- 1The Second Clinical Medical College, Lanzhou University, Lanzhou, China
- 2Department of Orthopedics, Lanzhou University Second Hospital, Lanzhou, China
- 3Lintao County Traditional Chinese Medicine Hospital of Gansu Province, Lintao, China
- 4The Fourth People’s Hospital of Qinghai Province, Xining, China
- 5Department of Orthopaedics, Xining First People’s Hospital, Xining, China
- 6Affiliated Hospital of Qinghai University, Xining, China
- 7Affiliated Cancer Hospital of Qinghai University, Xining, China
- 8Breast Disease Diagnosis and Treatment Center, Affiliated Hospital of Qinghai University & Affiliated Cancer Hospital of Qinghai University, Xining, China
Background: Osteosarcoma is a frequent bone malignancy in children and young adults. Despite the availability of some prognostic biomarkers, most of them fail to accurately predict prognosis in osteosarcoma patients. In this study, we used bioinformatics tools and machine learning algorithms to establish an autophagy-related long non-coding RNA (lncRNA) signature to predict the prognosis of osteosarcoma patients.
Methods: We obtained expression and clinical data from osteosarcoma patients in the Therapeutically Applicable Research to Generate Effective Treatments (TARGET) and Gene Expression Omnibus (GEO) databases. We acquired an autophagy gene list from the Human Autophagy Database (HADb) and identified autophagy-related lncRNAs by co-expression analyses. Gene Ontology (GO) and Kyoto Encyclopedia of Genes and Genomes (KEGG) pathway enrichment analyses of the autophagy-related lncRNAs were conducted. Univariate and multivariate Cox regression analyses were performed to assess the prognostic value of the autophagy-related lncRNA signature and validate the relationship between the signature and osteosarcoma patient survival in an independent cohort. We also investigated the relationship between the signature and immune cell infiltration.
Results: We initially identified 69 autophagy-related lncRNAs, 13 of which were significant predictors of overall survival in osteosarcoma patients. Kaplan-Meier analyses revealed that the 13 autophagy-related lncRNAs could stratify patients based on their outcomes. Receiver operating characteristic curve analyses confirmed the superior prognostic value of the lncRNA signature compared to clinically used prognostic biomarkers. Importantly, the autophagy-related lncRNA signature predicted patient prognosis independently of clinicopathological characteristics. Furthermore, we found that the expression levels of the autophagy-related lncRNA signature were significantly associated with the infiltration levels of different immune cell subsets, including T cells, NK cells, and dendritic cells.
Conclusion: The autophagy-related lncRNA signature established here is an independent and robust predictor of osteosarcoma patient survival. Our findings also suggest that the expression of these 13 autophagy-related lncRNAs may promote osteosarcoma progression by regulating immune cell infiltration in the tumor microenvironment.
Introduction
Osteosarcoma is a common bone malignancy in children and young adults (Wedekind et al., 2018; Heymann et al., 2019). It is characterized by high metastasis and recurrence rates, with approximately 10–20% of patients with metastatic osteosarcoma experiencing pain and swelling (Liu et al., 2018). Osteosarcoma patients are typically treated with chemotherapy, radiotherapy, and surgery. Although recent clinical studies have shown that adjuvant chemotherapy can improve the survival rate of such patients, the prognosis remains poor (Tian et al., 2019; Wang J. et al., 2019). Therefore, the development of improved management and risk stratification methods, as well as the identification of robust prognostic biomarkers, are of high clinical importance.
Autophagy is a highly conserved physiological process involving the degradation of damaged proteins and cellular organelles through the formation of autophagosomes and their subsequent fusion with lysosomes (Nadjmi et al., 1987; Ravanan et al., 2017; Levine and Kroemer, 2019; Wong et al., 2020). Mounting evidence indicates that autophagy is a key determinant of cancer progression by regulating cell growth, metastasis, and response to chemotherapy (Folkerts et al., 2019). However, it has also become evident that autophagy is a double-edged sword. In the early stages of cancer, autophagy promotes the degradation of damaged proteins or organelles, thereby preventing cell damage and chromosomal instability and inhibiting cancer progression. On the other hand, once the cancer is formed, autophagy facilitates tumor cell survival under various environmental stresses, such as nutrient deprivation and hypoxia, promoting tumor progression and therapeutic resistance (Levy et al., 2017; Amaravadi et al., 2019; Cuomo et al., 2019). The role of autophagy in osteosarcoma has previously been reported. Notably, in mouse osteosarcoma models, silencing of the autophagy-promoting gene BECN1 has been shown to enhance cancer cell metastasis (Zhang et al., 2018). Autophagy modulation in the human osteosarcoma cell line MG-63 significantly enhances cell survival and chemoresistance to cisplatin (Kim et al., 2017). Recent studies have shown that autophagy is closely related to immune cell infiltration in the tumor microenvironment (Dehne et al., 2017). Chen D.P. et al. (2018) showed that autophagy induction in liver cancer cells promotes the epithelial–mesenchymal transition (EMT) and metastasis by activating the NF-κB/SNAI1 signaling pathway. Nevertheless, the role of autophagy in osteosarcoma cell metastasis is less clear.
Long non-coding RNA (lncRNA) is a novel family of non-coding RNAs that are more than 200 nucleotides long (Zhao G.S. et al., 2018; Chi et al., 2019). They regulate gene expression at the transcriptional and post-transcriptional levels and play a protumorigenic or tumor suppressor role in various human cancers, including breast, gastric, colorectal, and liver cancers (Choi et al., 2019; Feng et al., 2019; Ni et al., 2019; Song et al., 2019). Increasing evidence shows that the role of lncRNAs in cancer pathogenesis is partly mediated by the regulation of various autophagy-related proteins, in addition to proteins involved in cell proliferation, apoptosis, and metastasis (Chi et al., 2019; Jiang N. et al., 2019; Zheng et al., 2019). For instance, the lncRNA XIST inhibits autophagy in osteosarcoma cells by acting as a sponge for miR-375–3p, thereby regulating the AKT/mTOR signaling pathway (Sun et al., 2019). Similarly, the lncRNA Sox2OT-V7 regulates ULK1, ATG4A, and ATG5 through miR-142/miR-22 to modulate autophagy in osteosarcoma cells (Zhu et al., 2020). The lncRNA LINC00313 acts as a sponge for miR-342–3p, regulating FOSL2 levels and inhibiting autophagy in human osteosarcoma cells, ultimately suppressing osteosarcoma cell metastasis (Chen et al., 2020). LncRNA-ATB modulates autophagy in liver cancer cells by activating Yes-associated protein (YAP) and inducing ATG5 expression, associated with poor prognosis of liver cancer patients (Wang C.Z. et al., 2019). By regulating the expression levels of autophagy-related genes, lncRNAs regulate all stages of autophagy, including initiation, vesicle nucleation, autophagosome maturation, and autophagosome fusion (Pan et al., 2018; Tu et al., 2019). Therefore, autophagy-related lncRNAs may have potential prognostic and therapeutic value in osteosarcoma patients.
In this study, we used a bioinformatics approach to construct a co-expression network of autophagy-related lncRNA and identify an autophagy-related lncRNA signature based on machine learning algorithms (Figure 1). Furthermore, we analyzed the relationship between autophagy-related lncRNAs and immune cell infiltration in osteosarcoma. Our findings provide further insight into the role of autophagy-related lncRNAs in osteosarcoma and suggest that they are promising prognostic markers and therapeutic targets.
Materials and Methods
Data Acquisition, Processing, and Screening
Therapeutically Applicable Research to Generate Effective Treatments (TARGET)1 is an open database for childhood cancers. It seeks to use a comprehensive genomic approach to identify molecular changes in the occurrence and development of childhood cancers that are difficult to treat (Gao et al., 2020; Zhang et al., 2020). We obtained gene expression and clinical data of osteosarcoma patients from the TARGET database. After excluding samples lacking survival data, data from the remaining 82 osteosarcoma samples were further analyzed. The GSE39055 dataset was downloaded from the Gene Expression Omnibus (GEO) database (Barrett et al., 2013). It involved data from 37 unique diagnostic biopsy specimens analyzed on the GPL14951 platform (Illumina HumanHT-12 WG-DASL V4.0 R2 expression bead chip) (Kelly et al., 2013). We obtained an autophagy gene list from the Human Autophagy Database (HADb)2 and extracted the autophagy-related gene expression matrix using TARGET as the training set and GSE39055 as the validation set. After identifying mRNAs and lncRNAs in the gene expression matrix of the training set, we extracted the lncRNA expression matrix and calculated the Pearson coefficient of lncRNA gene expression and autophagy genes. LncRNAs with Pearson coefficient > ± 0.4 and P < 0.05 were considered autophagy-related lncRNAs.
Co-expression Analyses of Autophagy-Related lncRNAs, Gene Ontology (GO), and Kyoto Encyclopedia of Genes and Genomes (KEGG) Pathway Enrichment Analyses
To assess the potential role of autophagy-related lncRNAs in osteosarcoma development, we performed co-expression analyses using the mRNA and autophagy-related lncRNA expression matrixes. RNAs with Pearson coefficients > ± 0.4 and P < 0.05 were considered autophagy-related lncRNA target genes. These genes were subjected to GO and KEGG pathway enrichment analyses using the clusterProfiler package (Yu et al., 2012) and a significance threshold of adjusted P-value <0.05.
Construction and Validation of the Prognostic Model
Iterative lasso regression was used to identify high-frequency features (consensus genes). Consensus genes were incorporated into the Cox model until the area under the curve (AUC) in receiver operating characteristic (ROC) analyses reached a peak, at which point the model was considered optimal and contained the least features (Pungpapong et al., 2020). Next, we performed univariate Cox regression analyses using the obtained autophagy-related lncRNAs to identify autophagy-related lncRNAs associated with osteosarcoma prognosis (P < 0.05). Subsequently, we used the glmnet3 package to perform machine learning-based lasso Cox regression analyses using autophagy-related lncRNAs to identify the optimal prognostic signature. We identified the consensus genes (frequency > 50) after 500 lasso Cox regressions and performed multivariate Cox regression analyses using the consensus genes. After identifying the optimal prognostic signature, we calculated a prognostic score for each osteosarcoma sample and stratified patients into high and low risk according to the median value of the prognostic score. We used the R package to draw a risk factor chart to show the prognostic score of patients in the high- and low-risk groups. Diferential expressed genes (DEGs) were obtained by analyzing the gene expression matrix using the limma package (Li et al., 2013). The screening criteria were: | log2 fold change (log2FC) | > 1, adjust P < 0.05.
Comparison of the lncRNA Signature With Other Prognostic Biomarkers and Validation in an Independent Cohort
Many prognostic biomarkers have been reported for osteosarcoma. For example, the immune-related gene SP140 is a biomarker of poor prognosis in osteosarcoma (Hong et al., 2020), CCNE1 is a prognostic biomarker and potential therapeutic target (Wei et al., 2020), and SNHG3 is selectively expressed in osteosarcoma tissues (Chen et al., 2019). In addition, miR-221 expression is elevated in osteosarcoma, and its levels are associated with poor prognosis (Yang et al., 2015). The time-dependent receiver operating characteristic (ROC) curve was drawn to evaluate the predictive value of the prognostic gene signature for overall survival using the R package “survivalROC” (Heagerty et al., 2000). To compare the prognostic value of our signature with that of known biomarkers, we conducted comparative time-dependent ROC analyses. We also assessed the prognostic value of our lncRNA signature in an independent cohort (using the GSE39055 dataset).
Assessment of the lncRNA Signature as an Independent Prognostic Factor and Nomogram Construction
To further assess the potential use of the lncRNA signature as an independent prognostic factor, we performed Kaplan-Meier survival analyses on osteosarcoma patients grouped based on their clinicopathological characteristics. We also performed univariate and multivariate Cox regression analyses. To establish a quantitative method for predicting osteosarcoma patient prognosis, we constructed a nomogram integrating the lncRNA signature and different clinicopathological factors (Iasonos et al., 2008). Then we used a decision curve to compare the prognostic performance of our model with that of the traditional staging system.
