- 1Wake Forest Institute for Regenerative Medicine, Wake Forest University School of Medicine, Winston Salem, NC, United States
- 2Pathology section, Comparative Medicine, Wake Forest School of Medicine, Winston Salem, NC, United States
- 3Department of Cancer Biology, Wake Forest School of Medicine, Winston Salem, NC, United States
- 4Hypertension and Vascular Research Center, Wake Forest University School of Medicine, Winston Salem, NC, United States
- 5Division of Surgical Oncology, Wake Forest Baptist Health, Winston Salem, NC, United States
Immunotherapies are a revolutionary approach to treating cancer by utilizing the body’s immune system to target and combat cancer cells. This approach offers promising alternatives to traditional chemotherapies. Its potential to induce long-lasting remissions and specificity for cancer cells, which minimizes side effects, makes it a cutting-edge treatment with tremendous potential. With the increase of the clinical usage of immunotherapy, evidence emerges of the microbiome’s impact on both tumor growth and response to immunotherapy. The proposed involvement of the microbiome can change treatment efficacy by altering drug metabolism and reshaping the immune system response. Understanding the specific interactions between tumor cells, immune cells, and the microbiome is a critical step in the advancement of immunotherapy. To study the complex interaction between cancer immunity and the microbiome, various preclinical in vivo and in vitro models have been developed. We have recently described the use of an ex vivo preclinical model for anti-cancer treatment outcome prediction –tumor tissue equivalents (organoids). Specifically, immune-reactive tumor organoids are proposed as a novel tool for understanding how the microbiome influences cancer immunity and immunotherapy. More importantly, this platform can utilize patient samples to dissect patient-specific elements regulating cancer immune response and microbiome influence. This review presents the rationale for using the immune-reactive tumor organoids model to study the interactions between the microbiome and cancer immunotherapy. It will discuss available components of the model and analyze their interplay, summarize relevant experimental data, and assess their validity. Additionally, it explores the potential of immune-reactive organoids for personalized treatment approaches. Understanding the microbiome’s role in immunotherapy outcomes will lead to transformative cancer treatment via a simple change of diet or other microbiome manipulations. Ongoing research on microbiome-cancer interactions utilizing the described model systems will lead to innovative treatment strategies and improved patient outcomes.
1 Cancer immunology and immune therapy
The foundations of tumor immunology were established by Lloyd J. Old when he described the concepts of immunosurveillance and identified both tumor antigens and escape mechanisms (Old, 1981; Old, 1985; Kaplan et al., 1998). They demonstrated that tumors could be rejected by transferring immune cells from immunized mice into naive mice providing early evidence for cancer immunotherapy using immune cells (Old et al., 1962). These and other early discoveries paved the way to a broad array of cancer treatments that could be classified as cancer immunotherapies. Cancer immunotherapy, put simply, is a type of treatment that harnesses the power of the immune system to recognize and attack cancer cells. There are several approaches that cancer immunotherapy can utilize, and these therapies have revolutionized cancer treatment in since their introduction (Kotla et al., 2009; Kronke et al., 2015). Currently, the two main clinical therapies are checkpoint inhibitors and adoptive cell therapy (ACT).
Checkpoint inhibitors are drugs that obstruct specific proteins on immune cells or cancer cells to prevent the suppression of immune responses against tumors. Current clinically approved targets for inhibitory checkpoints include CTLA-4 and PD-1. Researchers have found that by blocking these inhibitory checkpoint proteins, immune checkpoint inhibitor antibodies effectively take the brakes off the immune system, amplifying its cancer-fighting abilities (Esfahani et al., 2020).
Adoptive cellular therapy is based on the activation and manipulation of autologous T cells in an in vitro setting followed by reinfusion to achieve better tumor targeting. This modality has three main approaches: tumor-infiltrating lymphocyte therapy (TILs), T cell receptor (TCR) gene therapy, and chimeric antigen receptor (CAR) modified T cells (June et al., 2015).
Tumor infiltrating lymphocyte (TILs) therapy involves expanding autologous TILs from resected tumors ex vivo and infusing them back into patients after lymphodepletion. In animal models, TILs from various mouse tumors have demonstrated the ability to combat tumors in vivo (Forget et al., 2018). Human patient studies by Radvanyi et al. showed TILs from melanomas reliably recognize autologous tumors (Radvanyi et al., 2012). In various phase I/II clinical trials for metastatic melanoma, TIL therapy resulted in tumor responses nearing 50% (Andersen et al., 2016). As of early 2024, the Food and Drug Administration approved Lifileucel from Iovance Biotherapeutics (Amtagvi) as the first approved solid tumor derived autologous T cell immunotherapy.
T cell receptor gene therapy (TCR) serves as another avenue for ACT, where patient T cells are reprogrammed to identify tumor antigens by introducing genes that encode tumor-specific TCRs (D’Angelo et al., 2018). One of the notable targets in this therapy includes cancer germline antigens such as NY-ESO-1. NY-ESO-1 is expressed in up to 52% of melanomas (Goydos et al., 2001), neuroblastomas (Camisaschi et al., 2018), synovial sarcomas and mixoid and round cell liposarcomas, and ovarian cancer (Robbins et al., 2015). Research has shown promising results, with response rates reaching up to 30% in melanoma cases (Robbins et al., 2015). However, there are concerns that toxicities might arise from off-tumor reactivity.
Chimeric Antigen Receptor T-cell (CAR-T) therapy is a prominent form of adoptive cell transfer (ACT), using engineered T cells to target tumor antigens through chimeric antigen receptors. These chimeric receptors incorporate parts of various other receptors to optimize cellular function. The CAR-T cells have achieved remarkable success targeting CD19 in blood cancers, with high response rates in lymphoma (Davila et al., 2014; Miller and Maus, 2015). However, translating CAR-T cell efficacy to solid tumors poses numerous challenges like the tumor immunosuppressive microenvironment and extensive extracellular matrix surrounds solid tumors, often physically blocking CAR-T migration and tumor penetration (Martinez and Moon, 2019; Donnadieu et al., 2020).
Overall, immunomodulatory drugs offer diverse approaches within cancer immunotherapy. Often used in combinations to maximize effectiveness, immunotherapeutic regimen design depends on the type of cancer, the stage, and the patient’s overall health (Wang et al., 2022). Additionally, emerging research suggests that a patient’s microbiome can significantly influence the effectiveness of cancer immunotherapy, leading to the development of therapies aimed to manipulate the microbiome and enhance the immune response against cancer.
2 The influence of the microbiome on cancer immune therapy effectiveness
The human microbiome, comprising trillions of microorganisms, has gained attention due to its pivotal role in health and disease, particularly its potential influence on cancer and cancer immunotherapy. The microbiome’s composition and diversity can significantly influence immune responses, making it an intriguing factor to investigate in the context of personalized cancer treatment (Gopalakrishnan et al., 2018a).
The intersections between the microbiome and cancer can be categorized at two levels (Jain et al., 2021). The first is between microbiome composition and the development of tumor, and the second between the microbiome and the immune system which in turn can direct the immune response to cancer immune therapy. The impact of microbes on the immune response and their potential as therapeutic targets in cancer treatment have been subjects of interest in recent research (Barukčić, 2017; Turna et al., 2017; Priya et al., 2022).
Metabolites and cytokines are one of the main methods of crosstalk between the microbiome and the immune system. The gut microbiota produces various metabolites and cytokines that can shape systemic immunity. For example, certain bacteria metabolize dietary fiber into short-chain fatty acids (SCFA) like butyrate, which have anti-inflammatory effects and promote T-cell differentiation (Maslowski et al., 2009; Kim et al., 2014). Gut microbe released SCFAs enter circulation and modulate systemic immune cell differentiation and function. SCFAs can promote regulatory and T helper cell 1 and 17 cell differentiation and hence affect the immune therapy activity (Kim et al., 2016). Also, the bacterium B. pseudolongum produces inosine which enhances checkpoint inhibitor efficacy (Mager et al., 2020). This effect could be modulated through the inhibition of ubiquitin-activating enzyme UBA6 in tumor cells which in turn augment its sensitivity to the cytotoxic activity of T cells (Zhang et al., 2022). Interestingly, some metabolites like butyrate can also have concentration-dependent opposite effects such that low levels induce T regulatory cells while higher levels boost CD8+ T cell effector activity. In the clinical setting, responsive cancer patients were reported to show a higher systemic SCFA level (Arpaia et al., 2013).
Several strategies, including fecal microbiota transplantation (FMT) and probiotic administration, have thus been adopted in attempts to enhance cancer immunotherapy efficacy. In a study by the Vétizou group (Mao et al., 2021) examining both mouse models and melanoma patient samples, it was revealed that the therapeutic efficacy of CTLA-4 blockade is dependent on key gut commensals such as Bacteroides thetaiotaomicron and B. fragilis. Furthermore, the presence of T cells specific for these bacteria was associated with superior CTLA-4 response rates. Depletion of these bacterial populations, either in germ-free mice or through antibiotic treatment, mitigated the therapeutic effect. However, repopulation using probiotics or T-cell-targeted approaches could restore responsiveness to CTLA-4 inhibition.
Along the lines of microbiota manipulation to enhance the cancer immunotherapy response, another study identified that Bifidobacterium administration, either through fecal transfer or oral routes, was able to halt melanoma progression similarly to PD-L1 antibody therapy. Furthermore, the combined effects of Bifidobacterium and PD-L1 blockade together led to a significant inhibition of tumor growth. This highlights the synergistic potential of microbial secretants and checkpoint inhibitors in overcoming cancer progression (Sivan et al., 2015). Another preclinical study in germ-free mice showed that FMT from responder patients can replicate patient response to checkpoint inhibitors; non-responder phenotype in mice is reversible with additional FMT from responders (Routy et al., 2018). Several clinical trials are now testing microbiome restoration to improve immunotherapy response. For example, in melanoma patients refractory to anti-PD-1 treatment, FMT was tested in combination with pembrolizumab. This combined therapy resulted in stabilized disease or tumor regression in 2 patients out of 3 [clinical trial: NCT 03341143]. Given these promising results, the mechanism behind the gut microbiome modulating the cancer immune therapy response is of great interest to optimize and direct the use of microbiome manipulation, either at the study design level or for therapeutic intervention.
