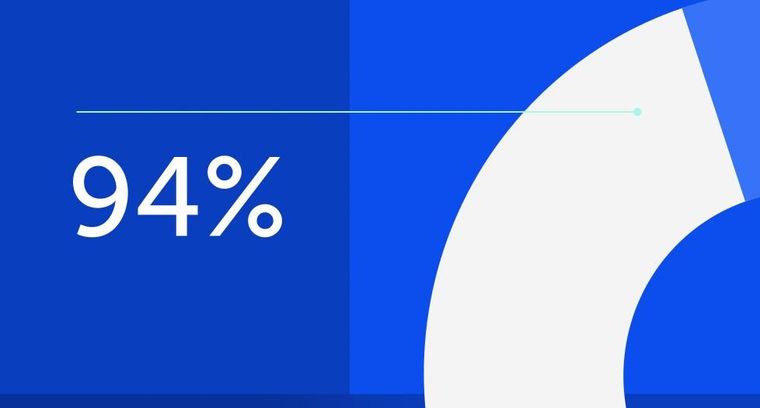
94% of researchers rate our articles as excellent or good
Learn more about the work of our research integrity team to safeguard the quality of each article we publish.
Find out more
SYSTEMATIC REVIEW article
Front. Microbiomes, 06 March 2025
Sec. Nutrition, Metabolism and the Microbiome
Volume 4 - 2025 | https://doi.org/10.3389/frmbi.2025.1506387
Diabetes mellitus is a prevalent chronic non-communicable disease, and recent studies have explored the link between gut microbiota and its development. Despite some evidence suggesting an association, the influence of gut microbiota on type 2 diabetes (T2D) remains unclear. A systematic search of PubMed (January 2016– December 2023) using the keywords “16S” and “diabetes” or “DM2” or “T2DM” or “T2D” and “gut microbiota” and “diabetes” or “DM2” or “T2DM” or “T2D”. The studies included compared gut microbiome diversity between diabetic and non-diabetic adults using 16S rRNA sequencing, excluding children, interventions, and type 1 diabetes. Alpha diversity indices and bacterial mean abundance were analyzed, with statistical assessments using a random-effects model and I2 for heterogeneity. Thirteen studies met the criteria, with the Shannon index being the most commonly used measure. Results showed significant heterogeneity (I2 > 75%) and no notable differences between diabetic and non-diabetic groups. Other indices, such as Chao1 and phylogenetic whole tree, similarly showed no consistent differences. Taxonomic analysis also failed to find phyla consistently correlated with T2D, with variability across studies. The relationship between gut microbiota and diabetes remains uncertain due to technical and biological factors that are often overlooked. The inconsistencies across studies highlight the low reproducibility common in microbiota research.
As global populations increasingly adopt urbanized lifestyles, the prevalence of chronic non-communicable diseases, such as diabetes mellitus (DM), has become a significant public health concern, particularly in low- and middle-income countries (World Health Organization, 2023). Type 2 diabetes (T2D), which constitutes approximately 90% of all diabetes cases, is estimated to affect over 500 million adults worldwide, representing a substantial and increasingly significant economic burden (International Diabetes Federation, 2021; Ong et al., 2023). Beyond its impact on glucose regulation, T2D is a major risk factor for cardiovascular diseases, which remain the leading cause of death globally (World Health Organization, 2024).
T2D is a chronic condition marked by the reduced ability of the pancreas to produce insulin or the decreased effectiveness of insulin, leading to persistent hyperglycemia (World Health Organization, 2024). This multifactorial disease is influenced by genetic predisposition, environmental factors, and, more recently, alterations in the gut microbiome (Gilbert et al., 2018; Qin et al., 2012).
Numerous studies have proposed a role for the gut microbiome in the pathophysiology of T2D, attributed to its influence on host metabolic homeostasis. The gut microbiota contributes to maintaining the integrity of the epithelial barrier, maturing the immune system, and producing a variety of metabolites that exert systemic effects on the host (Bäckhed et al., 2012; Rogers and Wesselingh, 2016). Furthermore, reports have shown that microbial metabolization of dietary nutrients affects the energetic yield within the host, potentially contributing to the onset of obesity and pre-diabetes (Takeuchi et al., 2023). This process suggests a possible involvement of the microbiome in metabolic disorders by influencing insulin resistance and low-grade inflammation through the metabolism of dietary monosaccharides (Zhou et al., 2019).
