information (NCBI) Gene Expression Omnibus (GEO) repository (https://www.ncbi.nlm.nih.gov/geo/), accession number GSE165608.
Ethics Statement
The studies involving human participants were reviewed and approved by the University of Kentucky Institutional Review Board. The patients/participants provided their written informed consent to participate in this study.
Author Contributions
WXW, KWH, and JES: project concept and design and manuscript preparation. WXW and KWH: experimental implementations and data acquisition and analysis. KX, DWF, and WXW: statistical analysis. KWH, JES, and WXW: specimen procurement. WXW, KWH, JES, KX, and DWF: manuscript editing and review. All authors contributed to the article and approved the submitted version.
Funding
This work was supported by Grants 15-12A and 18-8A from the Kentucky Spinal Cord and Head Injury Research Trust (WXW and JES) and an endowment from the Kentucky Spinal Cord and Head Injury Research Trust (JS). Healthy control specimens were obtained from the University of Kentucky Alzheimer’s Disease Center (UK-ADC), supported by NIH Grant P30 AG280303.
Conflict of Interest
The authors declare that the research was conducted in the absence of any commercial or financial relationships that could be construed as a potential conflict of interest.
Acknowledgments
We are indebted to the patients for their role in this study.
Supplementary Material
The Supplementary Material for this article can be found online at: https://www.frontiersin.org/articles/10.3389/fmolb.2021.657258/full#supplementary-material
Supplementary Figure 1 | Workflow and estimated time needed for miRNA isolation and TaqMan TLDA analysis.
Supplementary Figure 2 | Distribution of miRNA expression values for 26 miRNAs between DCV+ and DCV- of the Group A cases. Each filled circle corresponds to a single case with “Yes” referring to DCV+ and “No” referring to DCV-.
Supplementary Figure 3 | ROC curves constructed using CSF miRNA data (PBD3) from aSAHs (DCV+ plus DCV- group) and HCs.
Supplementary File 1 | The file contains (1) variables without missing data; (2) DCV prediction cutoff value; (3) CSF dataset; (4) plasma dataset.
References
Al-Mufti, F., Amuluru, K., Changa, A., Lander, M., Patel, N., Wajswol, E., et al. (2017). Traumatic brain injury and intracranial hemorrhage-induced cerebral vasospasm: a systematic review. Neurosurg. Focus 43:E14.
Applied Biosystems (2016). Crt, A Relative Threshold Method For QPCR Data Analysis On The Quantstudiotm 12K Flex system with openarray® technology. Appl Biosyst quantstudiotm 12K Flex Real-Time PCR Syst Appl Note CO28730, (Carlsbad, CA: Life Technologies Corporation). 4.
Bache, S., Rasmussen, R., Rossing, M., Laigaard, F. P., Nielsen, F. C., and Moller, K. (2017). MicroRNA Changes in cerebrospinal fluid after subarachnoid hemorrhage. Stroke 48, 2391–2398. doi: 10.1161/strokeaha.117.017804
Bache, S., Rasmussen, R., Wolcott, Z., Rossing, M., Møgelvang, R., Tolnai, D., et al. (2020). Elevated miR-9 in cerebrospinal fluid is associated with poor functional outcome after subarachnoid hemorrhage. Trans. Stroke Res. 11, 1243–1252. doi: 10.1007/s12975-020-00793-1
Brami, J., Chousterman, B., Boulouis, G., Dorze, M. L., Majlath, M., Saint-Maurice, J. P., et al. (2020). Delayed cerebral infarction is systematically associated with a cerebral vasospasm of large intracranial arteries. Neurosurgery 86, E175–E183.
Carr, K. R., Zuckerman, S. L., and Mocco, J. (2013). Inflammation, cerebral vasospasm, and evolving theories of delayed cerebral ischemia. Neurol. Res. Int. 2013:506584.
