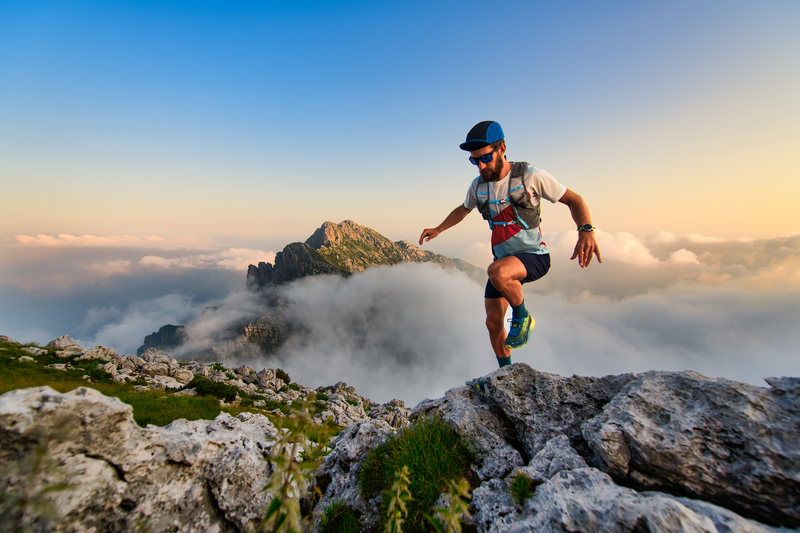
94% of researchers rate our articles as excellent or good
Learn more about the work of our research integrity team to safeguard the quality of each article we publish.
Find out more
REVIEW article
Front. Microbiol. , 31 January 2025
Sec. Microbe and Virus Interactions with Plants
Volume 16 - 2025 | https://doi.org/10.3389/fmicb.2025.1516723
This article is part of the Research Topic Diagnostic Innovations for Microbial Pathogens in Edible Plants: Cutting-Edge Technologies for Enhanced Detection View all 3 articles
The increasing prevalence of plant pathogens presents a critical challenge to global food security and agricultural sustainability. While accurate, traditional diagnostic methods are often time-consuming, resource-intensive, and unsuitable for real-time field applications. The emergence of portable diagnostic tools represents a paradigm shift in plant disease management, offering rapid, on-site detection of pathogens with high accuracy and minimal technical expertise. This review explores portable diagnostic technologies’ development, deployment, and future potential, including handheld analyzers, smartphone-integrated systems, microfluidics, and lab-on-a-chip platforms. We examine the core technologies underlying these devices, such as biosensors, nucleic acid amplification techniques, and immunoassays, highlighting their applicability to detect bacterial, viral, and fungal pathogens in diverse agricultural settings. Furthermore, the integration of these devices with digital technologies, including the Internet of Things (IoT), artificial intelligence (AI), and machine learning (ML), is transforming disease surveillance and management. While portable diagnostics have clear advantages in speed, cost-effectiveness, and user accessibility, challenges related to sensitivity, durability, and regulatory standards remain. Innovations in nanotechnology, multiplex detection platforms, and personalized agriculture promise to further enhance the efficacy of portable diagnostics. By providing a comprehensive overview of current technologies and exploring future directions, this review underscores the critical role of portable diagnostics in advancing precision agriculture and mitigating the impact of plant pathogens on global food production.
Rapid and accurate diagnosis of plant pathogens is a cornerstone in ensuring global food security, safeguarding ecosystem integrity, and sustaining agricultural productivity. As plant diseases increasingly challenge crop yields and quality, the timely detection and identification of bacterial, viral, fungal, and other phytopathogens have become paramount in guiding efficient disease management and limiting the economic losses associated with epidemics (Savary and Willocquet, 2020; Ali et al., 2021). Traditional laboratory-based diagnostic methodologies—such as plating, microscopy, enzyme-linked immunosorbent assays (ELISAs), and polymerase chain reaction (PCR)—have undoubtedly advanced our capacity to detect pathogens at remarkable levels of accuracy and sensitivity (Boonham et al., 2014). However, these approaches often require specialized equipment, stable infrastructure, and trained personnel, creating practical bottlenecks in real-world, resource-limited scenarios.
Portable diagnostic technologies—ranging from handheld biosensors and smartphone-based platforms to lab-on-a-chip devices—are promising tools that bring the diagnostic power of advanced molecular assays directly into the field (Srinivasan and Tung, 2015). Such innovations align well with the broader paradigm shift toward precision agriculture, enabling evidence-based decision-making, early warning systems, and improved surveillance networks that integrate seamlessly into the Internet of Things (IoT) and cloud-based architectures (Srinivasan and Tung, 2015).
Despite this momentum, notable gaps remain. While several reviews discuss molecular methods and high-throughput genomics-based diagnostics (Studholme et al., 2011; Aslam et al., 2017; Hariharan and Prasannath, 2021; Ijaz et al., 2022; Chakraborty and Chakraborty, 2021), a comprehensive synthesis focusing explicitly on the evolving landscape of portable diagnostic platforms and their integration into digital agronomy frameworks has yet to be presented. Existing literature often addresses individual technologies—such as loop-mediated isothermal amplification (LAMP) devices or smartphone-based lateral flow assays—in isolation. However, a holistic review that connects these diverse technologies, elucidates their underlying principles, and critically examines their field applicability, data integration strategies, and regulatory frameworks is lacking. Moreover, as portable diagnostics move from proof-of-concept prototypes toward scalable, commercialized products, there is a pressing need to map out the challenges in validation, standardization, and quality assurance that must be surmounted for widespread adoption (Ahmad-Nejad et al., 2021). A recent review article by Nguyen et al. (2024) focuses on biosensors as one specific technology for plant disease diagnosis and highlights advances in biosensor technologies, focusing on technical and functional innovations in their design and application. Another review article provides a comprehensive overview of plant pathogen detection techniques, encompassing cultivation, PCR, sequencing-based methods, and immunoassays. It includes portable biosensors as a subsection, highlighting recent advancements and their application in point-of-care diagnostics (Venbrux et al., 2023). The above two articles provide a holistic, multidisciplinary perspective on portable plant pathogen diagnostics, while broader scope, deeper integration of digital technologies, and consideration of real-world challenges and future innovations set the present review apart as a more comprehensive resource.