The relationship between the gut microbiota and T2D, however, remains contentious, with inconsistent findings across different populations (He et al., 2018; Zhou et al., 2019). For instance, the genus Bacteroides has been reported to have both higher and lower relative abundance in diabetic patients across various studies (He et al., 2018; Yamaguchi et al., 2016). Some meta-analyses have highlighted this inconsistency, suggesting that the gut microbiome may not play a significant role in T2D development (Gurung et al., 2020; MetaHIT consortium et al., 2015). This has led to the hypothesis that it is the overall functional repertoire and metabolic output of the microbial community, rather than specific taxa, that are critical in the interaction between the microbiome and T2D Vatanen et al., 2018.
Concerns about the reproducibility of metagenomic studies, particularly in methodology, have also emerged. Notably, a highly cited article foundational to many studies was found to have methodological flaws (Gihawi et al., 2023). In response to these issues, we conducted a meta-analysis of datasets where gut microbiota, assessed through 16S rRNA gene sequencing, was studied in relation to Type 2 Diabetes Mellitus.
This systematic review and meta-analysis aimed to evaluate the relationship between the gut microbiome and type 2 diabetes mellitus (T2D) by analyzing 16S rRNA sequencing data. The study was designed to synthesize available evidence, identify patterns or discrepancies in the findings, and assess the reproducibility of results across different studies. Our approach followed the PRISMA (Preferred Reporting Items for Systematic Reviews and Meta-Analyses) guidelines to ensure a rigorous and transparent methodology.
A comprehensive and systematic search was conducted in PubMed to identify relevant studies published between January 2016 to December 2023. The search strategy combined Medical Subject Headings (MeSH) terms and keywords to capture all pertinent literature. The search string included the following terms: (“16S” AND “diabetes” OR “DM2” OR “T2DM” OR “T2D”) AND (“gut microbiota” AND “diabetes” OR “DM2” OR “T2DM” OR “T2D”). To ensure the quality and relevance of the data, only peer-reviewed articles published in English were considered. The search was complemented by manual screening of reference lists from selected studies to identify any additional relevant publications.
The selection process involved a multi-step approach. Initially, titles and abstracts were screened to eliminate studies that clearly did not meet the inclusion criteria. Full-text reviews were then conducted for studies that appeared potentially eligible. Studies were included if they met the following criteria: (1) compared gut microbiome diversity between adult diabetic and non-diabetic populations; (2) employed 16S rRNA sequencing as the primary method for microbiome analysis; and (3) were published in English. Studies were excluded based on the following criteria: (1) studies involving pediatric populations, due to differences in microbiome composition; (2) studies relying solely on quantitative PCR (qPCR) for bacterial abundance, as this method lacks the depth of 16S rRNA sequencing; (3) studies employing shotgun sequencing, which differ significantly in methodology and scope from 16S studies; and (4) studies focusing primarily on inflammatory markers or other non-microbiome-related associations with diabetes. This rigorous selection process ensured that the included studies were comparable and relevant to the research question.
Data extraction was conducted meticulously from various sources within the studies, including text, tables, and figures. Key data points extracted included: authors, year of publication, sample size per group, 16S rRNA primer sequences, DNA extraction kits used, data availability (e.g., public repositories), country of origin of the study, inclusion and exclusion criteria, statistical methods employed, mean values of alpha diversity indices, mean values of bacterial abundance, and the choice of Operational Taxonomic Units (OTUs) versus Amplicon Sequence Variants (ASVs) for sequence classification.
For studies where data were presented in figures, values were extracted using PlotDigitizer (PlotDigitizer, 2024), an image processing software that allows accurate digitization of graphical data. The extracted data were then analyzed using a mean difference test to compare alpha diversity indices and bacterial abundance between diabetic and non-diabetic groups. Subgroup analyses were conducted based on the sequencing method used (OTU vs. ASV) to explore potential differences in findings related to methodological variations. A random-effects model was employed for meta-analysis, as recommended by Review Manager (Higgins and Green, 2011) version 5.4, to account for variability across studies. Statistical significance was determined at a p-value threshold of < 0.05. Heterogeneity among studies was assessed using the I2 statistic, with the following classifications: low (0%-40%), moderate (30%-60%), substantial (50%-90%), and considerable (75%-100%) (ibid.). All statistical analyses were performed using RStudio (RStudio Team, 2024), with R version 4.3.0 (R Core Team, 2024) and the ‘meta’ package version 7.0.0 (Schwarzer et al., 2015), ensuring reproducibility and transparency of the analytical process.
