- 1Centre for Ecological and Evolutionary Synthesis (CEES), Department of Biosciences, University of Oslo, Oslo, Norway
- 2Department of Anthropology and Environmental Studies Program, California State University Fullerton, Fullerton, CA, United States
- 3Department of Oncology, Division of Pediatric and Adolescent Medicine, Oslo University Hospital Rikshospitalet, Oslo, Norway
- 4Department of Pediatric Research, Division of Paediatric and Adolescent Medicine, Oslo University Hospital Rikshospitalet, Oslo, Norway
Background: There is an increasing awareness of the importance of the gut microbiome in disease progression and the maintenance of human health. However, links between the microbiome and cancer onset remain relatively unexplored. This is especially the case for childhood cancers, which although rare, are the predominant cause of death among children in Western countries.
Methods: Fecal samples were collected from patients, before the onset of treatment, by the Norwegian Childhood Cancer Biobank in Oslo and from children attending kindergartens in Oslo, Norway. Using 16S rRNA gene amplicon sequencing, we compared the gut microbiome compositions of the children diagnosed with cancer with children attending kindergarten.
Results: We observed significant differences in the relative abundances of several taxa, including a striking depletion of Faecalibacterium prausnitzii, an important taxa linked to gut health maintenance.
Conclusions: Our observations provide evidence that the gut microbiome may play an important role in physiological changes associated with the onset of childhood cancer. However, further studies should be designed in order to validate our findings. Furthermore, these results suggest that variations in the microbial community could potentially be used as an early indicator of childhood cancer.
Introduction
The adult human gastrointestinal microbiome is a stable and complex community comprised of trillions of microorganisms (Cho and Blaser, 2012). Humans are born with an essentially sterile gastrointestinal tract, with microbial colonization starting during birth, and stabilizing into an adult-like composition around three years of age (Milani et al., 2017; Kennedy et al., 2023). The establishment of the microbial community in infants has become widely recognized as a fundamental developmental process (Bokulich et al., 2016; Tamburini et al., 2016; Chu et al., 2017; Milani et al., 2017).
The human gut microbiome plays several essential roles for health and physiology of the host, including influencing the development of the immune system, protection from pathogens, and the production of vitamins and short-chain fatty acids. It has been suggested by (Levy et al., 2017), that the gut microbiome may be influenced by host factors that could be used to indicate gut dysbiosis. In the case of Ulcerative colitis (UC) and Crohn’s disease (CD), shifts in the bacterial community with depletion of Faecalibacterium prausnitzii have been found (Zhang et al., 2016) and similar shifts have been observed in colon cancer (Lopez-Siles et al., 2016).
The link between cancer and the microbiome may be causal, as some microbes are considered oncogenic (Chang and Parsonnet, 2010). However, it is still unclear whether the general composition of the gut microbiota can influence the development of cancer (Zitvogel et al., 2017), e.g. if certain microbial community profiles increase individual susceptibility to the disease. It is also possible that the composition of the gut microbiota is altered by changes in the host physiology resulting from or by cancer, with important implications for host health and treatment prospects.
Childhood cancer is still the predominant cause of death among children over a year in Western countries despite significantly improved treatment and increased knowledge of the molecular mechanisms of various cancers (Siegel et al., 2021). Pediatric cancers are entirely different in comparison to adult cancers, in terms of frequencies, clinical behavior, and the potential factors involved in the onset of the disease. Current knowledge about the role of the gut microbiome in childhood cancer is scarce (Bai et al., 2018). It has, however, been shown that the gastrointestinal microbial community differs between pediatric versus adolescent Acute Lymphomatic Leukemia (ALL) patients in comparison to a healthy control group at the time of diagnosis (Rajagopala et al., 2016), indicating that the gastrointestinal microbiome may play a role in the establishment and progression of the disease. Further, it has been speculated that in pediatric acute leukemia (AL), the perturbation of the gut microbiome may extend the actual treatment with antibiotics, chemo- or immune therapeutics and thus, contribute to long term side-effects in AL survivors (Masetti et al., 2021).
To our knowledge, this pilot study is one of the first studies investigating the gut microbiota of children diagnosed with cancer (D’Amico et al., 2022; Masetti et al., 2022 Cancers). We collected fecal samples from patients diagnosed with cancer prior to the beginning of the treatment regime. In addition, as normal controls, we obtained fecal samples from children attending two different kindergartens in Oslo. We performed 16S rRNA gene amplicon sequencing of the DNA extracted from fecal samples in order to look for differences in the gut microbiota composition between children diagnosed with cancer and a group of healthy children.
Methods
Participants
Fecal samples from children diagnosed with cancer (N=48) were collected (prior to treatment) at the hospital and frozen directly upon sampling, without any kind of preservation medium (Figure S1). Samples were then withdrawn from the Norwegian Childhood Cancer Biobank (NCCB), where biological samples are stored according to standard protocols (Hermansen et al., 2021). Sample collection began in March 2017 and was ongoing until DNA extraction and sequencing in 2020. After removal of samples due to labeling issues and low read counts, 33 patients remained. Of these, 12 had been diagnosed with tumors of the central nervous system (CNS), 13 with solid tumors (ST), and eight with leukemia (Figure S1; Table 1).