This review addresses critical knowledge gaps in plant pathogen diagnostics by offering a comprehensive perspective on portable solutions. It examines the core principles and technologies behind handheld analyzers, smartphone-integrated biosensors, and microfluidic-based lab-on-a-chip systems while highlighting the transformative role of IoT, cloud-based analytics, artificial intelligence (AI), and machine learning (ML) in enhancing data interpretation, connectivity, and global disease surveillance.
The review explores applications across bacterial, viral, and fungal pathogens, emphasizing how on-site testing informs disease surveillance, management decisions, and early warning systems. It addresses performance evaluation, validation in resource-limited settings, regulatory challenges, and commercial applicability to bridge the gap between innovation and real-world implementation. Additionally, it identifies future innovations, such as scalable manufacturing, cost reduction, and stakeholder engagement, aimed at advancing accessible and effective portable diagnostics. Through this integrated approach, the review provides actionable insights to drive advancements in plant pathogen diagnostics.
By providing a comprehensive analysis of the state-of-the-art in portable phytopathogen diagnostic technologies—alongside insights into data management, standardization, and integrated decision-making—this review will empower researchers, policymakers, industry stakeholders, and practitioners. Such an integrative understanding will not only help advance this rapidly evolving field but also support evidence-driven disease management strategies, enhance global surveillance networks, and pave the way for more resilient and sustainable agricultural systems.
Portable phytopathogen detection devices integrate actuators and sensors initially developed for consumer electronics, including smartphones and smartwatches, to enable on-site, real-time diagnostics. Actuator components, such as miniaturized light-emitting diodes (LEDs), are capable of emitting wavelengths spanning the visible range (approximately 400–700 nm) and have been employed to stimulate fluorescence or other optical responses in biochemical assays, particularly in the detection of pathogen-associated molecular markers. LEDs for portable biosensing, when coupled with smartphone imaging, have been well documented, offering a promising route toward rapid, field-based diagnostics of plant pathogens (Gudkov et al., 2023). Similarly, near-field communication (NFC) modules operating at 13.56 MHz allow short-range interactions with magnetic or resistive sensing elements, thereby enabling wireless activation of biosensors and facilitating assessments of soil moisture or other environmental parameters that influence pathogen spread (Gawade, 2022).
Display screens in smartphones and smartwatches, with resolutions often exceeding 720 × 1,280 px, can emit controlled wavelength outputs—such as red (628 nm), green (536 nm), and blue (453 nm)—to serve as dynamic light sources for colorimetric analyses of plant extracts. In addition, vibration motors providing haptic feedback in the range of 130–180 Hz can be leveraged to enhance assay kinetics by mixing reagents directly in the field, while integrated speakers emitting acoustic signals are being explored to disrupt sample matrices or stimulate particular biochemical reactions (Fan et al., 2021). The integration of thermal actuators is also critical, as these elements enable precise temperature control essential for nucleic acid amplification tests (NATs), including polymerase chain reaction (PCR) assays, thus facilitating on-the-spot genomic detection of pathogens without the need for a fully equipped laboratory.
Sensor components are equally transformative. Imaging detectors, including high-resolution smartphone cameras equipped for UV–Vis spectrometry, provide powerful means to capture fluorescence, color changes, or other optical indicators of infection. These imaging strategies have proven helpful for plant disease phenotyping and early stress detection (Zubler and Yoon, 2020). Environmental light sensors enhance sensitivity by accounting for ambient conditions and improving the reliability of fluorescence or colorimetric quantifications. NFC readers serve dual roles as communication modules and near-field sensors, enabling rapid, localized measurements vital for wearable and in-field biosensors.
Initially designed for human-device interaction, capacitive touchscreen sensors are increasingly recognized for their ability to detect subtle changes in pressure, moisture, or conductivity when brought into contact with plant tissues, thereby providing indirect indications of infection or physiological stress. Wearable photodetectors integrated into smartwatches further expand the scope for continuous, mobile monitoring of plant health markers, and microphones sensitive to acoustic signals emitted during microbial metabolism or pathogen-vector interactions offer unique, non-invasive diagnostic approaches (Xu et al., 2024). Coupling these sensory capabilities with geolocation data from GPS modules enables spatial mapping of disease outbreaks and supports the implementation of data-driven management strategies (Xin et al., 2009).
The convergence of these actuators and sensors within portable platforms is revolutionizing how researchers and field professionals monitor phytopathogens. Smartphones and smartwatches have evolved into versatile biosensing tools, providing real-time imaging, thermal regulation, optical stimulation, wireless connectivity, and acoustic or tactile feedback (Buja et al., 2021). These devices facilitate on-site nucleic acid amplification and rapid pathogen detection and enable continuous environmental and plant health monitoring. These integrated technologies can guide timely interventions by tracking chlorophyll fluorescence fluctuations indicative of early disease onset, mapping pathogen prevalence across agricultural landscapes, and detecting subtle changes in leaf temperature profiles. As a result, mobile devices equipped with tailored actuators and sensors are poised to become indispensable in modern precision agriculture, improving our capacity to detect, map, and manage phytopathogens and safeguarding global crop productivity and food security.