One of the commonly reported mechanisms for microbiome interaction with immunotherapy response is through the presentation of pro-inflammatory antigens. The gut microbiome interacts with host immune cells via pattern recognition receptors like Toll-like receptors, stimulating inflammatory responses that help shape tumor immunity (Sato and Kawakami, 2022). Gut bacteria provide antigens that are sampled by intestinal immune cells and transported to lymphoid tissues to initiate B cell and T cell responses. Cross-reactivity between microbial and tumor antigens can boost anti-tumor immunity not only locally but systematically (Mowat and Agace, 2014).
A study highlighted the concept of systemic circulation of bacterial antigens and their role in cross-reactivity with pancreatic tumor antigens (Daillère et al., 2016). It reported that translocation of Enterococcus hirae from the gut to lymph nodes/spleen during cyclophosphamide chemotherapy enhanced anti-tumor immune responses in mice. This effect was linked to an E. hirae bacteriophage antigen cross-reactive with a tumor antigen, highlighting microbiome antigen mimicry of tumor antigens as an immunomodulatory mechanism (Fluckiger et al., 2020).
2.1 Clinical evidence of association between the microbiome and cancer immunotherapy outcomes
Multiple clinical trials across diverse cancer types like melanoma, lung, renal, gastrointestinal, thoracic, and hepatobiliary cancers have explored associations between the gut microbiome composition and outcomes with immuno-oncology treatments, especially immune checkpoint inhibitors (ICIs) like anti-PD-1 therapy (Gopalakrishnan et al., 2018b; Routy et al., 2018; Jin et al., 2019; Baruch et al., 2021; Mao et al., 2021; Yin et al., 2021; Che et al., 2022; Derosa et al., 2022; Lee et al., 2022; Pietrzak et al., 2022). Common techniques utilized included 16S rRNA sequencing, metagenomics shotgun sequencing, and metabolomics profiling of longitudinally collected fecal samples before and during ICI treatment to characterize taxonomic and functional changes. Key findings across several studies suggested that intrinsic microbiome diversity and richness are strongly associated with improved rates of clinical response to ICIs including progression-free survival, and overall survival (Jin et al., 2019; Baruch et al., 2021; Derosa et al., 2022; Pietrzak et al., 2022). Specific taxa enriched in responder groups included Akkermansia muciniphila, Bifidobacterium pseudocatenulatum, Prevotella spp., Ruminococcaceae spp., and Bacteroides while non-responders had increased abundance of Proteobacteria and Firmicutes species (Zheng et al., 2019; Peng et al., 2020) (Baruch et al., 2021; Lee et al., 2022; Pietrzak et al., 2022; Wu et al., 2022). Though no microbiome biomarkers could consistently differentiate response groups across diverse cohorts, these bacteria could modulate antitumor immunity via metabolite production or immune stimulation including tryptophan-derived metabolite indole-3-propionic acid (IPA) that was shown to enhance the efficacy of CD8+ T cell-mediated αPD-1 immunotherapy and via immune stimulation (Yin et al., 2021; Lee et al., 2022). Interventions like fecal microbiota transplantation from responders to germ-free mice or patients heightened ICI efficacy by favorably restoring gut homeostasis (Routy et al., 2018; Baruch et al., 2021). These studies are summarized in Table 1. It describes the cancer type, therapy used, microbiome intervention, antibiotic use, readouts, outcomes, and details for the trials.
Developing microbiome-informed therapeutics to treat cancer still has several challenges. Challenges include the resilience of the gut microbiota which makes achieving a sustainable change in microbiota hard (Bloom et al., 2021). Also, the relationship between specific microbiota species and therapeutic outcomes in cancer patients is not fully understood due to unpredicted and undesigned outcomes of clinical trials (Matson et al., 2018). To further compound the difficulty of utilizing microbiome elements to enhance therapies, the is a body of evidence showing that microbiome in older patients commonly lead to detrimental clinical outcomes. The decreased diversity and loss of beneficial species in the microbiomes of older hosts results in detrimental effects such as decreased vaccine efficacy, chronic inflammation, and chronic illness (Bosco and Noti, 2021; Ghosh et al., 2022). Given the extensive relationship between the microbiome and immunotherapy response, it is unsurprising that aging related microbiome changes can effect immunotherapy response, with potential effects being particularly noticeable in ICB therapy (Spakowicz et al., 2021). To make the translation of the microbiome research into microbiome-informed therapeutic interventions in cancer patients we must improve our modeling capabilities for elucidating underlying tumor-immune-microbiome mechanisms and interactions.
3 Cancer modeling
In efforts to best study tumor pathophysiology and therapeutic responses, researchers employ a multitude of in vivo and in vitro platforms, each with their own benefits and limitations (Table 2). Two-dimensional plate-based cell culture and animal-based cancer models utilize almost exclusively different methodologies to examine disease growth and progression.
Conventional in vitro platforms include numerous tumor cell lines used in 2D formats for genetic pathway analysis and drug screening. While these models contribute important findings to the field of cancer biology and produce high throughput results and easily replicable, cell lines can have limited validity. With continual passages, genetic and phenotypic morphology can drift from the original tumor composition (Torsvik et al., 2014; Yu et al., 2019; Quevedo et al., 2020). Additionally, lack of cell-cell and cell-extracellular matrix contact in traditional 2D cultures reduces cell capacity to faithfully model in vivo cancer-associated pathways and interactions (Luca et al., 2013).
In vivo cancer models include genetically engineered animals to mimic human tumors, patient and cell line derived tumor xenografts, and spontaneous tumor development in veterinary species. While these models more readily capture the complexity of tumor development in the whole organism, they can be limited by their reproducibility, cost, and construction time. Murine xenograft models, which allow for the study of human derived tumor and associated microenvironment in a living system, are expensive, require immunocompromised host animals, can take up to 6 months or longer to produce, and cannot support all tumor types (Abdolahi et al., 2022). More readily available genetically engineered animals, typically mice, serve an important role in tumor development and drug discovery research. However, their predictive value in translational research can be limited by the lack of etiologic similarity in tumor development and key microbiome and immune system structure differences from humans (Mestas and Hughes, 2004; Dawson et al., 2009). Spontaneous, naturally occurring tumor animal models, particularly in nonhuman primates, allow for effective translational study of tumor development and tumor, immune, microbiome interaction (Deycmar et al., 2023). Yet, these animals also incur significant expense, can have limited throughput, and not all human tumors are represented in other species. To bridge the limitations between the conventional 2D tumor cell culture models and in vivo models, tumor organoids are quickly gaining popularity.
3.1 Bioengineered tumor organoids in cancer modeling
Cancer organoid models provide a reliable platform that bridges the gap between the 2D cancer cell lines and animal models. The tumor microenvironment (TME) is described as a heterogeneous and dynamic milieu composed of stromal, cancer, and immune cells surrounded by a dynamic extracellular matrix (ECM) undergoing continuous remodeling that substantially impacts cancer promotion, progression, and metastasis. ECM plays a key role in shaping the cancer treatment response (Galdiero et al., 2018; Arneth, 2019). Through recapitulating the heterogeneity of the tumor microenvironment cancer organoids provide a relevant pre-clinical model to study cancer pathophysiology. The basic technology behind tissue organoid models utilizes extracellular matrices to support self-organizing of different cell types in a 3D culture and create a more physiologically relevant tissue model. With proper use, these models can better simulate both overall morphology and cell proliferation, differentiation, and migration (Fair et al., 2020; Gjorevski et al., 2022; Mead et al., 2022). Particularly in the context of cancer, bioengineered cancer organoid consist of cancer cells embedded with stromal cells such as the cancer associated fibroblasts (CAFs) encapsulated in specialized ECM based support (Figure 1). These cancer cells can be obtained from established cell lines such as shown in the study by Oz et al., where Hep3B, Huh7, and HepG2 cell lines were encapsulated in Matrigel to produce hepatocellular carcinoma (HCC)-like organoids (Oz et al., 2021). Alternatively, unsorted tumor and stromal cells derived from the patient’s tumor are enclosed within specialized hydrogel or ECM to facilitate the formation of organoids (Nagle et al., 2018; Mazzocchi et al., 2018; Votanopoulos et al., 2019a; Forsythe et al., 2021). Organoids derived from patient samples preserve the heterogeneity of the original tumor, offering a more accurate in vitro representation of the tissue. Various natural and synthetic ECM options are available for organoid model generation. These include natural ECM components like Collagen type I, Matrigel, decellularized tissues (Giobbe et al., 2019), synthetic hydrogels and recombinant proteins. These materials have been reported to support the growth of organoid models, providing diverse environments for studying cancer within a controlled setting (Kozlowski et al., 2021). The organoids’ 3D architecture was found to alter protein expression and chemosensitivity compared to 2D cultures. Unlike 2D cancer lines, the Cancer organoid model comes with many advantages in cancer research and drug development. They preserve the heterogeneity of the parent tissues and allow the study of drug response and resistance mechanisms. Also, it is a convenient platform to understand the cell’s crosstalk and the role of the stromal compartment in modulating cancer progression and shaping drug response. These models grow relatively quickly, facilitating high-throughput screening and personalized medicine approaches Furthermore, cancer organoids are more cost-effective and human-relevant than animal studies, providing an edge in genetic manipulation experiments (Table 2). Several 3D tumor models were reported starting from the multicellular spheroids that were generated to provide the heterogenous cancer microenvironment. Multicellular spheroids are scaffold-free 3D models that are easy to generate and used for cancer research studies because of their simple production through hanging drop or rotatory methods. They are used for several applications including drug screening. A study by Kim et al. generated HCC multicellular spheroids through the co-culture of HEPG2 and insulin-secreting cell line (RIN-5F). They reported higher albumin secretion, which reflected an augmented cell functionality in the 3D heterogeneous culture condition (Kim et al., 2012). In another study Hwang’s team generated pancreatic ductal adenocarcinoma organoids through the co-culture of PANC-1 tumor spheroids with pancreatic stellate cells encapsulated in a collagen matrix. This model enabled them to study ECM remodeling in the context of EMT and anti-invasiveness treatment efficacy (Hwang et al., 2019). In another example, pancreatic cancer organoids were generated from the co-culture of S2-013 cell line, HUVEC, and mesenchymal stem cells, all encapsulated in Matrigel and supplemented with cancer organoid medium to be used later for drug screening (Tanaka et al., 2022). Gastric cancer organoids were also generated from gastric cancer tissues after being digested and then embedded in Matrigel for anti-cancer therapeutics screening (Steele et al., 2019). Through the same approach, cancer organoid models were reported to be engineered for breast cancer (Berndt-Paetz et al., 2023) and bladder cancer. Significant research efforts have focused on enhancing the biological relevance of cancer organoid models by addressing reported limitations such as insufficient heterogeneity, absence of vasculature, suboptimal ECM scaffolds, and lacking immune components. Additional elements such as microbes and their metabolites can be added to create a biomimetic tumor microenvironment (Kadosh et al., 2020; Wan et al., 2021; Shelkey et al., 2022; Xiao et al., 2022). As such, the tumor organoids better mimic in vivo characteristics and can be applied as unique models in biomedical research and clinical practice applications precision medicine (Myungjin Lee et al., 2013; Imamura et al., 2015; Riedl et al., 2017; Dzobo et al., 2018; Melissaridou et al., 2019).