Fecal samples from healthy children were collected at home by the parents or at kindergarten by the teachers into tubes containing 95% ethanol as part of another study during the first half of 2020, in order to investigate the effects of environmental exposure on the microbiome in children. Sample storage for these samples was between seven and eight months before DNA extraction. One subpopulation included children (age 3-5 years) attending a traditional Norwegian kindergarten (n=10) and the other subpopulation was based on samples from children spending their time in a forest kindergarten (age 2-5 years). A major factor that differentiates a traditional kindergarten from a forest kindergarten is the amount of time spent outdoors in closer contact with nature and its natural variety of bacteria. Both populations of children (n=15) resided in Oslo and exhibited no obvious signs of physical ailments or cognitive deficits. In order to test for systematic bias due to sampling, three fecal samples from three individuals were divided into aliquots that were frozen with equivalent aliquots stored in ethanol (18 samples in total). For one individual, two of the frozen samples did not amplify. For that individual we only include one frozen and one ethanol stored sample in the analysis. (14 samples total, 7 frozen and 7 stored in ethanol).
DNA extraction and sequencing
Fecal DNA was extracted using the MagAttract PowerSoil DNA KF kit (Qiagen) according to the manufacturer’s instructions. Library preparation for DNA sequencing was carried out as previously described (de Muinck et al., 2017), targeting the V4 region of the 16S rRNA gene with the 515f-806r primer pair. A 2x300 paired-end sequencing reaction was performed using the MiSeq platform at the Norwegian Sequencing Centre.
Data analysis and statistics
Sequence read demultiplexing was carried out using a custom routine developed at NSC (https://github.com/nsc-norway/triple_index-demultiplexing). Further sequence data processing was performed using the Divisive Amplicon Denoising Algorithm as implemented in the dada2 v1.16 R-package (Callahan et al., 2016). All dada2 settings were default except for the filterAndTrim function where truncLen was set to 240 for read one and 160 for read two, and the max EE argument was set to two for read one and two for read two. Nine samples were excluded from further analysis in the childhood cancer cohort due to labeling issues, six were removed due to low read numbers, leaving 33 samples for analyses (Figure S1; Table 1). The total sum of sequences for these samples, after filtering, pair merging, and chimera removal, was 3,428,605 (mean 59,114 ± 29,457 s.d.). Taxonomic classification of amplicon sequence variants (ASVs) was done using the Ribosomal Database Project v16 training set (Cole et al., 2014). Singleton ASVs were removed from the data, as were ASVs that did not receive a reliable taxonomic assignment at the phylum level. This resulted in a total of 1224 ASVs (mean 124 ± 48 s.d.).
The following R packages were used: PERMANOVA was carried out using the adonis2 function in the Vegan v2.5.6, using Bray-Curtis distances. The random forest model was computed using random Forest v4.6.14 (Ho, 1995). Significance of the random forest model was calculated using the rfUtilities v2.1.5. Differential occurrence analysis of ASVs between the patient and control group based on the negative binomial distribution was carried out using DESeq2 v1.28.1 (Love et al., 2014), with reported p-values subjected to Benjamini-Hochberg correction for multiple hypothesis testing. For DESeq2 testing, we wanted to focus on prevalent ASVs that occurred in several individuals. Therefore, the count data was filtered to only include ASVs that occurred at a minimum of 100 sequence reads in a minimum of five individuals, leaving 133 ASVs.
Results
Of the 33 patients included in the final analyses, 12 had been diagnosed with tumors of the central nervous system (CNS), 13 with solid tumors (ST), and eight with leukemia (Table 1). The cohort of patients included children within an age range from newborn to 17 years. In particular in the ST group was the median age two years (inter quartile range 0-11) relative to 4.5 (3.5-6.5) for leukemia and 9.5 (3-15) for CNS tumors. A PERMANOVA model did not find any significant effect of sex (p=0.95). However, non-metric multidimensional scaling (NMDS) analysis demonstrated the large effect age had on the microbiota in this cohort (Figure S2). Yatsunenko et al. (2012) and others (Stewart et al., 2018) have reported that the gut microbiota develops an adult composition by three years of age. Thus, we divided the patients into those less than three years old and those three years or older. A PERMANOVA model including both, diagnosis and age group, did not demonstrate any significant effects on diagnosis (p=0.27), while age group was highly significant (p<0.001).
Diversity was significantly reduced (Figures S3A, B) in the patients younger than three years, both relative to those three years and older and healthy controls three years and older (henceforth referred to as controls), in terms of species richness and Shannon entropy (p<0.002 for all comparisons, Wilcoxon rank sum test). This result was in line with the expectations (e.g. Yatsunenko et al., 2012). In order to carry out a more realistic comparison with our kindergarten controls, we excluded children younger than three years (11 in total) from our patient cohort in the following analyses. Within the patients of age three years and over, there was no significant effect of age on the microbiota (p=0.57, PERMANOVA). Furthermore, all patients will henceforth be treated as a single group in the comparisons with the control group.
Children diagnosed with cancer had a significantly different microbiota composition (R2 = 0.09, p<0.001, PERMANOVA) compared to the microbiotas of the cohort of children sampled from the kindergartens (Figure 1). This result was confirmed by a machine learning approach using a random forest classification model. This resulted in a classification accuracy of 100.0%, with no misclassified samples out of 43 (p<0.001, permutation test). It should be noted that inclusion of children two years of age in both the patient and the healthy cohort did not alter the discrimination of the microbiotas between the groups (R2 = 0.09, p<0.001, PERMANOVA).