Figure 1 shows that portable phytopathogen detection devices integrate sensor components (such as imaging detectors, light sensors, and GPS modules) and actuator components (like LEDs and thermal actuators) to enable various applications, including phytopathogen detection, agricultural monitoring, and remote sensing for enhanced crop management.
Figure 2 illustrates various advanced biosensing technologies categorized based on their unique functionalities. Immunoaffinity devices provide sensitive detection using fluorescence techniques, while imaging biosensors enable label-free, multiplexed biomarker detection. Plasmonic sensors facilitate high-throughput screening of biomolecular interactions, and lab-on-a-chip systems integrate microfluidics for rapid analyte detection. Additionally, optofluidic devices offer real-time monitoring of molecular interactions, and smartphone integration enables biosensing in resource-limited settings by leveraging portable and accessible technologies. Together, these innovations highlight the convergence of biosensing technologies’ precision, portability, and scalability.
Handheld analyzers and mobile biosensors are essential for rapid, on-site diagnostics, especially in environments where traditional laboratory infrastructure is unavailable. These portable devices integrate advanced biosensing technologies, enabling the detection of pathogens, chemicals, and other analytes directly in the field. Their compact size, ease of use, and fast response times make them highly suited for clinical diagnostics, environmental monitoring, and resource-limited settings that require immediate results.
A significant innovation in this space is using plasmonic biosensors, which utilize nanohole arrays and CMOS camera technology for sensitive, label-free viral detection. Its adaptability lies in using a plasmonic chip surface coated with specific antibodies, which can be modified to target different viral types depending on the surface coating (Cetin et al., 2021). Similarly, portable electrochemical biosensors, like those used for detecting hepatitis C, offer Bluetooth-enabled real-time monitoring. These devices use cyclic voltammetry to identify viral markers and boast a wireless setup with over three hours of battery life, making them ideal for mobile diagnostics (de Campos da Costa et al., 2019).
Integrating smartphones into biosensing technologies further enhances these tools by providing advanced data processing, storage, and sharing capabilities. For instance, a “three-in-one” biosensor detects infections and provides diagnostic information for each stage of the infection cycle, enabling continuous patient health monitoring (Dou et al., 2022).
Moreover, microfluidic technology has been incorporated into portable analyzers, allowing rapid and multiplexed detection. A paper-based microfluidic biosensor, for instance, has been developed to detect viral pathogens in samples. This electrochemical immunosensing platform can simultaneously perform enzyme-linked immunosorbent assays (ELISA) on multiple samples, offering a low-cost and user-friendly solution for point-of-care diagnostics (Zhao and Liu, 2016).
Another valuable addition to mobile diagnostic technology is interferometric biosensors, such as the Young interferometer sensor. These highly sensitive devices combine optical sensors with antibody-antigen recognition for rapid and accurate viral detection. Their portability and ease of use make them especially useful in remote areas that lack sophisticated laboratory facilities (Ymeti et al., 2007).
Beyond medical applications, handheld analyzers have also proven valuable in agricultural settings. For example, a bioelectric recognition assay has been tested to detect plant pathogens like Potato virus Y and Cucumber mosaic virus. This technology can process up to 96 samples in approximately 70 min, making it ideal for on-site pathogen diagnostics, where quick results are critical for preventing widespread crop damage (Perdikaris et al., 2011). Another example of this advancement is depicted in Figure 3, which demonstrates the design of a wearable biosensor glove with an integrated printed circuit board for real-time pathogen detection in various field conditions.
Smartphone and smartwatch integrated diagnostic tools are transforming phytopathogen detection by combining molecular assays, AI-powered image analysis, and sensor technologies, providing rapid, accurate, and real-time pathogen identification in agricultural settings. Tools such as Smart LAMP use Loop-Mediated Isothermal Amplification (LAMP) to detect pathogens like Ralstonia solanacearum in crops like tomatoes and potatoes. This setup eliminates the need for complex thermocyclers operating at a single temperature. Using the smartphone camera to capture color changes in the LAMP reaction, the tool provides visual indicators of infection in real-time, making it highly accessible for remote or resource-limited environments (Ivanov et al., 2021).
The Biomeme two3 device integrates qPCR with smartphone connectivity, enabling DNA and RNA analysis for pathogens such as Fusarium oxysporum in bananas and tomatoes. This mobile platform uses fluorescence signals to quantify pathogen load in approximately 45 min, displaying results via a smartphone app. Smartwatches can extend the utility of this platform by receiving and displaying diagnostic alerts, allowing for immediate field-level responses even when the smartphone is not in use. This real-time data can be shared remotely, making it ideal for rapid, informed decision-making (Buja et al., 2021). Similarly, nano-sensor technology uses VOC detection to analyze biomarkers emitted by infected plants, allowing early disease detection even before visible symptoms appear. This smartphone-based system has proven effective in crops like wheat and potatoes, helping farmers proactively manage crop health (Li et al., 2019).