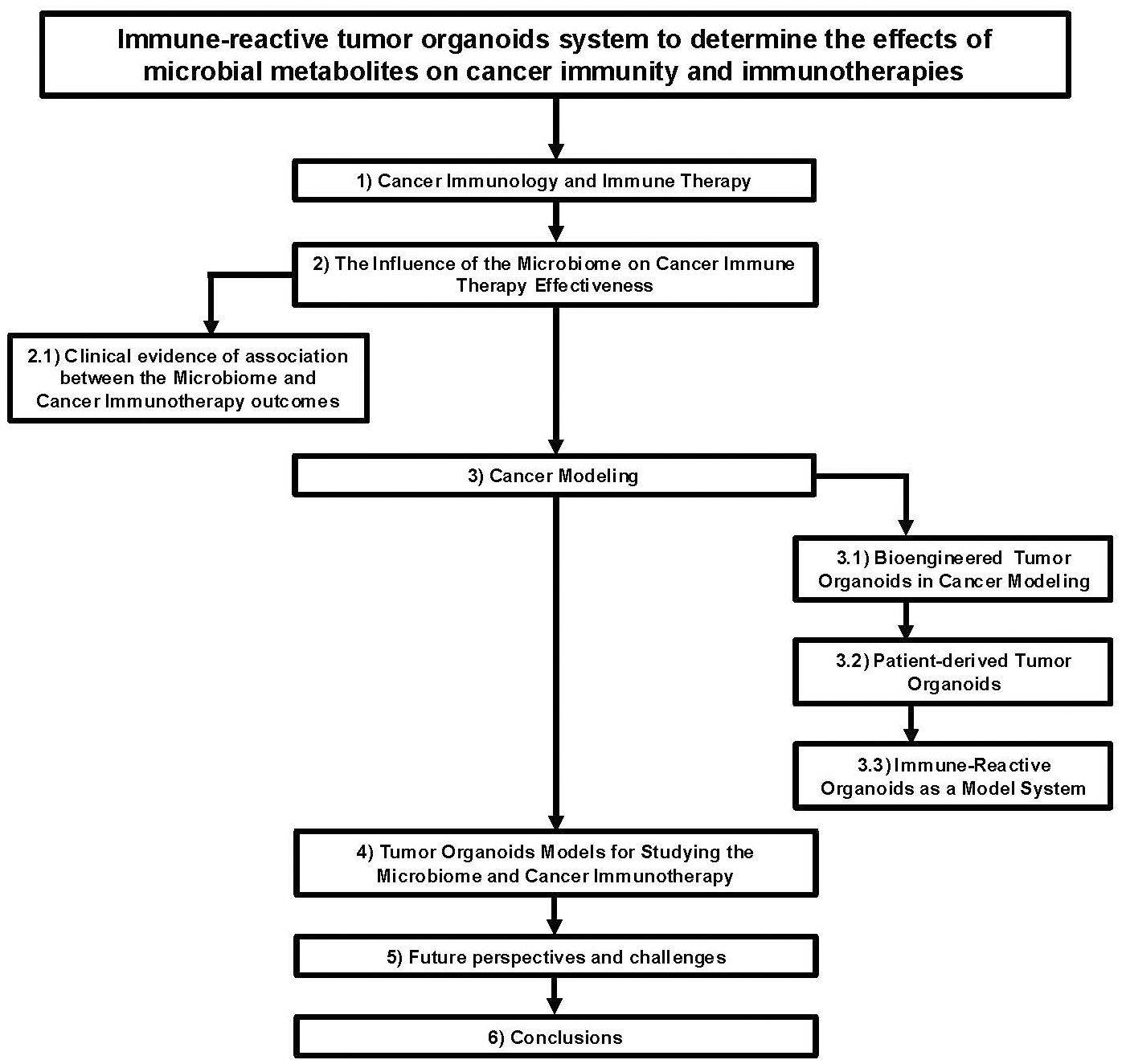
Figure 1. Flow chart diagram describing the application of immune-reactive tumor organoids to study the effects of microbial metabolites on cancer immunity and immunotherapies.
3.2 Patient-derived tumor organoids
Precision medicine dramatically changes the clinical approach for disease prevention, diagnosis, and treatment from a one size fits all approach to using a patient’s unique genetic, molecular, immune and cellular profile to guide clinical decisions. As this methodology gains traction in cancer therapeutic research, patient biopsy derived tumor organoids are critical for understanding inter-patient and intra-patient differences in tumorigenesis and treatment sensitivity (Xing et al., 2021; Guan and Huang, 2022). Tumor biopsies, obtained through surgical resection of primary or metastatic tumor sites, can be used to produce patient specific tumor organoids. These preclinical models more accurately capture disease genomic complexity compared to traditional 2D cell line models (Xia et al., 2019). Additionally, with increasing numbers of patient-derived tumor organoid studies, living biobanks of tumors are being established to aid in the discovery of subtype heterogeneity, novel drug targets, and therapeutic screening (Fujii et al., 2016; Yan et al., 2018; Guan and Huang, 2022).
Recent publications from Forsythe et al., 2020, 2022, and 2023, and Votanopoulos et al., 2019a, highlight the application of tumor organoids from sarcoma, peritoneal mesothelioma, colorectal, and appendiceal cancers for chemotherapeutic efficacy screening on an individual patient basis (Votanopoulos et al., 2019a; Forsythe et al., 2020; Forsythe et al., 2022a; Forsythe et al., 2023). These studies underscored the improved chemo-response modeling of tumor organoids compared to 2D cell lines when comparing chemotherapeutic responses (Forsythe et al., 2020). Beyond their usefulness to model patient-specific chemo-response, tumor organoids created from different metastatic sites within the same patient showed differential therapeutic response, demonstrating the ability to model intra-patient lesion-specific chemo-response and the underlying disease clonality (Forsythe et al., 2023).
3.3 Immune-reactive organoids as a model system
By integrating patient matched immune cells such as T cells, macrophages, and dendritic cells, tumor-immune cell organoids have emerged as an important platform to study tumor-immune interactions critical to tumor progression and therapeutic response. As previously mentioned above, numerous studies have utilized autologous immune enhanced patient derived tumor organoids in efforts to predict clinical immune checkpoint inhibitor success (Votanopoulos et al., 2019a; Forsythe et al., 2020; Forsythe et al., 2022a; Forsythe et al., 2023). On a more mechanistic level, tumor-immune cell or immune-reactive organoids serve the purpose to study immune cell infiltration into the tumor mass (Tsai et al., 2018). This is essential for investigating the spatial distribution of immune cells within the TME and the immuno-biologic reactions in the tumor microenvironment.
Immune-reactive organoid platforms can be established by incorporating immune components through one of two primary strategies: 1) retaining endogenous immune cells that are intrinsically present in parental tissue, and 2) co-culturing autologous immune cells with tissue-matched tumor organoids (Figure 2).
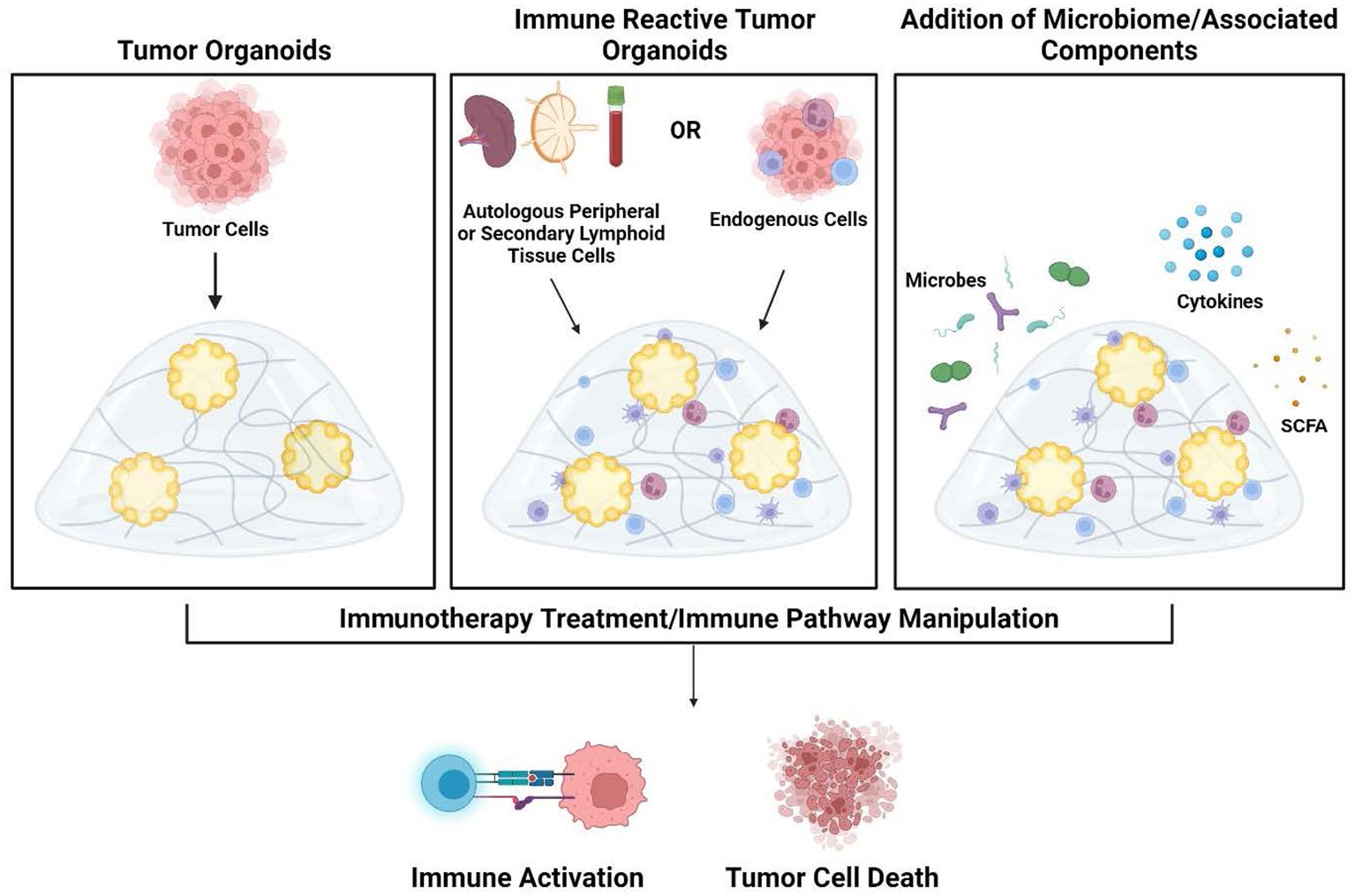
Figure 2. Development of tumor organoids to test immune response pathways, tumor cytotoxicity, and microbiome impact. Organoids are created by encapsulation of dissociated tumor cells in extracellular matrix hydrogels (left panel). To create immune-reactive organoids, immune cells can be isolated from either autologous peripheral blood/secondary lymphoid organs (lymph node or spleen) or isolated from the tumor. Immune cells can then be directly incorporated into the 3D matrix (middle panel). As an additional component, microbes or their metabolites and products such as cytokines and short-chain fatty acids can also be added to the organoid cultures (right panel). Once culture systems are established, testing such as the addition of immunotherapy treatments can be performed to analyze subsequent immune activation and tumor cell death within the organoids. Figure created in BioRender.