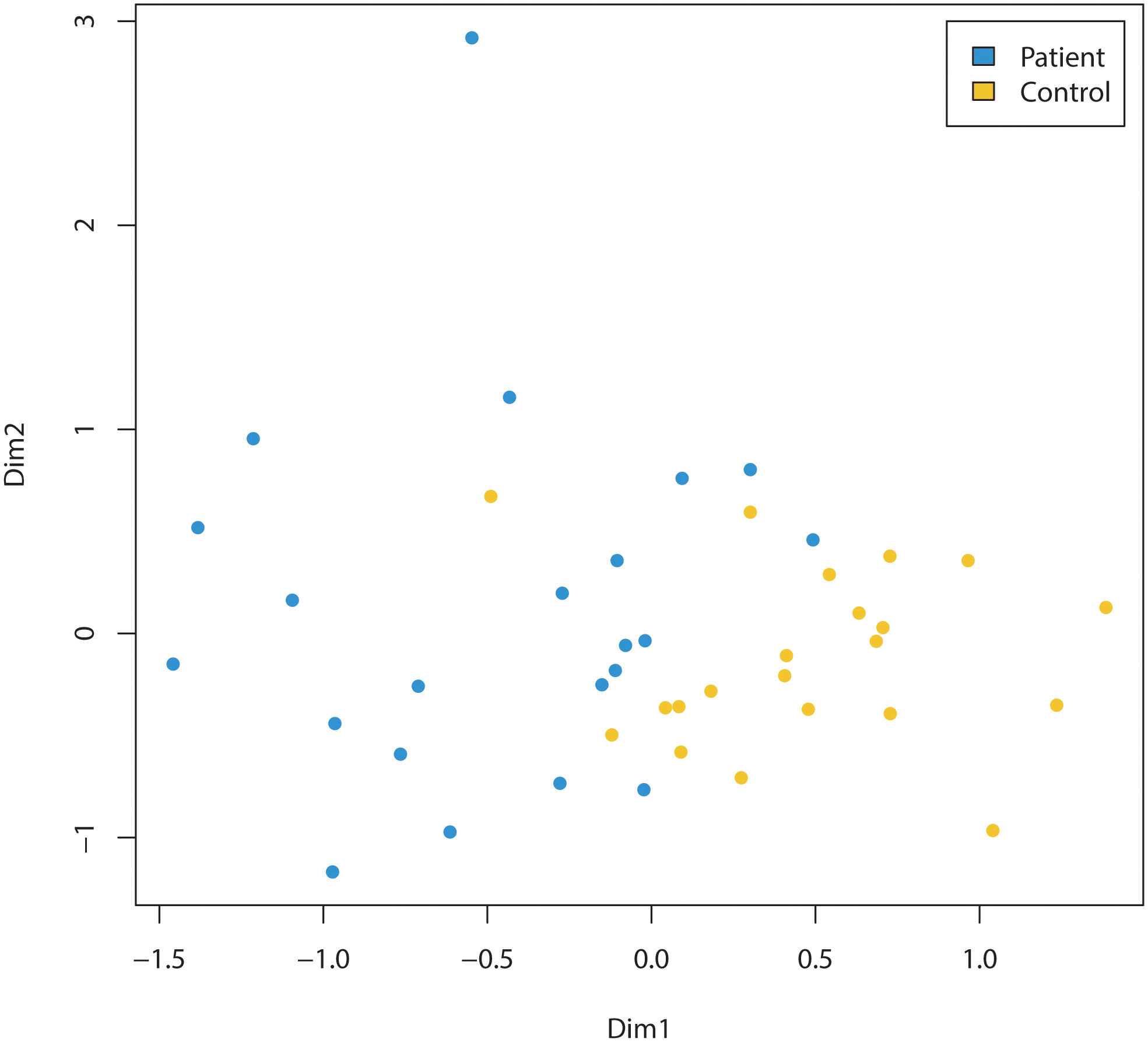
Figure 1 Comparison of the microbiomes of children (three years of age and older) diagnosed with cancer (Patient) and healthy (Control) children (three years of age and older). Non-metric multidimensional scaling of amplicon sequence variants (ASVs) relative abundance data, based on Bray-Curtis between-sample distances in children diagnosed with cancer (=patients, indicated by blue dots) compared to control cohort of children sampled from the kindergartens (=controls, indicated by yellow dots). The microbiota composition was significantly different (R2 = 0.09, p<0.001, PERMANOVA) between the two groups.
We observed a marginally higher Shannon entropy in the patient group relative to controls (p<0.09, Wilcoxon rank sum test, Figure S3B), while ASV richness did not differ significantly between the groups (Figure S3A). At the phylum level, we observed an increased mean relative abundance of Actinobacteria in the patient group (p=0.042, Wilcoxon rank sum test) (Figure S4), but no other significant differences.