The initial search identified 7140 articles. After applying the inclusion criteria and narrowing down the results, 71 articles were selected for full-text review (Figure 1). Following this thorough screening process, thirteen studies met the criteria for inclusion in the final analysis. Of these, nine studies employed Operational Taxonomic Unit (OTU) sequences, while four utilized Amplicon Sequence Variants (ASV) for microbiome analysis.
Figure 1. Flowchart representing inclusion and exclusion criteria and resulting article number after exclusion.
Geographically, the majority of the studies were conducted in Asia, with eleven originating from this continent (China: 7, Japan: 1, Pakistan: 2). Two studies were conducted in North America (USA: 2), and one study was from the Middle East (Egypt: 1). Regarding taxonomic classification, the reference databases most frequently used were GreenGenes and SILVA, with each being utilized in four studies. The V3V4 region of the 16S rRNA gene was the most commonly targeted region for primer production, appearing in eight studies (Table 1). Across all included studies, a total of 4,066 sequenced samples were analyzed, providing a robust dataset for the meta-analysis.
The majority of the studies included in this meta-analysis (n = 11) utilized the Shannon index to evaluate alpha diversity between control and diabetic groups. As illustrated in Figure 2, there is substantial heterogeneity among the studies (I2 > 75%), suggesting that multiple factors contribute to the observed variability in alpha diversity results. This high heterogeneity indicates that the results are influenced by differences in study design, population characteristics, sequencing methods, or data analysis techniques.
Figure 2. Forest plot of Shannon index in normoglycemic vs. diabetic subjects. Stratified by OTU and ASV identification methods.
Additionally, the results show considerable variation in the Shannon index across studies, as reflected by the wide confidence intervals and the non-significant p-value, which suggests no consistent difference in alpha diversity between diabetic and non-diabetic groups. When the studies were stratified into subgroups based on the identification method, it became evident that studies using the OTU approach exhibited greater heterogeneity compared to those employing the ASV method. The relatively low variability among ASV-based studies could be partly due to the smaller number of studies in this subgroup (only three), which may limit the generalizability of these findings.
When the Chao1 index data from all included studies were analyzed using a forest plot, substantial heterogeneity was observed, with I2 values falling within the range of 50% to 75% (Figure 3). This suggests that while there is notable variability among studies, it is not extreme.
Figure 3. Forest plot of Chao1 index in normoglycemic vs. diabetic subjects. Stratified by OTU and ASV identification methods.
In four studies, higher alpha diversity was reported in diabetic individuals when OTUs were used for analysis. This trend was similarly observed in the ASV data, where two studies indicated an increased Chao1 index in diabetes, although the results were not consistent.
A specific subgroup of three studies that employed the OTU method exhibited substantial heterogeneity (I2 < 75%) and showed statistically significant variation (p-value < 0.01). However, despite this variation, no significant difference was found between the diabetic and non-diabetic groups within this subgroup.
Five studies included in the analysis utilized the phylogenetic whole tree index to evaluate alpha diversity (Figure 4). This index showed substantial variation, with heterogeneity ranging from 50% to 90% (I2), suggesting notable variability across studies. Despite this, the overall p-value was significant (p < 0.01), indicating that there was no significant difference in phylogenetic distances between diabetic and non-diabetic groups. Among these studies, only one employed the ASV method, while the remaining four used the OTU method. The studies using the OTU method exhibited higher heterogeneity compared to the combined analysis of all five studies. Despite these methodological differences, none of the indices showed a significant difference in alpha diversity between the diabetic and non-diabetic groups.
Figure 4. Forest plot of phylogenetic whole tree index in normoglycemic vs diabetic subjects. Stratified by OTU and ASV identification methods.
The analysis aimed to determine whether diabetic individuals have a distinct abundance of specific phyla compared to non-diabetic individuals. However, no clear trend was observed across the studies. Significant heterogeneity was evident (I2 > 75%), highlighting the diversity in the collected data. This variability suggests that the underlying factors contributing to differences in phylum abundance remain unclear and require further investigation.
Among the 13 studies analyzed, four phyla were frequently associated with diabetes, each showing considerable variation (I2 > 75%) and significant p-values (p < 0.05) (Figure 5). This disparity underscores the need for additional research to better understand these associations.