For image-based detection, ViT-SmartAgri utilizes a Vision Transformer model to classify tomato leaf images, distinguishing between healthy and diseased plants with high accuracy. By capturing and processing leaf images through the smartphone camera, ViT-SmartAgri provides instant diagnostic feedback, supporting early intervention against diseases like late blight and mosaic virus. Smartwatches can act as companion devices, offering quick access to diagnostic summaries and alerts, further improving efficiency in field operations (Alzahrani and Alsaade, 2023).
The Agdia ImmunoStrip® combines lateral flow assays with smartphone technology to detect Candidatus Liberibacter asiaticus, the pathogen behind the citrus greening disease. After the DNA amplification process, the test line’s visibility on the strip provides a straightforward readout, which can be captured and analyzed by a smartphone app. This tool has been instrumental in Florida citrus groves, allowing quick, in-field diagnostic results to mitigate disease spread (Russell et al., 2015).
Portable microfluidic and lab-on-a-chip (LOC) systems fundamentally transform phytopathogen detection in agriculture. These compact devices integrate various functions, including sample preparation, amplification, and detection, onto a single chip, enabling quick responses to pathogen outbreaks and supporting proactive crop disease management. Examples such as the CRISPR-Cas13a-based centrifugal microfluidic system exemplifies these advancements, automating processes like nucleic acid extraction and detection within a single device, achieving sensitivity levels as low as one copy per reaction in plasmid samples, which is particularly useful for field applications requiring high sensitivity (Zhang et al., 2024).
Similarly, hand-powered microfluidic devices enable affordable sample processing by utilizing simple mechanisms, such as syringes, to facilitate digital PCR applications without external power sources, making them ideal for remote agricultural settings where cost-effective, high-throughput diagnostics are essential (Xie et al., 2021). Other LOC systems use LAMP-based technologies with electrochemical sensors capable of operating at lower temperatures (39°C), delivering real-time results within 20 min—an advantage for rapid, in-field diagnostics of viral phytopathogens (Hsieh et al., 2015).
Figure 4 illustrates a typical microfluidic LOC system for pathogen detection. The chip consists of several key components, including a collection point where the sample is introduced, a test strip for initial sample processing, and a mixing chamber that allows the sample to interact with reagents. The chip is also equipped with multiple reservoirs, which store necessary reagents and direct them through the system for diagnostic processes. This configuration enables efficient, automated pathogen detection by minimizing manual handling, integrating various analytical methods, and facilitating high-throughput testing suitable for on-field diagnostics.
More advanced microfluidic devices, such as the mChip, expand on these capabilities with fluorescence-based detection for pathogens like Xylella fastidiosa, impacting crops such as olives. When integrated with smartphone-based fluorescence readers, this platform enables early pathogen detection with high accuracy, helping farmers in regions like Southern Italy promptly identify infections (Milson and Kevin, 2023). Similarly, multiplex platforms like PlexBio™ OptoSelect™ allow simultaneous detection of multiple pathogens. Using color-coded microbeads, PlexBio has successfully identified bacterial diseases such as spot, blight, and mosaic virus in Californian tomato farms within an hour, streamlining large-scale agricultural testing (Foudeh et al., 2012).
For simpler applications, paper-based microfluidic devices (e.g., μPADs) use capillary action for the colorimetric detection of pathogens without requiring external power. Such devices are precious in resource-limited environments across Southeast Asia, where visible color changes in response to pathogen presence enable users to interpret them more straightforwardly (Chen et al., 2021). Furthermore, ChemBio™ offers a chemiluminescent immunoassay-based solution that has proven effective in detecting pathogens associated with citrus greening disease in Chinese citrus groves, thereby allowing for tailored disease management practices (Hu et al., 2017).
Lastly, platforms like LabDisk use centrifugal forces for automated sample handling, directing fluids through preloaded reagents to reaction chambers for pathogen detection. This technology has been employed in vineyards to monitor Botrytis cinerea, responsible for grey mold, by reducing manual handling and facilitating high-throughput diagnostics suitable for large-scale field applications (Neethirajan et al., 2011).
Portable point-of-care (POC) devices for phytopathogen detection are essential in agricultural and environmental management, enabling on-site diagnostics and immediate decision-making to curb plant diseases. These devices’ portability and user-friendliness are designed for field deployment, allowing use by individuals without specialized training. Lightweight and compact, POC devices are optimized for rapid, on-site testing, crucial in areas lacking lab access. Their ability to deliver quick results in a few minutes to hours compared to lab tests that may take days—supports timely intervention to prevent further pathogen spread, which is especially critical in large-scale agricultural settings where delays can lead to economic losses (Buja et al., 2021; Safavieh et al., 2014). Table 1 lists the core technologies used in portable diagnostic devices.
The latest developments integrate nucleic acid amplification and optical detection, allowing these devices to differentiate pathogenic from non-pathogenic organisms—critical for reliable diagnostics (Hsieh et al., 2015). Durability is equally important, as these devices often endure harsh conditions. Features like ruggedized casings, moisture resistance, and self-contained power sources ensure reliability and minimize maintenance in varied agricultural environments (Kou et al., 2020).
Portable biosensor devices have revolutionized phytopathogen detection, enabling rapid, accurate, field-ready diagnostics supporting effective agricultural disease management. Key innovations include smartphone-based systems, LOC technology, bioluminescent cell-based sensors, and plasmonic biosensors, all offering practical alternatives to traditional laboratory methods.
Smartphone-based biosensors utilize smartphones’ cameras and processing power, often relying on colorimetric assays that change color upon pathogen detection. Custom apps then analyze these changes, providing rapid results ideal for resource-limited environments (Huang et al., 2018). LOC devices also enhance portability by miniaturizing lab processes onto a chip, allowing quick diagnostics with minimal reagents. For instance, LOC microcantilever-based biosensors can detect Salmonella at low concentrations of 103 CFU−1 mL, demonstrating their efficacy in field applications (Ricciardi et al., 2010).