Gene Set Enrichment Analyses (GSEA) and Gene Set Variation Analyses (GSVA) of High- and Low-Risk Osteosarcoma Patients
To compare the gene expression profiles between high- and low-risk osteosarcoma patients, we conducted GSEA and GSVA analyses. For GSEA, we used “c2.all.v7.1.symbols.gm,” “c5.all.v7.1.symbols.gmt,” and “h.all.v7.1.symbols.gmt” as reference gene sets. The analyses were conducted using GSEA 4.0.3 software and significance thresholds of nominal P < 0.05 and false discovery rate (FDR) <0.05. For GSVA, we used “h.all.v7.1.symbols.gmt” as the reference gene set. GSVA was conducted using the clusterProfiler and GSVA packages (Hänzelmann et al., 2013) with a significance threshold of adjusted P-value <0.05.
Immune Cell Infiltration Analyses
CIBERSORT uses a linear support vector regression to deconvolve the transcriptome expression matrix and estimate the composition and abundance of immune cells within a mixed cell population (Chen B. et al., 2018). To explore the relationship between the 13 prognostic autophagy-related lncRNAs and immune cell infiltration, we uploaded the gene expression matrix data of osteosarcoma patients to CIBERSORT and filtered for the samples with P < 0.05. The obtained matrix was analyzed using the ggplot2 package x to assess the composition of immune cell infiltrate and determine the differences in immune cell infiltration between osteosarcoma tissues and normal tissues. Subsequently, we performed univariate Cox regression analyses to identify immune cells associated with osteosarcoma patient prognosis (P < 0.05).
Correlation Analyses Between Autophagy-Related lncRNAs and Immune Cells
To further analyze the relationship between autophagy-related lncRNAs and immune cells, we performed Spearman correlation analyses using a monotonic equation to evaluate the correlation between the levels of autophagy-related lncRNAs and immune cells.
Results
Identification of Autophagy-Related lncRNAs
The gene expression profile data of OS patients and the corresponding clinical data were downloaded from the TARGET database, and excluded samples without survival data. A total of 82 OS samples were included in this study, and the clinicopathological characteristics of whom are summarized in Table 1. The dataset GSE39055 was downloaded from the GEO database, including 37 unique diagnostic biopsy specimens. We download the autophagy gene list from the HADb website, and extracted the autophagy-related gene expression matrix. TARGET was used as the training set, GSE39055 was used as the verification set, and we classified mRNA and lncRNA on the gene list of the expression matrix of the training set and extracted the lncRNA expression matrix. After background correction, data normalization, and removal of inter-batch differences, we identified a total of 222 autophagy-related genes.
Co-expression Analyses of Autophagy-Related lncRNAs and Enrichment Analyses of GO and KEGG Pathways
A total of 695 autophagy-related lncRNAs were identified by co-expression analyses. GO enrichment analyses of these autophagy-related lncRNAs revealed that significantly enriched biological processes included response to nutrient levels, gland development, sensory system development, and visual system development. Significantly enriched cell components included collagen-containing extracellular matrix, cell leading edge, and cell-substrate junction, while enriched molecular function terms included cell adhesion molecule binding, DNA-binding transcription activator activity, and protein serine/threonine kinase activity (Figure 2A). KEGG enrichment analyses uncovered an enrichment in pathways involved in responses to human papillomavirus infection, focal adhesion, fluid shear stress, and atherosclerosis (Figure 2B).
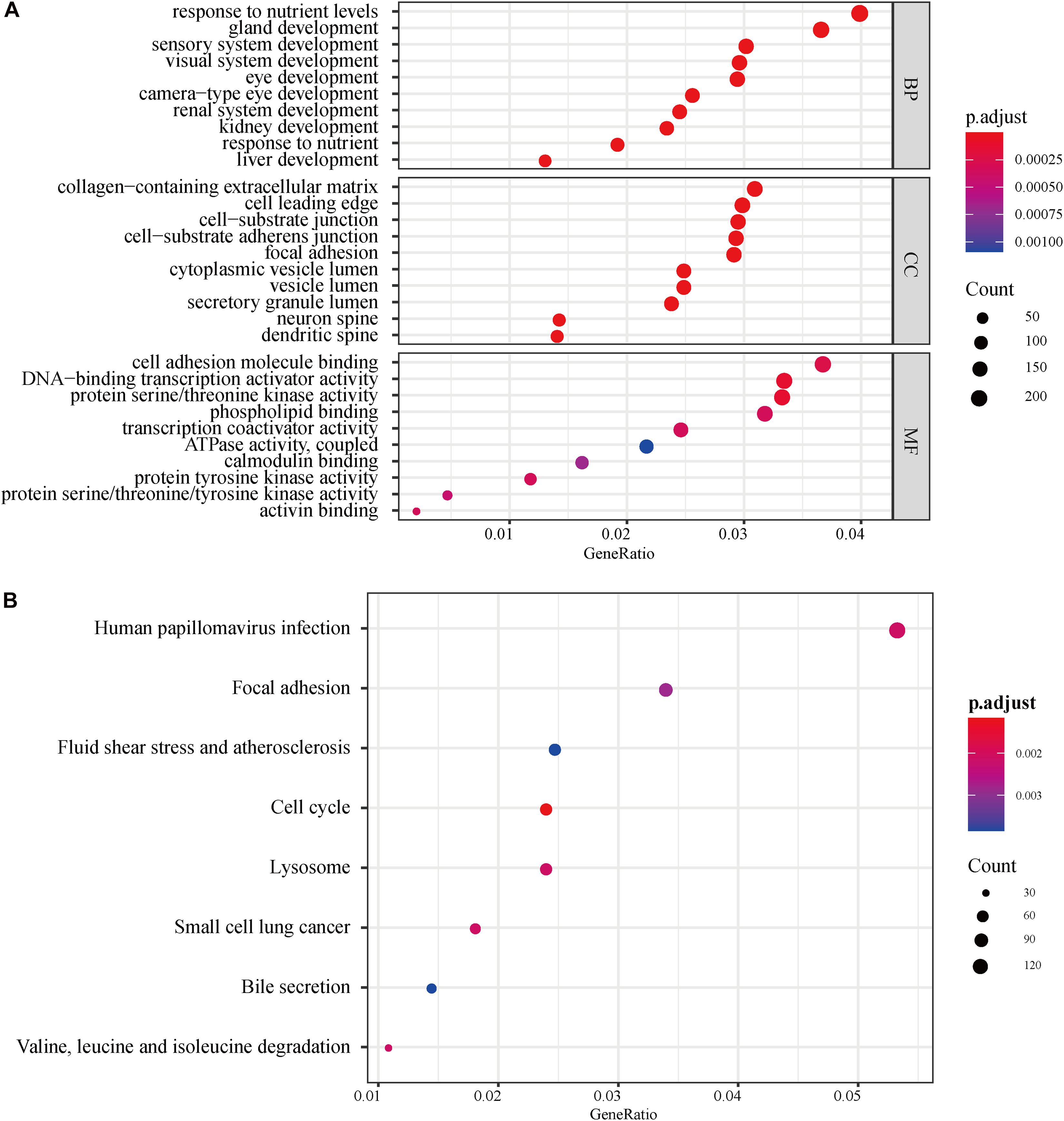
Figure 2. GO and KEGG pathway enrichment analyses of autophagy-related lncRNAs. (A) GO analysis results. The color of the dots represents the adjusted P-value: red, low; blue, high. The size of the dots represents the number of autophagy-related genes. (B) KEGG pathway enrichment analyses. The color of the dots represents the adjusted P-value, and the size of the dots represents the number of autophagy-related genes in the pathway. GO, gene ontology; KEGG, Kyoto Encyclopedia of Genes and Genomes; p.adjust, adjusted P-value; BP, biological process; CC, cellular component; MF, molecular function.
Construction and Validation of the lncRNA-Based Prognostic Model
To assess the prognostic value of autophagy-related lncRNAs in osteosarcoma, we performed univariate Cox regression analyses and identified 69 lncRNAs with significant prognostic value (Figure 3). To improve the accuracy of these results, we performed machine learning-based lasso Cox regression analyses using the 69 autophagy-related lncRNAs. We identified 13 lncRNAs (DSCR8, IGF2BP2-AS1, MIS18A-AS1, RUSC1-AS1, FGD5-AS1, LINC00269, LINC00307, LINC00355, LINC00482, LINC00544, LINC01545, SNHG17, and LINC00304) that were the most relevant to prognosis (Figure 4A and Table 2). ROC analyses yielded an AUC of 0.999, indicating the high prognostic value of these 13 lncRNAs (Figures 4A,B). Kaplan-Meier survival analyses revealed that the 5-year survival rate of patients in the high-risk group was significantly lower than that of patients in the low-risk group (P < 0.0001) (Figure 4C). Consistently, we found a significantly higher number of deaths in the high-risk group than in the low-risk group. LINC00482, SNHG17, IGF2BP2-AS1, RUSC1-AS1, LINC00544, LINC00269, and LINC00307 were expressed at higher levels in high-risk patients than in low-risk patients, whereas FDG5-AS1, MIS18A-AS1, LINC01545, LINC00304, LINC00355, and DSCR8 were expressed at lower levels (Figure 5).
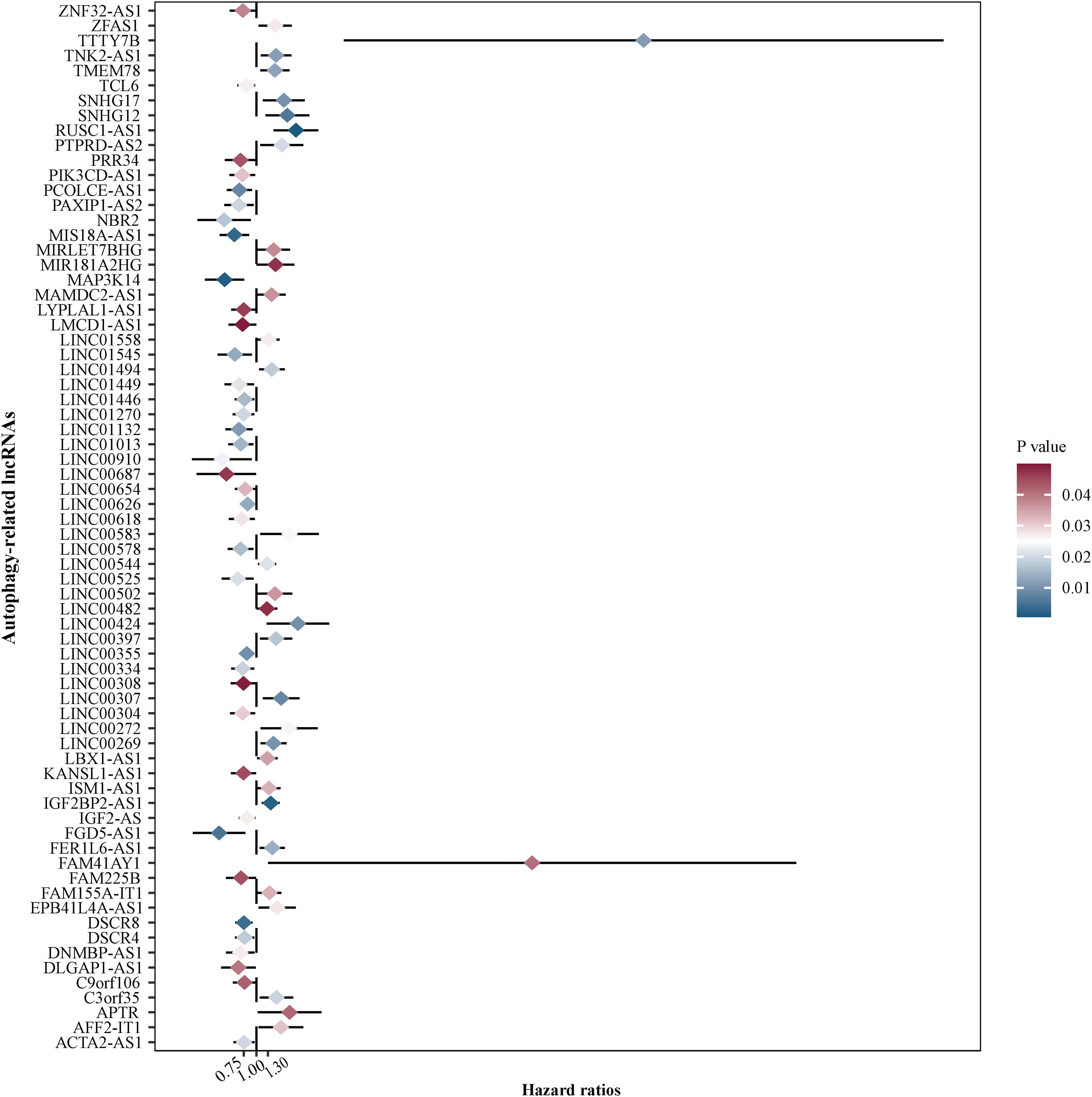
Figure 3. Identification of autophagy-related lncRNAs with prognostic value in osteosarcoma. We performed univariate Cox regression analyses and identified 69 autophagy-related lncRNAs associated with osteosarcoma patient prognosis. Red color represents high P-values, whereas blue color represents low P-values.
