- 1Microbiology Laboratory, Department of Genetic Engineering and Biotechnology, University of Rajshahi, Rajshahi, Bangladesh
- 2Department of Genetic Engineering and Biotechnology, University of Rajshahi, Rajshahi, Bangladesh
- 3Department of Pharmacy, International Islamic University Chittagong, Chittagong, Bangladesh
- 4Department of Pharmacology, College of Pharmacy, King Khalid University, Abha, Saudi Arabia
- 5Department of Medicinal Chemistry, Faculty of Pharmacy, Philadelphia University-Jordan, Amman, Jordan
- 6Department of Pharmacy, BGC Trust University Bangladesh, Chittagong, Bangladesh
The recently emerged coronavirus (SARS-CoV-2) has created a crisis in world health, and economic sectors as an effective treatment or vaccine candidates are still developing. Besides, negative results in clinical trials and effective cheap solution against this deadly virus have brought new challenges. The viral protein, the main protease from SARS-CoV-2, can be effectively targeted due to its viral replication and pathogenesis role. In this study, we have enlisted 88 peptides from the AVPdb database. The peptide molecules were modeled to carry out the docking interactions. The four peptides molecules, P14, P39, P41, and P74, had more binding energy than the rest of the peptides in multiple docking programs. Interestingly, the active points of the main protease from SARS-CoV-2, Cys145, Leu141, Ser139, Phe140, Leu167, and Gln189, showed nonbonded interaction with the peptide molecules. The molecular dynamics simulation study was carried out for 200 ns to find out the docked complex’s stability where their stability index was proved to be positive compared to the apo and control complex. Our computational works based on peptide molecules may aid the future development of therapeutic options against SARS-CoV-2.
Introduction
The novel coronavirus, currently known as SARS-CoV-2 (severe acute respiratory syndrome coronavirus-2), originated in Wuhan, Hubei Province, China, and then spread rapidly worldwide (Singhal, 2020; Wang et al., 2020a). SARS-CoV-2 is a virus with a high contagious property. As the virus is highly contagious, it spreads quickly to many other regions of China and other countries of the world within just 1 month (Luo et al., 2020). As of this study’s writing, 3,060,651 people have died so far in COVID-19, and confirmed cases are 143,663,539 (https://www.worldometers.info/coronavirus/#countries). This virus has spread worldwide, but Europe can be called the epicenter of COVID-19 because of its worse attack than China (Ceylan, 2020).
Later in December 2019, pneumonia-like symptoms of indecipherable etiology were confirmed in Wuhan, Hubei Province, China, where seafood in conjunction with wild and farmed animal species is vented in a wholesale seafood market, that is, preliminarily thought to be enacting the phenomenon of this worldwide outbreak (Zheng, 2020). As of this study’s writing, 2,600,162 people have died so far in COVID-19 and confirmed cases are 117,089,586 (https://www.worldometers.info/coronavirus/#countries). SARS-CoV-2 is a zoonotic transformation disease where the disease spreads from animal to human via the intermediate host (Dehelean et al., 2020). Bat-coronavirus (bat-nCoV) RaTG13 exerted near about 96% sequence identity with SARS-CoV-2 that sorely indicates bats are the main natural reservoir. In contrast, few criteria like clinical signs, histological changes, and circulating antibodies of Malayan pangolins (Manis javanica) partook 90.1% identity and 99% similarity of genome sequence SARS-CoV-2 implying Malayan pangolins as an immediate, intermediate host of deadly COVID-19 disease (Li et al., 2020; Zhao et al., 2020). The very first four cases of SARS-CoV-2 disease are directly in contact with the seafood market from where this disease spreads rapidly among humans by person-to-person contamination via several ways of transference, among which droplet transmission and respiratory droplets are the most causative reasons for transmitting infection toward noninfected people. When people are nearly connected within 1.5 m, there is a highly risky chance of droplet transmission by forming droplets of mucosae or conjunctiva of an infected person. In contrast, airborne transmission, fecal–oral transmission, and vertical transmissions are lower risky transmission mediums of emerging SARS-CoV-2 strain (Acuti Martellucci et al., 2020; Wang et al., 2020b).
Glycoprotein-associated homotrimeric spike protein (S) is a fusion protein that comprises the S1 and S2 subunits. For the S1 subunit, spike protein held a binding with receptor-binding domain (RBD) within the host cell contrary to a fusion between cellular and viral membranes for the S2 subunit. This type of binding cascade leads the viral genome to enter into the cell from which it reproduces countless viruses, and the engrossing structure of spikes plays the premier role in spreading infection (Chan et al., 2020; Lan et al., 2020; Yang et al., 2020). Angiotensin-converting enzyme 2, abbreviated as ACE2, is recognized as a well-known receptor of life-threatening SARS-CoV-2, and by binding to ACE2, spike protein–containing virus then enters into the mucosal endothelial cells. It requires 96 h to enter human respiratory epithelial cells and near about take 6 days for the entire cell line, and after contamination, interacting of saliva and respiratory secretions through coughing or sneezing or talking or singing, this virus can touch the nose, mouth, or eyes of a noninfected person from which virus spreads toward circumstances (Zhang et al., 2020b; Shang et al., 2020; WHO, 2020).
Coronaviruses contain enveloped, positive-sense, single-stranded RNA. They are also known as the largest RNA viruses because of their large genome size. These viruses range in size from (65–125 nm) in diameter as they are minute in size (Ge et al., 2020; Shereen et al., 2020). The SARS-CoV-2 belonged to the family Coronaviridae, which is in the order Nidovirales and Betacoronavirus genus (Zhong et al., 2003; Ge et al., 2020; Shereen et al., 2020). Seven coronaviruses can cause disease in the human body. The four human coronaviruses (HKU1, NL63, HCoV 229E, and OC43) are known to be globally endemic and cause damage to the respiratory tract of the elderly through infection of the upper portion of the tract. Alpha, beta, gamma, and delta—these are the four genera of coronaviruses (Chen et al., 2020; Ge et al., 2020; Wu et al., 2020a). As there are four subfamilies of coronaviruses, the alpha coronaviruses and beta coronaviruses possibly not only infect mammals but also originate from them. These mammals are specifically bats. Birds and pigs are the origins of another two subfamilies, gamma coronaviruses and delta coronaviruses. It is already known that coronaviruses have the largest genome and their genome size differs from 26 to 32 kb. Four leading structural genes encipher the membrane glycoprotein (M), the spike protein (S), nucleocapsid protein (N), and a small membrane protein (SM) (Velavan and Meyer, 2020; Zhou et al., 2020).
Since it has been predicted that SARS-CoV-2 originated from bats, the genome of this virus naturally matches about 96% of bats’ genome. This virus’s genome contains a single open reading frame (ORF) that encodes a polyprotein, cap structure at 5′, and a poly-A-tail at 3'. Cap structure and poly-A-tail are the two untranslated regions (UTRs). The genomic structure of SARS-CoV-2 starts from the 5′ end. Then, there are two viral replicases, open reading frame (ORF 1a and 1b). COVID-19 takes on a pandemic form in a very short time.