Pseudomonadota was the most commonly reported phylum, appearing in six studies. Despite its frequent mention, there was no consensus on its relationship with diabetes. The studies showed high heterogeneity (I2 = 94%), and no significant differences were found between normoglycemic and diabetic individuals concerning Pseudomonadota abundance. Furthermore, factors such as dietary habits and population characteristics, which may influence microbiota composition, have not been thoroughly investigated in this context.
Bacteroidota was the second most commonly associated phylum, mentioned in five studies. It was the only phylum with heterogeneity below 90%. The findings were mixed: Ahmad et al. (2019) and Du et al. (2022) reported higher Bacteroidota abundance in diabetic individuals, while Hashimoto et al. (2020) and Walker et al. (2021) found lower levels in diabetics. The confidence intervals and p-values suggest that there is no clear association between Bacteroidota abundance and diabetes.
Bacillota and Actinomycetota were each associated with diabetes in four studies. Bacillota exhibited a large confidence interval, mainly due to the findings in Ahmad et al. (2019) which indicated a significant difference in abundance between groups. However, the other three studies did not support this result. As for Actinomycetota, although slight variations in means were observed, the p-values and confidence intervals indicate no significant relationship between its abundance and diabetes.
The association between complex traits such as Type 2 Diabetes Mellitus (T2D) and gut microbiota has been extensively proposed in the literature (Larsen et al., 2010; Baothman et al., 2016; Doumatey et al., 2020). However, our analysis reveals no significant differences in alpha diversity between normoglycemic and diabetic groups. This outcome is likely influenced by the substantial heterogeneity observed across the studies, suggesting that variations in results may be driven by multiple factors beyond microbial diversity, including methodological differences, personal eating habits, and population characteristics.
A key methodological factor is the choice between OTU and ASV approaches, with most studies favoring OTUs (n = 8). The OTU method, while common, is prone to replication issues due to its reliance on clustering algorithms, potentially merging different sequences into the same cluster. On the other hand, the ASV method, particularly when using the DADA2 workflow, offers more precise sequence identification through machine-learning algorithms and stricter merging criteria. Studies have shown that these methodological differences can lead to varying alpha diversity values even when analyzing the same dataset (Joos et al., 2020; Chiarello et al., 2022). Our results suggest that the lack of significant findings may stem from these methodological disparities, underscoring the need for standardized approaches in microbiome research.
Another critical factor is the sequencing depth, which can significantly impact alpha diversity indices. Indices like Shannon and Simpson’s are relatively robust, but Chao1, which was frequently used in these studies, is more sensitive to sequencing depth variations. This sensitivity might contribute to the observed variability, particularly when comparing OTU and ASV methods (Chiarello et al., 2022; Ramakodi, 2021). Additionally, the small sample sizes in most studies (10 to 40 individuals per group) may not accurately capture the true microbial diversity, introducing another layer of bias.
When evaluating taxonomic composition, our findings indicate no consistent differences in gut microbiota between diabetic and non-diabetic individuals, despite individual studies reporting differential abundances. The choice of reference databases, such as the outdated Greengenes (DeSantis et al., 2006; Bolyen et al., 2019) or the more recent Silva (Quast et al., 2012) can introduce significant variation in taxonomic identification, leading to inconsistent results. This lack of standardization highlights a major challenge in microbiome research, where the diversity of reference databases and methodological approaches creates noise and complicates the interpretation of findings.
The reported alterations in specific phyla, such as Pseudomonadota and Bacteroidota, also exhibit significant heterogeneity (I2 > 75%), suggesting that these findings are not reproducible across studies. For example, Proteobacteria, although frequently associated with diabetes, showed no consistent pattern of alteration, likely due to methodological differences and unconsidered confounding factors such as diet and population-specific characteristics. Similarly, Bacteroidota, despite being the second most commonly reported phylum, showed conflicting results across studies, further emphasizing the need for standardized methodologies.
The limited statistical power of alpha diversity indices in characterizing gut microbiota is another important consideration. The inherent inter-individual variability in gut microbiome studies necessitates larger sample sizes to achieve reliable assessments (He et al., 2018; Kers and Saccenti, 2022; Rothschild et al., 2018). Most studies analyzed here did not account for this variability adequately, leading to potential biases. The lack of consistent exclusion criteria, such as accounting for recent diarrhea or constipation, can further exacerbate the heterogeneity observed in microbial diversity and abundance (Vandeputte et al., 2016; Park et al., 2024).