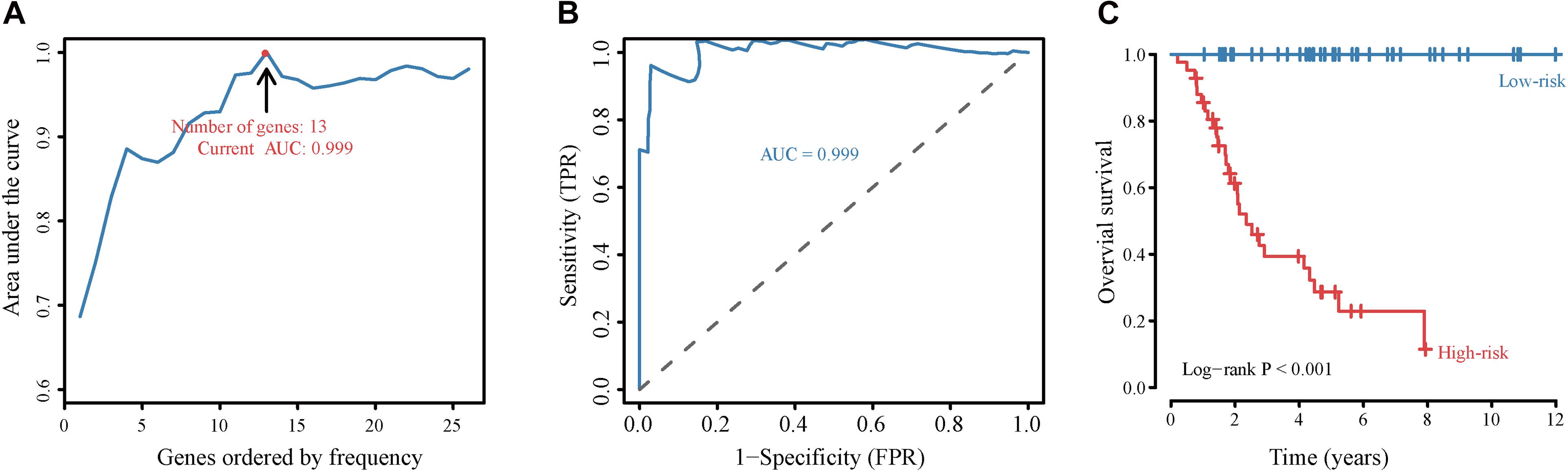
Figure 4. Construction and validation of the autophagy-related lncRNA signature-based prognostic model. (A) Identification of the autophagy-related lncRNA signature by iterative lasso Cox regression analyses. (B) ROC curve showing the prognostic performance of the autophagy-related lncRNA signature. (C) Kaplan-Meier survival analyses of patients in the high-risk score and the low-risk score groups. Patients with high scores (red line) have significantly worse overall survival than those with low scores (blue line); P < 0.05 was considered statistically significant.
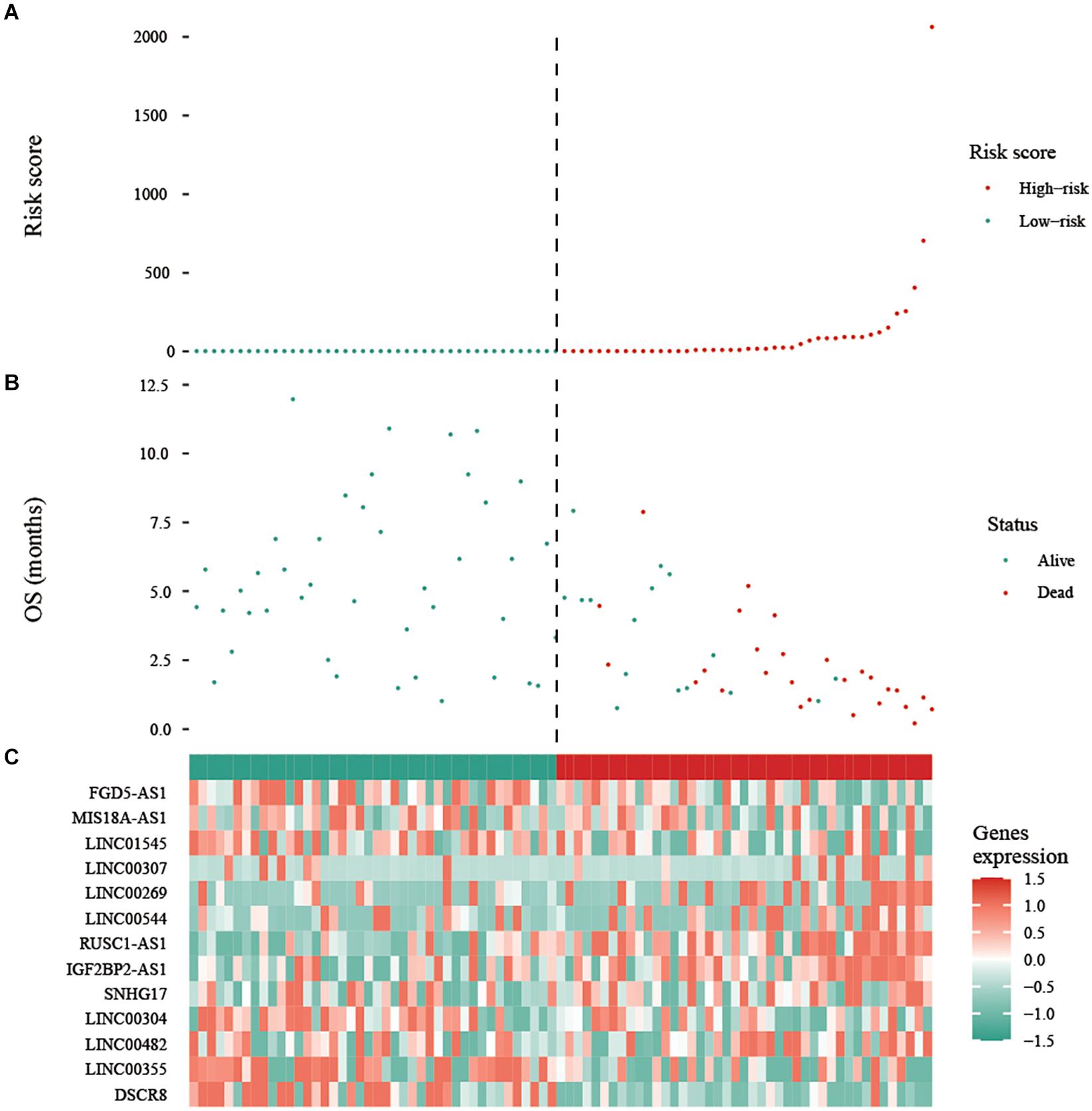
Figure 5. Expression of the prognostic autophagy-related lncRNA signature. (A) Risk score distribution of patients in the prognostic autophagy-related lncRNA signature. Red means high risk, green means low risk. (B) Survival status scatter plots for patients in the prognostic autophagy-related lncRNA signature. The green and red dots represent survival and death, respectively. (C) Expression patterns of risk genes in the prognostic autophagy-related lncRNA signature. Red means high expression, green means low expression. OS: overall survival.
Autophagy-Related lncRNA Signature Validation and Comparison With Other Known Prognostic Biomarkers
Using data from the training cohort, we performed ROC analyses of the autophagy-related lncRNA signature along with other known prognostic biomarkers (SP140, CCNE1, SNHG3, and MIR221). Interestingly, the AUC of the autophagy-related lncRNA signature was higher than that of other known biomarkers (Figure 6), indicating that the autophagy-related lncRNAs signature is a better prognostic biomarker, providing a more accurate prediction of osteosarcoma patient survival.
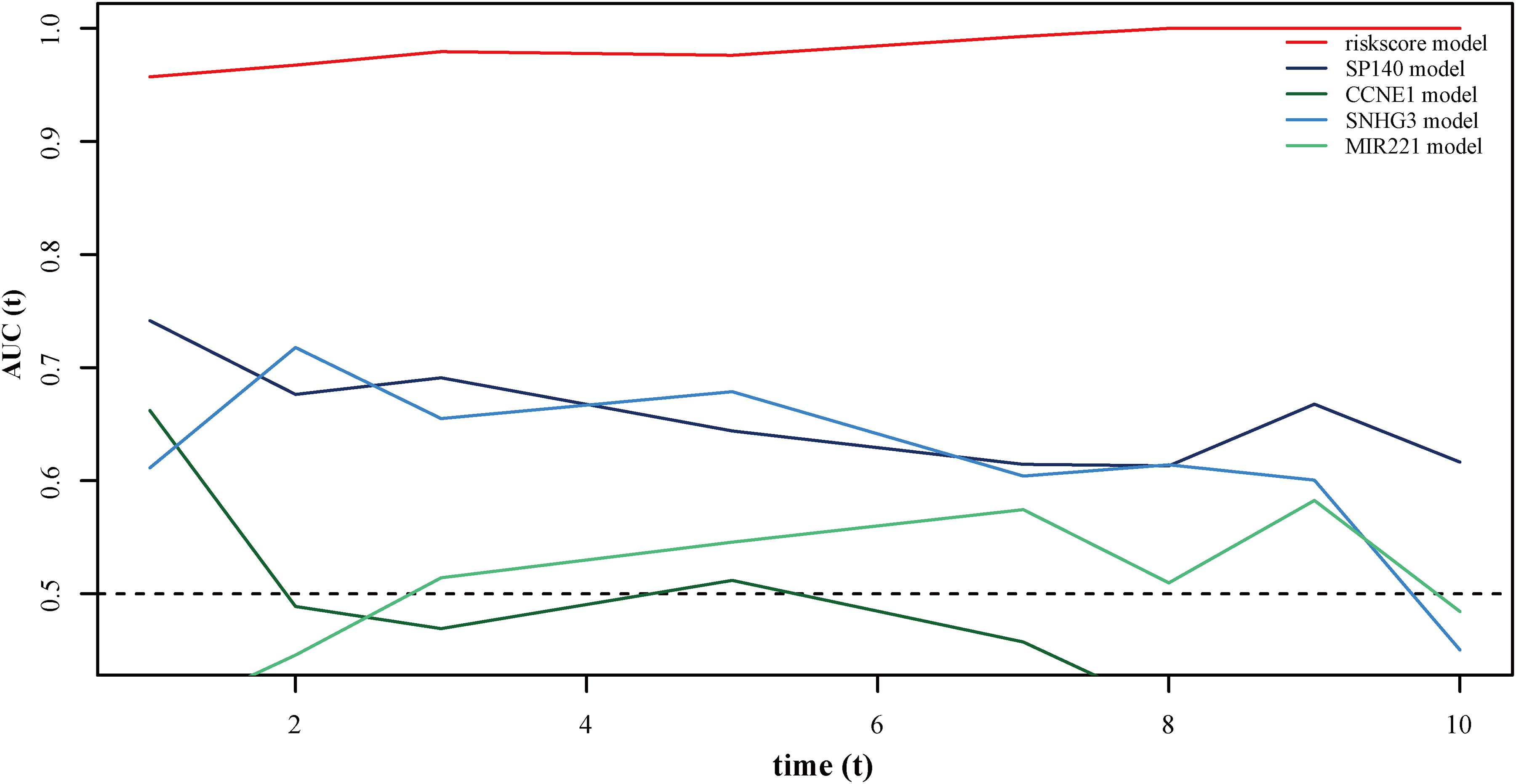
Figure 6. ROC curves showing the prognostic value of the autophagy-related lncRNA signature and other prognostic biomarkers in osteosarcoma patients.