The main protease of SARS-CoV-2 (Mpro) is a leading enzyme that plays a crucial role in regulating viral replication and transcription, the functioning of viral replicase enzymes and self-maturation, and in the acclimatization of the immune response in the host, among further fundamental actions for progressing the pathogen within a host cell (Dai et al., 2020; Joshi et al., 2020; Silva Andrade et al., 2020; Wu et al., 2020b; Zhang et al., 2020a). The SARS-CoV main protease is a 33.8 kDa protease (also known as the 3C-like protease or 3CLpro), a promising target for drug design due to its unexpected inhibitor mode binding site (Yang et al., 2003). Along with, SARS-CoV main protease has a pivotal role in mediating viral replication, multiplication, and transcription, as well as it is a key enzyme in the viral life cycle. On the point of being primarily inactive, the last C-terminal helix in domain III of Mpro monomer is involved in the dimerization of the main protease, and the derived homodimer is the active form of the enzyme which contains an intercommunicating attachment, chiefly between domain II of molecule A and the N-finger (NH2-terminal residues) of molecule B, aligned perpendicular to one another (Suárez and Díaz, 2020; Ullrich and Nitsche, 2020; Zhang et al., 2020a). In protomers, dimerization of the enzyme is mandatory for catalytic activity because the N-finger (residues 1–7) in domain I of one protomer intervenes with Glu166 of another protomer and thus assists in forming the S1 pocket of the substrate-binding site and the oxyanion loop as well in protomers. Emphatically, the Thr285Ala substitution marked in the SARS-CoV-2 Mpro concedes the two domains III to appear slightly closer, evolving higher catalytic efficiency (Anand et al., 2002; Yang et al., 2003; Suárez and Díaz, 2020; Zhang et al., 2020a).
Few vaccine candidates have been applied to nonhuman primates (NHPs) during trial phases (Dagotto et al., 2020). Some notable vaccine candidates are PiCoVacc (Wang et al., 2020c), DNA vaccines (Yu et al., 2020), RNA vaccines (Corbett et al., 2020), (mRNA-1273), and adenovirus-based vaccines ChAdOx1 (van Doremalen et al., 2020) and Ad26 (Mercado et al., 2020). Moreover, very few promising vaccine candidates have been showing good results during the clinical trial and early phase clinical trial (Dagotto et al., 2020; Folegatti et al., 2020; Jackson et al., 2020; Zhu et al., 2020).
Materials and Methods
Peptide and Protein Preparation
The antiviral database AVPdb (Qureshi et al., 2014) was utilized for screening peptides. Primarily, 88 peptide sequences were extracted from the database based on the activity against SARS-CoV-2. The peptide sequences were modeled before the peptide modeling. The PEP-FOLD-3 webtool (Maupetit et al., 2009; Thévenet et al., 2012) was used to model the peptide structure by utilizing amino acid sequences from the peptide. The three-dimensional protein structure of the main protease from SARS-CoV-2 (6LU7) was taken from the protein data bank database (RCSB) (Berman et al., 2000). The protein structure was initially cleaned; water molecules and heteroatoms were removed. The cleaned protein structure was subjected to an energy minimization process in YASARA (Krieger et al., 2013) by employing the YASARA force field. The minimized structure was saved for further docking and simulation study.
Active Site Prediction
The CASTp (Computed Atlas of Surface Topology of protein) provides protein pockets and the protein's buried interface by solvent-accessible surface and molecular surface models. This webtool (www.scts.bioe.uic.edu/castp/) can precisely predict the functional and surface features. This program’s catalytic sites/active sites of the protein model can be identified (Binkowski et al., 2003).
Molecular Docking
The peptides were initially docked in PatchDock (Schneidman-Duhovny et al., 2005) tools by targeting the main protease of SARS-CoV-2. The protein structure was used as a receptor, and the peptides were input as ligand molecules. The best 10 conformations of each peptide–protein complex were further docked in FireDock tools (Mashiach et al., 2008). The peptides were further docked in ClusPro (Kozakov et al., 2017) tools to maintain the accuracy in the docking study.
SAR Analysis of Peptides
The ProtParam (Garg et al., 2016) tools were initially used for peptides properties calculation. The best 15 peptides based on binding energy were considered for the SAR analysis, where acidic, basic, polar, nonpolar amino acid, net charge at pH, molecular weight, and volume were considered. Furthermore, the principal component analysis of the peptide properties was also calculated to further explore the structural variance.
Molecular Dynamics Simulations
The molecular dynamics simulation study was employed in YASARA dynamics (Krieger et al., 2013) commercial package where AMBER14 force field was utilized. The co-crystalized protein complex of main protease (PDB ID: 6LU7) was used as control-1, whereas peptide molecules QYIKWPWYI and the main protease complex were used as the control-2 system. The peptide from control-2 has COVID-19-induced T cell recognition and currently in the preparatory stage of clinical trials (EudraCT 2020-002502-75, EudraCT 2020-002519-23) (Nelde et al., 2021). The ligand-free protein complex (apo) was also included in this study to compare the protein's stability after binding with the protein molecules. The protein complex was initially cleaned, and the hydrogen bond network system was optimized along with the removal of the bumps from protein structure. The peptides and protein complexes were taken into the simulation box, and the total environment of the simulation system was neutralized by adding water molecules; 0.9% NaCl and the pH was set 7.4 (Krieger and Vriend, 2015). The temperature of the simulation system was 310 k, and the simulation temperature was maintained with the aid of the Berendsen thermostat. The TIP3P water model was used, and the cell density was set as 1.012 gm/cm3. The simulation cell box was bigger than the peptide and epitope complexes by 20 Å so that the complex can move freely within the complex (Land and Humble, 2018). The long-range electrostatic interactions were calculated through PME or the particle mesh Ewald method (Krieger et al., 2006). The simulation was initially optimized with the help of steepest gradient approaches using 5,000 cycles. The simulation was run by using 1.25 fs time step. The trajectories were saved after every 100 ps to analyze the root mean square deviation, root mean square fluctuations, hydrogen bond, radius of gyration, and solvent accessible surface area (Bappy et al., 2020; Khan et al., 2020; Islam et al., 2020; Mahmud et al., 2020a; Mahmud et al., 2020b; Mahmud et al., 2021b; Swargiary et al., 2020; Chowdhury et al., 2021; Rakib et al., 2021; Uddin et al., 2021).
Results and Discussion
Active Site Prediction
The CASTp webserver helps to identify the active groove of the main protease from SARS-CoV-2. About twenty-five active points were determined in CASTp tools; Arg 188, Asn119, Asn142, Asp187, Cys44, Cys145, Gln189, Gln192, Glu166, Gly143, His41, His164, Leu27, Leu167, Met49, Met165, Phe181, Pro168, Pro52, Thr24, Thr25, Thr26, Thr45, Tyr54, and Val186. These amino acids from the main protease were carefully assessed and considered for the peptide screening process via molecular docking.