Lifestyle factors, often overlooked in these studies, play a crucial role in shaping the gut microbiome. Recent evidence suggests that microbiota variations are more strongly associated with diet and environmental factors than with disease status alone (He et al., 2018; Trefflich et al., 2020; Gihawi et al., 2023). This perspective aligns with our findings, which indicate that diabetes alone is insufficient to explain the observed microbiota variation. Comprehensive analyses that consider multiple variables are essential for a more accurate understanding of microbiome dynamics.
Finally, the application of 16S rRNA sequencing to human samples presents unique challenges, as even minor environmental differences can lead to significant microbiome variations (Zuniga-Chaves et al., 2023). Detailed patient metadata, including dietary habits, stool consistency, and other health conditions, should be a standard inclusion in microbiome studies to improve the reproducibility and interpretability of results. Moreover, integrating metabolic biomarkers with microbiota data may offer more insights into diabetes-related variations than microbiota analysis alone (Yan et al., 2023; Gihawi et al., 2023).
In conclusion, the reproducibility issues observed in gut microbiota research related to Type 2 diabetes highlight the need for standardized methodologies, comprehensive biological data, and careful consideration of confounding factors. Addressing these challenges is crucial for advancing our understanding of the complex interplay between gut microbiota and metabolic diseases. Our analysis reveals that the observed inconsistencies across studies on gut microbiota and type 2 diabetes (T2D) are likely influenced by methodological differences, particularly in taxonomic identification and reference database selection. To enhance reproducibility in future research, it’s crucial to standardize methodologies and incorporate comprehensive patient metadata, including dietary habits and stool consistency. Additionally, applying advanced statistical techniques, such as bootstrapping, can simulate subpopulations and assess the consistency of findings across these subgroups, offering a more robust understanding of the microbiome’s role in T2D. By addressing these variables and adopting more rigorous statistical approaches, the field can move toward more reliable and reproducible results in microbiome research.
The original contributions presented in the study are included in the article/supplementary material. Further inquiries can be directed to the corresponding author.
JM: Conceptualization, Data curation, Methodology, Writing – original draft, Writing – review & editing. AS: Writing – original draft, Writing – review & editing. IM: Writing – review & editing. GF: Writing – review & editing.
The author(s) declare that financial support was received for the research, authorship, and/or publication of this article. This study was partially funded by the Coordenação de Aperfeiçoamento de Pessoal de Nível Superior – Brasil (CAPES) – Finance Code 001 and by the Fundação de Amparo à Pesquisa do Estado de Minas Gerais (FAPEMIG) – APQ-03423-18.
JM and GF concept the idea. JM contributed to data acquisition. JM and AS wrote the manuscript. JM, AS, IM, and GF edited, and draft approved the final version.
The authors declare that the research was conducted in the absence of any commercial or financial relationships that could be construed as a potential conflict of interest.
The author(s) declare that no Generative AI was used in the creation of this manuscript.
All claims expressed in this article are solely those of the authors and do not necessarily represent those of their affiliated organizations, or those of the publisher, the editors and the reviewers. Any product that may be evaluated in this article, or claim that may be made by its manufacturer, is not guaranteed or endorsed by the publisher.
Ahmad A., Yang W., Chen G., Shafiq M., Javed S., Ali Zaidi S. S., et al. (2019). Analysis of gut microbiota of obese individuals with type 2 diabetes and healthy individuals. PloS One 14, 12. doi: 10.1371/journal.pone.0226372
