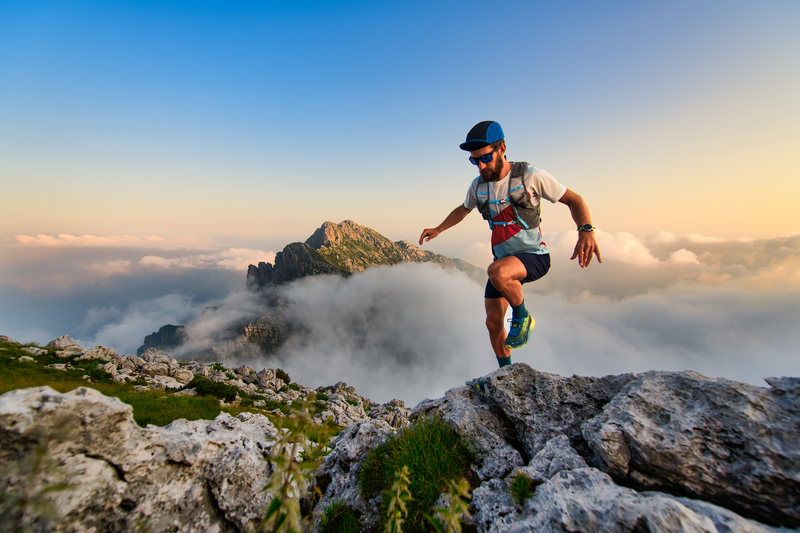
94% of researchers rate our articles as excellent or good
Learn more about the work of our research integrity team to safeguard the quality of each article we publish.
Find out more
ORIGINAL RESEARCH article
Front. Microbiol.
Sec. Microorganisms in Vertebrate Digestive Systems
Volume 16 - 2025 | doi: 10.3389/fmicb.2025.1547348
This article is part of the Research Topic Gut Microbiota's Role in High-Altitude Animal Adaptation View all 17 articles
The final, formatted version of the article will be published soon.
You have multiple emails registered with Frontiers:
Please enter your email address:
If you already have an account, please login
You don't have a Frontiers account ? You can register here
Gut microbes are essential for host nutrition, immunity, and development. Various factors influence the composition and function of the gut microbial community. However, there is limited knowledge regarding the comparison of gut microbiota across different deer species, particularly those in the World Deer Park of Baotou (Inner Mongolia, China). This study utilized 16S rRNA gene amplicon sequencing to analyze the fecal microbiota and potential microbial function in Pè re David's Deer (Elaphurus davidianus), Sika deer (Cervus nippon), American Wapiti (Cervus canadensis), Red Deer (Cervus elaphus), Fallow Deer (Dama dama), and Reindeer (Rangifer tarandus). The findings indicated no significant differences in alpha diversity, yet there was a noteworthy distinction in beta diversity among the six deer groups. At the phylum level, the predominant bacteria in the deer populations were Firmicutes, Bacteroidetes, and Proteobacteria. At the genus level, 54 core bacterial microbiota were identified. The top four genera in AW, FD, PD, and SD were Ruminococcaceae UCG-005, Rikenellaceae RC9 gut group, Ruminococcaceae UCG-010 and Christensenellaceae R-7 group. The results of the neutral model revealed that neutral processes predominantly governed the gut microbiota community assembly in different deer species, particularly in Père David's deer. PICRUSt2 predictions showed significant enrichment of fecal bacterial functions related to fatty acid, lipid, metabolic regulator, and amino acid biosynthesis. This comparative analysis sheds light on the microbial community structure, community assembly, and potential functions, offering improved insights into the management and conservation of deer species, especially Père David's deer. Future research might focus on exploring metagenomic functions and dynamics in wild settings or across different seasons using metagenomics or metatranscriptomics.
Keywords: Père david's deer, Gut Microbiota, 16S rRNA gene, Metabolic pathways, conservation
Received: 18 Dec 2024; Accepted: 10 Mar 2025.
Copyright: © 2025 Zhao, Yang, Zhao and Bai. This is an open-access article distributed under the terms of the Creative Commons Attribution License (CC BY). The use, distribution or reproduction in other forums is permitted, provided the original author(s) or licensor are credited and that the original publication in this journal is cited, in accordance with accepted academic practice. No use, distribution or reproduction is permitted which does not comply with these terms.
* Correspondence:
Lige Bai, College of Ecology and Environment, Baotou Teachers' College, Baotou, China
Disclaimer: All claims expressed in this article are solely those of the authors and do not necessarily represent those of their affiliated organizations, or those of the publisher, the editors and the reviewers. Any product that may be evaluated in this article or claim that may be made by its manufacturer is not guaranteed or endorsed by the publisher.
Research integrity at Frontiers
Learn more about the work of our research integrity team to safeguard the quality of each article we publish.