Molecular Docking
The peptide sequences were modeled in PEP-FOLD server and incorporated in the Supplementary Figure S1. The docking energy from each peptide is documented in Supplementary Table S1. The most favorable binding energy found for P14, P39, P41, and P74 than other peptide models. The binding energy from FireDock server was found for best four peptides in between −55 and −60 kcal/mol. The FireDock energy for four peptides was as −58.45, −59.18, −55.13, and −59.16 kcal/mol, respectively, for P14, P39, P41, and P74 (Table 1). Moreover, to understand the binding with the main protease and peptide molecules, flexible docking approaches from ClusPro were also assessed. The binding pocket or targeted amino acid sequences were not specified for this docking study. However, strong binding energy was also found in ClusPro docking program. The P14, P39, P41, and P74 had binding energy in ClusPro program as −885.9, −839.2, −888.4, and −926.3 kcal/mol, respectively. The docking energy of the other peptides was reported in Supplementary Table S1.

TABLE 1. Docking score of the top four peptides based on binding energy. The binding energy was calculated through FireDock and ClusPro program.
Docking Interaction
A virtual screening process including molecular docking is regarded as a feasible approach for identifying reliable antiviral therapeutics among diverse peptide sequences available in the reliable online-based database. Notably, the interaction type, and the binding energy in conjunction with the bond distance of interaction between a protein substrate and a peptide ligand, can be evaluated through the utility of molecular docking. Therefore, screening a plausible peptide among numerous peptide sequences based on binding energy assessment is feasible within minimum time by running molecular docking to evaluate supreme peptide candidates according to the docking interaction. Moreover, diverse docking programs are compatible for engaging in the computational scheme to achieve accuracy (Shoichet, 2006; Sousa et al., 2010; Hengphasatporn et al., 2020; Maia et al., 2020). Consequently, a computational-based approach is considered a reliable technique for apprehending a repurposing antiviral peptide candidate against the SARS-CoV-2.
The P14 peptide created eight hydrogen bonds with the main protease from SARS-CoV-2 at Lys102, Gln110, Arg298, Ser301, Ile152, Ser158, and Val297 positions where each of the amino acid residue has bond distance below 2.5 Å indicating stronger binding interactions. This complex had five more hydrophobic bonds at Phe294 (Pi-sulfur), Tyr154 (Pi–Pi T-shaped), Pro252 (alkyl), Ile249 (alkyl), and His246 (Pi-alkyl) residues (Table 2 and Figure 1).
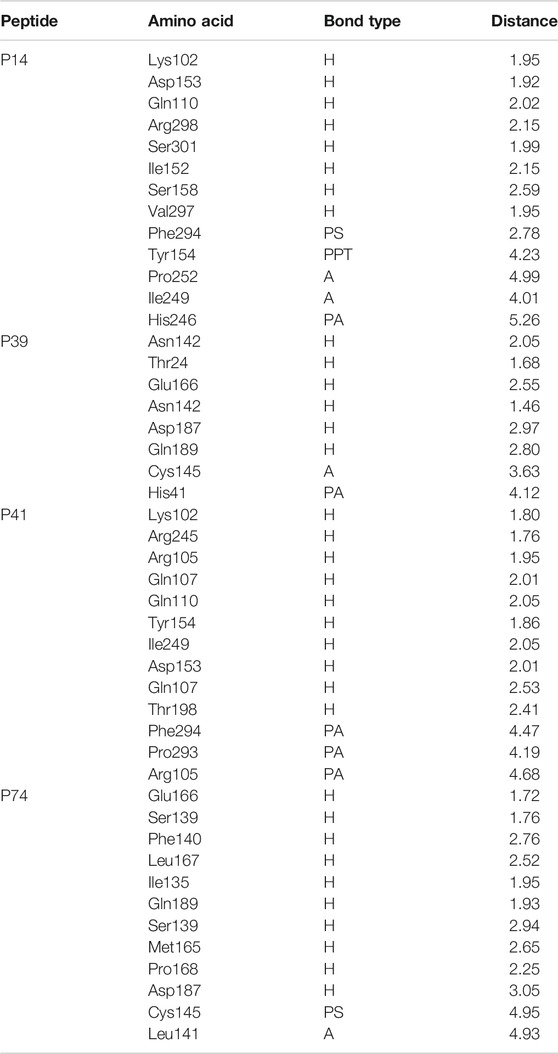
TABLE 2. Nonbonded interaction of the top four peptides and main protease of the SARS-CoV-2, here, H, A, PA, PS, and PPT denote hydrogen bond, alkyl bond, Pi-alkyl bond, Pi-sulfur bond, and Pi–Pi T-shaped interactions, respectively.
Therefore, the P39 complex stabilized by creating six hydrogen bonds at Asn142, Thr24, Glu166, Asn142, Asp187, and Gln189 positions. Two more hydrophobic bonds were also observed at Cys145 and His41 residues. Interestingly, all of the interacting residues, except Asp187, from P39 complex were in the active cavity of the main protease enzyme. These binding at the active groove may be responsible for the possible inhibition of the targeted protein (Table 2 and Figure 2).
Also, the P41 peptide creates ten hydrogen bonds with the target protein at Lys102, Arg245, Arg105, Gln107, Gln110, Tyr154, Ile249, Asp153, Gln107, and Thr198 residues. This complex also exhibited three more hydrophobic bonds at Phe294, Pro293, and Arg105 (Table 2 and Figure 3).
The P74 peptide and protein complex also generate ten hydrogen bonds at Glu166, Ser139, Phe140, Leu167, Ile135, Gln189, Ser139, Met165, Pro168, and Asp187 residues. This complex had one Pi-sulfur bond at Cys145 and one alkyl bond at Leu141. The P74 complex also had interaction in the catalytic residues at Cys145, Leu141, Ser139, Phe140, Leu167, and Gln189. These interactions at the active site of the protein may be responsible for the higher binding energy compared to the other peptides’ molecules (Table 2 and Figure 4).
Molecular Dynamics Simulation
In the computational drug discovery process, the flexibility of protein plays a significant role in ligand binding. Thus, to predict the motion of the protein, many computational techniques are needed. Unfortunately, the required calculations for demonstrating chemical reactions between complex molecular processes and farcical quantum-mechanical motions are mostly very much perplexing to understand. To surpass these complexities and invigorate atomic motions, molecular dynamics (MD) simulations use facile presumptions that are a cornerstone of Newtonian physics (Liu et al., 2018). To identify cryptic binding sites and keep the proteins and ligands flexible and calculate the ligands’ binding energies and allow binding sites’ relaxation close to the ligand, the evaluation of (MD) is essential (Okimoto et al., 2009). The study of molecular dynamics simulation in the main protease of SARS-CoV-2 was being used to figure out how flexible all the docked complex (four peptide complexes), and this evaluation was lasted up to 200 ns. Assimilating these peptides’ complex properties of dynamics and motion is another application of dynamics simulation. Additionally, RMSD was seen to comprehend confirmational fixity. Besides the analysis of the docked complex solvent, the accessible surface area was observed. Further, gyration profiles radius was investigated to recognize the variation in protein mobility. MD simulation resulting in all the peptide complex conditions was firm and inflexible while binding with the SARS-CoV-2 main protease.