Bäckhed F., Fraser C. M., Ringel Y., Sanders M. E., Sartor R. B., Sherman P. M., et al. (2012). Defining a healthy human gut microbiome: current concepts, future directions, and clinical applications. Cell Host Microbe 12, 611–622. doi: 10.1016/j.chom.2012.10.012
Baothman O. A., Zamzami M. A., Taher I., Abubaker J., Abu-Farha M. (2016). The role of Gut Microbiota in the development of obesity and Diabetes. Lipids Health Dis. 15, 108. doi: 10.1186/s12944-016-0278-4
Bolyen E., Rideout J. R., Dillon M. R., Bokulich N. A., Abnet C. C., Al-Ghalith G. A., et al. (2019). Reproducible, interactive, scalable and extensible microbiome data science using QIIME 2. Nat. Biotechnol. 37, 852–857. doi: 10.1038/s41587-019-0209-9
Chiarello M., McCauley M., Villéger S., Jackson C. R. (2022). Ranking the biases: The choice of OTUs vs. ASVs in 16S rRNA amplicon data analysis has stronger effects on diversity measures than rarefaction and OTU identity threshold. PloS One 17, e0264443. doi: 10.1371/journal.pone.0264443
DeSantis T. Z., Hugenholtz P., Larsen N., Rojas M., Brodie E. L., Keller K., et al. (2006). Greengenes, a chimera-checked 16S rRNA gene database and workbench compatible with ARB. Appl. Environ. Microbiol. 72, 5069–5072. doi: 10.1128/AEM.03006-05
Ding H., Xu Y., Cheng Y., Zhou H., Dong S., Wu J., et al. (2023). Gut microbiome profile of Chinese hypertension patients with and without type 2 diabetes mellitus. BMC Microbiol. 23, 254. doi: 10.1186/s12866-023-02967-x
Doumatey A. P., Adeyemo A., Zhou J., Lei L., Adebamowo S. N., Adebamowo C., et al. (2020). Gut microbiome profiles are associated with type 2 diabetes in urban africans. Front. Cell. Infect. Microbiol. 10. doi: 10.3389/fcimb.2020.00063
Du Y., Li X., An Y., Song Y., Lu Y. (2022). Association of gut microbiota with sort-chain fatty acids and inflammatory cytokines in diabetic patients with cognitive impairment: A cross-sectional, non-controlled study. Front. Nutr. 9. doi: 10.3389/fnut.2022.930626
Gihawi A., Ge Y., Lu J., Puiu D., Xu A., Cooper C. S., et al. (2023). Major data analysis errors invalidate cancer microbiome findings. mBio 14, e01607-23. doi: 10.1128/mbio.01607-23
Gilbert J. A., Blaser M. J., Caporaso J. G., Jansson J. K., Lynch S. V., Knight R. (2018). Current understanding of the human microbiome. Nat. Med. 24, 392–400. doi: 10.1038/nm.4517
Guo X., Dai S., Lou J., Ma X., Hu X., Tu L., et al. (2023). Distribution characteristics of oral microbiota and its relationship with intestinal microbiota in patients with type 2 diabetes mellitus. Front. Endocrinol. 14, 1119201. doi: 10.3389/fendo.2023.1119201
Gurung M., Li Z., You H., Rodrigues R., Jump D. B., Morgun A., et al. (2020). Role of gut microbiota in type 2 diabetes pathophysiology. EBioMedicine 51, 102590. doi: 10.1016/j.ebiom.2019.11.051
Hashimoto Y., Hamaguchi M., Kaji A., Sakai R., Osaka T., Inoue R., et al. (2020). Intake of sucrose affects gut dysbiosis in patients with type 2 diabetes. J. Diabetes Invest. 11, 1623–1634. doi: 10.1111/jdi.13293
He Y., Wu W., Zheng H.-M., Li P., McDonald D., Sheng H.-F., et al. (2018). Regional variation limits applications of healthy gut microbiome reference ranges and disease models. Nat. Med. 24, 1532–1535. doi: 10.1038/s41591-018-0164-x
Higgins J. P. T., Green S. (2011). Cochrane Handbook for Systematic Reviews of Interventions Version 5.1.0 [updated 2022] (The Cochrane Collaboration). Available online at: https://training.cochrane.org/handbook (Accessed 2024-09-15).
Huang S., Shao L., Chen M., Wang L., Liu J., Zhang W., et al. (2023). Biotransformation differences of ginsenoside compound K mediated by the gut microbiota from diabetic patients and healthy subjects. Chin. J. Natural Medicines 21, 723–729. doi: 10.1016/S1875-5364(23)60402-9
International Diabetes Federation (2021). IDF Diabetes Atlas. 10th edn (International Diabetes Federation). Available online at: https://www.diabetesatlas.org (Accessed June 12, 2024).