Bioluminescent cell-based biosensors employ genetically engineered cells that emit light in response to specific pathogens, enabling high-intensity nanomolar detection levels suitable for direct field diagnostics (Roda et al., 2011). Enzyme-based biosensors with metal-organic frameworks (MOFs) are another portable option; these smartphone-assisted sensors provide real-time colorimetric detection, making them a cost-effective choice for agricultural pathogen monitoring (Kou et al., 2020). Additionally, nanoparticle-based biosensors enable sensitive pathogen detection by leveraging nanoparticles’ high surface area and functional versatility. Gold nanoparticles (AuNPs), known for their optical properties, produce visible color changes that assist in detecting agrichemicals, such as pesticides, within seconds, which is ideal for on-site testing in resource-limited settings (Baek et al., 2017). Magnetic nanoparticles further support pathogen detection; biosensors using Fe3O4 nanoparticles with acetylcholinesterase are highly sensitive and reusable, well-suited for repeated agricultural tests (Chauhan and Pundir, 2011).
Plasmonic biosensors amplify optical signals using metal nanoparticles, facilitating phytopathogen detection. Handheld devices with plasmonic microarrays provide quantitative data through computational imaging, offering reliable results with minimal sample prep (Cetin et al., 2014). Plant-wearable biosensors employing gold nanoparticles monitor pesticides in real-time, transmitting data to a smartphone for precision application and supporting sustainable agricultural practices (Zhao et al., 2020). Moreover, mobile apps like LAMP-CAM enhance diagnostics by utilizing colorimetric detection to identify pathogens such as Phytophthora infestans, a primary cause of late blight in crops. LAMP-CAM interprets color changes detected by a smartphone camera and translates them into diagnostic results, improving accuracy in remote areas (Raguraman et al., 2021).
Electrochemical biosensors are also noteworthy. For example, apps like PSoC Programmer and PSoC Creator connect to electrochemical sensors via USB OTG or Bluetooth, detecting pathogens like Xanthomonas oryzae in rice and enabling real-time data visualization (Currie, 2021). Meanwhile, ViT-SmartAgri uses deep learning for plant disease identification, employing a Vision Transformer (ViT) model to accurately differentiate between healthy and diseased tomato plants, providing a user-friendly disease management solution (Barman et al., 2024). Cloud-connected portable LAMP systems enhance biosensor capabilities by enabling rapid, on-site detection of Candidatus Liberibacter asiaticus, the citrus greening pathogen. These devices integrate fluorescence-based detection with smartphone apps for real-time data sharing, aiding swift management decisions (Fujikawa & Iwanami, 2012). Data is transmitted to cloud services, supporting centralized analysis and rapid outbreak response. Table 2 describes the fabrication methods used to develop sensors in phytopathogen diagnostics.
Figure 5 presents two key biosensor mechanisms. In part A, the top schematic illustrates the interaction in an immunosensor where antibodies bind specifically to target proteins (analyte). This interaction is detected by a transducer, which processes the signal for data analysis. In part B, the bottom schematic shows a nucleic acid biosensor where DNA/RNA sequences interact with complementary DNA probes on the biosensor surface. The interaction generates a signal processed through the transducer, similar to immunosensors, to detect the presence of specific nucleic acids.
Figure 5. Schematic representation of biosensor mechanisms. (A) Immunosensor-based mechanism. (B) Nucleic acid-based biosensor mechanism.
Figure 6 illustrates the mechanism of aptamer-antigen interaction in biosensor applications. The process begins with an aptamer sequence, which forms a specific structure through folding. Once the aptamer assumes its active conformation, it selectively binds to the target antigen, creating a stable aptamer-antigen complex. This interaction is crucial in biosensor applications, as it triggers a detectable signal that can be used to identify and quantify specific pathogens or analytes in various agricultural and environmental samples.
Figure 7 illustrates the operational mechanism of enzyme-based electrochemical biosensors for pathogen detection. In Pathway A, the enzyme catalyzes the conversion of an analyte into a product with the help of a mediator, which facilitates electron transfer to the electrode, generating a detectable signal. In Pathway B, the direct interaction between the enzyme and the electrode without a mediator results in the same outcome, demonstrating two common approaches used in biosensor technology.
Figure 7. Mechanism of enzyme-based biosensors for pathogen detection. (A) Mediator-assisted enzyme-based biosensor. (B) Direct enzyme-based biosensor.
Optical biosensors detect changes in optical properties such as absorbance, fluorescence, or refractive index upon interaction with the target pathogen. Surface plasmon resonance (SPR) biosensors have been employed for label-free detection of plant viruses like Tobacco Mosaic Virus (TMV), offering real-time monitoring and high sensitivity (Hariharan and Prasannath, 2021). Surface SPR biosensors are increasingly used for the label-free and highly sensitive detection of various plant viruses beyond TMV. These sensors provide real-time monitoring and can be specifically tailored to detect various plant viral pathogens. An SPR biosensor has been developed to detect MCMV by utilizing a gold surface coated with 11-mercaptoundecanoic acid, which is then functionalized with anti-MCMV antibodies. This setup achieved a detection limit of around 1 part per billion (ppb), showcasing its high sensitivity compared to conventional methods like ELISA (Zeng et al., 2013). In another application, SPR biosensors were used to monitor the interaction of Potato Virus Y with monoclonal antibodies, allowing researchers to study various serotypes and their interactions with antibodies in real-time. This biosensor setup was beneficial for optimizing serologic diagnostic tools and enhancing our understanding of viral serotype variability (Gutiérrez-Aguirre et al., 2014).