The ligand-free complex (apo), co-crystalized ligand (control-1), and peptide-bound main protease (control-2) were included in the dynamic simulation study to compare the motion and dynamics properties of the four peptide complexes. The root mean square deviation of the C-alpha atoms from the seven complexes was found in stable condition in Figure 5. The average RMSD descriptors from seven complexes: apo, control-1, control-2, P14, P39, P41, and P74 were 1.17, 1.35, 1.479, 1.53, 1.45, 1.75, and 1.74 Å, respectively (Figure 5A). The P41 and P74 peptide complexes were initially stable from 0 to 90 ns, and a higher RMSD trends were observed for both complexes. But large deviation was not seen for both complexes, but they were in the below range of 2.5 Å. The control and apo complexes along with P14 and P39 complexes exhibit lower RMSD trend across the whole simulation time. This lower RMSD trend among the all seven complexes indicated the conformational stability of the docked and control complexes. Therefore, upon peptide binding with the target protein of SARS-CoV-2, lower degree of conformational variation was observed as all of the complexes were found rigid in dynamic simulation (Nainu et al., 2020; Rakib et al., 2020a; Rakib et al., 2020b; Rakib et al., 2020c; Tallei et al., 2020; Harapan et al., 2021; Mahmud et al., 2021a).
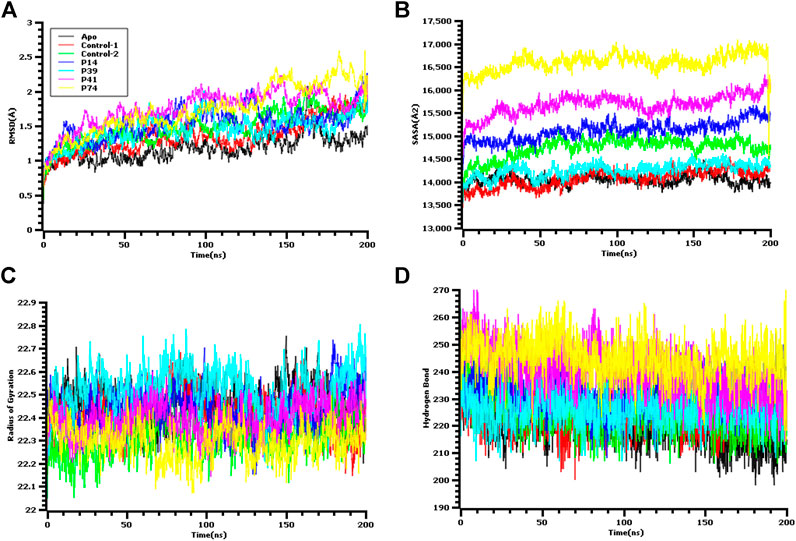
FIGURE 5. Molecular dynamics simulation study of the peptide and main protease enzyme. (A) Root mean square deviation of the C-alpha atom, (B) the solvent accessible surface area of the protein volume to understand the change in protein volume, (C) the radius of gyration, and (D) the hydrogen bond of the protein complex to evaluate their stability in simulation.
The solvent accessible surface area of the docked complexes was also analyzed. These simulation descriptors correlate with the surface volume of the complexes where higher SASA profile indicates the expansion in the surface area. The P39 complex had similar SASA trend as the peptide size was comparatively lower than other peptides (Figure 5B). So, this complex had similar surface area like control and apo. Although higher SASA trend were observed for rest of the peptides, but deviations in SASA value were absent for these complexes, thus these peptide complex had strict surface area across the simulation complex. The average SASA profile for the six complexes: apo, control-1, control-2, P14, P39, P41, and P41 were as 14048.28, 14075.69, 14738, 15115.54, 14261.35, 15679.8, and 16117.22 Å2, respectively.
The radius of gyration profile indicated the change in the protein mobility across the simulation time. The P74 peptide had lower Rg value than the control and other peptide molecules. After 60 ns, this complex lowered its Rg profile more and higher again after 100 ns (Figure 5C). The lower radius of gyration profile of this complex indicates the compacted nature of the protein. On the other hand, the P39 complex, apo, and control complex had higher deviation which correlates with lability of the complex and also possibility of the folding or unfolding nature of the complexes. Moreover, the hydrogen bond of the systems can determine the stable nature of the complexes. The P74 and P41 peptides had more hydrogen bonds than the control and apo complexes. The other two peptides, P14 and P39, had similar hydrogen pattern with the ligand-free complexes and control (Figure 5D). Although number of hydrogen bonds were different for all seven complexes, but they did not change the stable nature as lower flexibility were observed for the peptide complexes.
The root mean square fluctuation or RMSF of the amino acid residues from main protease enzyme was also analyzed (Figure 6). The complex had lower RMSF value except Ser1 (helix), Ala7 (helix), Val13 (helix), Gln19 (helix), Leu32 (helix), Asp33 (helix), Pro39 (helix), Arg40 (helix), Pro96 (beta turn), Tyr154 (beta turn) Glu166, Arg222, (beta turn), Gly302, (beta turn), Val303, (beta turn), Thr304, (beta turn), Phe305, (beta turn), and Gln306 (beta turn) residues. The last amino acid segment from beta turn domain and first segment from helical region of this protein exhibit more flexibility. Overall, all other complex had lower RMSF profile than the other complex by denoting more rigidity and structural stability of the amino acid residues from main protease.
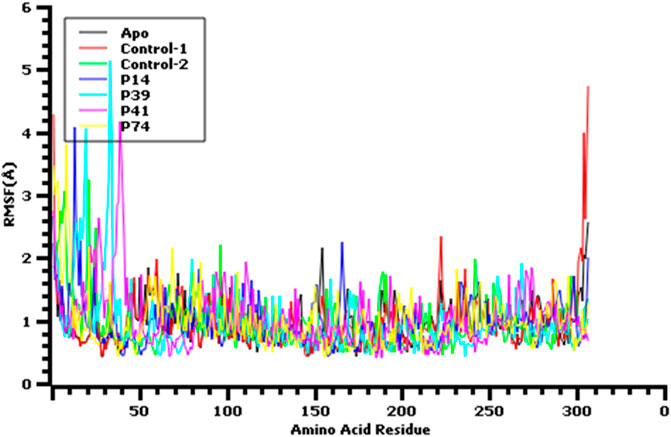
FIGURE 6. Root mean square fluctuation of the amino acid residue of the protein complex to understand their fluctuations across the residues.
SAR Analysis
The physiochemical properties of the best 15 peptides molecules were tabulated in Supplementary Table S2. The structural variation among the best 15 peptide molecules was assessed through clustering behavior. Figure 7 depicted that the best four peptides P14, P39, P41, and P74 and their clustering pattern were replicated the energy score plot of their complexes with main protease from SARS-CoV-2 but that P14 is close to P41 (Figure 7). Besides theoretical pl, total number of negatively charged residues (Asp + Glu) and total number of atoms and molecular weight residues play a significant role in the clustering pattern of the test peptides as negatively charged and total number of atoms residues are heavily loaded onto PC1 and the theoretical pl residue onto PC2, which altogether explains 96.33% structural variance.