Joos L., Beirinckx S., Haegeman A., Debode J., Vandecasteele B., Baeyen S., et al. (2020). Daring to be differential: metabarcoding analysis of soil and plant-related microbial communities using amplicon sequence variants and operational taxonomical units. BMC Genomics 21, 733. doi: 10.1186/s12864-020-07126-4
Kers J. G., Saccenti E. (2022). The power of microbiome studies: some considerations on which alpha and beta metrics to use and how to report results. Front. Microbiol. 12. doi: 10.3389/fmicb.2021.796025
Larsen N., Vogensen F. K., Van Den Berg F. W.J., Nielsen D. S., Andreasen A. S., Pedersen B. K., et al. (2010). Gut microbiota in human adults with type 2 diabetes differs from non-diabetic adults. PloS One 5, e9085. doi: 10.1371/journal.pone.0009085
Li Q., Chang Y., Zhang K., Chen H., Tao S., Zhang Z. (2020). Implication of the gut microbiome composition of type 2 diabetic patients from northern China. Sci. Rep. 10, 5450. doi: 10.1038/s41598-020-62224-3
Maskarinec G., Raquinio P., Kristal B. S., Setiawan V. W., Wilkens L. R., Franke A. A., et al. (2021). The gut microbiome and type 2 diabetes status in the Multiethnic Cohort. PloS One 16, e0250855. doi: 10.1371/journal.pone.0250855
MetaHIT consortium, , Forslund K., Hildebrand F., Nielsen T., Falony G., Le Chatelier E., et al. (2015). Disentangling type 2 diabetes and metformin treatment signatures in the human gut microbiota. Nature 528, 262–266. doi: 10.1038/nature15766
Ong K. L., Stafford L. K., McLaughlin S. A., Boyko E. J., Vollset S. E., Smith A. E., et al. (2023). Global, regional, and national burden of diabetes from 1990 to 2021, with projections of prevalence to 2050: a systematic analysis for the Global Burden of Disease Study 2021. Lancet 402, 203–234. doi: 10.1016/S0140-6736(23)01301-6
Park G., Kim S., Lee W., Kim G., Shin H. (2024). Deciphering the impact of defecation frequency on gut microbiome composition and diversity. Int. J. Mol. Sci. 25, 4657. doi: 10.3390/ijms25094657
PlotDigitizer (2024). PlotDigitizer. Available online at: https://plotdigitizer.com/app (Accessed 2024-09-15).
Qin J., Li Y., Cai Z., Li S., Zhu J., Zhang F., et al. (2012). A metagenome-wide association study of gut microbiota in type 2 diabetes. Nature 490, 55–60. doi: 10.1038/nature11450
Quast C., Pruesse E., Yilmaz P., Gerken J., Schweer T., Yarza P., et al. (2012). The SILVA ribosomal RNA gene database project: improved data processing and web-based tools. Nucleic Acids Res. 41, D590–D596. doi: 10.1093/nar/gks1219
Ramakodi M. P. (2021). Effect of amplicon sequencing depth in environmental microbiome research. Curr. Microbiol. 78, 1026–1033. doi: 10.1007/s00284-021-02345-8
R Core Team (2024). R: A Language and Environment for Statistical Computing (Vienna, Austria: R Foundation for Statistical Computing). Available online at: https://www.R-project.org (Accessed 2024-09-15).
Rogers G. B., Wesselingh S. (2016). Precision respiratory medicine and the microbiome. Lancet Respir. Med. 4, 73–82. doi: 10.1016/S2213-2600(15)00476-2
Rothschild D., Weissbrod O., Barkan E., Kurilshikov A., Korem T., Zeevi D., et al. (2018). Environment dominates over host genetics in shaping human gut microbiota. Nature 555, 210–215. doi: 10.1038/nature25973
RStudio Team (2024). RStudio: Integrated Development for R (PBC Boston, MA: RStudio). Available online at: http://www.rstudio.com (Accessed 2024-09-15).