ASV level analysis using DESeq2 identified 13 ASVs with significantly (Benjamini-Hochberg corrected p<0.05) differential occurrence between the patient and the control group (Table 2). six of these ASVs were depleted in children diagnosed with cancer. Strikingly, three of these eight were classified as Faecalibacterium prausnitzii (ASV2, ASV3, and ASV6). The combined relative abundance of these three ASVs were significantly higher in the control cohort (p<<0.001) (Figure 2A). All three groups were highly prevalent in the data (Figures 2B–D) and additional testing confirmed the DESeq2 results (Wilcoxon rank sum test ASV2=p<0.0001, ASV3 p<0.012, and ASV6 p<0.001). In order to investigate the robustness of our results if the young children were included, we checked that no significant relationship was observed between the relative abundance of these three ASVs and the age parameter in the patient group (Figures S5A–C). Furthermore, all three ASVs were found in both the patients and the controls. Since systematic bias could be introduced by differences in sample collection, we compared the combined relative abundances of ASVs assigned to F. prausnitzii in fecal samples that were frozen (7 samples, 3 individuals) with paired samples collected in ethanol (7 samples, 3 individuals). No statistically significant difference was observed (Wilcoxen rank sum test p=0.32). The other three ASVs depleted in patients were Haemophilus parainfluenzae, Sutterella wadsworthiensis, as well as Ruminococcus. Among the ASVs that were significantly overrepresented in the patient group, were Prevotella copri, Steptococcuss, and Dorea longicatena.
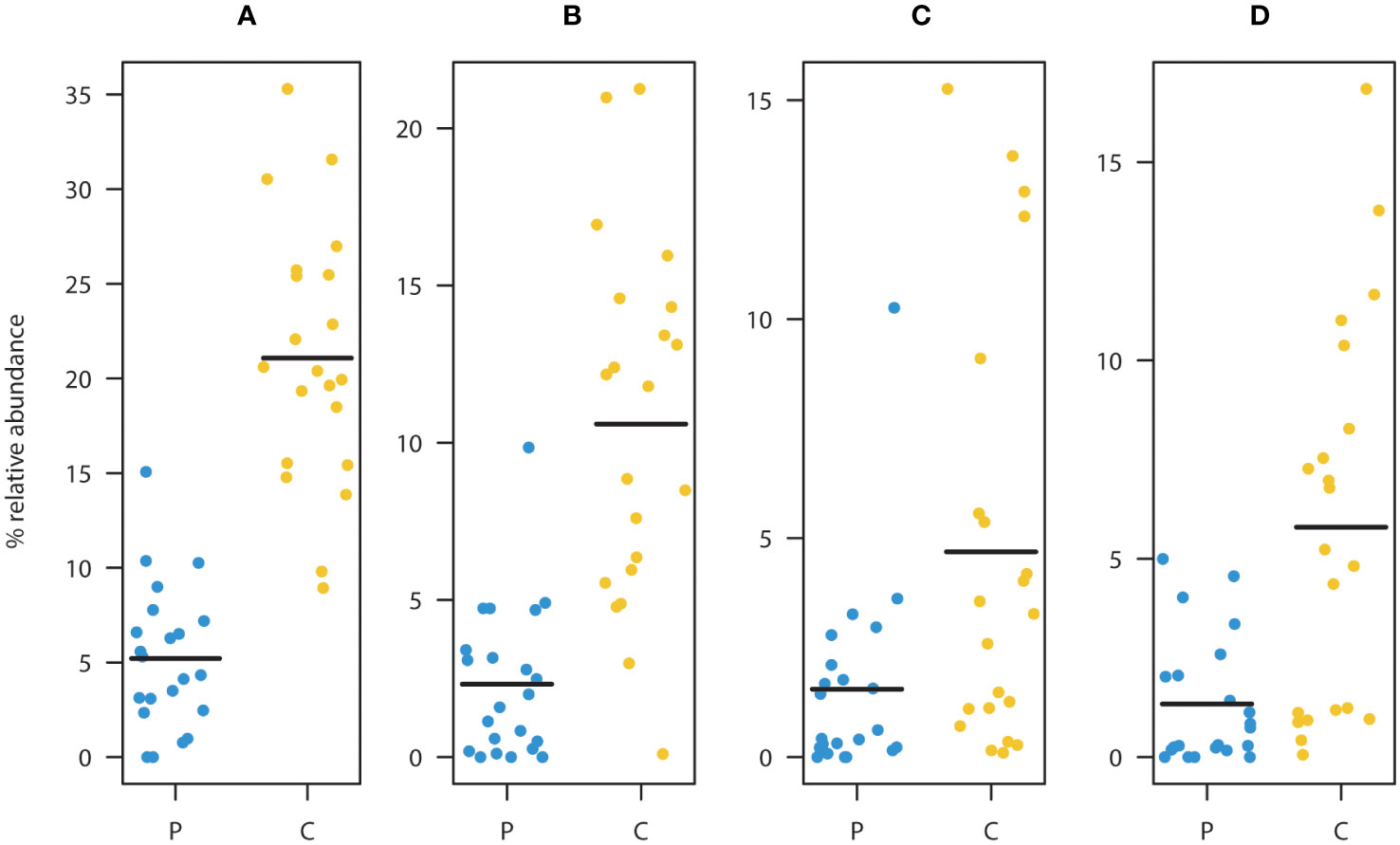
Figure 2 Comparison between patients (P) and controls (C) of the relative abundance of ASV’s assigned to as Faecalibacterium prausnitzii (A). Summed relative abundances of ASV2, ASV3, and ASV6 (main taxa assigned to F. prausnitzii). Relative abundances of ASV2 (B), ASV3 (C), and ASV6 (D). These ASVs were classified as F. prausnitzii and were found to be significantly depleted in the patient group relative to the healthy controls. (Black lines represent means, yellow dots represent the individual relative abundances of the controls three years of age and older, and blue dots represent the individual relative abundances in the children three years of age and older in the diagnosed group).