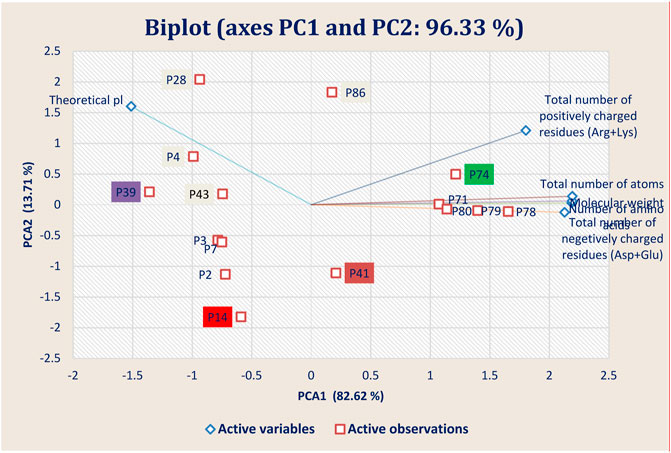
FIGURE 7. Principal component analysis (PCA) showing the Biplot of top 15 high-binding affinity peptides clustered based on four peptide properties.
Theoretical pl and total number of positively charged residues samples are clustered in the first and second quadrants, whereas the negatively charged residues samples were grouped in the fourth quadrants of the observation and biplots. This grouping accounted for 96.33% of the total variation present in the data set. Based on the sample ordination in principal component (PC1), total number of positively charged residues samples are located at positive values or close to zero compared to the theoretical pl samples, indicating a difference in the abundances of the compounds generated in both types of peptides.
Conclusion
Modeling and designing antiviral peptides by targeting viral protein from SARS-CoV-2 can be promising, unique techniques to tackle SARS-CoV-2 treatment systems. The comparative modeling of screened peptide molecules allows us to evaluate the best peptide molecules’ binding energy. The combinatorial docking approaches were further assessed to find the vital active points of interactions where most of the binding residues were found in the binding sites. This docking approach had favorable results in a dynamics simulation study where every peptide was in a rigid state, and flexible nature was not found. Furthermore, these experiments, along with future in vivo and in vitro experiments, can lead to the rational and rigorous development of peptide-based inhibitors by targeting the viral protein of SARS-CoV-2.
Data Availability Statement
The original contributions presented in the study are included in the article/Supplementary Material, further inquiries can be directed to the corresponding authors.
Author Contributions
Study concept and design: SM, GP, SB, SA, MM, MH, MSS, AH, MP, and MAS. Acquisition of data: SM, GP, SB, SA, MM, MH, MSS, AH, MP, and FE. Analyses and interpretation of data: SM, SB, SA, MM, MH, MSS, AH, and MP. Drafting the manuscript: SM, GP, SB, SA, MM, MH, MSS, AH, MP, and TE. Critical revision of the manuscript for important intellectual content: KC, BC, AA, TE, and MAS. Technical or material support: AA, TE, and MAS. Study supervision: AA, TE, and MAS.
Funding
This work is conducted with the individual funding of all authors.
Conflict of Interest
The authors declare that the research was conducted in the absence of any commercial or financial relationships that could be construed as a potential conflict of interest.
Acknowledgments
The authors would like to thank Cambridge Proofreading® and Editing LLC. (https://proofreading.org/) for editing a draft of this manuscript.
Supplementary Material
The Supplementary Material for this article can be found online at: https://www.frontiersin.org/articles/10.3389/fmolb.2021.628585/full#supplementary-material
References
Acuti Martellucci, C., Flacco, M. E., Cappadona, R., Bravi, F., Mantovani, L., and Manzoli, L. (2020). SARS-CoV-2 Pandemic: An Overview. Adv. Biol. Regul. 77, 100736. doi:10.1016/j.jbior.2020.100736
Anand, K., Palm, G. J., Mesters, J. R., Siddell, S. G., Ziebuhr, J., and Hilgenfeld, R. (2002). Structure of Coronavirus Main Proteinase Reveals Combination of a Chymotrypsin Fold with an Extra Alpha-Helical Domain. EMBO J. 21, 3213–3224. doi:10.1093/emboj/cdf327
Bappy, S. S., Sultana, S., Adhikari, J., Mahmud, S., Khan, M. A., Kibria, K. M. K., et al. (2020). Extensive Immunoinformatics Study for the Prediction of Novel Peptide-Based Epitope Vaccine with Docking Confirmation against Envelope Protein of Chikungunya Virus: A Computational Biology Approach. J. Biomol. Struct. Dyn. 39, 1139–1154. doi:10.1080/07391102.2020.1726815
Berman, H. M., Westbrook, J., Feng, Z., Gilliland, G., Bhat, T. N., Weissig, H., et al. (2000). The Protein Data Bank. Nucl. Acids Res. 28, 235–242. doi:10.1093/nar/28.1.235
Binkowski, T. A., Naghibzadeh, S., and Liang, J. (2003). CASTp: Computed Atlas of Surface Topography of Proteins. Nucleic Acids Res. 31, 3352–3355. doi:10.1093/nar/gkg512
Ceylan, Z. (2020). Estimation of COVID-19 Prevalence in Italy, Spain, and France. Sci. Total Environ. 729, 138817. doi:10.1016/j.scitotenv.2020.138817
Chan, K. K., Dorosky, D., Sharma, P., Abbasi, S. A., Dye, J. M., Kranz, D. M., et al. (2020). Engineering Human ACE2 to Optimize Binding to the Spike Protein of SARS Coronavirus 2. Science 369, 1261–1265. doi:10.1126/SCIENCE.ABC0870
Chen, Y., Liu, Q., and Guo, D. (2020). Emerging Coronaviruses: Genome Structure, Replication, and Pathogenesis. J. Med. Virol. 92, 418–423. doi:10.1002/jmv.25681
Chowdhury, K. H., Chowdhury, M. R., Mahmud, S., Tareq, A. M., Hanif, N. B., Banu, N., et al. (2021). Drug Repurposing Approach against Novel Coronavirus Disease (COVID-19) Through Virtual Screening Targeting SARS-CoV-2 Main Protease. Biology 10, 2–14. doi:10.3390/biology10010002
Corbett, K. S., Flynn, B., Foulds, K. E., Francica, J. R., Boyoglu-Barnum, S., Werner, A. P., et al. (2020). Evaluation of the mRNA-1273 Vaccine Against SARS-CoV-2 in Nonhuman Primates. N. Engl. J. Med. 383, 1544–1555. doi:10.1056/NEJMoa2024671
Dagotto, G., Yu, J., and Barouch, D. H. (2020). Approaches and Challenges in SARS-CoV-2 Vaccine Development. Cell Host & Microbe 28, 364–370. doi:10.1016/j.chom.2020.08.002
Dai, W., Zhang, B., Jiang, X.-M., Su, H., Li, J., Zhao, Y., et al. (2020). Structure-Based Design of Antiviral Drug Candidates Targeting the SARS-CoV-2 Main Protease. Science 368, 1331–1335. doi:10.1126/science.abb4489
Dehelean, C. A., Lazureanu, V., Coricovac, D., Mioc, M., Oancea, R., Marcovici, I., et al. (2020). SARS-CoV-2: Repurposed Drugs and Novel Therapeutic Approaches-Insights into Chemical Structure-Biological Activity and Toxicological Screening. Jcm 9, 2084. doi:10.3390/jcm9072084