Salah M., Azab M., Ramadan A., Hanora A. (2019). New insights on obesity and diabetes from gut microbiome alterations in Egyptian adults. OMICS.: A. J. Integr. Biol. 23, 477–485. doi: 10.1089/omi.2019.0063
Saleem A., Ikram A., Dikareva E., Lahtinen E., Matharu D., Pajari A.-M., et al. (2022). Unique Pakistani gut microbiota highlights population-specific microbiota signatures of type 2 diabetes mellitus. Gut. Microbes 14, 2142009. doi: 10.1080/19490976.2022.2142009
Schwarzer G., Carpenter J. R., Rücker G. (2015). Meta-analysis with R en. Use R! (Cham: Springer International Publishing), ISBN: ISBN: 978-3-319-21415-3 978-3-319-21416-0. doi: 10.1007/978-3-319-21416-0
Takeuchi T., Kubota T., Nakanishi Y., Tsugawa H., Suda W., Kwon A. T.-J., et al. (2023). Gut microbial carbohydrate metabolism contributes to insulin resistance. Nature 621, 389–395. doi: 10.1038/s41586-023-06466-x
Trefflich I., Jabakhanji A., Menzel J., Blaut M., Michalsen A., Lampen A., et al. (2020). Is a vegan or a vegetarian diet associated with the microbiota composition in the gut? Results of a new cross-sectional study and systematic review. Crit. Rev. Food Sci. Nutr. 60, 2990–3004. doi: 10.1080/10408398.2019.1676697
Vandeputte D., Falony G., Vieira-Silva S., Tito R. Y., Joossens M., Raes J. (2016). Stool consistency is strongly associated with gut microbiota richness and composition, enterotypes and bacterial growth rates. Gut 65, 57–62. doi: 10.1136/gutjnl-2015-309618
Vatanen T., Franzosa E. A., Schwager R., Tripathi S., Arthur T. D., Vehik K., et al. (2018). The human gut microbiome in early-onset type 1 diabetes from the TEDDY study. Nature 562, 589–594. doi: 10.1038/s41586-018-0620-2
Walker R. L., Vlamakis H., Lee J. W.J., Besse L. A., Xanthakis V., Vasan R. S., et al. (2021). Population study of the gut microbiome: associations with diet, lifestyle, and cardiometabolic disease. Genome Med. 13, 188. doi: 10.1186/s13073-021-01007-5
Wang Y., Luo X., Mao X., Tao Y., Ran X., Zhao H., et al. (2017). Gut microbiome analysis of type 2 diabetic patients from the Chinese minority ethnic groups the Uygurs and Kazaks. PloS One 12, e0172774. doi: 10.1371/journal.pone.0172774
Wang J., Li W., Wang C., Wang L., He T., Hu H., et al. (2020). Enterotype bacteroides Is associated with a high risk in patients with diabetes: a pilot study. J. Diabetes Res. 2020, 1–11. doi: 10.1155/2020/6047145
World Health Organization (2024). Cardiovascular diseases - World Health Organization (WHO). Available online at: https://www.who.int/news-room/fact-sheets/detail/cardiovascular-diseases-(cvds) (Accessed 2024-06-21).
World Health Organization (2023). Diabetes - World Health Organization (WHO). Available online at: https://www.who.int/news-room/fact-sheets/detail/diabetes (Accessed 2023-04-05).
Yamaguchi Y., Adachi K., Sugiyama T., Shimozato A., Ebi M., Ogasawara N., et al. (2016). Association of intestinal microbiota with metabolic markers and dietary habits in patients with type 2 diabetes. Digestion 94, 66–72. doi: 10.1159/000447690
Yan M., Guo X., Ji G., Huang R., Huang D., Li Z., et al. (2023). Mechanismbased role of the intestinal microbiota in gestational diabetes mellitus: A systematic review and meta-analysis. Front. Immunol. 13. doi: 10.3389/fimmu.2022.1097853
Zhou W., Sailani M. R., Contrepois K., Zhou Y., Ahadi S., Leopold S. R., et al. (2019). Longitudinal multi-omics of host–microbe dynamics in prediabetes. Nature 569, 663–671. doi: 10.1038/s41586-019-1236-x
Keywords: gut microbiota, type 2 diabetes, methodology, meta-analysis, 16S
Citation: Machado JLP, Schaan AP, Mamede I and Fernandes GR (2025) Gut microbiota and type 2 diabetes associations: a meta-analysis of 16S studies and their methodological challenges. Front. Microbiomes 4:1506387. doi: 10.3389/frmbi.2025.1506387
Received: 05 October 2024; Accepted: 11 February 2025;
Published: 06 March 2025.
Edited by:
Ana Gomes, Universidade Católica Portuguesa, PortugalReviewed by:
Smith Etareri Evivie, University of Benin, NigeriaCopyright © 2025 Machado, Schaan, Mamede and Fernandes. This is an open-access article distributed under the terms of the Creative Commons Attribution License (CC BY). The use, distribution or reproduction in other forums is permitted, provided the original author(s) and the copyright owner(s) are credited and that the original publication in this journal is cited, in accordance with accepted academic practice. No use, distribution or reproduction is permitted which does not comply with these terms.
*Correspondence: Jésica Lígia Picanço Machado, amVzc2ljYWxpZ2lhLnBtQGdtYWlsLmNvbQ==
Disclaimer: All claims expressed in this article are solely those of the authors and do not necessarily represent those of their affiliated organizations, or those of the publisher, the editors and the reviewers. Any product that may be evaluated in this article or claim that may be made by its manufacturer is not guaranteed or endorsed by the publisher.
Research integrity at Frontiers
Learn more about the work of our research integrity team to safeguard the quality of each article we publish.