3.3.1 Retention of endogenous immune cells from parental tissue strategy
In this strategy, an unsorted cell population, including endogenous immune cells from normal or cancerous tissue, was mixed and encapsulated into various ECM or hydrogels to create tumor immune organoids.
In this innovative approach of immune reactive organoid culture system, efforts are made to maintain an environment conducive to the survival and proliferation of both the tumor cells and resident immune cell population, specifically macrophages and natural killer cells. Several non-tumor organoids have demonstrated the success of these models. A recent study derived mouse adipose organoids via enzymatic digestion of C57BL/6 visceral fat tissue, grown in ultra-low attachment plates to form spheroids. This culture system was able to retain resident macrophage cells, which are critical participants in lipid metabolism. The resulting immune-enhanced model enabled studying innate immune-adipocyte interplay (Taylor et al., 2020). In another work, Kue et al. reported a lung organoid model cultured at an air-liquid interface that retained endogenous lung tissue-resident immune subsets including T-cells, B-cells, natural killers, and myeloid cells (Choi et al., 2023). This immune-enhanced lung organoid provided a significant advance in modeling tissue-resident immunity through an integrated immune-reactive organoid and was used to study T cell activation and responses to SARS-CoV-2 virus exposure. Wan et al., described the use of this organoid culture approach in generating high-grade serous ovarian cancer (HGSC) immune reactive organoids. They used this model to evaluate the efficacy of simultaneous use of PD-1 and PD-L1 Immune Checkpoint Blockades in HGSC (Wan et al., 2021). In another study, Neal et al., developed patient derived tumor organoids with endogenous immune and stromal elements for in vitro immunotherapy modeling (Neal et al., 2018). They found that the inclusion of the native tumor infiltrating lymphocyte population allowed for functional activation, expansion, and cytotoxic response to PD-1/PD-L1 checkpoint blockade therapy.
A key advantage of strategies retaining endogenous immune cells is the preservation of physiologic immune composition diversity and heterogeneity reflective of parental tissue. This better captures the complex dynamics of immune cell interplay with other organoid components versus simplified co-culture approaches. However, maintaining the reproducibility of heterogeneous models with consistent phenotypic stability remains an ongoing challenge.
3.3.2 Co-culture with autologous immune cell types
The most common coculture approaches for the generation of immune-reactive organoids involve coculturing tissue-derived cells with autologous immune cells, including those derived from peripheral blood or secondary lymphoid tissues such as lymph nodes and spleen (Votanopoulos et al., 2019b; Forsythe et al., 2022b; Shelkey et al., 2022).
Shelkey et al. developed an immune-reactive organoid system that included murine colon adenocarcinoma and breast cancer cell lines. Tumor organoids were cocultured with T-lymphocytes derived from murine lymph node tissue. These tumor immune organoids were fabricated by encapsulating the tumor and immune cells in a modified collagen based hydrogel (Shelkey et al., 2021). In another study, they reported generating a similar immune reactive system via coculture of the 4T1 TNBC murine cell line and matched splenocytes. Both models were used as platforms for testing the influence of bacterial metabolites on the efficacy of checkpoint inhibitors PD-1 and CTLA-4. They reported a beneficial effect of the bacterial metabolite on immune cell viability and potency. They also concluded there was a synergistic effect of the bacterial metabolite on the immunotherapy regimen (Shelkey et al., 2022).
Immune reactive organoid models are also generated from patient-derived tumor samples to model the tumor microenvironment and predict immunotherapy response. A patient-specific, immune-enhanced organoid platform for melanoma was developed by co-culturing either lymph node cells or peripheral blood mononuclear cells into matched patient tumor-derived organoids (Votanopoulos et al., 2019b). Organoid response to immunotherapy drugs like nivolumab and pembrolizumab showed 85% predictive accuracy compared to actual patient clinical outcomes. This study advanced personalized immune therapy regimens using patient-derived organoids. Similar investigations into tumor immunobiology utilized a patient-derived organoid model of gastric cancer co-cultured with PBMC-derived immune cells. Here, Chakrabarti et al., 2021 found that HER2 regulates PD-L1 expression in gastric cancer to drive tumor-immune cell evasion (Chakrabarti et al., 2021). These findings support further research into combinatorial therapy for gastric cancer, as well as the use of organoid/immune co-cultures to screen for targeted therapeutic approaches.
In the same vein, immune cells can be sourced from the tumor sample itself via coculture of tumor-infiltrating lymphocytes (TILs). TILs are isolated from tumor samples, expanded ex vivo, then reintroduced to tumor cells to potentially enhance anti-tumor cytotoxicity toward specific antigens (Magré et al., 2023). One study reported a co-culture system between patient-derived rectal cancer tumoroids and matched TILs that were isolated, expanded, and reintroduced. This immune reactive system was used to assess the immune response to checkpoint blockade inhibitors. The study showed a restored TIL cytotoxicity and increased PD-1 expression upon treatment with anti-PD-1 antibody (Kong et al., 2018).
The co-culture system permits the pretreatment and genetic modification of immune cells, such as CAR-T cells, to target specific antigens. This aspect is crucial for advancing the mechanisms and methods in immunotherapy (Yu et al., 2021). Furthermore, these systems facilitate research into immune cell role in shaping tumor behavior and drug responses at both the cellular and the more expansive tissue-mimetic organoid levels. A notable example of this approach is the work by Jiang et al., who elucidated the role of macrophages in modulating Gemcitabine resistance in pancreatic adenocarcinoma. They generated an immune-reactive model using a co-culture of patient-derived tumor samples and tumor-derived macrophage cells. This study revealed the critical role of the CCL5-p1-AREG axis feedback loop between macrophages and pancreatic cancer cells (PCCs) in conferring drug resistance (Jiang et al., 2023).
From these examples and numerous others, tumor organoid platforms are increasingly recognized as a novel means to both improve our understanding of immunotherapy mechanisms and drive therapeutic progress.
4 Tumor organoids models for studying the microbiome and cancer immunotherapy
Recent developments in biomaterials have resulted in more physiologically accurate culture methods that can be used to study complex human systems (Rossi et al., 2018). Specifically, advances in immune population and microbiome ex vivo models have allowed for composite models to examine interactions between the two systems (Figure 1). Organoids have been used to model many aspects of the microbiome and immune environment including different bacterial species, viral infections, and effects on various cellular niches (Min et al., 2020). Anti-PD-1 and anti-CTLA-4 have been tested in microfluidic devices to model efficacy (Aref et al., 2018). Organoids and spheroid culture have also demonstrated their use as a model for studying ICI efficacy (Jenkins et al., 2018; Neal et al., 2018). Microbiome derived factors and viable immune responses can therefore be combined to create a model that can demonstrate the interplay between microbiome derived metabolites and ICI in an organoid system (Shelkey et al., 2022). Chip systems have even been constructed that incorporate microbiota compartments to produce immunomodulatory effects on the tissue present with specific species causing identifiable inflammatory responses through factors like metabolite production and reactive oxygen species (De Gregorio et al., 2022). It is even possible to modulate the microbiome in chip-based systems to cause inflammation and bacterial outgrowth, which can then be monitored in ways that incompatible with animal models (Kim et al., 2016). The high throughput nature of ex vivo culture makes it ideal for conducting large scale studies that work to isolate individual components underpinning the mechanisms of action for complex systems (Rae et al., 2021). Further advances in advanced cell culture models will continue to contribute to understanding immunotherapy-microbiome interactions.
5 Future perspectives and challenges
Ex vivo culture of cells and tissue constructs is continuously advancing with applications in precision medicine, immune modeling, and organ system replication. Organoids have proven to be particularly effective at predicting cancer patient response to therapeutic treatment (Nagle et al., 2018; Mazzocchi et al., 2018; Votanopoulos et al., 2019a; Forsythe et al., 2021). Advances in microfluidic production have allowed multiple organ systems to be integrated in one construct to demonstrate how all of the different systems interact (Skardal et al., 2017; Ingber, 2022). These systems are also significantly better for continuous monitoring of cell populations of interest (Kim et al., 2016). Microbiome modulation and analysis in correlation with cancer immune therapy and cancer progression has been investigated in several clinical studies to evaluate the positive outcomes and drawbacks. These studies also aim to estimate the potential of antibiotics that affect gut microbiome composition and the subsequent influence on either cancer promotion or response to therapeutics. There have previously been difficulties in producing ex vivo culture models that are exposed to live bacterial populations, with tissue and live bacterial interactions being limited to animal models. Some researchers compensated by focusing on bacterial metabolites that could be used in aseptic culture; however, intestine-on-a-chip models have now been produced that are able to sustain equivalent microbiomes in culture with intestinal epithelium. These models replicate oxygen gradients, intestinal barriers, and can be utilized going forward for the controlled testing of therapeutics (Jalili-Firoozinezhad et al., 2019; Shin et al., 2019). Different versions of the gut-on-a-chip model can even model peristaltic movements while maintaining a normal microbial population (Kim et al., 2012). With the continuous advances in cell culture technology producing models that were previously impossible, it is likely that more ex vivo models better able to reproduce the complicated cancer immunotherapy-microbiome interactions will be produced. These models can more easily be leveraged for high-throughput studies both for mechanistic investigation and predictive precision medicine, resulting in better patient care. These results will continue to supplement and corroborate the ongoing clinical trials that aim to elucidate the interactions between the microbiome and cancer immunotherapy.