Assessment of the lncRNA Signature as an Independent Prognostic Factor and Nomogram Construction
We grouped osteosarcoma patients based on different clinicopathological characteristics (age, sex, tumor location, and metastasis) and performed Kaplan-Meier survival analyses to assess the prognostic value of the autophagy-related lncRNA signature. We found that low-risk group patients lived significantly longer, regardless of age, sex, location, and metastasis (Figure 7). Univariate Cox regression analyses showed that tumor metastasis (hazard ratio [HR] = 0.212, P < 0.001), tumor location (HR = 2.241, P < 0.032), and expression levels of the 13 autophagy-related signature lncRNAs (HR = 1.003, P < 0.001) were significant predictors of osteosarcoma patient prognosis. Multivariate Cox regression analyses revealed that tumor metastasis (HR = 0.013, P < 0.001), stage (HR = 0.196, P = 0.014), tumor site (HR = 2.999, P = 0.007), and expression level of the autophagy-related lncRNA signature (HR = 1.002, P < 0.001) were significantly associated with osteosarcoma patient prognosis (Figure 8). These results suggest that the autophagy-related lncRNA signature is an independent prognostic factor in osteosarcoma patients. In addition, we combined the clinicopathological characteristics of the patients with the expression levels of the 13 autophagy-related lncRNAs to construct a nomogram (Supplementary Figure 1) and found that the signature provided an accurate prediction of osteosarcoma patient prognosis.
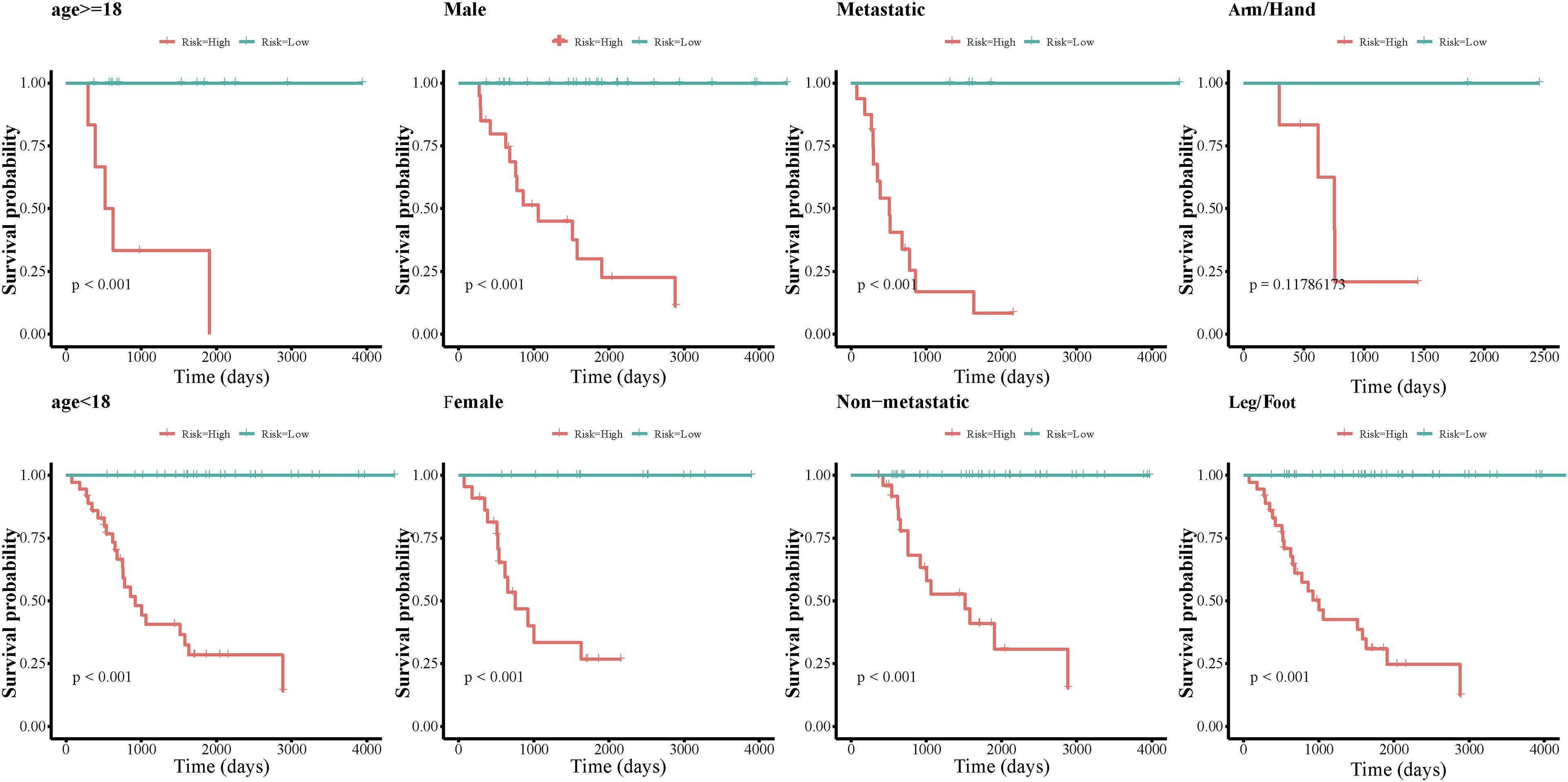
Figure 7. Kaplan-Meier survival analyses of the autophagy-related lncRNA signature in different osteosarcoma patient subgroups.
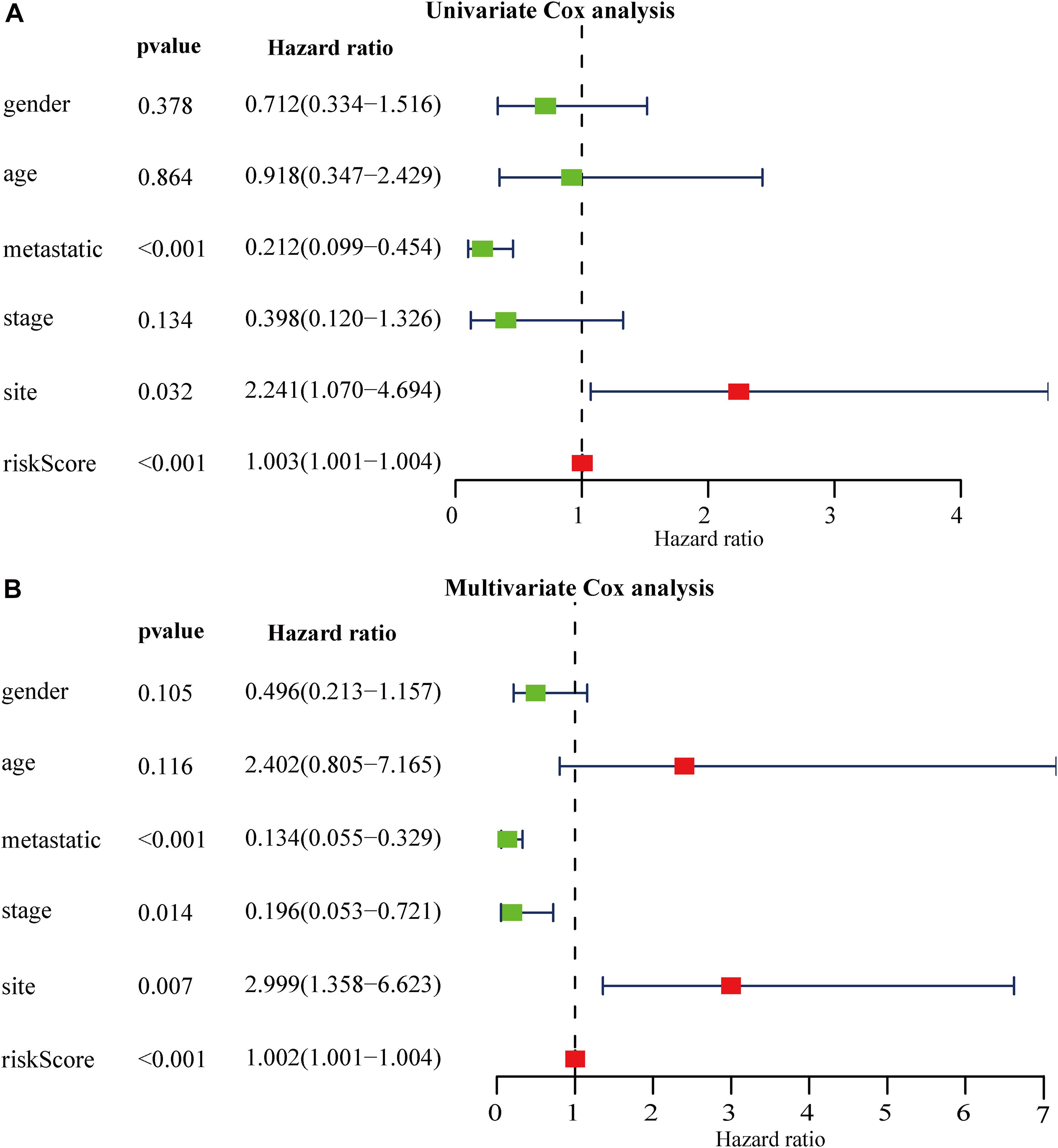
Figure 8. Relationship between autophagy-related lncRNA signature expression, clinicopathological characteristics, and osteosarcoma patient prognosis. (A,B) Forest plot showing the results of (A) univariate Cox regression analyses and (B) multivariate Cox regression analyses.
GSEA and GSVA High- and Low-Risk Osteosarcoma Patients
GSEA and GSVA were performed to identify important functional phenotypes between high- and low-risk osteosarcoma patients. GSEA revealed enrichment of gene sets associated with the CTLA4 pathway, TOB1 pathway, cell aggregation, antimicrobial humoral immune response, and EMT in high-risk osteosarcoma patients (Figures 9A–C). GSVA results indicated an enrichment of gene sets related to the MYC pathway, bile acid metabolism, allograft rejection, NOTCH signaling, and hedgehog signaling in high-risk osteosarcoma patients (Figure 9D).