Folegatti, P. M., Ewer, K. J., Aley, P. K., Angus, B., Becker, S., Belij-Rammerstorfer, S., et al. (2020). Safety and Immunogenicity of the ChAdOx1 nCoV-19 Vaccine against SARS-CoV-2: a Preliminary Report of a Phase 1/2, Single-Blind, Randomised Controlled Trial. Lancet 396, 467–478. doi:10.1016/S0140-6736(20)31604-4
Garg, V. K., Avashthi, H., Tiwari, A., Jain, P. A., Ramkete, P. W., Kayastha, A. M., et al. (2016). MFPPI‐Multi FASTA ProtParam Interface. Bioinformation 12, 74–77. doi:10.6026/97320630012074
Ge, H., Wang, X., Yuan, X., Xiao, G., Wang, C., Deng, T., et al. (2020). The Epidemiology and Clinical Information About COVID-19. Eur. J. Clin. Microbiol. Infect. Dis. 39, 1011–1019. doi:10.1007/s10096-020-03874-z
Harapan, H., Ryan, M., Yohan, B., Abidin, R. S., Nainu, F., Rakib, A., et al. (2021). Covid‐19 and Dengue: Double Punches for Dengue‐Endemic Countries in Asia. Rev. Med. Virol. 31, e2161. doi:10.1002/rmv.2161
Hengphasatporn, K., Garon, A., Wolschann, P., Langer, T., Yasuteru, S., Huynh, T. N. T., et al. (2020). Multiple Virtual Screening Strategies for the Discovery of Novel Compounds Active against Dengue Virus: A Hit Identification Study. Sci. Pharm. 88, 2. doi:10.3390/scipharm88010002
Islam, M. S., Mahmud, S., Sultana, R., and Dong, W. (2020). Identification and In Silico Molecular Modelling Study of Newly Isolated Bacillus Subtilis SI-18 Strain against S9 Protein of Rhizoctonia solani. Arabian J. Chem. 13, 8600–8612. doi:10.1016/j.arabjc.2020.09.044
Jackson, L. A., Anderson, E. J., Rouphael, N. G., Roberts, P. C., Makhene, M., Coler, R. N., et al. (2020). An mRNA Vaccine against SARS-CoV-2 - Preliminary Report. N. Engl. J. Med. 383, 1920–1931. doi:10.1056/NEJMoa2022483
Joshi, R. S., Jagdale, S. S., Bansode, S. B., Shankar, S. S., Tellis, M. B., Pandya, V. K., et al. (2020). Discovery of Potential Multi-Target-Directed Ligands by Targeting Host-specific SARS-CoV-2 Structurally Conserved Main Protease. J. Biomol. Struct. Dyn. 1, 1–16. doi:10.1080/07391102.2020.1760137
Khan, M. A., Mahmud, S., Alam, A. S. M. R. U., Rahman, M. E., Ahmed, F., and Rahmatullah, M. (2020). Comparative Molecular Investigation of the Potential Inhibitors against SARS-CoV-2 Main Protease: a Molecular Docking Study. J. Biomol. Struct. Dyn. 1, 1–7. doi:10.1080/07391102.2020.1796813
Kozakov, D., Hall, D. R., Xia, B., Porter, K. A., Padhorny, D., Yueh, C., et al. (2017). The ClusPro Web Server for Protein-Protein Docking. Nat. Protoc. 12, 255–278. doi:10.1038/nprot.2016.169
Krieger, E., Nielsen, J. E., Spronk, C. A. E. M., and Vriend, G. (2006). Fast Empirical pKa Prediction by Ewald Summation. J. Mol. Graphics Model. 25, 481–486. doi:10.1016/j.jmgm.2006.02.009
Krieger, E., and Vriend, G. (2015). New Ways to Boost Molecular Dynamics Simulations. J. Comput. Chem. 36, 996–1007. doi:10.1002/jcc.23899
Krieger, G., Vriend, E., and Spronk, C. (2013). YASARA–Yet Another Scientific Artificial Reality Application. Available at: http://yasara.org/ (Accessed August 18, 2004).
Lan, J., Ge, J., Yu, J., Shan, S., Zhou, H., Fan, S., et al. (2020). Structure of the SARS-CoV-2 Spike Receptor-Binding Domain Bound to the ACE2 Receptor. Nature 581, 215–220. doi:10.1038/s41586-020-2180-5
Land, H., and Humble, M. S. (2018). YASARA: A Tool to Obtain Structural Guidance in Biocatalytic Investigations. Methods Mol. Biol. 1685, 43–67. doi:10.1007/978-1-4939-7366-8_4
Li, X., Zai, J., Zhao, Q., Nie, Q., Li, Y., Foley, B. T., et al. (2020). Evolutionary History, Potential Intermediate Animal Host, and Cross‐species Analyses of SARS‐CoV‐2. J. Med. Virol. 92, 602–611. doi:10.1002/jmv.25731
Liu, X., Shi, D., Zhou, S., Liu, H., Liu, H., and Yao, X. (2018). Molecular Dynamics Simulations and Novel Drug Discovery. Expert Opin. Drug Discov. 13, 23–37. doi:10.1080/17460441.2018.1403419
Luo, H., Tang, Q.-l., Shang, Y.-x., Liang, S.-b., Yang, M., Robinson, N., et al. (2020). Can Chinese Medicine Be Used for Prevention of Corona Virus Disease 2019 (COVID-19)? A Review of Historical Classics, Research Evidence and Current Prevention Programs. Chin. J. Integr. Med. 26, 243–250. doi:10.1007/s11655-020-3192-6
Mahmud, S., Parves, M. R., Riza, Y. M., Sujon, K. M., Ray, S., Tithi, F. A., et al. (2020a). Exploring the Potent Inhibitors and Binding Modes of Phospholipase A2 through In Silico Investigation. J. Biomol. Struct. Dyn. 38, 4221–4231. doi:10.1080/07391102.2019.1680440
Mahmud, S., Uddin, M. A. R., Zaman, M., Sujon, K. M., Rahman, M. E., Shehab, M. N., et al. (2020b). Molecular Docking and Dynamics Study of Natural Compound for Potential Inhibition of Main Protease of SARS-CoV-2. J. Biomol. Struct. Dyn. 2020, 1–9. doi:10.1080/07391102.2020.1796808
Mahmud, S., Paul, G. K., Afroze, M., Islam, S., Gupt, S. B. R., Razu, M. H., et al. (2021a). Efficacy of Phytochemicals Derived from Avicennia officinalis for the Management of COVID-19: A Combined In Silico and Biochemical Study. Molecules 26, 2210. doi:10.3390/molecules26082210
Mahmud, S., Uddin, M. A. R., Paul, G. K., Shimu, M. S. S., Islam, S., Rahman, E., et al. (2021b). Virtual Screening and Molecular Dynamics Simulation Study of Plant-Derived Compounds to Identify Potential Inhibitors of Main Protease from SARS-CoV-2. Brief. Bioinform. 22, 1402–1414. doi:10.1093/bib/bbaa428
Maia, E. H. B., Assis, L. C., de Oliveira, T. A., da Silva, A. M., and Taranto, A. G. (2020). Structure-Based Virtual Screening: From Classical to Artificial Intelligence. Front. Chem. 8, 343. doi:10.3389/fchem.2020.00343
Mashiach, E., Schneidman-Duhovny, D., Andrusier, N., Nussinov, R., and Wolfson, H. J. (2008). FireDock: a Web Server for Fast Interaction Refinement in Molecular Docking. Nucleic Acids Res. 36, W229–W232. doi:10.1093/nar/gkn186
Maupetit, J., Derreumaux, P., and Tuffery, P. (2009). PEP-FOLD: An Online Resource for De Novo Peptide Structure Prediction. Nucleic Acids Res. 37, W498–W503. doi:10.1093/nar/gkp323
Mercado, N. B., Zahn, R., Wegmann, F., Loos, C., Chandrashekar, A., Yu, J., et al. (2020). Single-shot Ad26 Vaccine Protects against SARS-CoV-2 in Rhesus Macaques. Nature 586, 583–588. doi:10.1038/s41586-020-2607-z
