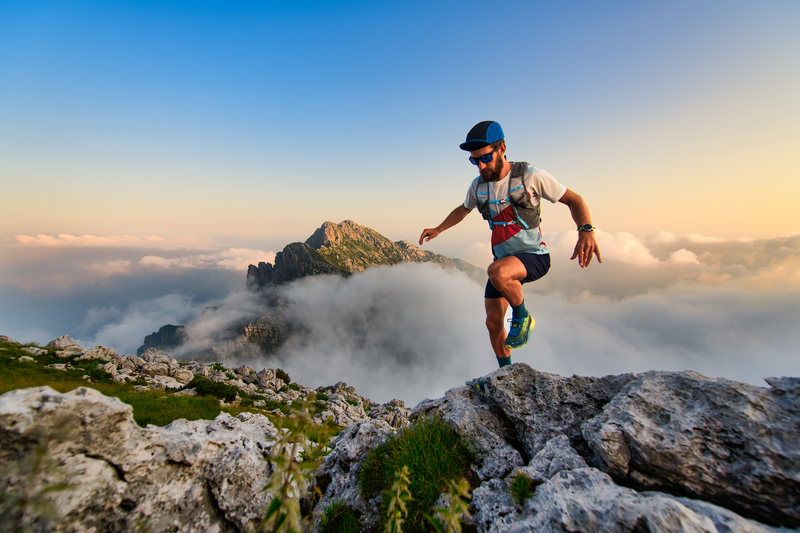
94% of researchers rate our articles as excellent or good
Learn more about the work of our research integrity team to safeguard the quality of each article we publish.
Find out more
REVIEW article
Front. Microbiol.
Sec. Systems Microbiology
Volume 16 - 2025 | doi: 10.3389/fmicb.2025.1520227
The final, formatted version of the article will be published soon.
You have multiple emails registered with Frontiers:
Please enter your email address:
If you already have an account, please login
You don't have a Frontiers account ? You can register here
Quorum Sensing (QS) and Machine Learning (ML) hybrid systems represent a groundbreaking innovation in synthetic biology, offering unprecedented control and adaptability in microbial gene regulation and metabolic processes. QS, a microbial communication mechanism, is crucial for coordinating gene expression in response to population density, impacting behaviors such as biofilm formation, virulence, and resource optimization. However, traditional QS systems are constrained by their reliance on static, pre-programmed feedback loops, limiting their flexibility in dynamic, complex environments. This review highlights how integrating advanced ML algorithms-such as reinforcement learning and deep learning-into QS systems can overcome these limitations by enabling real-time data processing, predictive modeling, and dynamic feedback control. Through these innovations, QS-ML systems can autonomously adjust gene expression and metabolic outputs, making them more efficient and scalable in applications ranging from pathogen control to precision medicine and industrial biomanufacturing. Key case studies illustrate the successful deployment of QS-ML systems to combat antimicrobial resistance, optimize bio-production, and enhance therapeutic precision in cancer and immune modulation.Despite the clear advantages, challenges remain in data integration, system robustness, and regulatory oversight. Addressing these hurdles through interdisciplinary collaboration and developing scalable, multi-omics data platforms will be critical for advancing QS-ML systems from experimental settings to real-world applications. This review underscores the transformative potential of QS-ML systems in revolutionizing synthetic biology, with profound implications for personalized medicine, sustainable biomanufacturing, and environmental health.
Keywords: quorum sensing (QS), Machine Learning (ML), Hybrid systems, Synthetic Biology, Real-time data processing, Predictive Modeling, gene regulation, adaptive systems
Received: 11 Nov 2024; Accepted: 17 Feb 2025.
Copyright: © 2025 Tuan, Uyen, Ho and Vo Thien Nhan. This is an open-access article distributed under the terms of the Creative Commons Attribution License (CC BY). The use, distribution or reproduction in other forums is permitted, provided the original author(s) or licensor are credited and that the original publication in this journal is cited, in accordance with accepted academic practice. No use, distribution or reproduction is permitted which does not comply with these terms.
* Correspondence:
Dang Anh Tuan, University of Chemistry and Technology in Prague, Prague, Czechia
Disclaimer: All claims expressed in this article are solely those of the authors and do not necessarily represent those of their affiliated organizations, or those of the publisher, the editors and the reviewers. Any product that may be evaluated in this article or claim that may be made by its manufacturer is not guaranteed or endorsed by the publisher.
Research integrity at Frontiers
Learn more about the work of our research integrity team to safeguard the quality of each article we publish.