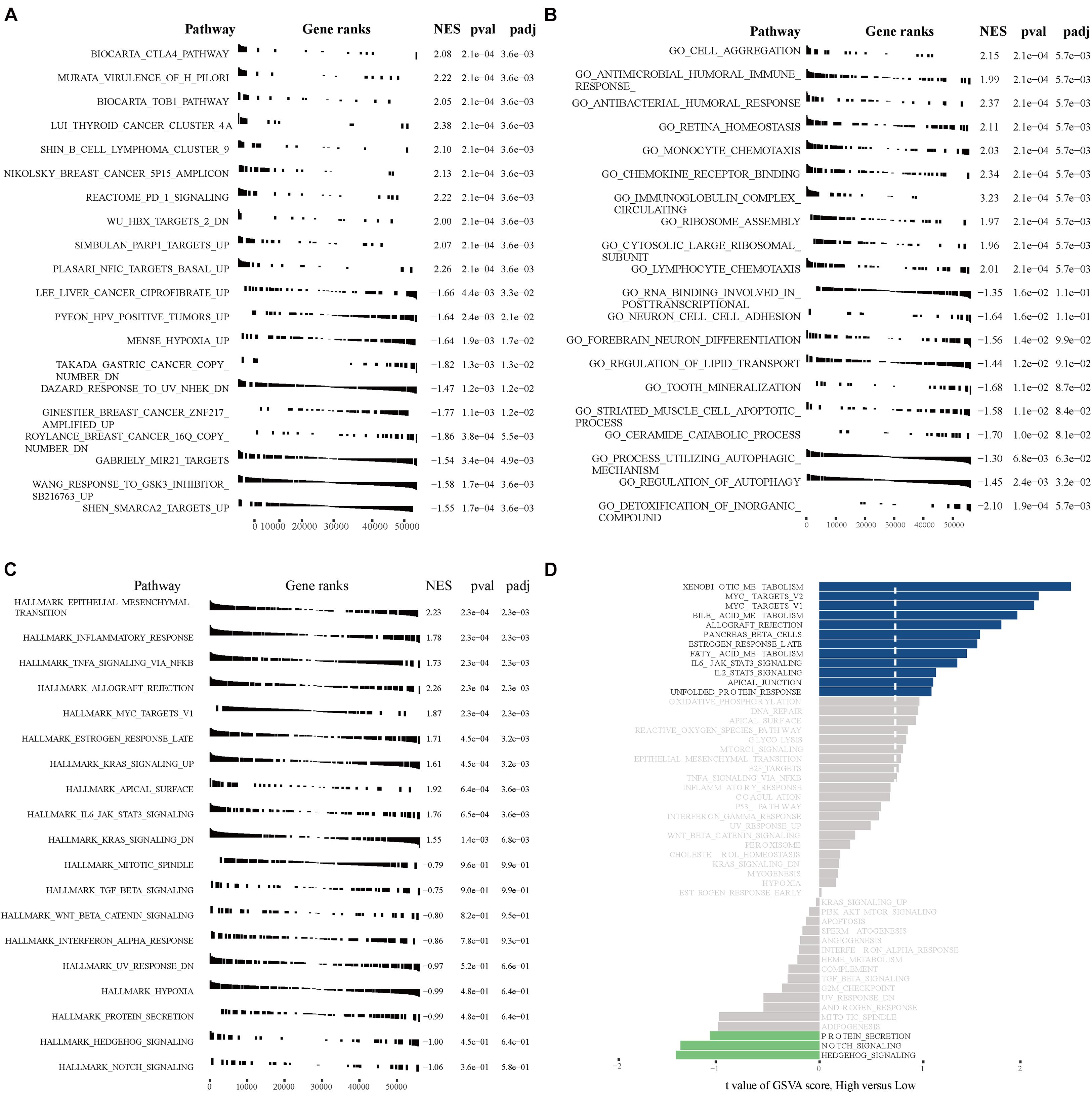
Figure 9. GSEA and GSVA in high- and low-risk osteosarcoma patients. (A) GSEA analyses with “c2.all.v7.1.symbols.gmt” as the reference gene set. (B) GSEA analyses with “c5.all.v7.1.symbols.gmt” as the reference gene set. (C) GSEA analyses with “h.all.v7.1.symbols.gmt” as the reference gene set. (D) GSVA analyses with “h.all.v7.1.symbols.gmt” as the reference gene set.
Analyses of Immune Cells in Osteosarcoma Tissues and Their Role in Osteosarcoma Patient Prognosis
Immune cell infiltration analyses revealed that T helper cells, effector memory T (TEM) cells, macrophages, CD8+ T cells, natural killer (NK) cells, and T helper 2 (Th2) cells were accumulated in osteosarcoma tissues, whereas the levels of infiltrating mast cells and regulatory T cells (Tregs) were low (Figure 10A). Immune cell interaction analyses indicated that dendritic cells (DCs), activated DCs (aDCs), immature DCs (iDCs), B cells, cytotoxic cells, and macrophages interacted strongly with other immune cells found in the tumor microenvironment (TME), in contrast to gamma delta T cells (Tgd), Th2 cells, central memory T (TCM) cells, and T helper cells (Figure 10B). Interestingly, we found that the levels of eosinophils, NK cells, plasmacytoid DCs (pDCs), TCM, Tgd, and Th2 cells, were higher in the TME of high-risk group than of low-risk group. By contrast, cytotoxic cells, macrophages, T cells, Th1 cells, DCs, neutrophils, iDC, NK cells, and Tregs were found in lower levels in the high-risk group (Figure 10C). Next, we analyzed the effects of different immune cells on osteosarcoma patient prognosis and found that low levels of cytotoxic cells, macrophages, T cells, Th1 cells, DCs, neutrophils, iDCs, NK cells, follicular helper T (TFH) cells, and Tregs were associated with poor prognosis. High levels of Th2 and Tgd cells in the TME were associated with poor prognosis in osteosarcoma patients (Figure 11).
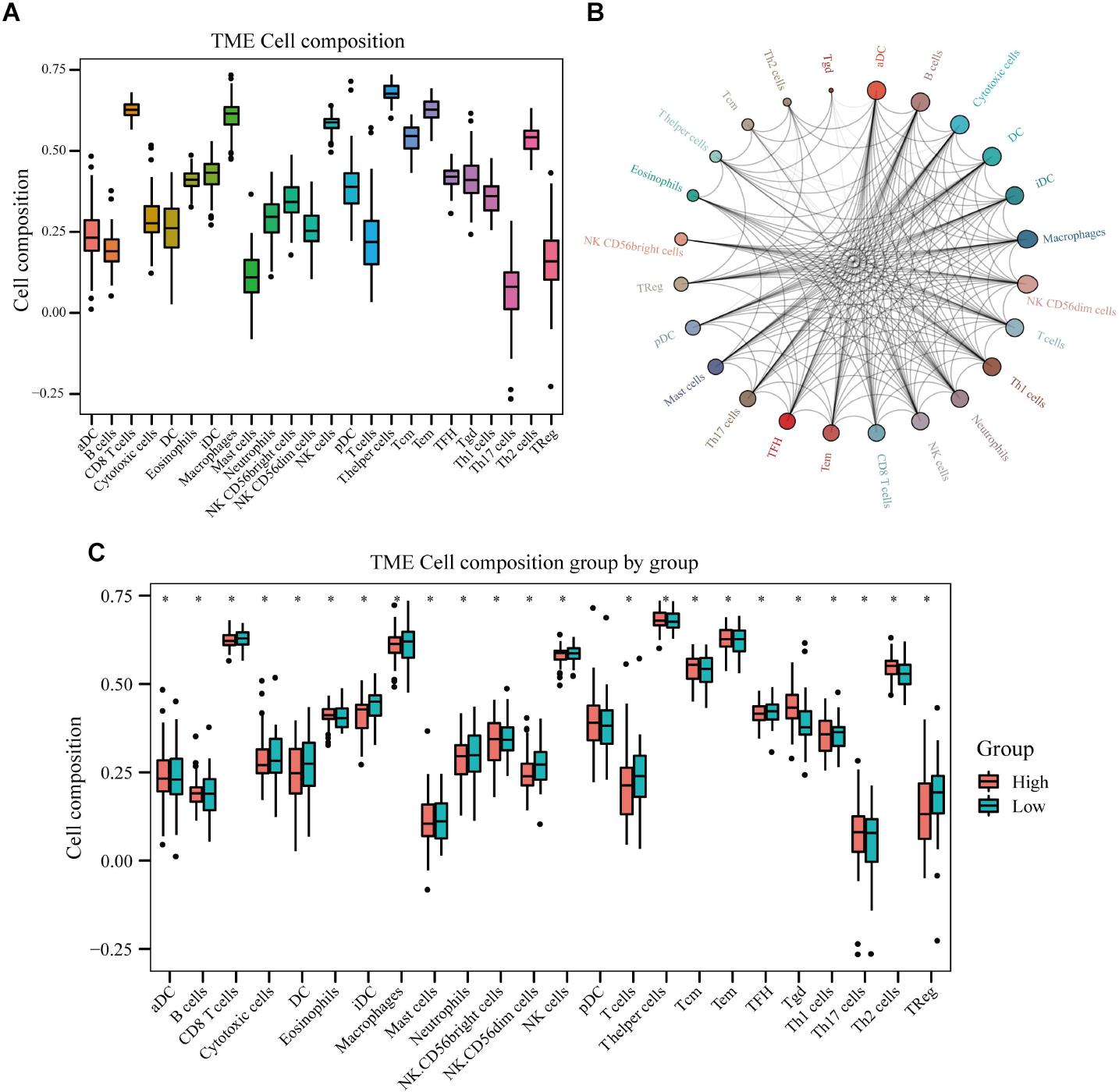
Figure 10. Immune cell infiltration in osteosarcoma tissues of high- and low-risk patients. (A) Results of immune cell infiltration composition analyses. (B) Immune cell interaction network. (C) Differences in immune cell infiltration between high- and low-risk osteosarcoma patients.
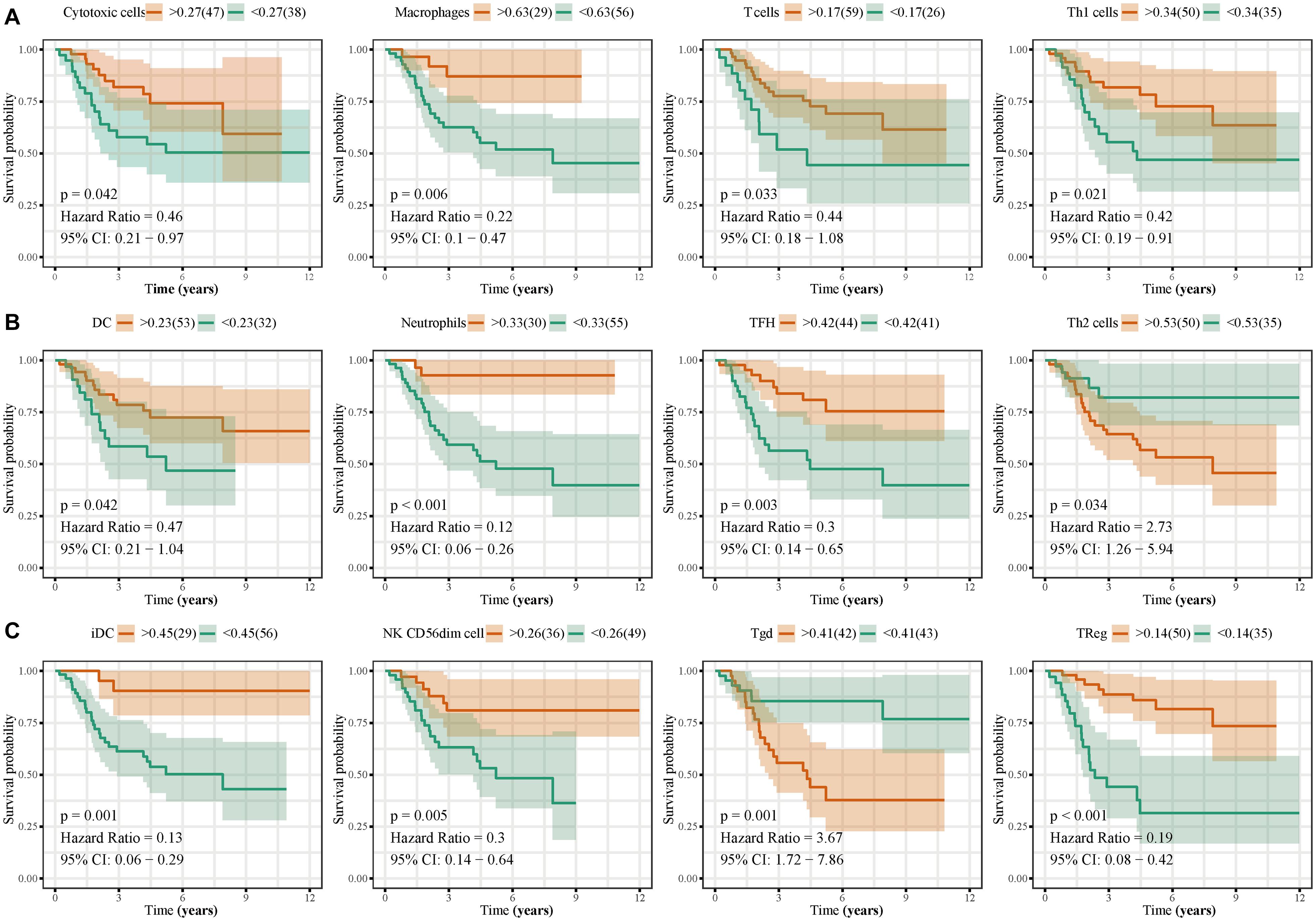
Figure 11. Effects of immune cell infiltration on the prognosis of osteosarcoma patients. Low levels of cytotoxic cells, macrophages, T cells, Th1 cells, DCs, neutrophils, TFH, iDC, NK CD56dim cells, and Tregs, were associated with poor osteosarcoma patient prognosis. High levels of Th2 and Tgd cells were associated with poor prognosis in osteosarcoma patients.