Fluorescence-based sensors utilize fluorescent markers that emit signals upon binding to the target pathogen. Portable fluorescence detectors have been developed to detect pathogens like Erwinia amylovora, the causative agent of fire blight in apples and pears. Figure 8 depicts an optical sensor where an analyte interacts with a sensing element, altering light from a source, which is then detected by a photodetector to measure the analyte.
Electrochemical biosensors, which detect changes in electrical signals caused by biochemical reactions, have emerged as powerful tools for detecting pathogens in agriculture. By incorporating nanomaterials like gold nanoparticles, carbon nanotubes, and quantum dots, these sensors have significantly advanced in-field diagnostics, offering rapid and sensitive detection of phytopathogens.
Gold nanoparticles, widely known for their signal-amplifying properties, play a key role in lateral flow assays. These nanoparticles bind to pathogen-specific antibodies, enabling quick, on-site diagnostics without lab-based testing. For instance, they have been effectively used to detect Phytophthora infestans, a major pathogen responsible for potato blight, supporting disease management directly in the field (Bobrinetskiy et al., 2021; Kashyap et al., 2016b). Similarly, carbon nanotubes, prized for their high conductivity, enhance signal transduction in biosensors, making them particularly useful in detecting viral pathogens like the Tomato Yellow Leaf Curl Virus. These nanotubes detect even minute concentrations of viral DNA, crucial for early intervention and preventing widespread outbreaks (Rajwade et al., 2020; Attaluri and Dharavath, 2023).
Another innovative approach involves the use of quantum dots in smartphone-integrated biosensors. These quantum dots fluoresce when they interact with target genetic material, and the fluorescence is captured and analyzed by smartphone cameras, offering instant and quantitative pathogen assessments. This technology, used to detect fungal pathogens like Fusarium oxysporum, enables rapid decision-making and facilitates data sharing across networks, improving coordinated responses to agricultural threats (Rajwade et al., 2020; Attaluri and Dharavath, 2023).
Enzyme-linked biosensors are another vital tool for field diagnostics. These sensors utilize enzyme-catalyzed reactions to produce measurable signals, such as colorimetric or electrochemical outputs, allowing on-site detection without specialized lab equipment. Miniaturized versions of ELISA are widely used to detect viruses such as Plum Pox Virus in stone fruits and Maize Chlorotic Mottle Virus in maize. By linking pathogen-specific antibodies to enzymes that produce a visible color change upon pathogen detection, these devices offer real-time monitoring capabilities with sensitivity comparable to PCR, making them essential for prompt agricultural interventions (Ali et al., 2021; Ray et al., 1856).
Beyond viral detection, enzyme-linked biosensors have also proven effective in identifying bacterial and fungal pathogens, such as Fusarium oxysporum, which causes wilt disease in tomatoes. These sensors utilize peroxidase-based reactions to generate optical signals upon detecting fungal markers, making them ideal for on-site testing in remote agricultural areas where lab access is limited. Additionally, these biosensors are also capable of monitoring organophosphate pesticide residues. Acetylcholinesterase-based sensors detect pesticide residues by measuring enzyme inhibition, providing a dual benefit of pathogen and pest monitoring, which is crucial for sustainable agricultural practices (Ali et al., 2021; Kaur and Singh, 2020; Karadurmus et al., 2021).
Figure 9 illustrates an electrochemical biosensor designed for phytopathogen detection. The analyte, such as microbial pathogens or their markers, interacts with a specific receptor (antibody, DNA, lipid, or peptide) immobilized on a nanoparticle matrix. This interaction generates a signal transduced into an electrochemical output, enabling pathogen identification and quantification.
Isothermal nucleic acid amplification techniques, like LAMP and recombinase polymerase amplification (RPA), offer the advantage of operating at constant temperatures, eliminating the need for complex thermal cycling equipment, characteristic of PCR-based methods. These techniques are increasingly integrated into portable, field-deployable devices for rapid diagnostics. For instance, LAMP assays have been successfully used in portable formats to detect plant pathogens like Phytophthora infestans, the causative agent of late blight in potatoes, with amplification times usually under 30 min and colorimetric readouts for ease of interpretation (Ristaino et al., 2020). Similarly, RPA technology, operating at low temperatures (37–42°C), has been employed in handheld devices for the detection of viruses, such as the Tomato Yellow Leaf Curl Virus (TYLCV), making it highly suitable for field diagnostics (Wang and Yang, 2019). Figure 10 illustrates a detailed workflow for portable isothermal nucleic acid amplification techniques, including sample collection, preparation (involving cell lysis and nucleic acid purification), and the essential primer binding step for amplification.
PCR is a critical molecular biology technique known for its high specificity and sensitivity in nucleic acid amplification and pathogen detection. Traditionally confined to centralized labs due to the complexity of thermal cycling and real-time detection, recent advancements in microfluidics, miniaturization, and power efficiency have created portable PCR devices. Portable PCR devices replicate the core functions of conventional machines. Still, they are optimized for field use through features such as battery-operated thermal cyclers and microfluidic chambers, which enable rapid temperature cycling and efficient reagent use. Integrated fluorescence detection further allows real-time monitoring of DNA amplification, making quantitative PCR (qPCR) feasible in non-laboratory environments. These innovations have made portable PCR invaluable for detecting pathogens, including plant and bacterial contaminants, especially in on-site diagnostics and environmental assessments (Koo et al., 2013).