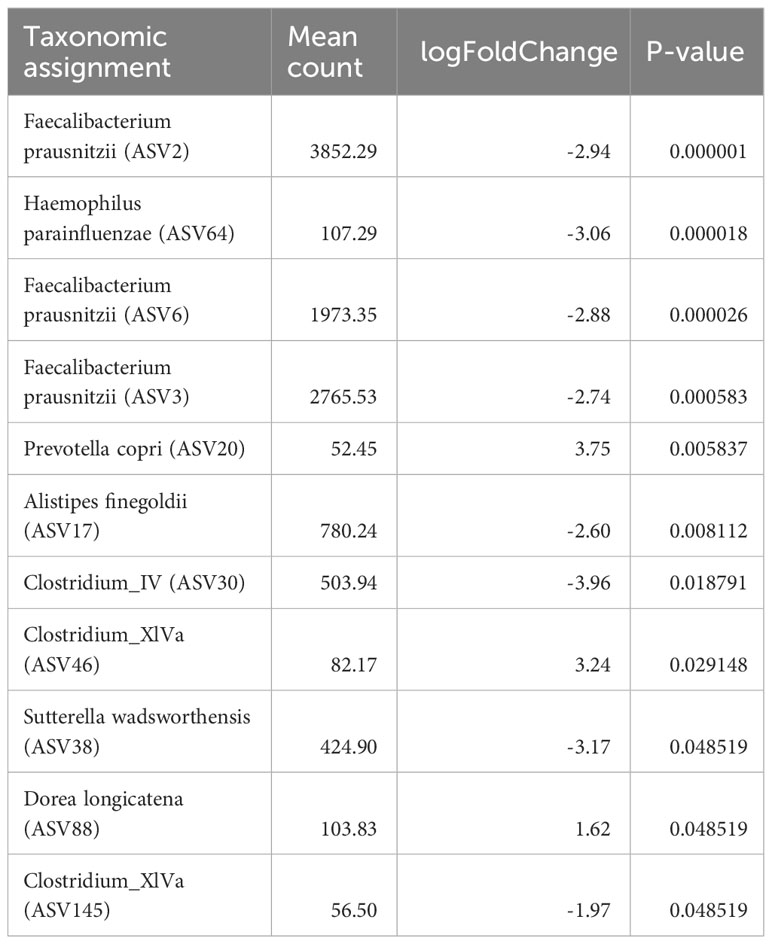
Table 2 Amplicon sequence variants (ASVs) level analysis using DESeq2 identified 11 ASVs with significant differential occurrence between the patient and the control group (Benjamini-Hochberger corrected p<0.05).
ASV2, ASV3, and ASV6 were also variables of high importance for successful discrimination between the patients and the controls in the random forest model (Figures S6A, B). The random forest model also identified the following short chain fatty acid producing taxa as being among the top 30 variables of highest importance for successful classification: Roseburia spp., Ruminococcus spp., Anaerostipes hadrus, Coprococcus comes, Intestinimonas, Lachnospiracea, Roseburia inulinivorans, and Blautia faeces.
Discussion
Outline of the study
We characterized the fecal microbiota in a cohort of children diagnosed with cancer prior to the beginning of treatment in relation to 25 healthy children attending kindergarten. The cohort of patients included children ranging from newborn to 17 years of age. While the overall age effect within that cohort was large, excluding children younger than three years removed this effect. Previous work has shown that the gut microbiota tends to stabilize at an adult-like stage by three years of age (Yatsunenko et al., 2012; Stewart et al., 2018). Thus, while not optimal, removing the youngest children from the patient cohort should largely account for age differences relative to controls. We would like to point out that the present study is a small pilot, with imperfect age matching between patients and controls, and the results presented here should be substantiated in larger scale studies.
Differences in the sampling procedure
Sampling procedures were different in the patient and the control group. Specifically, the samples from the kindergarten cohorts were collected at home by the parents or at kindergarten by the teachers into tubes containing 95% ethanol. Samples from the cohort diagnosed with cancer were collected at the hospital and frozen directly upon sampling, without any kind of preservation medium. In a study analyzing 1200 samples, the authors found that these preservation methods did not significantly interfere with the biological signature of the samples (Song et al., 2016). A second study by Moossavi et al. (2019), found consistent microbiota profiles in samples preserved in ethanol and by freezing. This study also did not observe discernible differences in Faecalibacterium spp. abundances. Similar conclusions have been drawn in other studies (Blekhman et al., 2016; Byrd et al., 2019; Marotz et al., 2021).
