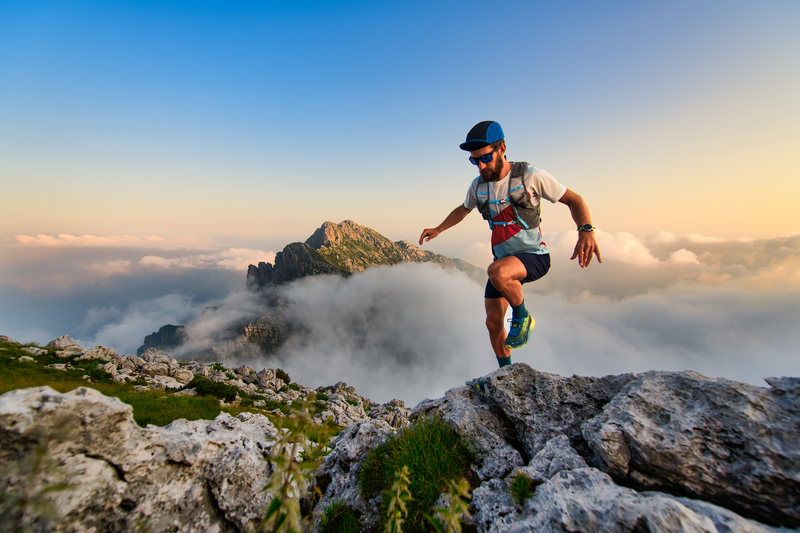
94% of researchers rate our articles as excellent or good
Learn more about the work of our research integrity team to safeguard the quality of each article we publish.
Find out more
ORIGINAL RESEARCH article
Front. Microbiol.
Sec. Food Microbiology
Volume 16 - 2025 | doi: 10.3389/fmicb.2025.1553885
The final, formatted version of the article will be published soon.
You have multiple emails registered with Frontiers:
Please enter your email address:
If you already have an account, please login
You don't have a Frontiers account ? You can register here
Guidelines for combinations of product characteristics to prevent unacceptable growth of Bacillus cereus in foods are lacking and models are therefore valuable to predict these responses. B. cereus isolates of dairy origin were used to generate a comprehensive dataset to develop two cardinal parameter growth and growth boundary models for, respectively, mesophilic and psychrotolerant B. cereus. Each model incorporated the inhibitory effect of eleven environmental factors i.e. temperature, pH, NaCl/aw, organic acids (acetic, benzoic, citric, lactic, and sorbic), phosphate salts (ortho-, di-, and tri-phosphates), and the effect of interaction between these factors. Cardinal parameter values for mesophilic or psychrotolerant strain cocktails were estimated using, respectively, 231 and 203 maximum specific growth rates (μmax values), generated in a standard liquid laboratory medium (BHI broth). Furthermore, an additional 113 and 100 μmax values were generated for the two strain cocktails using a dairy-specific liquid medium (an ultra-filtration permeate from whey) to evaluate growth responses obtained in BHI broth. Cardinal parameter values for the two extensive growth boundary models were selected conservatively using data from BHI broth or UF permeate, such that the widest growth range was obtained for each environmental factor.The studied cocktail of six vegetative mesophilic B. cereus isolates exhibited greater acid tolerance in UF permeate than in BHI broth with lower pHmin (pHmin values of 4.75 versus 4.98), higher minimum inhibitory concentrations (MIC) of undissociated lactic acid (MICu,LAC of 2.99 versus 2.34 mM) and of total citric acid (MICT,CAC of 169.1 versus 82.5 mM). The psychrotolerant B. cereusstrain cocktail also had lower pHmin and higher values for MICLAC and MICT,CAC in UF permeate compared to BHI broth. The remaining cardinal parameter values were determined from growth rates in BHI broth.The two new models can predict the combined effect of storage temperature and a wide range of dairy product characteristics including combinations of organic acids and phosphate melting salts.These growth and growth boundary models can support the evaluation and management of the two B. cereus subgroups in various dairy products. However, product validation of the two predictive models is required to determine their performance and range of applicability.
Keywords: predictive modelling, Cardinal parameters, Organic acids, Phosphate salts, growth boundaries, Food Safety
Received: 31 Dec 2024; Accepted: 24 Feb 2025.
Copyright: © 2025 Maktabdar, Wemmenhove, Gkogka and Dalgaard. This is an open-access article distributed under the terms of the Creative Commons Attribution License (CC BY). The use, distribution or reproduction in other forums is permitted, provided the original author(s) or licensor are credited and that the original publication in this journal is cited, in accordance with accepted academic practice. No use, distribution or reproduction is permitted which does not comply with these terms.
* Correspondence:
Paw Dalgaard, Food Microbiology and Hygiene, National Food Institute, Technical University of Denmark, Kgs Lyngby, Denmark
Disclaimer: All claims expressed in this article are solely those of the authors and do not necessarily represent those of their affiliated organizations, or those of the publisher, the editors and the reviewers. Any product that may be evaluated in this article or claim that may be made by its manufacturer is not guaranteed or endorsed by the publisher.
Research integrity at Frontiers
Learn more about the work of our research integrity team to safeguard the quality of each article we publish.