One notable example of these advancements is the handheld qPCR device, which offers high specificity and sensitivity for detecting plant pathogens on-site. This compact and user-friendly tool is particularly beneficial in agriculture, where early detection of diseases can prevent significant crop losses (DeShields et al., 2018). Additionally, devices such as the BioFire FilmArray system integrate multiple processes, from nucleic acid extraction to real-time PCR, into a single platform, delivering results in under an hour, demonstrating the potential of portable PCR devices in decentralized pathogen detection (Pham et al., 2024).
Field-based PCR and other molecular devices have revolutionized pathogen detection by providing real-time diagnostics in agricultural settings (Figure 11). These tools allow rapid identification of diseases, enabling timely intervention. Devices like the miniPCR, a compact thermocycler, have been effectively used to detect Phytophthora infestans, the causative agent of potato late blight, providing lab-equivalent results in minutes. The miniPCR connects to smartphones or tablets for real-time result monitoring, crucial for field-based diagnostics in remote areas (Gonzalez-Gonzalez et al., 2020; Ssengo et al., 2020). Similarly, the Biomeme two3 platform integrates qPCR with smartphone technology, simultaneously supporting up to three reactions and allowing real-time pathogen detection, such as Fusarium oxysporum, within 45 min. The system’s smartphone interface facilitates remote data sharing and decision-making, enhancing its utility in the field (Buja et al., 2021; Marx, 2015).
Figure 11. Portable PCR workflow with the integration of microfluidics for rapid DNA amplification and detection.
Immunoassays leverage the specificity of antigen-antibody interactions for pathogen detection. Lateral Flow Immunoassays (LFIA) are commonly used for their simplicity and rapid results. Portable devices utilizing LFIA have been developed for on-site detection of various plant pathogens, including Xanthomonas species in citrus plants (Srinivasan and Tung, 2015). These assays provide results within minutes and are user-friendly, requiring minimal training.
Figure 12 illustrates the portable ELISA process, operating on the same principle as LFIA, utilizing antigen-antibody interactions for pathogen detection. In ELISA, antibodies are first immobilized on the plate to capture target proteins, similar to how LFIA detects pathogens like Xanthomonas species in citrus plants. This cost-effective and widely used technique ensures selective binding, essential for accurate detection. The target protein binds to the immobilized antibodies, reflecting the precise interaction seen in LFIA. A secondary enzyme-conjugated antibody then binds to a different epitope, forming a sandwich structure that mirrors the detection phase of LFIA. Finally, a substrate is added, reacting with the enzyme to produce a visible color change, confirming the presence of the target. This rapid detection is akin to LFIA’s quick results in field applications. The figure effectively shows how ELISA and LFIA, though different in their settings, share the same core immunoassay principles for pathogen detection.
Portal lateral flow assays (LFAs) and immunoassay devices are vital for rapid and cost-effective phytopathogen detection. With the increasing importance of timely diagnostics in agriculture, these devices have shifted diagnostics from the laboratory to the field, allowing farmers and agronomists to implement real-time solutions. Their simplicity, portability, and quick result delivery make LFIAs ideal for field-based diagnostics, while recent advancements in sensitivity have expanded their utility in agriculture (Figure 13). Using nanoparticles like gold and quantum dots enhances the assay signal, allowing the detection of even minimal pathogen concentrations, such as in the detection of potato virus X through nanoparticle enlargement, which lowered detection limits and enhanced precision (Banerjee and Jaiswal, 2018). Another innovation involves graphene oxide, which improves sensitivity through photoluminescence, enabling the detection of pathogens like Escherichia coli, suggesting potential for a range of phytopathogen applications (Avila-Huerta et al., 2020).
Figure 13. Example of a portable lateral flow immunoassay for pathogen detection, showing the test and control lines in positive and negative results.
A significant advancement is the incorporation of CRISPR technology into lateral flow diagnostics. The CRISPR-based Bio-SCAN LFA allows for rapid detection of plant pathogens and transgenic elements within an hour. This field-deployable system has been tested on crops like wheat and rice, proving effective for disease management and crop breeding (Ali et al., 2022). Additionally, nucleic acid-based LFAs with RPA technology present a promising approach for viral pathogen detection. This method has demonstrated PCR-comparable sensitivity in detecting potato virus X and is suitable for rapid, on-site applications that require swift results (Ivanov et al., 2020).
Innovations aimed at enhancing assay sensitivity continue to drive progress. For instance, post-assay nanoparticle growth techniques have achieved much lower detection limits, allowing precise diagnostics for even small quantities of target analytes. Techniques such as multicolor signal integration on a single assay line enable the simultaneous detection of multiple pathogens, increasing test efficiency (Di Nardo et al., 2019). In addition, time-resolved luminescent nanoparticles extend these devices’ dynamic range and sensitivity, making them suitable for more complex field conditions (Song and Knotts, 2008).
In the context of phytopathogen diagnostics, aptasensors can be tailored to identify specific microbial or fungal markers associated with plant pathogens. By leveraging MXene’s excellent electrical conductivity, high surface area, and tunable properties, these sensors can achieve high sensitivity and specificity. Integrating aptamers into MXene-based platforms further enables rapid, cost-effective, and portable detection methods that are particularly advantageous for on-site plant disease diagnostics (Parihar et al., 2022).