Nainu, F., Abidin, R. S., Bahar, M. A., Frediansyah, A., Emran, T. B., Rabaan, A. A., et al. (2020). SARS-CoV-2 Reinfection and Implications for Vaccine Development. Hum. Vaccin. Immunother. 16, 3061–3073. doi:10.1080/21645515.2020.1830683
Nelde, A., Bilich, T., Heitmann, J. S., Maringer, Y., Salih, H. R., Roerden, M., et al. (2021). SARS-CoV-2-derived Peptides Define Heterologous and COVID-19-Induced T Cell Recognition. Nat. Immunol. 22, 74–85. doi:10.1038/s41590-020-00808-x
Okimoto, N., Futatsugi, N., Fuji, H., Suenaga, A., Morimoto, G., Yanai, R., et al. (2009). High-performance Drug Discovery: Computational Screening by Combining Docking and Molecular Dynamics Simulations. Plos Comput. Biol. 5, e1000528. doi:10.1371/journal.pcbi.1000528
Qureshi, A., Thakur, N., Tandon, H., and Kumar, M. (2014). AVPdb: A Database of Experimentally Validated Antiviral Peptides Targeting Medically Important Viruses. Nucl. Acids Res. 42, D1147–D1153. doi:10.1093/nar/gkt1191
Rakib, A., Nain, Z., Sami, S. A., Mahmud, S., Islam, A., Ahmed, S., et al. (2021). A Molecular Modelling Approach for Identifying Antiviral Selenium-Containing Heterocyclic Compounds that Inhibit the Main Protease of SARS-CoV-2: an In Silico Investigation. Brief. Bioinform. 22, 1476–1498. doi:10.1093/bib/bbab045
Rakib, A., Paul, A., Chy, M. N. U., Sami, S. A., Baral, S. K., Majumder, M., et al. (2020a). Biochemical and Computational Approach of Selected Phytocompounds from Tinospora Crispa in the Management of COVID-19. Molecules 25, 3936. doi:10.3390/molecules25173936
Rakib, A., Sami, S. A., Islam, M. A., Ahmed, S., Faiz, F. B., Khanam, B. H., et al. (2020b). Epitope-Based Immunoinformatics Approach on Nucleocapsid Protein of Severe Acute Respiratory Syndrome-Coronavirus-2. Molecules 25, 5088. doi:10.3390/molecules25215088
Rakib, A., Sami, S. A., Mimi, N. J., Chowdhury, M. M., Eva, T. A., Nainu, F., et al. (2020c). Immunoinformatics-guided Design of an Epitope-Based Vaccine against Severe Acute Respiratory Syndrome Coronavirus 2 Spike Glycoprotein. Comput. Biol. Med. 124, 103967. doi:10.1016/j.compbiomed.2020.103967
Schneidman-Duhovny, D., Inbar, Y., Nussinov, R., and Wolfson, H. J. (2005). PatchDock and SymmDock: Servers for Rigid and Symmetric Docking. Nucleic Acids Res. 33, W363–W367. doi:10.1093/nar/gki481
Shang, J., Wan, Y., Luo, C., Ye, G., Geng, Q., Auerbach, A., et al. (2020). Cell Entry Mechanisms of SARS-CoV-2. Proc. Natl. Acad. Sci. USA 117, 11727–11734. doi:10.1073/pnas.2003138117
Shereen, M. A., Khan, S., Kazmi, A., Bashir, N., and Siddique, R. (2020). COVID-19 Infection: Emergence, Transmission, and Characteristics of Human Coronaviruses. J. Adv. Res. 24, 91–98. doi:10.1016/j.jare.2020.03.005
Shoichet, B. K. (2006). Virtual Screening of Chemical Libraries Problems with Virtual Screening. Nature 432, 862–865. doi:10.1038/nature03197
Silva Andrade, B., Ghosh, P., Barh, D., Tiwari, S., José Santana Silva, R., Rodrigues de Assis Soares, W., et al. (2020). Computational Screening for Potential Drug Candidates against the SARS-CoV-2 Main Protease. F1000Res 9, 514. doi:10.12688/f1000research.23829.2
Singhal, T. (2020). A Review of Coronavirus Disease-2019 (COVID-19). Indian J. Pediatr. 87, 281–286. doi:10.1007/s12098-020-03263-6
Sousa, S. F., Cerqueira, N. M., Fernandes, P. A., and Ramos, M. J. (2010). Virtual Screening in Drug Design and Development. Comb. Chem. High Throughput Screen. 13, 442–453. doi:10.2174/138620710791293001
Suárez, D., and Díaz, N. (2020). SARS-CoV-2 Main Protease: A Molecular Dynamics Study. J. Chem. Inf. Model. 60, 5815–5831. doi:10.1021/acs.jcim.0c00575
Swargiary, A., Mahmud, S., and Saleh, M. A. (2020). Screening of Phytochemicals as Potent Inhibitor of 3-chymotrypsin and Papain-like Proteases of SARS-CoV2: an In Silico Approach to Combat COVID-19. J. Biomol. Struct. Dyn. 2020, 1–15. doi:10.1080/07391102.2020.1835729
Tallei, T. E., Tumilaar, S. G., Niode, N. J., Fatimawali, B. J., Kepel, R., et al. (2020). Potential of Plant Bioactive Compounds as SARS-CoV-2 Main Protease (Mpro) and Spike (S) Glycoprotein Inhibitors: A Molecular Docking Study. Scientifica 2020, 1–18. doi:10.1155/2020/6307457
Thévenet, P., Shen, Y., Maupetit, J., Guyon, F., Derreumaux, P., and Tufféry, P. (2012). PEP-FOLD: An Updated De Novo Structure Prediction Server for Both Linear and Disulfide Bonded Cyclic Peptides. Nucleic Acids Res. 40, W288–W293. doi:10.1093/nar/gks419
Uddin, M. Z., Paul, A., Rakib, A., Sami, S. A., Mahmud, S., Rana, M. S., et al. (2021). Chemical Profiles and Pharmacological Properties with In Silico Studies on Elatostema Papillosum Wedd. Molecules 26, 809. doi:10.3390/molecules26040809
Ullrich, S., and Nitsche, C. (2020). The SARS-CoV-2 Main Protease as Drug Target. Bioorg. Med. Chem. Lett. 30, 127377. doi:10.1016/j.bmcl.2020.127377
van Doremalen, N., Lambe, T., Spencer, A., Belij-Rammerstorfer, S., Purushotham, J. N., Port, J. R., et al. (2020). ChAdOx1 nCoV-19 Vaccine Prevents SARS-CoV-2 Pneumonia in Rhesus Macaques. Nature 586, 578–582. doi:10.1038/s41586-020-2608-y
Velavan, T. P., and Meyer, C. G. (2020). The COVID‐19 Epidemic. Trop. Med. Int. Health 25, 278–280. doi:10.1111/tmi.13383
Wang, C., Horby, P. W., Hayden, F. G., and Gao, G. F. (2020a). A Novel Coronavirus Outbreak of Global Health Concern. The Lancet 395, 470–473. doi:10.1016/S0140-6736(20)30185-9
Wang, H., Li, X., Li, T., Zhang, S., Wang, L., Wu, X., et al. (2020b). The Genetic Sequence, Origin, and Diagnosis of SARS-CoV-2. Eur. J. Clin. Microbiol. Infect. Dis. 39, 1629–1635. doi:10.1007/s10096-020-03899-4
Wang, H., Zhang, Y., Huang, B., Deng, W., Quan, Y., Wang, W., et al. (2020c). Development of an Inactivated Vaccine Candidate, BBIBP-CorV, with Potent Protection against SARS-CoV-2. Cell 182, 713–721. doi:10.1016/j.cell.2020.06.008
WHO (2020). Transmission of SARS-CoV-2: Implications for Infection Prevention Precautions: Scientific Brief, 09 July 2020. Geneva, Switzerland: World Health Organization.
