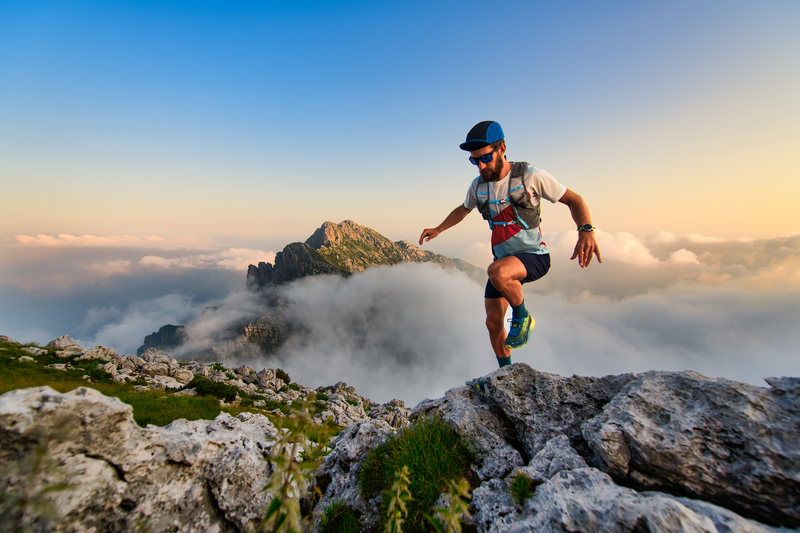
95% of researchers rate our articles as excellent or good
Learn more about the work of our research integrity team to safeguard the quality of each article we publish.
Find out more
ORIGINAL RESEARCH article
Front. Microbiol.
Sec. Microbe and Virus Interactions with Plants
Volume 16 - 2025 | doi: 10.3389/fmicb.2025.1554406
This article is part of the Research Topic Microbial Stress Mitigation and Crop Improvement Using Multiomics Holistic Approach View all 4 articles
The final, formatted version of the article will be published soon.
You have multiple emails registered with Frontiers:
Please enter your email address:
If you already have an account, please login
You don't have a Frontiers account ? You can register here
Psammosilene tunicoides is a plant with significant medicinal and ecological value, exhibiting remarkable medicinal properties, particularly in anti-inflammatory, antioxidant, and immuneregulatory effects. Root rot is one of the primary diseases affecting Psammosilene tunicoides, leading to a significant decline in its quality. In this study, we utilized an integrated analysis of transcriptomics, metabolomics, high-throughput amplicon sequencing, and culturomics for revealing the difference of healthy samples (CH) and diseased samples (CD) and studying the defense mechanism of P. tunicoides in resisting root rot. Transcriptome revealed distinct patterns of gene expression between healthy root samples (HR) and diseased root samples (DR) of P. tunicoides. The Key enzyme genes involved in triterpene (e.g., HMGS, DXS, SQS, CYP450) and flavonoid (e.g., PAL, CHS, CHI) biosynthesis pathways were significantly upregulated in DR. Consistent results were observed in the metabolomic analysis, where triterpene saponins and flavonoids were more highly accumulated in DR than in HR. Microbiome data indicated a significant enrichment of Actinobacteria at the genus level in the rhizosphere soil of diseased samples (DS) compared to healthy samples (HS) while the mostly beneficial growth-promoting bacterial groups were found in DR root endophytes, including Enterobacter, Pseudomonas, Klebsiella, Stenotrophomonas and Bacillus. Through culturomics, we successfully isolated and identified over 220 bacterial strains from the rhizosphere soil of diseased samples, including genera including Bacillus, Streptomyces, Cupriavidus, Pseudomonas, and Paenarthrobacter. Notably, the strain Pseudomonas sp., which was significantly enriched in DR, exhibited a clear antagonistic effect against Fusarium oxysporum. Cooccurrence network analysis of multi-omics data revealed that many Actinomycetes positively correlated with triterpenoid and flavonoid compounds and their key genes. Therefore, we conclude that these secondary metabolites may could resist pathogen invasion directly or serve as an "intermediate medium" to recruit growth-promoting microorganisms to resistant the root rot. This study explores the "Plant-Microbe" interaction network underlying root rot resistance in P. tunicoides, and this network should be considered a sustainable tool for future ecological cultivation and management of P. tunicoides. This study investigates the 'Plant-Microbe' interaction network associated with root rot resistance in P. tunicoides, revealing its significant implications for the ecological cultivation and management of this species.
Keywords: root rot1, Psammosilene tunicoides2, multi-omics3, "Plant-Microbe" interaction network4, Plant defense mechanisms5, Root microbiome6
Received: 02 Jan 2025; Accepted: 31 Mar 2025.
Copyright: © 2025 tao, Feng, juan, yun, Zhou, ping and Li. This is an open-access article distributed under the terms of the Creative Commons Attribution License (CC BY). The use, distribution or reproduction in other forums is permitted, provided the original author(s) or licensor are credited and that the original publication in this journal is cited, in accordance with accepted academic practice. No use, distribution or reproduction is permitted which does not comply with these terms.
* Correspondence:
ZHANG Wen ping, Yunnan University of Traditional Chinese Medicine, Kunming, China
ZHANG Ai Li, Yunnan University of Traditional Chinese Medicine, Kunming, China
Disclaimer: All claims expressed in this article are solely those of the authors and do not necessarily represent those of their affiliated organizations, or those of the publisher, the editors and the reviewers. Any product that may be evaluated in this article or claim that may be made by its manufacturer is not guaranteed or endorsed by the publisher.
Research integrity at Frontiers
Learn more about the work of our research integrity team to safeguard the quality of each article we publish.