Decreased relative abundance of Faecalibacterium prausnitzii in the diagnosed cohort
The most striking result was the depletion of three abundant ASVs classified as F. prausnitzii in children diagnosed with cancer (Figure 2). The depletion was highly significant for all three strains and overall F. prausnitzii was decreased by a factor of four in patients relative to the controls. F. prausnitzii is a dominant member of the human microbiota and a lowered relative abundance can correlate with negative health outcomes, like inflammatory bowel disease and colorectal cancer (Lopez-Siles et al., 2016; Lopez-Siles et al., 2017). Due to its high butyrate production and anti-inflammatory properties, it is considered to present a promising candidate for probiotic formulations (Ferreira-Halder et al., 2017). Short chain fatty acids (acetate, propionate and butyrate) (Reviewed in Blaak et al., 2020) are produced by bacteria in the colon and are important in vital gut processes, like gastrointestinal motility and energy utilization. For example, butyrate is the main nutrient source for colonocytes and it has been shown to have protective properties against colorectal cancer (Archer et al., 1998). Furthermore, butyrate can reduce inflammation of the intestinal mucosa through regulation of immune signaling (Reviewed in Deleu et al., 2021). F. prausnitzii is one of the most important butyrate producers in the gastrointestinal tract and thus of high interest in biomedical research. Modulation of this species, as well as other short chain fatty acid (SCFA) producers, may have consequences for treatment efficacy in cancer. Here in this study, several other SCFA producing taxa were identified as important for classification of microbiota profiles into the patient and the control group.
Our results indicate that changes in the microbial community involved in SCFA production in the gut may function as an early indicator of the initiation or presence of cancer. In particular, the butyrate producing F. prausnitzii was depleted by a factor of four in the patient group. F. prausnitzii reduction has also been reported in gastrointestinal conditions, like inflammatory bowel disease (Zhang et al., 2016), indicating that this species could be a more general indicator of disease-associated dysbiosis. From the data presented here, it is unclear whether F. prausnitzii depletion results from cancer, or if a dysbiotic microbiome can actually play a role in the development of disease. While the main results from this pilot study seem quite robust, it should be noted that there are clear limitations to this study that may affect the generalizability of the conclusions. Childhood cancer is relatively rare and study recruitment is challenging. This has affected the scale of this study and has implications for the statistical power. Furthermore, samples were collected from patients at a single pediatric ward which could also introduce bias due to a shared sampling environment. Lastly, although we were unable to detect differences in F. prausnitzii relative abundances due to sample storage methods, this can a source of bias and future studies should strive to have a more consistent sampling regime. Other factors are known to influence the composition of the gut microbiome and should be addressed in the design of future, larger studies, including questionnaires about cancer symptoms at diagnosis, body mass index and diet.
Data availability statement
The datasets presented in this study can be found in online repositories. The names of the repository/repositories and accession number(s) can be found below: https://www.ncbi.nlm.nih.gov/genbank/, PRJNA814879.
Ethics statement
The studies involving humans were approved by Regional Committee for Medical and Health Research Ethics (REK nr: 2018/2491 and 2019/66945). The studies were conducted in accordance with the local legislation and institutional requirements. Written informed consent for participation in this study was provided by the participants’ legal guardians/next of kin.
Author contributions
LB and MM-K initiated the overall study. LB and MM-K conceived and designed the childhood cancer, EM, PT, NN, and PF the control study. EM, PT, NN, PF, VS, and JH tested and established various methods for the study. NR and JH collected, prepared and managed the samples from children diagnosed with cancer, EM, NN, and PF from children of the control study from Norwegian kindergartens. EM, PT, and VS performed DNA extraction and sequencing. EM and PT established the analysis pipeline and carried out the data analysis. EM, PT, MM-K, and LB interpreted the data. EM and PT wrote the draft of the manuscript, JH, MM-K, NN, and PJ critical reviewed the manuscript, and EM and LB finalized it. All authors read and approved the final manuscript. All authors contributed to the article.
Funding
For collection and handling of the samples provided by the NCCB, we received funding from The Norwegian Childhood Cancer Society, the foundations of the Oslo University Hospital (Fondsstiftelsen and Barnestiftelsen). For the kindergarten study, we received funding from the U.S.-Norway Fulbright Foundation. For the sample sequencing and data processing, funds were provided by the Norwegian Research Council under grant number 300948. The funding bodies had no direct influence in the design of the study and collection, analysis, or interpretation of data or in writing the manuscript.
Acknowledgments
We wish to thank all participants, their parents, and the kindergarten teachers for their help and valuable contributions.
Conflict of interest
The authors declare that the research was conducted in the absence of any commercial or financial relationships that could be construed as a potential conflict of interest.
Publisher’s note
All claims expressed in this article are solely those of the authors and do not necessarily represent those of their affiliated organizations, or those of the publisher, the editors and the reviewers. Any product that may be evaluated in this article, or claim that may be made by its manufacturer, is not guaranteed or endorsed by the publisher.
Supplementary material
The Supplementary Material for this article can be found online at: https://www.frontiersin.org/articles/10.3389/frmbi.2023.1151889/full#supplementary-material
Abbreviations
ALL, Acute Lymphomatic Leukemia; ASV, amplicon sequence variant; CD, Crohn’s disease; F. prausnitzii, Faecalibacterium prausnitzii; NCCB, Norwegian Childhood Cancer Biobank; CNS, central nervous system; NMDS, non-metric multidimensional scaling; ST, solid tumor; and UC, Ulcerative colitis.