Relationship Between Autophagy-Related lncRNAs and Immune Cell Infiltration
Correlation analyses between autophagy-related lncRNAs and infiltrating immune cells revealed that the expression levels of SNHG17, LINC00482, MIS18A-AS1, and LINC01545 were positively correlated with the levels of CD8+ T cells, eosinophils, TFH cells, and Tregs, respectively. RUSC1-AS1 expression levels were negatively correlated with the levels of infiltrating iDCs, macrophages, and mast cells. In addition, LINC00307, IGF2BP2-AS1, and DSCR8 levels were negatively correlated with the levels of neutrophils, NK CD56dim cells, and Th2 cells, respectively. Furthermore, the expression levels of the autophagy-related lncRNA signature were positively correlated with Tgd levels and negatively correlated with NK CD56dim, neutrophil, Treg, and iDC levels (Figure 12).
Discussion
Osteosarcoma is a common bone malignancy in young adults and children. It is characterized by high rates of early distant metastasis, high mortality rate, and extremely poor prognosis. Osteosarcoma patients often require aggressive treatments and frequent monitoring. Despite the need for robust biomarkers, most candidate biomarkers exhibit unsatisfactory prognostic and predictive performance. The most well-established prognostic markers used in a clinical setting are predominantly based on tumor features, such as AJCC-TNM classification. In osteosarcoma, however, the sensitivity, specificity, and accuracy of these factors are limited. Recent studies have shown that aberrant expression of lncRNAs is associated with osteosarcoma development, progression, and metastasis, and thus can be used as a predictive and prognostic biomarker in osteosarcoma (Lu et al., 2019; Negri et al., 2019). In this study, for the first time, we analyzed the sequencing data of osteosarcoma samples from the TARGET database using various bioinformatic tools and machine learning algorithms to establish a robust autophagy-related lncRNA signature to predict prognosis in osteosarcoma patients.
We initially identified a total of 695 autophagy-related lncRNAs. We found that these were enriched in functional terms related to response to nutrient levels, gland development, and sensory system development, cell adhesion, and protein serine/threonine kinase activity. Cell leading edge (Perike et al., 2014), focal adhesion (Gu and Zhou, 2018), cell adhesion molecule binding (Du et al., 2018), protein serine/threonine kinase activity (Pruksakorn et al., 2018), phospholipid-binding (Jiang et al., 2018), ATPase activity (Meszaros and Karin, 1995), and protein tyrosine kinase activity (Kostas et al., 2018) have been previously shown to be associated with osteosarcoma development and metastasis. Consistent with the GO enrichment findings, enriched KEGG pathways included focal adhesion and lysosomal degradation. Studies have shown that certain focal adhesion molecules promoted tumor progression and were associated with poor clinical outcomes in osteosarcoma patients (Gu and Zhou, 2018).
Using univariate Cox and iterative lasso Cox regression analyses we identified 13 lncRNAs (DSCR8, IGF2BP2-AS1, MIS18A-AS1, RUSC1-AS1, FGD5-AS1, LINC00269, LINC00307, LINC00355, LINC00482, LINC00544, LINC01545, SNHG17) with prognostic value in osteosarcoma. Interestingly, abnormal expression of DSCR8 is associated with poor prognosis in the liver (Wang et al., 2018) and ovarian cancer (You et al., 2020). Abnormal expression of RUSC1-AS1 has also been implicated in the development of cervical cancer (Guo et al., 2020). FGD5-AS1 has been shown to promote colorectal cancer cell proliferation, migration, and invasion by functioning as a sponge for miR-302e and regulating CDCA7 expression (Li et al., 2019). Similarly, LINC00355 has been shown to act as an miR-195 sponge, thereby upregulating HOXA10 and promoting migration, invasion, and EMT in head and neck squamous cell carcinoma (Lu et al., 2020). Importantly, we compared the prognostic ability of these 13 autophagy-related lncRNAs with those of other known prognostic biomarkers in an independent cohort (using the GSE39055 dataset). The autophagy-related lncRNA signature predicted osteosarcoma patient prognosis with high sensitivity and specificity and independently of age, sex, tumor location, and tumor metastasis. Consistently, univariate and multivariate Cox regression analyses indicated that the autophagy-related lncRNA signature was an independent prognostic factor in osteosarcoma patients. We also integrated the signature and clinicopathological factors to construct a nomogram and confirmed that our autophagy-related lncRNA signature was an independent and robust prognostic biomarker and could be used to monitor osteosarcoma patients.
The CTLA4, TOB1, MYC, Notch, Hedgehog, and EMT pathways play a critical role in cancer progression (Artym et al., 2003; Hamester et al., 2019; Jiang K. et al., 2019; Karacosta et al., 2019; Kalbasi and Ribas, 2020). Intriguingly, GSEA/GSVA results indicated that the 13 autophagy-related lncRNAs were predicted to affect all of these pathways. Wang et al. (2016) reported that CTLA-4 is constitutively expressed in memory T cells and Tregs promoting osteosarcoma progression. MYC-driven signals have also been linked to osteosarcoma development, and targeting the MYC-driven super-enhancer signaling has emerged as a promising treatment strategy for osteosarcoma patients (Chen D. et al., 2018). Canalis (2018) showed that changes in Notch signaling are associated with osteosarcoma development, as well as with the metastatic potential of breast and prostate cancer cells. Zhao Z. et al. (2018) showed that the aberrant activation of the Hedgehog pathway is also associated with enhanced osteosarcoma growth and metastasis. In accordance with these previous findings, we found that the autophagy-related lncRNA signature was significantly associated with osteosarcoma initiation and progression.
It has become widely accepted that immune cell infiltration in the TME regulates various tumor features, including tumor malignancy and metastatic potential (Manandhar and Lee, 2018; Steven and Seliger, 2018; Lee and Cho, 2020). Here, we analyzed the composition of immune cells in the osteosarcoma TME. We found elevated levels of macrophages, CD8+ T cells, NK cells, and Th2 cells in osteosarcoma tissues, whereas the levels of mast cells and Tregs were very low. Interestingly, high-risk group patients exhibited higher levels of eosinophils, NK cells, pDCs, TCM, Tgd, and Th2 cells compared to low-risk group patients. On the other hand, the levels of infiltrating cytotoxic cells, macrophages, T cells, Th1 cells, DCs, neutrophils, TFH cells, iDCs, NK CD56dim cells, and Tregs were lower in patients that expressed high levels of the autophagy-related lncRNA signature than those that expressed low levels of it. We also found that low levels of infiltrating cytotoxic cells, macrophages, T cells, Th1 cells, DCs, neutrophils, TFH cells, iDCs, NK CD56dim cells, and Tregs were associated with poor prognosis in osteosarcoma patients. High levels of infiltrating Th2 and Tgd cells were also associated with poor outcomes. Macrophages have been shown to accumulate in osteosarcoma tissues, establishing an immunotolerant TME that facilitates tumor progression (Heymann et al., 2019). During osteosarcoma progression, macrophages in the TME are reprogrammed toward an M2-like phenotype, further promoting osteosarcoma growth (Kelleher and O’Sullivan, 2017). Clinical studies have shown that in osteosarcoma patients, the number of circulating NK cells in the peripheral blood is significantly reduced, suggesting that NK cells play an important role in the development of osteosarcoma (Tullius et al., 2020). In addition, ANGPTL2 secretion by tumor cells has been demonstrated to recruit neutrophils into the lungs, triggering osteosarcoma lung metastasis (Charan et al., 2020). In this study, we analyzed the relationship between the 13 autophagy-related lncRNAs and immune cell infiltration and found that the expression levels of SNHG17, LINC00482, MIS18A-AS1, and LINC01545 were positively correlated with the levels of CD8+ T cells, eosinophils, TFH cells, and Tregs, respectively. RUSC1-AS1 levels were negatively correlated with the levels of infiltrating iDCs, macrophages, and mast cells. Similarly, LINC00307, IGF2BP2-AS1, and DSCR8 expression levels were negatively correlated with the levels of infiltrating neutrophils, NK CD56dim cells, and Th2 cells, respectively. Most importantly, we found that the expression levels of the autophagy-related lncRNA signature were positively correlated with the levels of infiltrating Tgd cells and negatively correlated with the infiltration levels of NK CD56dim cells, neutrophils, and iDCs. However, the mechanisms underlying the effects of autophagy-related lncRNAs and immune cell infiltration remain poorly understood and merit further investigation.
Despite the novelty and significant clinical implications of our findings, our study had several limitations. First, we only analyzed data from the TARGET database, and the sample size was relatively small. Second, although we provide some evidence regarding the possible mechanisms by which autophagy-related lncRNAs affect prognosis, further comprehensive studies are required to elucidate the precise mechanisms underlying the prognostic effects of these autophagy-related lncRNAs in osteosarcoma. Finally, our results are based on bioinformatic analyses and require experimental validation in large-cohort clinical studies.
Conclusion
We performed comprehensive bioinformatics analyses of expression data from osteosarcoma patients and developed a novel signature composed of 13 autophagy-related lncRNAs. Compared to clinically used prognostic biomarkers, the autophagy-related lncRNA signature provides a more robust and accurate prediction of osteosarcoma patient prognosis. We also show that the expression of these 13 autophagy-related lncRNAs may promote osteosarcoma development and progression by regulating immune cell infiltration in the TME. Therefore, this autophagy-related lncRNA signature may represent a promising prognostic biomarker in osteosarcoma.
Data Availability Statement
The original contributions presented in the study are included in the article/Supplementary Material, further inquiries can be directed to the corresponding author/s.
Author Contributions
G-ZZ, Z-LW, E-HR, and Q-QX conceived and designed the study. C-YL, W-HY, and Y-JD contributed to dataset selection and bioinformatics analysis. G-ZZ and Q-QX wrote and revised the manuscript. All authors read and approved the final manuscript.
Funding
This work was supported by The Key Research & Development and Transformation Project of Qinghai Province for 2018 (2018-SF-113).
Conflict of Interest
The authors declare that the research was conducted in the absence of any commercial or financial relationships that could be construed as a potential conflict of interest.
Acknowledgments
This work has benefited from TARGET and GEO. We thank the TARGET and GEO network for its generous sharing large amounts of data. We would like to thank FigureYa (Xiao Ya Hua Tu) for the figure technology support. We would also like to thank Textcheck (www.textcheck.com) for English language editing. We would also like to thank the reviewers for their constructive comments.
Supplementary Material
The Supplementary Material for this article can be found online at: https://www.frontiersin.org/articles/10.3389/fmolb.2021.615084/full#supplementary-material
Supplementary Figure 1 | Prognostic nomogram for OS patients.