Chou, S. H. (2018). Inflammation, cerebral vasospasm, and brain injury in subarachnoid hemorrhage-A shifting paradigm and a new beginning. Crit. Care Med. 46, 1883–1885. doi: 10.1097/ccm.0000000000003373
de Rooij, N. K., Linn, F. H., Van Der Plas, J. A., Algra, A., and Rinkel, G. J. (2007). Incidence of subarachnoid haemorrhage: a systematic review with emphasis on region, age, gender and time trends. J. Neurol. Neurosurg. Psychiatry 78, 1365–1372. doi: 10.1136/jnnp.2007.117655
Dietrich, H. H., and Dacey, R. G. Jr. (2000). Molecular keys to the problems of cerebral vasospasm. Neurosurgery 46, 517–530. doi: 10.1097/00006123-200003000-00001
Dority, J. S., and Oldham, J. S. (2016). Subarachnoid hemorrhage: an update. Anesthesiol. Clin. 34, 577–600.
Etheridge, A., Lee, I., Hood, L., Galas, D., and Wang, K. (2011). Extracellular microRNA: a new source of biomarkers. Mutat. Res. 717, 85–90. doi: 10.1016/j.mrfmmm.2011.03.004
Etminan, N., Vergouwen, M. D., Ilodigwe, D., and Macdonald, R. L. (2011). Effect of pharmaceutical treatment on vasospasm, delayed cerebral ischemia, and clinical outcome in patients with aneurysmal subarachnoid hemorrhage: a systematic review and meta-analysis. J. Cereb. Blood Flow Metab. 31, 1443–1451. doi: 10.1038/jcbfm.2011.7
Frontera, J. A., Claassen, J., Schmidt, J. M., Wartenberg, K. E., Temes, R., and Connolly, E. S. Jr., et al. (2006). Prediction of symptomatic vasospasm after subarachnoid hemorrhage: the modified fisher scale. Neurosurgery 59, 21–27. discussion 21-27,Google Scholar
Hill, J. M., and Lukiw, W. J. (2016). MicroRNA (miRNA)-mediated pathogenetic signaling in Alzheimer’s disease (AD). Neurochem. Res. 41, 96–100. doi: 10.1007/s11064-015-1734-7
Huntzinger, E., and Izaurralde, E. (2011). Gene silencing by microRNAs: contributions of translational repression and mRNA decay. Nat. Rev. Genet. 12, 99–110. doi: 10.1038/nrg2936
Janjua, N., and Mayer, S. A. (2003). Cerebral vasospasm after subarachnoid hemorrhage. Curr. Opin. Crit. Care 9, 113–119.
Khoshnam, S. E., Winlow, W., Farbood, Y., Moghaddam, H. F., and Farzaneh, M. (2017). Emerging roles of micrornas in ischemic stroke: as possible therapeutic agents. J. Stroke 19, 166–187. doi: 10.5853/jos.2016.01368
Kikkawa, Y., Ogura, T., Nakajima, H., Ikeda, T., Takeda, R., Neki, H., et al. (2017). Altered expression of microRNA-15a and kruppel-like factor 4 in cerebrospinal fluid and plasma after aneurysmal subarachnoid hemorrhage. World Neurosurg. 108, 909–916.e903.
Kirsch, J. D., Mathur, M., Johnson, M. H., Gowthaman, G., and Scoutt, L. M. (2013). Advances in transcranial Doppler US: imaging ahead. Radiographics 33, E1–E14.
Kolias, A. G., Sen, J., and Belli, A. (2009). Pathogenesis of cerebral vasospasm following aneurysmal subarachnoid hemorrhage: putative mechanisms and novel approaches. J. Neurosci. Res. 87, 1–11. doi: 10.1002/jnr.21823
Koutsis, G., Siasos, G., and Spengos, K. (2013). The emerging role of microRNA in stroke. Curr. Top Med. Chem. 13, 1573–1588. doi: 10.2174/15680266113139990106
Lai, N. S., Zhang, J. Q., Qin, F. Y., Sheng, B., Fang, X. G., and Li, Z. B. (2017). Serum microRNAs are non-invasive biomarkers for the presence and progression of subarachnoid haemorrhage. Biosci. Rep. 37:BSR20160480.
Li, H. T., Wang, J., Li, S. F., Cheng, L., Tang, W. Z., and Feng, Y. G. (2018). Upregulation of microRNA24 causes vasospasm following subarachnoid hemorrhage by suppressing the expression of endothelial nitric oxide synthase. Mol. Med. Rep. 18, 1181–1187.