Wu, A., Peng, Y., Huang, B., Ding, X., Wang, X., Niu, P., et al. (2020a). Genome Composition and Divergence of the Novel Coronavirus (2019-nCoV) Originating in China. Cell Host & Microbe 27, 325–328. doi:10.1016/j.chom.2020.02.001
Wu, F., Zhao, S., Yu, B., Chen, Y.-M., Wang, W., Song, Z.-G., et al. (2020b). A New Coronavirus Associated with Human Respiratory Disease in China. Nature 579, 265–269. doi:10.1038/s41586-020-2008-3
Yang, H., Yang, M., Ding, Y., Liu, Y., Lou, Z., Zhou, Z., et al. (2003). The Crystal Structures of Severe Acute Respiratory Syndrome Virus Main Protease and its Complex with an Inhibitor. Proc. Natl. Acad. Sci. 100, 13190–13195. doi:10.1073/pnas.1835675100
Yang, J., Petitjean, S. J. L., Koehler, M., Zhang, Q., Dumitru, A. C., Chen, W., et al. (2020). Molecular Interaction and Inhibition of SARS-CoV-2 Binding to the ACE2 Receptor. Nat. Commun. 11, 4541. doi:10.1038/s41467-020-18319-6
Yu, J., Tostanoski, L. H., Peter, L., Mercado, N. B., McMahan, K., Mahrokhian, S. H., et al. (2020). DNA Vaccine Protection against SARS-CoV-2 in Rhesus Macaques. Science 369, 806–811. doi:10.1126/science.abc6284
Zhang, L., Lin, D., Sun, X., Curth, U., Drosten, C., Sauerhering, L., et al. (2020a). Crystal Structure of SARS-CoV-2 Main Protease Provides a Basis for Design of Improved α-ketoamide Inhibitors. Science 368, 409–412. doi:10.1126/science.abb3405
Zhang, Y., Geng, X., Tan, Y., Li, Q., Xu, C., Xu, J., et al. (2020b). New Understanding of the Damage of SARS-CoV-2 Infection outside the Respiratory System. Biomed. Pharmacother. 127, 110195. doi:10.1016/j.biopha.2020.110195
Zhao, J., Cui, W., and Tian, B.-p. (2020). The Potential Intermediate Hosts for SARS-CoV-2. Front. Microbiol. 11, 1–11. doi:10.3389/fmicb.2020.580137
Zheng, J. (2020). SARS-CoV-2: An Emerging Coronavirus that Causes a Global Threat. Int. J. Biol. Sci. 16, 1678–1685. doi:10.7150/ijbs.45053
Zhong, N., Zheng, B., Li, Y., Poon, L., Xie, Z., Chan, K., et al. (2003). Epidemiology and Cause of Severe Acute Respiratory Syndrome (SARS) in Guangdong, People's Republic of China, in February, 2003. The Lancet 362, 1353–1358. doi:10.1016/S0140-6736(03)14630-2
Zhou, P., Yang, X.-L., Wang, X.-G., Wang, B., Zhang, L., Zhang, W., et al. (2020). A Pneumonia Outbreak Associated with a New Coronavirus of Probable Bat Origin. Nature 579, 270–273. doi:10.1038/s41586-020-2012-7
Zhu, F.-C., Guan, X.-H., Li, Y.-H., Huang, J.-Y., Jiang, T., Hou, L.-H., et al. (2020). Immunogenicity and Safety of a Recombinant Adenovirus Type-5-Vectored COVID-19 Vaccine in Healthy Adults Aged 18 Years or Older: a Randomised, Double-Blind, Placebo-Controlled, Phase 2 Trial. The Lancet 396, 479–488. doi:10.1016/s0140-6736(20)31605-6
Keywords: SARS-CoV-2, COVID-19, M Pro, peptides, molecular dynamics, in silico
Citation: Mahmud S, Paul GK, Biswas S, Afrose S, Mita MA, Hasan MR, Shimu MSS, Hossain A, Promi MM, Ema FK, Chidambaram K, Chandrasekaran B, Alqahtani AM, Emran TB and Saleh MA (2021) Prospective Role of Peptide-Based Antiviral Therapy Against the Main Protease of SARS-CoV-2. Front. Mol. Biosci. 8:628585. doi: 10.3389/fmolb.2021.628585
Received: 12 November 2020; Accepted: 22 April 2021;
Published: 10 May 2021.
Edited by:
Megha Agrawal, University of Illinois at Chicago, United StatesReviewed by:
Hem Chandra Jha, Indian Institute of Technology Indore, IndiaSaroj Kumari, Nation institute of immunology, India
Copyright © 2021 Mahmud, Paul, Biswas, Afrose, Mita, Hasan, Shimu, Hossain, Promi, Ema, Chidambaram, Chandrasekaran, Alqahtani, Emran and Saleh. This is an open-access article distributed under the terms of the Creative Commons Attribution License (CC BY). The use, distribution or reproduction in other forums is permitted, provided the original author(s) and the copyright owner(s) are credited and that the original publication in this journal is cited, in accordance with accepted academic practice. No use, distribution or reproduction is permitted which does not comply with these terms.
*Correspondence: Ali M. Alqahtani, YW1zZnJAa2t1LmVkdS5zYQ==; Talha Bin Emran, dGFsaGFibWJAYmdjdHViLmFjLmJk; Md. Abu Saleh, c2FsZWhAcnUuYWMuYmQ=
†These authors have contributed equally to this work.