Abbreviations
OS, osteosarcoma; TARGET, Therapeutically Applied Research To Generate Effective Treatments; GEO, gene expression omnibus; GO, gene ontology; KEGG, Kyoto encyclopedia of genes and genomes; PPI, protein-protein interaction; HADb, human autophagy database; BP, biological process; CC, cell components; MF, molecular function; HR, hazard ratio; Lasso, least absolute shrinkage and selection operator.
Footnotes
- ^ https://ocg.cancer.gov/programs/target
- ^ http://autophagy.lu/clustering/index.html
- ^ https://CRAN.R-project.org/package=glmnet
References
Amaravadi, R. K., Kimmelman, A. C., and Debnath, J. (2019). Targeting Autophagy in Cancer: Recent Advances and Future Directions. Cancer Discov. 9, 1167–1181. doi: 10.1158/2159-8290.CD-19-0292
Artym, J., Zimecki, M., Paprocka, M., and Kruzel, M. L. (2003). Orally administered lactoferrin restores humoral immune response in immunocompromised mice. Immunol. Lett. 89, 9–15. doi: 10.1016/s0165-2478(03)00102-0
Barrett, T., Wilhite, S. E., Ledoux, P., Evangelista, C., Kim, I. F., Tomashevsky, M., et al. (2013). NCBI GEO: archive for functional genomics data sets–update. Nucleic Acids Res. 41, D991–D995. doi: 10.1093/nar/gks1193
Canalis, E. (2018). Notch in skeletal physiology and disease. Osteoporos. Int. 29, 2611–2621. doi: 10.1007/s00198-018-4694-3
Charan, M., Dravid, P., Cam, M., Setty, B., Roberts, R. D., Houghton, P. J., et al. (2020). Tumor secreted ANGPTL2 facilitates recruitment of neutrophils to the lung to promote lung pre-metastatic niche formation and targeting ANGPTL2 signaling affects metastatic disease. Oncotarget 11, 510–522. doi: 10.18632/oncotarget.27433
Chen, B., Khodadoust, M. S., Liu, C. L., Newman, A. M., and Alizadeh, A. A. (2018). Profiling Tumor Infiltrating Immune Cells with CIBERSORT. Methods Mol. Biol. 1711, 243–259. doi: 10.1007/978-1-4939-7493-1_12
Chen, D., Zhao, Z., Huang, Z., Chen, D. C., Zhu, X. X., Wang, Y. Z., et al. (2018). Super enhancer inhibitors suppress MYC driven transcriptional amplification and tumor progression in osteosarcoma. Bone Res. 6:11. doi: 10.1038/s41413-018-0009-8
Chen, D. P., Ning, W. R., Li, X. F., Wei, Y., Lao, X. M., Wang, J. C., et al. (2018). Peritumoral monocytes induce cancer cell autophagy to facilitate the progression of human hepatocellular carcinoma. Autophagy 14, 1335–1346. doi: 10.1080/15548627.2018.1474994
Chen, H., Wahafu, P., Wang, L., and Chen, X. (2020). LncRNA LINC00313 Knockdown Inhibits Tumorigenesis and Metastasis in Human Osteosarcoma by Upregulating FOSL2 through Sponging miR-342-3p. Yonsei Med. J. 61, 359–370. doi: 10.3349/ymj.2020.61.5.359
Chen, J., Wu, Z., and Zhang, Y. (2019). LncRNA SNHG3 promotes cell growth by sponging miR-196a-5p and indicates the poor survival in osteosarcoma. Int. J. Immunopathol. Pharmacol. 33:1680057991. doi: 10.1177/2058738418820743
Chi, Y., Wang, D., Wang, J., Yu, W., and Yang, J. (2019). Long Non-Coding RNA in the Pathogenesis of Cancers. Cells 8:1015. doi: 10.3390/cells8091015
Choi, R. S., Lai, W., Lee, L., Wong, W., Pei, X. M., Tsang, H. F., et al. (2019). Current and future molecular diagnostics of gastric cancer. Exp. Rev. Mol. Diagn. 19, 863–874. doi: 10.1080/14737159.2019.1660645
Cuomo, F., Altucci, L., and Cobellis, G. (2019). Autophagy Function and Dysfunction: Potential Drugs as Anti-Cancer Therapy. Cancers 11:1465. doi: 10.3390/cancers11101465
Dehne, N., Mora, J., Namgaladze, D., Weigert, A., and Brüne, B. (2017). Cancer cell and macrophage cross-talk in the tumor microenvironment. Curr. Opin. Pharmacol. 35, 12–19. doi: 10.1016/j.coph.2017.04.007
Du, K., Zhang, X., Lou, Z., Guo, P., Zhang, F., Wang, B., et al. (2018). MicroRNA485-3p negatively regulates the transcriptional co-repressor CtBP1 to control the oncogenic process in osteosarcoma cells. Int. J. Biol. Sci. 14, 1445–1456. doi: 10.7150/ijbs.26335
Feng, J., Yang, G., Liu, Y., Gao, Y., Zhao, M., Bu, Y., et al. (2019). LncRNA PCNAP1 modulates hepatitis B virus replication and enhances tumor growth of liver cancer. Theranostics 9, 5227–5245. doi: 10.7150/thno.34273
Folkerts, H., Hilgendorf, S., Vellenga, E., Bremer, E., and Wiersma, V. R. (2019). The multifaceted role of autophagy in cancer and the microenvironment. Med. Res. Rev. 39, 517–560. doi: 10.1002/med.21531
Gao, L., Lin, P., Chen, P., Gao, R. Z., Yang, H., He, Y., et al. (2020). A novel risk signature that combines 10 long noncoding RNAs to predict neuroblastoma prognosis. J. Cell Physiol. 235, 3823–3834. doi: 10.1002/jcp.29277
Gu, H. J., and Zhou, B. (2018). Focal adhesion kinase promotes progression and predicts poor clinical outcomes in patients with osteosarcoma. Oncol. Lett. 15, 6225–6232. doi: 10.3892/ol.2018.8152
Guo, Q., Zhang, Q., Lu, L., and Xu, Y. (2020). Long noncoding RNA RUSC1-AS1 promotes tumorigenesis in cervical cancer by acting as a competing endogenous RNA of microRNA-744 and consequently increasing Bcl-2 expression. Cell Cycle 19, 1222–1235. doi: 10.1080/15384101.2020.1749468
Hamester, F., Legler, K., Wichert, B., Kelle, N., Eylmann, K., Rossberg, M., et al. (2019). Prognostic relevance of the Golgi mannosidase MAN1A1 in ovarian cancer: impact of N-glycosylation on tumour cell aggregation. Br. J. Cancer 121, 944–953. doi: 10.1038/s41416-019-0607-2
Hänzelmann, S., Castelo, R., and Guinney, J. (2013). GSVA: gene set variation analysis for microarray and RNA-seq data. BMC Bioinform. 14:7. doi: 10.1186/1471-2105-14-7
Heagerty, P. J., Lumley, T., and Pepe, M. S. (2000). Time-dependent ROC curves for censored survival data and a diagnostic marker. Biometrics 56, 337–344. doi: 10.1111/j.0006-341x.2000.00337.x
Heymann, M. F., Lézot, F., and Heymann, D. (2019). The contribution of immune infiltrates and the local microenvironment in the pathogenesis of osteosarcoma. Cell Immunol. 343:103711. doi: 10.1016/j.cellimm.2017.10.011
Hong, W., Yuan, H., Gu, Y., Liu, M., Ji, Y., Huang, Z., et al. (2020). Immune-related prognosis biomarkers associated with osteosarcoma microenvironment. Cancer Cell Int. 20:83. doi: 10.1186/s12935-020-1165-7
Iasonos, A., Schrag, D., Raj, G. V., and Panageas, K. S. (2008). How to build and interpret a nomogram for cancer prognosis. J. Clin. Oncol. 26, 1364–1370. doi: 10.1200/JCO.2007.12.9791
Jiang, K., Zhi, X. H., Ma, Y. Y., and Zhou, L. Q. (2019). Long non-coding RNA TOB1-AS1 modulates cell proliferation, apoptosis, migration and invasion through miR-23a/NEU1 axis via Wnt/b-catenin pathway in gastric cancer. Eur. Rev. Med. Pharmacol. Sci. 23, 9890–9899. doi: 10.26355/eurrev_201911_19554
Jiang, N., Zhang, X., He, Y., Luo, B., He, C., Liang, Y., et al. (2019). Identification of key protein-coding genes and lncRNAs in spontaneous neutrophil apoptosis. Sci. Rep. 9:15106. doi: 10.1038/s41598-019-51597-9
Jiang, Z., Jiang, J., Zhao, B., Yang, H., Wang, Y., Guo, S., et al. (2018). CPNE1 silencing inhibits the proliferation, invasion and migration of human osteosarcoma cells. Oncol. Rep. 39, 643–650. doi: 10.3892/or.2017.6128
Kalbasi, A., and Ribas, A. (2020). Tumour-intrinsic resistance to immune checkpoint blockade. Nat. Rev. Immunol. 20, 25–39. doi: 10.1038/s41577-019-0218-4
Karacosta, L. G., Anchang, B., Ignatiadis, N., Kimmey, S. C., Benson, J. A., Shrager, J. B., et al. (2019). Mapping lung cancer epithelial-mesenchymal transition states and trajectories with single-cell resolution. Nat. Commun. 10:5587. doi: 10.1038/s41467-019-13441-6
Kelleher, F. C., and O’Sullivan, H. (2017). Monocytes, Macrophages, and Osteoclasts in Osteosarcoma. J. Adolesc. Young Adult Oncol. 6, 396–405. doi: 10.1089/jayao.2016.0078
Kelly, A. D., Haibe-Kains, B., Janeway, K. A., Hill, K. E., Howe, E., Goldsmith, J., et al. (2013). MicroRNA paraffin-based studies in osteosarcoma reveal reproducible independent prognostic profiles at 14q32. Genome Med. 5:2. doi: 10.1186/gm406
Kim, M., Jung, J. Y., Choi, S., Lee, H., Morales, L. D., Koh, J. T., et al. (2017). GFRA1 promotes cisplatin-induced chemoresistance in osteosarcoma by inducing autophagy. Autophagy 13, 149–168. doi: 10.1080/15548627.2016.1239676
Kostas, M., Haugsten, E. M., Zhen, Y., Sørensen, V., Szybowska, P., Fiorito, E., et al. (2018). Protein Tyrosine Phosphatase Receptor Type G (PTPRG) Controls Fibroblast Growth Factor Receptor (FGFR) 1 Activity and Influences Sensitivity to FGFR Kinase Inhibitors. Mol. Cell Proteom. 17, 850–870. doi: 10.1074/mcp.RA117.000538
Lee, Y. S., and Cho, Y. B. (2020). CCL7 Signaling in the Tumor Microenvironment. Adv. Exp. Med. Biol. 1231, 33–43. doi: 10.1007/978-3-030-36667-4_4
Levine, B., and Kroemer, G. (2019). Biological Functions of Autophagy Genes: A Disease Perspective. Cell 176, 11–42. doi: 10.1016/j.cell.2018.09.048
Levy, J., Towers, C. G., and Thorburn, A. (2017). Targeting autophagy in cancer. Nat. Rev. Cancer 17, 528–542. doi: 10.1038/nrc.2017.53
Li, D., Jiang, X., Zhang, X., Cao, G., Wang, D., and Chen, Z. (2019). Long noncoding RNA FGD5-AS1 promotes colorectal cancer cell proliferation, migration, and invasion through upregulating CDCA7 via sponging miR-302e. Vitro Cell Dev. Biol. Anim. 55, 577–585. doi: 10.1007/s11626-019-00376-x
Li, J., Xu, Y. H., Lu, Y., Ma, X. P., Chen, P., Luo, S. W., et al. (2013). Identifying differentially expressed genes and small molecule drugs for prostate cancer by a bioinformatics strategy. Asian Pac. J. Cancer Prev. 14, 5281–5286. doi: 10.7314/apjcp.2013.14.9.5281