References
Archer S., Meng S., Wu J., Johnson J., Tang R., Hodin R. (1998). Butyrate inhibits colon carcinoma cell growth through two distinct pathways. Surgery 124 (2). doi: 10.1016/S0039-6060(98)70127-8
Bai J., Behera M., Bruner D. W. (2018). The gut microbiome, symptoms, and targeted interventions in children with cancer: a systematic review. Support Care Cancer 26 (2), 427–439. doi: 10.1007/s00520-017-3982-3
Blaak E. E., Canfora E. E., Theis S., Frost G., Groen A. K., Mithieux G., et al. (2020). Short chain fatty acids in human gut and metabolic health. Benef Microbes 11 (5), 411–455. doi: 10.3920/BM2020.0057
Blekhman R., Tang K., Archie E. A., Barreiro L. B., Johnson Z. P., Wilson M. E., et al. (2016). Common methods for fecal sample storage in field studies yield consistent signatures of individual identity in microbiome sequencing data. Sci. Rep. 6, 31519. doi: 10.1038/srep31519
Bokulich N. A., Chung J., Battaglia T., Henderson N., Jay M., Li H., et al. (2016). Antibiotics, birth mode, and diet shape microbiome maturation during early life. Sci. Transl. Med. 8 (343), 343ra382. doi: 10.1126/scitranslmed.aad7121
Byrd D. A., Chen J., Vogtmann E., Hullings A., Song S. J., Amir A., et al. (2019). Reproducibility, stability, and accuracy of microbial profiles by fecal sample collection method in three distinct populations. PloS One 14 (11), e0224757. doi: 10.1371/journal.pone.0224757
Callahan B. J., McMurdie P. J., Rosen M. J., Han A. W., Johnson A. J., Holmes S. P. (2016). DADA2: High-resolution sample inference from Illumina amplicon data. Nat. Methods 13 (7), 581–583. doi: 10.1038/nmeth.3869
Chang A. H., Parsonnet J. (2010). Role of bacteria in oncogenesis. Clin. Microbiol. Rev. 23 (4), 837–857. doi: 10.1128/CMR.00012-10
Cho I., Blaser M. J. (2012). The human microbiome: at the interface of health and disease. Nat. Rev. Genet. 13 (4), 260–270. doi: 10.1038/nrg3182
Chu D. M., Ma J., Prince A. L., Antony K. M., Seferovic M. D., Aagaard K. M. (2017). Maturation of the infant microbiome community structure and function across multiple body sites and in relation to mode of delivery. Nat. Med. 23 (3), 314–326. doi: 10.1038/nm.4272
Cole J. R., Wang Q., Fish J. A., Chai B., McGarrell D. M., Sun Y., et al. (2014). Ribosomal Database Project: data and tools for high throughput rRNA analysis. Nucleic Acids Res. 42 (Database issue), D633–D642. doi: 10.1093/nar/gkt1244
D’Amico F., Decembrino N., Muratore E., Turroni S., Muggeo P., Mura R., et al. (2022). Oral lactoferrin supplementation during induction chemotherapy promotes gut microbiome eubiosis in pediatric patients with hematologic Malignancies. Pharmaceutics 14, 1705. doi: 10.3390/pharmaceutics14081705
Deleu S., Machiels K., Raes J., Verbeke K., Vermeire S. (2021). Short chain fatty acids and its producing organisms: An overlooked therapy for IBD? EBioMedicine 66, 103293. doi: 10.1016/j.ebiom.2021.103293
de Muinck E. J., Trosvik P., Gilfillan G. D., Hov J. R., Sundaram A. Y. M. (2017). A novel ultra high-throughput 16S rRNA gene amplicon sequencing library preparation method for the Illumina HiSeq platform. Microbiome 5 (1), 68. doi: 10.1186/s40168-017-0279-1
Ferreira-Halder C. V., Faria A. V. S., Andrade S. S. (2017). Action and function of Faecalibacterium prausnitzii in health and disease. Best Pract. Res. Clin. Gastroenterol. 31 (6), 643–648. doi: 10.1016/j.bpg.2017.09.011
Hermansen J. U., Wojcik D. M., Robinson N., Pahnke J., Haugland H. K., Jamtoy A. H., et al. (2021). The Norwegian childhood cancer biobank. Cancer Rep. (Hoboken) 5 (8), e1555. doi: 10.1002/cnr2.1555
Ho T. K. (1995). “Random decision forests,” in Proceedings of 3rd international conference on document analysis and recognition Montreal, QC, Canada, Vol. 1. 278–282. doi: 10.1109/ICDAR.1995.598994
Kennedy K. M., de Goffau M. C., Perez-Muñoz M. E., Arrieta M. C., Bäckhed F., Bork P., et al. (2023). Questioning the fetal microbiome illustrates pitfalls of low-biomass microbial studies. Nature 613 (7945), 639–649. doi: 10.1038/s41586-022-05546-8
Levy M., Kolodziejczyk A. A., Thaiss C. A., Elinav E. (2017). Dysbiosis and the immune system. Nat. Rev. Immunol. 17 (4), 219–232. doi: 10.1038/nri.2017.7
Lopez-Siles M., Duncan S. H., Garcia-Gil L. J., Martinez-Medina M. (2017). Faecalibacterium prausnitzii: from microbiology to diagnostics and prognostics. ISME J. 11 (4), 841–852. doi: 10.1038/ismej.2016.176
Lopez-Siles M., Martinez-Medina M., Suris-Valls R., Aldeguer X., Sabat-Mir M., Duncan S. H., et al. (2016). Changes in the abundance of faecalibacterium prausnitzii phylogroups I and II in the intestinal mucosa of inflammatory bowel disease and patients with colorectal cancer. Inflammation Bowel Dis. 22 (1), 28–41. doi: 10.1097/MIB.0000000000000590