Liu, D., Han, L., Wu, X., Yang, X., Zhang, Q., and Jiang, F. (2014). Genome-wide microRNA changes in human intracranial aneurysms. BMC Neurol 14:188.
Liu, N. K., and Xu, X. M. (2011). MicroRNA in central nervous system trauma and degenerative disorders. Physiol. Genom. 43, 571–580. doi: 10.1152/physiolgenomics.00168.2010
Lopes, K. P., Vinasco-Sandoval, T., Vialle, R. A., Paschoal, F. M. Jr., and Bastos, V. (2018). Global miRNA expression profile reveals novel molecular players in aneurysmal subarachnoid haemorrhage. Sci. Rep. 8:8786.
Lu, G., Wong, M. S., Xiong, M. Z. Q., Leung, C. K., Su, X. W., Zhou, J. Y., et al. (2017). Circulating microRNAs in delayed cerebral infarction after aneurysmal subarachnoid hemorrhage. J. Am. Heart Assoc. 6:e005363.
Macdonald, R. L., and Weir, B. K. (1991). A review of hemoglobin and the pathogenesis of cerebral vasospasm. Stroke 22, 971–982. doi: 10.1161/01.str.22.8.971
Mestdagh, P., Van Vlierberghe, P., De Weer, A., Muth, D., Westermann, F., Speleman, F., et al. (2009). A novel and universal method for microRNA RT-qPCR data normalization. Genome Biol. 10:R64.
Miller, B. A., Turan, N., Chau, M., and Pradilla, G. (2014). Inflammation, vasospasm, and brain injury after subarachnoid hemorrhage. Biomed. Res. Int. 2014:384342.
Mocco, J., Zacharia, B. E., Komotar, R. J., and Connolly, E. S. Jr. (2006). A review of current and future medical therapies for cerebral vasospasm following aneurysmal subarachnoid hemorrhage. Neurosurg. Focus 21:E9.
Mraz, M., Malinova, K., Mayer, J., and Pospisilova, S. (2009). MicroRNA isolation and stability in stored RNA samples. Biochem. Biophys. Res. Commun. 390, 1–4. doi: 10.1016/j.bbrc.2009.09.061
Muller, A. H., Povlsen, G. K., Bang-Berthelsen, C. H., Kruse, L. S., Nielsen, J., Warfvinge, K., et al. (2015). Regulation of microRNAs miR-30a and miR-143 in cerebral vasculature after experimental subarachnoid hemorrhage in rats. BMC Genomics 16:119. doi: 10.1186/s12864-015-1341-7
O’connell, R. M., Rao, D. S., and Baltimore, D. (2012). microRNA regulation of inflammatory responses. Annu. Rev. Immunol. 30, 295–312. doi: 10.1146/annurev-immunol-020711-075013
Powers, C. J., Dickerson, R., Zhang, S. W., Rink, C., Roy, S., and Sen, C. K. (2016). Human cerebrospinal fluid microRNA: temporal changes following subarachnoid hemorrhage. Physiol. Genom. 48, 361–366. doi: 10.1152/physiolgenomics.00052.2015
Pulcrano-Nicolas, A. S., Proust, C., Clarencon, F., Jacquens, A., Perret, C., Roux, M., et al. (2018). Whole-blood miRNA sequencing profiling for vasospasm in patients with aneurysmal subarachnoid hemorrhage. Stroke 49, 2220–2223. doi: 10.1161/strokeaha.118.021101
Sheng, B., Fang, X., Liu, C., Wu, D., Xia, D., Xu, S., et al. (2018a). Persistent high levels of miR-502-5p are associated with poor neurologic outcome in patients with aneurysmal subarachnoid hemorrhage. World Neurosurg. 116, e92–e99.
Sheng, B., Lai, N. S., Yao, Y., Dong, J., Li, Z. B., Zhao, X. T., et al. (2018b). Early serum miR-1297 is an indicator of poor neurological outcome in patients with aSAH. Biosci. Rep. 38:BSR20180646.