6 Conclusion
With several drugs in the clinical pipeline and more clinical trials in progress, immunotherapies are a promising therapeutic for a wide array of tumor types (Cloughesy et al., 2019; Ni et al., 2021; Rothschild et al., 2021; Vignali et al., 2022; Garon et al., 2023; Huang et al., 2023; Boesen et al., 2024). To continue to grow the list of available immunotherapies, advanced testing platforms are necessary. Tumor organoids, particularly those derived from human primary specimens, are an increasingly important platform to personalize current therapies and model various tumor microenvironment interactions to accelerate novel drug development. In particular, the capacity to co-culture microbiome, immune cells, and tumor organoids provides critical insight into complex interplays which regulate immunotherapy responses. As we highlight in this review, these dynamic mechanisms cannot be accurately modeled in traditional 2D culture systems, and animal models fail to provide fully translational findings. As microfabrication technologies continue to evolve and improve the organoid systems, these ex vivo assays are a crucial tool to innovate immunotherapy treatment strategies through understanding of the microbiome-immune-tumor interactions and improve patient outcomes.
Author contributions
AE-D: Investigation, Writing – original draft. CS: Writing – original draft, Writing – review & editing. ES: Conceptualization, Writing – original draft, Writing – review & editing. KC: Writing – review & editing. KV: Writing – review & editing. SS: Writing – original draft, Writing – review & editing.
Funding
The author(s) declare financial support was received for the research, authorship, and/or publication of this article. Partial funding was provided from the Atrium Wake Forest Baptist Comprehensive Cancer Center through NCI Grant P30CA012197.
Conflict of interest
The authors declare that the research was conducted in the absence of any commercial or financial relationships that could be construed as a potential conflict of interest.
Publisher’s note
All claims expressed in this article are solely those of the authors and do not necessarily represent those of their affiliated organizations, or those of the publisher, the editors and the reviewers. Any product that may be evaluated in this article, or claim that may be made by its manufacturer, is not guaranteed or endorsed by the publisher.
References
Abdolahi S., Ghazvinian Z., Muhammadnejad S., Saleh M., Asadzadeh Aghdaei H., Baghaei K. (2022). Patient-derived xenograft (PDX) models, applications and challenges in cancer research. J. Trans. Med. 20, 206. doi: 10.1186/s12967-022-03405-8
Andersen R., Donia M., Ellebaek E., Borch T. H., Kongsted P., Iversen T. Z., et al. (2016). Long-lasting complete responses in patients with metastatic melanoma after adoptive cell therapy with tumor-infiltrating lymphocytes and an attenuated IL2 regimen. Clin. Cancer Res. 22, 3734–3745. doi: 10.1158/1078-0432.CCR-15-1879
Aref A. R., Campisi M., Ivanova E., Portell A., Larios D., Piel B. P., et al. (2018). 3D microfluidic ex vivo culture of organotypic tumor spheroids to model immune checkpoint blockade. Lab. Chip 18, 3129–3143. doi: 10.1039/C8LC00322J
Arpaia N., Campbell C., Fan X., Dikiy S., Van Der Veeken J., Deroos P., et al. (2013). Metabolites produced by commensal bacteria promote peripheral regulatory T-cell generation. Nature 504, 451–455. doi: 10.1038/nature12726
Baruch E. N., Youngster I., Ben-Betzalel G., Ortenberg R., Lahat A., Katz L., et al. (2021). Fecal microbiota transplant promotes response in immunotherapy-refractory melanoma patients. Science 371, 602–609. doi: 10.1126/science.abb5920
Barukčić I. (2017). Helicobacter pylori—The cause of human gastric cancer. J. Biosci. Medicines 5, 1–9. doi: 10.4236/jbm.2017.52001
Berndt-Paetz M., Han S., Weimann A., Reinhold A., Nürnberger S., Neuhaus J. (2023). Cell line-based human bladder organoids with bladder-like self-organization—a new standardized approach in bladder cancer research. Biomedicines 11, 2958. doi: 10.3390/biomedicines11112958
Bloom P. P., Tapper E. B., Young V. B., Lok A. S. (2021). Microbiome therapeutics for hepatic encephalopathy. J. Hepatol. 75, 1452–1464. doi: 10.1016/j.jhep.2021.08.004
Boesen K., Hirt J., Dueblin P., Laubli H., Kassenda B., Hemkens L. G., et al. (2024). Rationale and design of the Cancer Immunotherapy Evidence Living (CIEL) Library: A continuously updated clinical trial database of cancer immunotherapies. medRxiv, 2024–2004. doi: 10.1101/2024.04.26.24306436
Bosco N., Noti M. (2021). The aging gut microbiome and its impact on host immunity. Genes Immun. 22, 289–303. doi: 10.1038/s41435-021-00126-8
Camisaschi C., Renne S. L., Beretta V., Rini F., Spagnuolo R. D., Tuccitto A., et al. (2018). Immune landscape and in vivo immunogenicity of NY-ESO-1 tumor antigen in advanced neuroblastoma patients. BMC Cancer 18, 1–11. doi: 10.1186/s12885-018-4910-8
Chakrabarti J., Koh V., Steele N., Hawkins J., Ito Y., Merchant J. L., et al. (2021). Disruption of her2-induced PD-L1 inhibits tumor cell immune evasion in patient-derived gastric cancer organoids. Cancers 13, 6158. doi: 10.3390/cancers13246158
Che H., Xiong Q., Ma J., Chen S., Wu H., Xu H., et al. (2022). Association of Helicobacter pylori infection with survival outcomes in advanced gastric cancer patients treated with immune checkpoint inhibitors. BMC Cancer 22, 904. doi: 10.1186/s12885-022-10004-9
Chen H., et al. (2022). Urological cancer organoids, patients' avatars for precision medicine: past, present and future. Cell Biosci. 12, 132. doi: 10.1186/s13578-022-00866-8
Choi S. S., Van Unen V., Zhang H., Rustagi A., Alwahabi S. A., Santos A. J. M., et al. (2023). Organoid modeling of lung-resident immune responses to SARS-CoV-2 infection. Res. Square. doi: 10.21203/rs.3.rs-2870695/v1
Cloughesy T. F., Mochizuki A. Y., Orpilla J. R., Hugo W., Lee A. H., Davidson T. B., et al. (2019). Neoadjuvant anti-PD-1 immunotherapy promotes a survival benefit with intratumoral and systemic immune responses in recurrent glioblastoma. Nat. Med. 25, 477–486. doi: 10.1038/s41591-018-0337-7
D’Angelo S. P., Melchiori L., Merchant M. S., Bernstein D., Glod J., Kaplan R., et al. (2018). Antitumor activity associated with prolonged persistence of adoptively transferred NY-ESO-1 c259T cells in synovial sarcoma. Cancer Discovery 8, 944–957. doi: 10.1158/2159-8290.CD-17-1417
Daillère R., Vétizou M., Waldschmitt N., Yamazaki T., Isnard C., Poirier-Colame V., et al. (2016). Enterococcus hirae and Barnesiella intestinihominis facilitate cyclophosphamide-induced therapeutic immunomodulatory effects. Immunity 45, 931–943. doi: 10.1016/j.immuni.2016.09.009
Davila M. L., Bouhassira D. C. G., Park J. H., Curran K. J., Smith E. L., Pegram H. J., et al. (2014). Chimeric antigen receptors for the adoptive T cell therapy of hematologic Malignancies. Int. J. Hematol. 99, 361–371. doi: 10.1007/s12185-013-1479-5
Davar D., Dzutsev A. K., McCulloch J. A., Rodrigues R. R., Chauvin J. M., Morrison R. M., et al. (2021). Fecal microbiota transplant overcomes resistance to anti-PD-1 therapy in melanoma patients. Science 595 (6529), 595–602. doi: 10.1126/science.abf3363
Dawson H. D., Reece J. J., Urban J. F. Jr. (2009). A comparative analysis of the porcine, murine, and human immune systems. Vet. Immunol. Immunopathol. 128, 309–309. doi: 10.1016/j.vetimm.2008.10.211
De Gregorio V., Sgambato C., Urciuolo F., Vecchione R., Netti P. A., Imparato G. (2022). Immunoresponsive microbiota-gut-on-chip reproduces barrier dysfunction, stromal reshaping and probiotics translocation under inflammation. Biomaterials 286, 121573. doi: 10.1016/j.biomaterials.2022.121573
Derosa L., Routy B., Thomas A.M., Iebba V., Zalcman G., Friard S., et al. (2022). Intestinal Akkermansia muciniphila predicts clinical response to PD-1 blockade in patients with advanced non-small-cell lung cancer. Nat. Med. 28, 315–324. doi: 10.1038/s41591-021-01655-5
Deycmar S., Gomes B., Charo J., Ceppi M., Cline J. M. (2023). Spontaneous, naturally occurring cancers in non-human primates as a translational model for cancer immunotherapy. J. Immunother. Cancer 11 (1), e005514. doi: 10.1136/jitc-2022-005514
Donnadieu E., Dupré L., Pinho L. G., Cotta-De-Almeida V. (2020). Surmounting the obstacles that impede effective CAR T cell trafficking to solid tumors. J. Leucocyte Biol. 108, 1067–1079. doi: 10.1002/JLB.1MR0520-746R
Dzobo K., Rowe A., Senthebane D. A., Almazyadi M. M., Patten V., Parker M. I. (2018). Three-dimensional organoids in cancer research: the search for the holy grail of preclinical cancer modeling. OMICS 22, 733–748. doi: 10.1089/omi.2018.0172
Esfahani K., Roudaia L., Buhlaiga N. A., Del Rincon S. V., Papneja N., Miller W. H. (2020). A review of cancer immunotherapy: from the past, to the present, to the future. Curr. Oncol. 27, 87–97. doi: 10.3747/co.27.5223