Liu, Y. J., Li, W., Chang, F., Liu, J. N., Lin, J. X., and Chen, D. X. (2018). MicroRNA-505 is downregulated in human osteosarcoma and regulates cell proliferation, migration and invasion. Oncol. Rep. 39, 491–500. doi: 10.3892/or.2017.6142
Lu, K. H., Lin, R. C., Yang, J. S., Yang, W. E., Reiter, R. J., and Yang, S. F. (2019). Molecular and Cellular Mechanisms of Melatonin in Osteosarcoma. Cells 8:1618. doi: 10.3390/cells8121618
Lu, S., Sun, Z., Tang, L., and Chen, L. (2020). LINC00355 Promotes Tumor Progression in HNSCC by Hindering MicroRNA-195-Mediated Suppression of HOXA10 Expression. Mol. Ther. Nucleic Acids 19, 61–71. doi: 10.1016/j.omtn.2019.11.002
Manandhar, S., and Lee, Y. M. (2018). Emerging role of RUNX3 in the regulation of tumor microenvironment. BMB Rep. 51, 174–181. doi: 10.5483/bmbrep.2018.51.4.033
Meszaros, J. G., and Karin, N. J. (1995). Inhibitors of ER Ca(2+)-ATPase activity deplete the ATP- and thrombin-sensitive Ca2+ pool in UMR 106-01 osteosarcoma cells. J. Bone Miner. Res. 10, 704–710. doi: 10.1002/jbmr.5650100506
Nadjmi, M., Hofmann, E., Ratzka, M., and Schuknecht, B. (1987). [Tinnitus and its radiological diagnosis and therapy]. Rofo 146, 335–341. doi: 10.1055/s-2008-1048493
Negri, G. L., Grande, B. M., Delaidelli, A., El-Naggar, A., Cochrane, D., Lau, C. C., et al. (2019). Integrative genomic analysis of matched primary and metastatic pediatric osteosarcoma. J. Pathol. 249, 319–331. doi: 10.1002/path.5319
Ni, W., Yao, S., Zhou, Y., Liu, Y., Huang, P., Zhou, A., et al. (2019). Long noncoding RNA GAS5 inhibits progression of colorectal cancer by interacting with and triggering YAP phosphorylation and degradation and is negatively regulated by the m(6)A reader YTHDF3. Mol. Cancer 18:143. doi: 10.1186/s12943-019-1079-y
Pan, Z., Liu, L., Nie, W., Miggin, S., Qiu, F., Cao, Y., et al. (2018). Long non-coding RNA AGER-1 functionally upregulates the innate immunity gene AGER and approximates its anti-tumor effect in lung cancer. Mol. Carcinog. 57, 305–318. doi: 10.1002/mc.22756
Perike, S., Özkucur, N., Sharma, P., Staroske, W., Bläsche, R., Barth, K., et al. (2014). Phospho-NHE3 forms membrane patches and interacts with beta-actin to sense and maintain constant direction during cell migration. Exp. Cell Res. 324, 13–29. doi: 10.1016/j.yexcr.2014.03.005
Pruksakorn, D., Klangjorhor, J., Lirdprapamongkol, K., Teeyakasem, P., Sungngam, P., Chaiyawat, P., et al. (2018). Oncogenic roles of serine-threonine kinase receptor-associated protein (STRAP) in osteosarcoma. Cancer Chemother. Pharmacol. 82, 1039–1047. doi: 10.1007/s00280-018-3696-3
Pungpapong, V., Zhang, M., and Zhang, D. (2020). Integrating Biological Knowledge Into Case-Control Analysis Through Iterated Conditional Modes/Medians Algorithm. J. Comput. Biol. 27, 1171–1179. doi: 10.1089/cmb.2019.0319
Ravanan, P., Srikumar, I. F., and Talwar, P. (2017). Autophagy: The spotlight for cellular stress responses. Life Sci. 188, 53–67. doi: 10.1016/j.lfs.2017.08.029
Song, Z., Zhang, X., Lin, Y., Wei, Y., Liang, S., and Dong, C. (2019). LINC01133 inhibits breast cancer invasion and metastasis by negatively regulating SOX4 expression through EZH2. J. Cell Mol. Med. 23, 7554–7565. doi: 10.1111/jcmm.14625
Steven, A., and Seliger, B. (2018). The Role of Immune Escape and Immune Cell Infiltration in Breast Cancer. Breast Care 13, 16–21. doi: 10.1159/000486585
Sun, X., Wei, B., Peng, Z. H., Fu, Q. L., Wang, C. J., Zheng, J. C., et al. (2019). Knockdown of lncRNA XIST suppresses osteosarcoma progression by inactivating AKT/mTOR signaling pathway by sponging miR-375-3p. Int. J. Clin. Exp. Pathol. 12, 1507–1517.
Tian, X., He, Y., Han, Z., Su, H., and Chu, C. (2019). The Cytoplasmic Expression Of CLDN12 Predicts An Unfavorable Prognosis And Promotes Proliferation And Migration Of Osteosarcoma. Cancer Manag. Res. 11, 9339–9351. doi: 10.2147/CMAR.S229441
Tu, Y., Wan, Y. G., Gu, Y. H., Liu, B. H., Liu, Y. L., Wang, W. W., et al. (2019). [Molecular mechanisms of non-coding RNA regulating autophagy and interventional effects of Chinese herbal medicine]. Zhongguo Zhong Yao Za Zhi 44, 4545–4551. doi: 10.19540/j.cnki.cjcmm.20190812.401
Tullius, B. P., Setty, B. A., and Lee, D. A. (2020). Natural Killer Cell Immunotherapy for Osteosarcoma. Adv. Exp. Med. Biol. 1257, 141–154. doi: 10.1007/978-3-030-43032-0_12
Wang, C. Z., Yan, G. X., Dong, D. S., Xin, H., and Liu, Z. Y. (2019). LncRNA-ATB promotes autophagy by activating Yes-associated protein and inducing autophagy-related protein 5 expression in hepatocellular carcinoma. World J. Gastroenterol. 25, 5310–5322. doi: 10.3748/wjg.v25.i35.5310
Wang, J., Liu, S., Shi, J., Li, J., Wang, S., Liu, H., et al. (2019). The Role of miRNA in the Diagnosis, Prognosis, and Treatment of Osteosarcoma. Cancer Biother. Radiopharm. 34, 605–613. doi: 10.1089/cbr.2019.2939
Wang, S. D., Li, H. Y., Li, B. H., Xie, T., Zhu, T., Sun, L. L., et al. (2016). The role of CTLA-4 and PD-1 in anti-tumor immune response and their potential efficacy against osteosarcoma. Int. Immunopharmacol. 38, 81–89. doi: 10.1016/j.intimp.2016.05.016
Wang, Y., Sun, L., Wang, L., Liu, Z., Li, Q., Yao, B., et al. (2018). Long non-coding RNA DSCR8 acts as a molecular sponge for miR-485-5p to activate Wnt/β-catenin signal pathway in hepatocellular carcinoma. Cell Death Dis. 9:851. doi: 10.1038/s41419-018-0937-7
Wedekind, M. F., Wagner, L. M., and Cripe, T. P. (2018). Immunotherapy for osteosarcoma: Where do we go from here? Pediatr. Blood Cancer 65:e27227. doi: 10.1002/pbc.27227
Wei, R., Thanindratarn, P., Dean, D. C., Hornicek, F. J., Guo, W., and Duan, Z. (2020). Cyclin E1 is a prognostic biomarker and potential therapeutic target in osteosarcoma. J. Orthop. Res. 38, 1952–1964. doi: 10.1002/jor.24659
Wong, S. Q., Kumar, A. V., Mills, J., and Lapierre, L. R. (2020). Autophagy in aging and longevity. Hum. Genet. 139, 277–290. doi: 10.1007/s00439-019-02031-7
Yang, Z., Zhang, Y., Zhang, X., Zhang, M., Liu, H., Zhang, S., et al. (2015). Serum microRNA-221 functions as a potential diagnostic and prognostic marker for patients with osteosarcoma. Biomed. Pharmacother. 75, 153–158. doi: 10.1016/j.biopha.2015.07.018
You, Q., Yao, Y., Wu, J., Cheng, C., Li, Y., and Yuan, H. (2020). YY1-induced lncRNA DSCR8 promotes the progression of ovarian cancer via miR-3192-5p/YY1 axis. Biomed. Pharmacother. 129:110339. doi: 10.1016/j.biopha.2020.110339
Yu, G., Wang, L. G., Han, Y., and He, Q. Y. (2012). clusterProfiler: an R package for comparing biological themes among gene clusters. OMICS 16, 284–287. doi: 10.1089/omi.2011.0118
Zhang, F., Yan, T., Guo, W., Sun, K., Wang, S., Bao, X., et al. (2018). Novel oncogene COPS3 interacts with Beclin1 and Raf-1 to regulate metastasis of osteosarcoma through autophagy. J. Exp. Clin. Cancer Res. 37:135. doi: 10.1186/s13046-018-0791-6
Zhang, F., Zeng, L., Cai, Q., Xu, Z., Liu, R., Zhong, H., et al. (2020). Comprehensive Analysis of a Long Noncoding RNA-Associated Competing Endogenous RNA Network in Wilms Tumor. Cancer Control. 27:1148372255. doi: 10.1177/1073274820936991
Zhao, G. S., Gao, Z. R., Zhang, Q., Tang, X. F., Lv, Y. F., Zhang, Z. S., et al. (2018). TSSC3 promotes autophagy via inactivating the Src-mediated PI3K/Akt/mTOR pathway to suppress tumorigenesis and metastasis in osteosarcoma, and predicts a favorable prognosis. J. Exp. Clin. Cancer Res. 37:188. doi: 10.1186/s13046-018-0856-6
Zhao, Z., Jia, Q., Wu, M. S., Xie, X., Wang, Y., Song, G., et al. (2018). Degalactotigonin, a Natural Compound from Solanum nigrum L., Inhibits Growth and Metastasis of Osteosarcoma through GSK3β Inactivation-Mediated Repression of the Hedgehog/Gli1 Pathway. Clin. Cancer Res. 24, 130–144. doi: 10.1158/1078-0432.CCR-17-0692
Zheng, Z. Q., Li, Z. X., Zhou, G. Q., Lin, L., Zhang, L. L., Lv, J. W., et al. (2019). Long Noncoding RNA FAM225A Promotes Nasopharyngeal Carcinoma Tumorigenesis and Metastasis by Acting as ceRNA to Sponge miR-590-3p/miR-1275 and Upregulate ITGB3. Cancer Res. 79, 4612–4626. doi: 10.1158/0008-5472.CAN-19-0799
Keywords: osteosarcoma, autophagy-related lncRNA, prognostic signature, survival, immune cell infiltration
Citation: Zhang G-Z, Wu Z-L, Li C-Y, Ren E-H, Yuan W-H, Deng Y-J and Xie Q-Q (2021) Development of a Machine Learning-Based Autophagy-Related lncRNA Signature to Improve Prognosis Prediction in Osteosarcoma Patients. Front. Mol. Biosci. 8:615084. doi: 10.3389/fmolb.2021.615084
Received: 22 October 2020; Accepted: 29 March 2021;
Published: 21 May 2021.
Edited by:
Umberto Malapelle, University of Naples Federico II, ItalyReviewed by:
Rossella Tufano, University of Naples Federico II, ItalyMauro Buono, University of Naples Federico II, Italy
Copyright © 2021 Zhang, Wu, Li, Ren, Yuan, Deng and Xie. This is an open-access article distributed under the terms of the Creative Commons Attribution License (CC BY). The use, distribution or reproduction in other forums is permitted, provided the original author(s) and the copyright owner(s) are credited and that the original publication in this journal is cited, in accordance with accepted academic practice. No use, distribution or reproduction is permitted which does not comply with these terms.
*Correspondence: Qi-Qi Xie, jieqq16@lzu.edu.cn
†These authors have contributed equally to this work