Love M. I., Huber W., Anders S. (2014). Moderated estimation of fold change and dispersion for RNA-seq data with DESeq2. Genome Biol. 15 (12), 550. doi: 10.1186/s13059-014-0550-8
Marotz C., Cavagnero K. J., Song S. J., McDonald D., Wandro S., Humphrey G., et al. (2021). Evaluation of the effect of storage methods on fecal, saliva, and skin microbiome composition. mSystems 6 (2), e01329-20. doi: 10.1128/mSystems.01329-20
Masetti R., D’Amico F., Zama D., Leardini D., Muratore E., Ussowicz M., et al. (2022). Febrile neutropenia duration is associated with the severity of gut microbiota dysbiosis in pediatric allogeneic hematopoietic stem cell transplantation recipients. Cancers 14, 1932. doi: 10.3390/cancers14081932
Masetti R., Muratore E., Leardini D., Zama D., Turroni S., Brigidi P., et al. (2021). Gut microbiome in pediatric acute leukemia: from predisposition to cure. Blood Adv. 5 (22), 4619–4629. doi: 10.1182/bloodadvances.2021005129
Milani C., Duranti S., Bottacini F., Casey E., Turroni F., Mahony J., et al. (2017). The first microbial colonizers of the human gut: composition, activities, and health implications of the infant gut microbiota. Microbiol. Mol. Biol. Rev. 81 (4), e00036-17. doi: 10.1128/MMBR.00036-17
Moossavi S., Engen P. A., Ghanbari R., Green S. J., Naqib A., Bishehsari F., et al. (2019). Assessment of the impact of different fecal storage protocols on the microbiota diversity and composition: a pilot study. BMC Microbiol. 19 (1), 145. doi: 10.1186/s12866-019-1519-2
Rajagopala S. V., Yooseph S., Harkins D. M., Moncera K. J., Zabokrtsky K. B., Torralba M. G., et al. (2016). Gastrointestinal microbial populations can distinguish pediatric and adolescent Acute Lymphoblastic Leukemia (ALL) at the time of disease diagnosis. BMC Genomics 17 (1), 635. doi: 10.1186/s12864-016-2965-y
Siegel R. L., Miller K. D., Fuchs H. E., Jemal A. (2021). Cancer statistics 2021. CA Cancer J. Clin. 71 (1), 7–33. doi: 10.3322/caac.21654
Song S. J., Amir A., Metcalf J. L., Amato K. R., Xu Z. Z., Humphrey G., et al. (2016). Preservation methods differ in fecal microbiome stability, affecting suitability for field studies. mSystems 1 (3), e00021-16. doi: 10.1128/mSystems.00021-16
Stewart C. J., Ajami N. J., O'Brien J. L., Hutchinson D. S., Smith D. P., Wong M. C., et al. (2018). Temporal development of the gut microbiome in early childhood from the TEDDY study. Nature 562 (7728), 583–588. doi: 10.1038/s41586-018-0617-x
Tamburini S., Shen N., Wu H. C., Clemente J. C. (2016). The microbiome in early life: implications for health outcomes. Nat. Med. 22 (7), 713–722. doi: 10.1038/nm.4142
Yatsunenko T., Rey F. E., Manary M. J., Trehan I., Dominguez-Bello M. G., Contreras M., et al. (2012). Human gut microbiome viewed across age and geography. Nature 486 (7402), 222–227. doi: 10.1038/nature11053
Zhang Q., Wu Y., Wang J., Wu G., Long W., Xue Z., et al. (2016). Accelerated dysbiosis of gut microbiota during aggravation of DSS-induced colitis by a butyrate-producing bacterium. Sci. Rep. 6, 27572. doi: 10.1038/srep27572
Keywords: cancer, children, fecal, gastrointestinal, gut, microbiome, microbiota Norway
Citation: de Muinck EJ, Trosvik P, Nguyen N, Fashing PJ, Stigum VM, Robinson N, Hermansen JU, Munthe-Kaas MC and Baumbusch LO (2023) Reduced abundance of Faecalibacterium prausnitzii in the gut microbiota of children diagnosed with cancer, a pilot study. Front. Microbiomes 2:1151889. doi: 10.3389/frmbi.2023.1151889
Received: 26 January 2023; Accepted: 23 October 2023;
Published: 07 November 2023.
Edited by:
Susana Fuentes, National Institute for Public Health and the Environment, NetherlandsReviewed by:
Howard A. Young, National Cancer Institute at Frederick (NIH), United StatesShenghui Li, Puensum Genetech Institute, China
Copyright © 2023 de Muinck, Trosvik, Nguyen, Fashing, Stigum, Robinson, Hermansen, Munthe-Kaas and Baumbusch. This is an open-access article distributed under the terms of the Creative Commons Attribution License (CC BY). The use, distribution or reproduction in other forums is permitted, provided the original author(s) and the copyright owner(s) are credited and that the original publication in this journal is cited, in accordance with accepted academic practice. No use, distribution or reproduction is permitted which does not comply with these terms.
*Correspondence: Eric J. de Muinck, ZS5qLmRlLm11aW5ja0BpYnYudWlvLm5v