Fair S. R., Julian D., Hartlaub A. M., Pusuluri S. T., Malik G., Summerfied T. L., et al. (2020). Electrophysiological maturation of cerebral organoids correlates with dynamic morphological and cellular development. Stem Cell Rep. 15, 855–868. doi: 10.1016/j.stemcr.2020.08.017
Fluckiger A., Daillere R., Sassi M., Sixt B. S., Liu P., Loos F., et al. (2020). Cross-reactivity between tumor MHC class I–restricted antigens and an enterococcal bacteriophage. Science 369, 936–942. doi: 10.1126/science.aax0701
Forget M.-A., Haymaker C., Hess K. R., Meng Y. J., Creasy C., Karpinets T., et al. (2018). Prospective analysis of adoptive TIL therapy in patients with metastatic melanoma: response, impact of anti-CTLA4, and biomarkers to predict clinical outcome. Clin. Cancer Res. 24, 4416–4428. doi: 10.1158/1078-0432.CCR-17-3649
Forsythe S., Mehta N., Devarasetty M., Sivakumar H., Gmeiner W., Soker S., et al. (2020). Development of a colorectal cancer 3D micro-tumor construct platform from cell lines and patient tumor biospecimens for standard-of-care and experimental drug screening. Ann. Biomed. Eng. 48, 940–952. doi: 10.1007/s10439-019-02269-2
Forsythe S. D., Erali R. A., Edenhoffer N., Meeker W., Wajih N., Schaaf C. R., et al. (2021). Organoid platform in preclinical investigation of personalized immunotherapy efficacy in appendiceal cancer: Feasibility study. Clin. Cancer Res. 27, 5141–5150. doi: 10.1158/1078-0432.CCR-21-0982
Forsythe S. D., Erali R. A., Laney P., Sivakumar H., Li W., Skardal A., et al. (2022a). Patient-specific sarcoma organoids for personalized translational research: unification of the operating room with rare cancer research and clinical implications. Ann. Surg. Oncol. 29, 7354–7367. doi: 10.1245/s10434-022-12086-y
Forsythe S. D., Erali R. A., Sasikumar S., Laney P., Shelkey E., D'agostino R. Jr., et al. (2022b). Application of immune enhanced organoids in modeling personalized Merkel cell carcinoma research. Sci. Rep. 12, 13865. doi: 10.1038/s41598-022-17921-6
Forsythe S. D., Sivakumar H., Erali R. A., Wajih N., Li W., Shen P., et al. (2023). Cisplatin exhibits superiority over MMC as a perfusion agent in a peritoneal mesothelioma patient specific organoid HIPEC platform. Sci. Rep. 13, 11640. doi: 10.1038/s41598-023-38545-4
Fujii M., Shimokawa M., Date S., Takano A., Matano M., Nanki K., et al. (2016). A colorectal tumor organoid library demonstrates progressive loss of niche factor requirements during tumorigenesis. Cell Stem Cell 18, 827–838. doi: 10.1016/j.stem.2016.04.003
Galdiero M. R., Varricchi G., Loffredo S., Mantovani A., Marone G. (2018). Roles of neutrophils in cancer growth and progression. J. leukocyte Biol. 103, 457–464. doi: 10.1002/JLB.3MR0717-292R
Garcia P. L., Miller A. L., Yoon K. J. (2020). Patient-derived xenograft models of pancreatic cancer: overview and comparison with other types of models. Cancers 12, 1327. doi: 10.3390/cancers12051327
Garon E. B., Visseren-Grul C., Rizzo M. T., Puri T., Chenji S., Reck M. (2023). Clinical outcomes of ramucirumab plus docetaxel in the treatment of patients with non-small cell lung cancer after immunotherapy: a systematic literature review. Front. Oncol. 13, 1247879. doi: 10.3389/fonc.2023.1247879
Ghosh T. S., Shanahan F., O’Toole P. W. (2022). The gut microbiome as a modulator of healthy ageing. Nat. Rev. Gastroenterol. Hepatol. 19, 565–584. doi: 10.1038/s41575-022-00605-x
Giobbe G. G., Crowley C., Luni C., Campinoti S., Khedr M., Kretzschmar K., et al. (2019). Extracellular matrix hydrogel derived from decellularized tissues enables endodermal organoid culture. Nat. Commun. 10, 5658. doi: 10.1038/s41467-019-13605-4
Gjorevski N., Nikolaev M., Brown T. E., Mitrofanova O., Brandenberg N., Delrio F. W., et al. (2022). Tissue geometry drives deterministic organoid patterning. Science 375, eaaw9021. doi: 10.1126/science.aaw9021
Gopalakrishnan V., Helmink B. A., Spencer C. N., Reuben A., Wargo J. A. (2018a). The influence of the gut microbiome on cancer, immunity, and cancer immunotherapy. Cancer Cell 33, 570–580. doi: 10.1016/j.ccell.2018.03.015
Gopalakrishnan V., Spencer C.N., Nezi L., Reuben A., Andrews M.C., Karpinets T., et al. (2018b). Gut microbiome modulates response to anti–PD-1 immunotherapy in melanoma patients. Science 359, 97–103.
Goydos J. S., Patel M., Shih W. (2001). NY-ESO-1 and CTp11 expression may correlate with stage of progression in melanoma. J. Surg. Res. 98, 76–80. doi: 10.1006/jsre.2001.6148
Guan X., Huang S. (2022). Advances in the application of 3D tumor models in precision oncology and drug screening. Front. Bioeng Biotechnol. 10, 1021966. doi: 10.3389/fbioe.2022.1021966
Huang H., Yao Y., Deng X., Weng H., Chen Z., Yu L., et al. (2023). Characteristics of immunotherapy trials for nasopharyngeal carcinoma over a 15-year period. Front. Immunol. 14, 1195659. doi: 10.3389/fimmu.2023.1195659
Hwang H. J., Oh M.-S., Lee D. W., Kuh H.-J. (2019). Multiplex quantitative analysis of stroma-mediated cancer cell invasion, matrix remodeling, and drug response in a 3D co-culture model of pancreatic tumor spheroids and stellate cells. J. Exp. Clin. Cancer Res. 38, 1–14. doi: 10.1186/s13046-019-1225-9
Imamura Y., Mukohara T., Shimono Y., Funakoshi Y., Chayahara N., Toyoda M., et al. (2015). Comparison of 2D- and 3D-culture models as drug-testing platforms in breast cancer. Oncol. Rep. 33, 1837–1843. doi: 10.3892/or.2015.3767
Ingber D. E. (2022). Human organs-on-chips for disease modelling, drug development and personalized medicine. Nat. Rev. Genet. 23, 467–491. doi: 10.1038/s41576-022-00466-9
Jain T., Sharma P., Are A. C., Vickers S. M., Dudeja V. (2021). New insights into the cancer–microbiome–immune axis: decrypting a decade of discoveries. Front. Immunol. 12, 622064. doi: 10.3389/fimmu.2021.622064
Jalili-Firoozinezhad S., Gazzaniga F. S., Calamari E. L., Camacho D. M., Fadel C. W., Bein A., et al. (2019). A complex human gut microbiome cultured in an anaerobic intestine-on-a-chip. Nat. Biomed. Eng. 3, 520–531. doi: 10.1038/s41551-019-0397-0
Jenkins R. W., Aref A. R., Lizotte P. H., Ivanova E., Stinson S., Zhou C. W., et al. (2018). Ex vivo profiling of PD-1 blockade using organotypic tumor spheroids. Cancer Discovery 8, 196–215. doi: 10.1158/2159-8290.CD-17-0833
Jiang S., Deng T., Cheng H., Liu W., Shi D., Yuan J., et al. (2023). Macrophage-organoid co-culture model for identifying treatment strategies against macrophage-related gemcitabine resistance. J. Exp. Clin. Cancer Res. 42, 199. doi: 10.1186/s13046-023-02756-4
Jin Y., Dong H., Xia L., Yang Y., Zhu Y., Shen Y., et al. (2019). The diversity of gut microbiome is associated with favorable responses to anti–programmed death 1 immunotherapy in Chinese patients with NSCLC. J. Thorac. Oncol. 14, 1378–1389. doi: 10.1016/j.jtho.2019.04.007
June C. H., Riddell S. R., Schumacher T. N. (2015). Adoptive cellular therapy: a race to the finish line. Sci. Trans. Med. 7, 280ps7–280ps7. doi: 10.1126/scitranslmed.aaa3643
Kadosh E., Snir-Alkalay I., Venkatachalam A., May S., Lasry A., Elyada E., et al. (2020). The gut microbiome switches mutant p53 from tumour-suppressive to oncogenic. Nature 586, 133–138. doi: 10.1038/s41586-020-2541-0
Kaplan D. H., Shankaran V., Dighe A. S., Stockert E., Aguet M., Old L. J., et al. (1998). Demonstration of an interferon γ-dependent tumor surveillance system in immunocompetent mice. Proc. Natl. Acad. Sci. 95, 7556–7561. doi: 10.1073/pnas.95.13.7556
Kim J. Y., Kim H. W., Bae S. J., Joo D. J., Huh K. H., Fang Y. H., et al. (2012). Hybrid cellular spheroids from hepatocellular carcinoma and insulin-secreting cell lines (Elsevier), 1095–1098. doi: 10.1016/j.transproceed.2012.02.016
Kim H. J., Huh D., Hamilton G., Ingber D. E. (2012). Human gut-on-a-chip inhabited by microbial flora that experiences intestinal peristalsis-like motions and flow. Lab. Chip 12, 2165–2174. doi: 10.1039/c2lc40074j
Kim K. S., Hong S.-W., Han D., Yi J., Jung J., Yang B.-G., et al. (2016b). Dietary antigens limit mucosal immunity by inducing regulatory T cells in the small intestine. Science 351, 858–863. doi: 10.1126/science.aac5560
Kim H. J., Li H., Collins J. J., Ingber D. E. (2016a). Contributions of microbiome and mechanical deformation to intestinal bacterial overgrowth and inflammation in a human gut-on-a-chip. Proc. Natl. Acad. Sci. 113, E7–E15. doi: 10.1073/pnas.1522193112
Kim C. H., Park J., Kim M. (2014). Gut microbiota-derived short-chain fatty acids, T cells, and inflammation. Immune netw. 14, 277–288. doi: 10.4110/in.2014.14.6.277
Kong J. C. H., Guerra G. R., Millen R. M., Roth S., Xu H., Neeson P. J., et al. (2018). Tumor-infiltrating lymphocyte function predicts response to neoadjuvant chemoradiotherapy in locally advanced rectal cancer. JCO Precis. Oncol. 2, 1–15. doi: 10.1200/PO.18.00075