Stylli, S. S., Adamides, A. A., Koldej, R. M., Luwor, R. B., Ritchie, D. S., Ziogas, J., et al. (2017). miRNA expression profiling of cerebrospinal fluid in patients with aneurysmal subarachnoid hemorrhage. J. Neurosurg. 126, 1131–1139. doi: 10.3171/2016.1.jns151454
Su, X. W., Chan, A. H., Lu, G., Lin, M., Sze, J., Zhou, J. Y., et al. (2015). Circulating microRNA 132-3p and 324-3p profiles in patients after acute aneurysmal subarachnoid hemorrhage. PLoS One 10:e0144724. doi: 10.1371/journal.pone.0144724
Tan, J. R., Koo, Y. X., Kaur, P., Liu, F., Armugam, A., Wong, P. T., et al. (2011). microRNAs in stroke pathogenesis. Curr. Mol. Med. 11, 76–92. doi: 10.2174/156652411794859232
R Core Team. (2020). R: A Language and Environment for Statistical Computing. Vienna: R Foundation for Statistical Computing.
Therneau, T. A., and Atkinson, B. (2019). rpart: Recursive Partitioning and Regression Trees. R package, version 4.1-15. Available online at: https://cran.r-project.org/package=rpart [Epub ahead of print].
Vikman, P., Beg, S., Khurana, T. S., Hansen-Schwartz, J., and Edvinsson, L. (2006). Gene expression and molecular changes in cerebral arteries following subarachnoid hemorrhage in the rat. J. Neurosurg. 105, 438–444. doi: 10.3171/jns.2006.105.3.438
Wang, W. X., Danaher, R. J., Miller, C. S., Berger, J. R., Nubia, V. G., Wilfred, B. S., et al. (2014). Expression of miR-15/107 family microRNAs in human tissues and cultured rat brain cells. Genom. Proteom. Bioinform. 12, 19–30. doi: 10.1016/j.gpb.2013.10.003
Wang, W. X., Fardo, D. W., Jicha, G. A., and Nelson, P. T. (2017). A customized quantitative PCR microRNA panel provides a technically robust context for studying neurodegenerative disease biomarkers and indicates a high correlation between cerebrospinal fluid and choroid plexus microrna expression. Mol. Neurobiol. 54, 8191–8202. doi: 10.1007/s12035-016-0316-2
Wang, W. X., Rajeev, B. W., Stromberg, A. J., Ren, N., Tang, G., Huang, Q., et al. (2008). The expression of microRNA miR-107 decreases early in Alzheimer’s disease and may accelerate disease progression through regulation of beta-site amyloid precursor protein-cleaving enzyme 1. J. Neurosci. 28, 1213–1223. doi: 10.1523/jneurosci.5065-07.2008
Wang, W. X., Visavadiya, N. P., Pandya, J. D., Nelson, P. T., Sullivan, P. G., and Springer, J. E. (2015). Mitochondria-associated microRNAs in rat hippocampus following traumatic brain injury. Exp. Neurol. 265, 84–93. doi: 10.1016/j.expneurol.2014.12.018
Keywords: microRNA, biomarker, aneurysmal subarachnoid hemorrhage, delayed cerebral vasospasm, cerebrospinal fluid, plasma
Citation: Wang WX, Springer JE, Xie K, Fardo DW and Hatton KW (2021) A Highly Predictive MicroRNA Panel for Determining Delayed Cerebral Vasospasm Risk Following Aneurysmal Subarachnoid Hemorrhage. Front. Mol. Biosci. 8:657258. doi: 10.3389/fmolb.2021.657258
Received: 22 January 2021; Accepted: 13 April 2021;
Published: 14 May 2021.
Edited by:
Denes V. Agoston, Karolinska Institutet (KI), SwedenReviewed by:
Shilpita Karmakar, Yale University, United StatesSiddharth Shukla, Howard Hughes Medical Institute (HHMI), United States
Copyright © 2021 Wang, Springer, Xie, Fardo and Hatton. This is an open-access article distributed under the terms of the Creative Commons Attribution License (CC BY). The use, distribution or reproduction in other forums is permitted, provided the original author(s) and the copyright owner(s) are credited and that the original publication in this journal is cited, in accordance with accepted academic practice. No use, distribution or reproduction is permitted which does not comply with these terms.
*Correspondence: Wang-Xia Wang, d3dhbmdjQHVreS5lZHU=; orcid.org/0000-0002-8104-3779; Kevin W. Hatton, a2V2aW4uaGF0dG9uQHVreS5lZHU=; orcid.org/0000-0002-9388-0232