Kotla V., Goel S., Nischal S., Heuck C., Vivek K., Das B., et al. (2009). Mechanism of action of lenalidomide in hematological Malignancies. J. Hematol. Oncol. 2, 1–10. doi: 10.1186/1756-8722-2-36
Kozlowski M. T., Crook C. J., Ku H. T. (2021). Towards organoid culture without Matrigel. Commun. Biol. 4, 1387. doi: 10.1038/s42003-021-02910-8
Kronke J., Fink E. C., Hollenbach P. W., Macbeth K. J., Hurst S. N., Udeshi N. D., et al. (2015). Lenalidomide induces ubiquitination and degradation of CK1 [alpha] in del (5q) MDS. Nature 523, 183–203. doi: 10.1038/nature14610
Lee K. A., Thomas A. M., Bolte L. A., Björk J. R., De Ruijter L. K., Armanini F., et al. (2022). Cross-cohort gut microbiome associations with immune checkpoint inhibitor response in advanced melanoma. Nat. Med. 28, 535–544. doi: 10.1038/s41591-022-01695-5
Luca A. C., Mersch S., Deenen R., Schmidt S., Messner I., Schäfer K.-L., et al. (2013). Impact of the 3D microenvironment on phenotype, gene expression, and EGFR inhibition of colorectal cancer cell lines. PloS One 8, e59689. doi: 10.1371/journal.pone.0059689
Mager L. F., Burkhard R., Pett N., Cooke N. C. A., Brown K., Ramay H., et al. (2020). Microbiome-derived inosine modulates response to checkpoint inhibitor immunotherapy. Science 369, 1481–1489. doi: 10.1126/science.abc3421
Magré L., Verstegen M. M. A., Buschow S., Van Der Laan L. J. W., Peppelenbosch M., Desai J., et al. (2023). Emerging organoid-immune co-culture models for cancer research: from oncoimmunology to personalized immunotherapies. J. ImmunoTher. Cancer 11, e006290. doi: 10.1136/jitc-2022-006290
Mao J., Wang D., Long J., Yang X., Lin J., Song Y., et al. (2021). Gut microbiome is associated with the clinical response to anti-PD-1 based immunotherapy in hepatobiliary cancers. J. Immunother. Cancer 9 (12), e003334. doi: 10.1136/jitc-2021-003334
Martinez M., Moon E. K. (2019). CAR T cells for solid tumors: new strategies for finding, infiltrating, and surviving in the tumor microenvironment. Front. Immunol. 10, 128. doi: 10.3389/fimmu.2019.00128
Maslowski K. M., Vieira A. T., Ng A., Kranich J., Sierro F., Yu D., et al. (2009). Regulation of inflammatory responses by gut microbiota and chemoattractant receptor GPR43. Nature 461, 1282–1286. doi: 10.1038/nature08530
Matson V., Fessler J., Bao R., Chongsuwat T., Zha Y., Alegre M.-L., et al. (2018). The commensal microbiome is associated with anti–PD-1 efficacy in metastatic melanoma patients. Science 359, 104–108. doi: 10.1126/science.aao3290
Mazzocchi A. R., Rajan S. P., Votanopoulos K. I., Hall A. R., Skardal A. (2018). In vitro patient-derived 3D mesothelioma tumor organoids facilitate patient-centric therapeutic screening. Sci. Rep. 8, 2886. doi: 10.1038/s41598-018-21200-8
Mead B. E., Hattori K., Levy L., Imada S., Goto N., Vukovic M., et al. (2022). Screening for modulators of the cellular composition of gut epithelia via organoid models of intestinal stem cell differentiation. Nat. Biomed. Eng. 6, 476–494. doi: 10.1038/s41551-022-00863-9
Melissaridou S., Wiechec E., Magan M., Jain M. V., Chung M. K., Farnebo L., et al. (2019). The effect of 2D and 3D cell cultures on treatment response, EMT profile and stem cell features in head and neck cancer. Cancer Cell Int. 19, 16. doi: 10.1186/s12935-019-0733-1
Mestas J., Hughes C. C. (2004). Of mice and not men: differences between mouse and human immunology. J. Immunol. 172, 2731–2738. doi: 10.4049/jimmunol.172.5.2731
Miller B. C., Maus M. V. (2015). CD19-targeted CAR T cells: a new tool in the fight against B cell Malignancies. Oncol. Res. Treat 38, 683–690. doi: 10.1159/000442170
Min S., Kim S., Cho S.-W. (2020). Gastrointestinal tract modeling using organoids engineered with cellular and microbiota niches. Exp. Mol. Med. 52, 227–237. doi: 10.1038/s12276-020-0386-0
Mowat A. M., Agace W. W. (2014). Regional specialization within the intestinal immune system. Nat. Rev. Immunol. 14, 667–685. doi: 10.1038/nri3738
Myungjin Lee J., Mhawech-Fauceglia P., Lee N., Cristina Parsanian L., Gail Lin Y., Andrew Gayther S., et al. (2013). A three-dimensional microenvironment alters protein expression and chemosensitivity of epithelial ovarian cancer cells in vitro. Lab. Invest. 93, 528–542. doi: 10.1038/labinvest.2013.41
Nagle P. W., Plukker J. T. M., Muijs C. T., Van Luijk P., Coppes R. P. (2018a). Patient-derived tumor organoids for prediction of cancer treatment response (Elsevier). doi: 10.1016/j.semcancer.2018.06.005
Neal J. T., Li X., Zhu J., Giangarra V., Grzeskowiak C. L., Ju J., et al. (2018b). Organoid modeling of the tumor immune microenvironment. Cell 175, 1972–1988. e16. doi: 10.1016/j.cell.2018.11.021
Ni W., Li X., Zhu J., Giangarra V., Grzeskowiak C. L., Ju J., et al. (2021). Photothermal-chemotherapy enhancing tumor immunotherapy by multifunctional metal–organic framework based drug delivery system. Nano Lett. 21, 7796–7805. doi: 10.1021/acs.nanolett.1c02782
Old L. J. (1981). Cancer immunology: the search for specificity—G. H. A. Clowes memorial lecture1. Cancer Res. 41, 361–375.
Old L. J., Boyse E. A., Clarke D. A., Carswell E. A. (1962). Antigenic properties of chemically induced tumors. Ann. New York Acad. Sci. 101, 80–106. doi: 10.1111/j.1749-6632.1962.tb26446.x
Oz O., Iscan E., Batur T., Ozturk M. (2021). 3d organoid modelling of hepatoblast-like and mesenchymal-like hepatocellular carcinoma cell lines. Hepatoma Res. 7, 60. doi: 10.20517/2394-5079
Peng Z., Cheng S., Kou Y., Wang Z., Jin R., Hu H., et al. (2020). The gut microbiome is associated with clinical response to anti–PD-1/PD-L1 immunotherapy in gastrointestinal cancer. Cancer Immunol. Res. 8, 1251–1261. doi: 10.1158/2326-6066.CIR-19-1014
Pietrzak B., Tomela K., Olejnik-Schmidt A., Galus Ł., Mackiewicz J., Kaczmarek M., et al. (2022). A clinical outcome of the anti-PD-1 therapy of melanoma in polish patients is mediated by population-specific gut microbiome composition. Cancers 14, 5369. doi: 10.3390/cancers14215369
Ponziani F. R., De Luca A., Picca A., Marzetti E., Petito V., Del Chierico F., et al. (2022). Gut dysbiosis and fecal calprotectin predict response to immune checkpoint inhibitors in patients with hepatocellular carcinoma. Hepatol Commun. 6 (6), 1492–1501. doi: 10.1002/hep4.1905
Priya S., Burns M. B., Ward T., Mars R. T., Adamowicz B., Lock E. F., et al. (2022). Identification of shared and disease-specific host gene–microbiome associations across human diseases using multi-omic integration. Nat. Microbiol. 7, 780–795. doi: 10.1038/s41564-022-01121-z
Quevedo R., Smirnov P., Tkachuk D., Ho C., El-Hachem N., Safikhani Z., et al. (2020). Assessment of genetic drift in large pharmacogenomic studies. Cell Syst. 11, 393–401. e2. doi: 10.1016/j.cels.2020.08.012
Radvanyi L. G., Bernatchez C., Zhang M., Fox P. S., Miller P., Chacon J., et al. (2012). Specific lymphocyte subsets predict response to adoptive cell therapy using expanded autologous tumor-infiltrating lymphocytes in metastatic melanoma patients. Clin. Cancer Res. 18, 6758–6770. doi: 10.1158/1078-0432.CCR-12-1177
Rae C., Amato F., Braconi C. (2021). Patient-derived organoids as a model for cancer drug discovery. Int. J. Mol. Sci. 22, 3483. doi: 10.3390/ijms22073483
Ravi M., et al. (2015). 3D cell culture systems: advantages and applications. J. Cell. Physiol. 230, 16–26. doi: 10.1002/jcp.24683
Riedl A., Schlederer M., Pudelko K., Stadler M., Walter S., Unterleuthner D., et al. (2017). Comparison of cancer cells in 2D vs 3D culture reveals differences in AKT–mTOR–S6K signaling and drug responses. J. Cell Sci. 130, 203–218. doi: 10.1242/jcs.188102
Robbins P. F., Kassim S. H., Tran T. L. N., Crystal J. S., Morgan R. A., Feldman S. A., et al. (2015). A pilot trial using lymphocytes genetically engineered with an NY-ESO-1–reactive T-cell receptor: long-term follow-up and correlates with response. Clin. Cancer Res. 21, 1019–1027. doi: 10.1158/1078-0432.CCR-14-2708
Rossi G., Manfrin A., Lutolf M. P. (2018). Progress and potential in organoid research. Nat. Rev. Genet. 19, 671–687. doi: 10.1038/s41576-018-0051-9
Rothschild S. I., Zippelius A., Eboulet E. I., Savic Prince S., Betticher D., Bettini A., et al. (2021). SAKK 16/14: durvalumab in addition to neoadjuvant chemotherapy in patients with stage IIIA (N2) non–small-cell lung cancer—a multicenter single-arm phase II trial. J. Clin. Oncol. 39, 2872–2880. doi: 10.1200/JCO.21.00276
Routy B., Le Chatelier E., Derosa L., Duong C. P. M., Alou M. T., Daillère R., et al. (2018). Gut microbiome influences efficacy of PD-1–based immunotherapy against epithelial tumors. Science 359, 91–97. doi: 10.1126/science.aan3706
Sato K., Kawakami K. (2022). PAMPs and host immune response in cryptococcal infection. Med. Mycol. J. 63, 133–138. doi: 10.3314/mmj.22.005
Shelkey E., Oommen D., Soto‐Pantoja D., Lu Y., Soker S. (2021). Immuno-reactive cancer organoid models to examine microbiome metabolite effects on immune checkpoint blockade efficacy. FASEB J. 35. doi: 10.1096/fasebj.2021.35.S1.03762
Shelkey E., Oommen D., Stirling E. R., Soto-Pantoja D. R., Cook K. L., Lu Y., et al. (2022). Immuno-reactive cancer organoid model to assess effects of the microbiome on cancer immunotherapy. Sci. Rep. 12, 9983. doi: 10.1038/s41598-022-13930-7
Shin W., Wu A., Massidda M. W., Foster C., Thomas N., Lee D.-W., et al. (2019). A robust longitudinal co-culture of obligate anaerobic gut microbiome with human intestinal epithelium in an anoxic-oxic interface-on-a-chip. Front. bioeng. Biotechnol. 7, 13. doi: 10.3389/fbioe.2019.00013
Sivan A., Corrales L., Hubert N., Williams J. B., Aquino-Michaels K., Earley Z. M., et al. (2015). Commensal Bifidobacterium promotes antitumor immunity and facilitates anti–PD-L1 efficacy. Science 350, 1084–1089. doi: 10.1126/science.aac4255
Skardal A., Murphy S. V., Devarasetty M., Mead I., Kang H.-W., Seol Y.-J., et al. (2017). Multi-tissue interactions in an integrated three-tissue organ-on-a-chip platform. Sci. Rep. 7, 8837. doi: 10.1038/s41598-017-08879-x
Spakowicz D., Bibi A., Muniak M., Williams N. F., Hoyd R., Presley C. J. (2021). The aging microbiome and response to immunotherapy: Considerations for the treatment of older adults with cancer. J. Geriatr. Oncol. 12, 985–989. doi: 10.1016/j.jgo.2021.02.001
Steele N. G., Chakrabarti J., Wang J., Biesiada J., Holokai L., Chang J., et al. (2019). An organoid-based preclinical model of human gastric cancer. Cell. Mol. Gastroenterol. Hepatol. 7, 161–184. doi: 10.1016/j.jcmgh.2018.09.008
Subia B., et al. (2015). Target specific delivery of anticancer drug in silk fibroin based 3D distribution model of bone–breast cancer cells. ACS Appl. mater. interf. 7, 2269–2279. doi: 10.1021/am506094c
Taelman J., Diaz M., Guiu J. (2022). Human intestinal organoids: promise and challenge. Front. Cell Dev. Biol. 10, 854740. doi: 10.3389/fcell.2022.854740
Tanaka C., Furihata K., Naganuma S., Ogasawara M., Yoshioka R., Taniguchi H., et al. (2022). Establishment of a mouse model of pancreatic cancer using human pancreatic cancer cell line S2-013-derived organoid. Hum. Cell 35, 735–744. doi: 10.1007/s13577-022-00684-7
Taylor J., Sellin J., Kuerschner L., Krähl L., Majlesain Y., Förster I., et al. (2020). Generation of immune cell containing adipose organoids for in vitro analysis of immune metabolism. Sci. Rep. 10, 21104. doi: 10.1038/s41598-020-78015-9
Tonneau M., Nolin-Lapalme A., Kazandjian S., Auclin E., Panasci J., Benlaifaoui M., et al. (2022). Helicobacter pylori serology is associated with worse overall survival in patients with melanoma treated with immune checkpoint inhibitors. Oncoimmunology. 11 (1), 2096535. doi: 10.1080/2162402X.2022.2096535
Torsvik A., Stieber D., Enger P. Ø., Golebiewska A., Molven A., Svendsen A., et al. (2014). U-251 revisited: genetic drift and phenotypic consequences of long-term cultures of glioblastoma cells. Cancer Med. 3, 812–824. doi: 10.1002/cam4.219
Tsai S., Mcolash L., Palen K., Johnson B., Duris C., Yang Q., et al. (2018). Development of primary human pancreatic cancer organoids, matched stromal and immune cells and 3D tumor microenvironment models. BMC Cancer 18, 335. doi: 10.1186/s12885-018-4238-4
Turna J., Patterson B., Van Ameringen M. (2017). An update on the relationship between the gut microbiome and obsessive-compulsive disorder. Psychiatr. Ann. 47, 542–551. doi: 10.3928/00485713-20171013-01
Vignali V., Hines P. A., Cruz A. G., Ziętek B., Herold R. (2022). Health horizons: Future trends and technologies from the European Medicines Agency’s horizon scanning collaborations. Front. Med. 9, 1064003. doi: 10.3389/fmed.2022.1064003
Votanopoulos K. I., Forsythe S., Sivakumar H., Mazzocchi A., Aleman J., Miller L., et al. (2019a). Appendiceal cancer patient-specific tumor organoid model for predicting chemotherapy efficacy prior to initiation of treatment: a feasibility study. Ann. Surg. Oncol. 26, 139–147. doi: 10.1245/s10434-018-7008-2
Votanopoulos K. I., Mazzocchi A., Sivakumar H., Forsythe S., Aleman J., Levine E. A., et al. (2019b). Model of patient-specific immune-enhanced organoids for immunotherapy screening: feasibility study. Ann. Surg. Oncol. 27, 1956–1967. doi: 10.1245/s10434-019-08143-8
Wan C., Keany M. P., Dong H., Al-Alem L. F., Pandya U. M., Lazo S., et al. (2021). Enhanced efficacy of simultaneous PD-1 and PD-L1 immune checkpoint blockade in high-grade serous ovarian cancer. Cancer Res. 81, 158–173. doi: 10.1158/0008-5472.CAN-20-1674
Wang W., Yuan T., Ma L., Zhu Y., Bao J., Zhao X., et al. (2022). Hepatobiliary tumor organoids reveal HLA class I neoantigen landscape and antitumoral activity of neoantigen peptide enhanced with immune checkpoint inhibitors. Adv. Sci. 9, 2105810. doi: 10.1002/advs.202105810
Wu Z., Zhang S., Li L., Huang Z., Huang D., Hu Y. (2022). The gut microbiota modulates responses to anti–PD-1 and chemotherapy combination therapy and related adverse events in patients with advanced solid tumors. Front. Oncol. 12, 887383. doi: 10.3389/fonc.2022.887383
Xia X., Li F., He J., Aji R., Gao D. (2019). Organoid technology in cancer precision medicine. Cancer Lett. 457, 20–27. doi: 10.1016/j.canlet.2019.04.039
Xiao W., Pahlavanneshan M., Eun C.-Y., Zhang X., Dekalb C., Mahgoub B., et al. (2022). Matrix stiffness mediates pancreatic cancer chemoresistance through induction of exosome hypersecretion in a cancer associated fibroblasts-tumor organoid biomimetic model. Matrix Biol. Plus 14, 100111. doi: 10.1016/j.mbplus.2022.100111
Xing F., Liu Y. C., Huang S., Lyu X., Su S. M., Chan U. I., et al. (2021). Accelerating precision anti-cancer therapy by time-lapse and label-free 3D tumor slice culture platform. Theranostics 11, 9415–9430. doi: 10.7150/thno.59533
Xu H., et al. (2018). Organoid technology and applications in cancer research. J. Hematol. Oncol. 11, 1–15. doi: 10.1186/s13045-018-0662-9
Yan H. H., Siu H. C., Law S., Ho S. L., Yue S. S., Tsui W. Y., et al. (2018). A comprehensive human gastric cancer organoid biobank captures tumor subtype heterogeneity and enables therapeutic screening. Cell Stem Cell 23, 882–897. e11. doi: 10.1016/j.stem.2018.09.016
Yee N. S., et al. (2015). Animal models of cancer biology (London, England: SAGE Publications Sage UK), CGM–S37907.
Yin H., Yang L., Peng G., Yang K., Mi Y., Hu X., et al. (2021). The commensal consortium of the gut microbiome is associated with favorable responses to anti-programmed death protein 1 (PD-1) therapy in thoracic neoplasms. Cancer Biol. Med. 18, 1040. doi: 10.20892/j.issn.2095-3941.2020.0450
Yu K., Chen B., Aran D., Charalel J., Yau C., Wolf D. M., et al. (2019). Comprehensive transcriptomic analysis of cell lines as models of primary tumors across 22 tumor types. Nat. Commun. 10, 3574. doi: 10.1038/s41467-019-11415-2
Yu L., Li Z., Mei H., Li W., Chen D., Liu L., et al. (2021). Patient-derived organoids of bladder cancer recapitulate antigen expression profiles and serve as a personal evaluation model for CAR-T cells in vitro. Clin. Trans. Immunol. 10, e1248. doi: 10.1002/cti2.1248
Zhang L., Jiang L., Yu L., Li Q., Tian X., He J., et al. (2022). Inhibition of UBA6 by inosine augments tumour immunogenicity and responses. Nat. Commun. 13, 5413. doi: 10.1038/s41467-022-33116-z
Keywords: cancer, immunotherapy, in vitro, microfluidics, hydrogels, organoid
Citation: El-Derby AM, Schaaf CR, Shelkey E, Cook KL, Votanopoulos KI and Soker S (2024) Immune-reactive tumor organoids system to determine the effects of microbial metabolites on cancer immunity and immunotherapies. Front. Microbiomes 3:1411322. doi: 10.3389/frmbi.2024.1411322
Received: 02 April 2024; Accepted: 05 September 2024;
Published: 07 October 2024.
Edited by:
Braden C. McFarland, University of Alabama at Birmingham, United StatesReviewed by:
Alireza Labani-Motlagh, Hackensack Meridian Health, United StatesShigao Huang, Air Force Medical University, China
Copyright © 2024 El-Derby, Schaaf, Shelkey, Cook, Votanopoulos and Soker. This is an open-access article distributed under the terms of the Creative Commons Attribution License (CC BY). The use, distribution or reproduction in other forums is permitted, provided the original author(s) and the copyright owner(s) are credited and that the original publication in this journal is cited, in accordance with accepted academic practice. No use, distribution or reproduction is permitted which does not comply with these terms.
*Correspondence: Shay Soker, c3Nva2VyQHdha2VoZWFsdGguZWR1
†Present address: Azza M. El-Derby, Center of Excellence for Stem Cells and Regenerative
Medicine (CESC), Zewail City of Science and Technology, 6th of October City, Giza, Egypt
Ethan Shelkey, R&D Scientist at Lonza Cell Discovery, Lonza Durham Research Triangle Park (RTP), NC, United States