MXene-based aptasensors also show significant promise for detecting phytopathogenic toxins, such as aflatoxins, in agricultural and food matrices. Their high electrical conductivity, biocompatibility, and customizable surface features facilitate quick, sensitive, and affordable toxin detection. This approach surmounts the limitations of conventional methods—like HPLC or ELISA—by offering faster and simpler analytical workflows. When combined with IoT and advanced data analytics, these portable systems enable real-time monitoring to ensure crop health and food safety (Parihar et al., 2023).
Building upon these advancements, the novel approach of electrochemical aptasensor fabrication for phytopathogen biomarker detection focuses on synthesizing a reduced graphene oxide-yttrium nanocomposite and employing it in electrochemical aptasensor construction. By integrating phytopathogen-specific aptamers, this technique delivers a label-free, highly sensitive, and cost-effective diagnostic platform. Critically, this strategy supports the early detection of plant diseases, making it an instrumental tool in strengthening agricultural diagnostics and improving overall crop health management (Parihar and Khan, 2024).
Advancements in digital technologies, particularly the Internet of Things (IoT) and artificial intelligence (AI), have revolutionized phytopathogen detection, offering real-time, field-based solutions that enhance precision agriculture. Integrating IoT with portable diagnostic methods enables seamless data collection and transmission from diagnostic devices to centralized systems, ensuring faster decision-making and improved disease management strategies.
The integration of IoT in agriculture has fundamentally improved the ability to monitor and manage agricultural practices with real-time, actionable data. This technology enables portable devices like PCR, LAMP, and ELISA to detect phytopathogens directly in the field, transmitting data to cloud systems for analysis and alerts. By facilitating immediate intervention through mobile alerts, IoT-connected devices help farmers take proactive steps to contain diseases such as Fusarium wilt, as demonstrated in successful applications across global agricultural settings (Dholu and Ghodinde, 2018; Kumar et al., 2023). Figure 14 describes the application of IoT in plant pathogen detection.
IoT-enabled devices have optimized pathogen detection in vineyards, reducing pesticide use while maintaining crop quality (Trilles et al., 2020). In Southeast Asia, IoT-based LAMP systems detected bacterial blight in rice, providing timely data in regions with limited lab access and enhancing regional pathogen tracking (Uddin et al., 2017). Similarly, U.S. farmers managing soybean rust have benefited from IoT kits that enabled targeted fungicide applications (Ayaz et al., 2019).
Beyond pathogen detection, IoT’s role in agriculture includes granular data collection supporting predictive models for disease prevention. However, challenges such as costs, connectivity issues in rural areas, and technical expertise remain significant obstacles to its broader adoption (Khuwaja et al., 2023; Siskandar et al., 2020). Nevertheless, IoT is pivotal in shaping resilient and data-driven farming practices, optimizing resource use, and ensuring sustainable agricultural operations.
Applying AI and Machine Learning (ML) in agriculture, particularly in portable diagnostic tools, has transformed how diseases are managed in real-time. AI-driven platforms, including PlantVillage Nuru and, ViT-SmartAgri harness the potential of frameworks like TensorFlow and PyTorch to provide farmers with rapid and precise disease diagnostics via smartphones. These tools empower smallholder farmers worldwide, reducing dependency on costly lab tests and enhancing disease management.
For example, PlantVillage Nuru identifies plant diseases with over 90% accuracy by analyzing smartphone images of leaves, enabling farmers in East Africa to mitigate crop losses effectively (Mrisho et al., 2020). ViT-SmartAgri leverages advanced vision transformer models to detect subtle symptoms in crops like tomatoes, further supporting precision agriculture with highly accurate disease identification (Boukabouya et al., 2022).
In addition to image-based diagnostics, AI algorithms such as Support Vector Machines (SVMs) and k-NN classifiers are being used in multiplex detection systems to classify pathogens like Ralstonia solanacearum, improving the speed and accuracy of field-based diagnostics (Ryo et al., 2009). Predictive modeling through platforms integrating IoT and AI further supports preemptive disease management. These models, such as Random Forest and RNNs, predict outbreaks of diseases like potato late blight, enabling farmers to implement preventive strategies before infections spread (Meno et al., 2023).
With millions of global users, apps like Plantix, which diagnose plant diseases and nutrient deficiencies, have significantly contributed to sustainable farming practices (Zhao et al., 2024).
Moreover, portable spectrometers combined with AI algorithms, such as Decision Trees and Support Vector Machines, analyze plant nutrient deficiencies by interpreting leaf reflectance spectra, providing farmers with immediate insights to optimize plant health (Li D. et al., 2020). AI models, including LSTM networks, are also used for forecasting pest outbreaks based on climatic data, allowing for targeted interventions (Chen et al., 2022). Drone-based systems equipped with AI offer large-scale crop monitoring, enabling efficient management of disease hotspots in expansive agricultural fields (Bawa et al., 2024).
These portable diagnostic devices generate diverse, real-time data streams—from diagnostic results to geospatial coordinates and temporal disease trends—and rely on robust pipelines for harmonization, analysis, and dissemination. Wireless connectivity through Wi-Fi, LPWANs, and 4G/5G networks ensures seamless data transfer to cloud servers and decision-support tools, enabling systems to integrate with nanopore sequencing for real-time pathogen analysis, machine learning-driven outbreak predictions and targeted fungicide recommendations (Fountas et al., 2020). Interoperable data formats and standardized protocols promoted by organizations like ISO facilitate large-scale disease surveillance, informed by spatial data on virulence races of pathogens such as wheat rust (Gilligan, 2024). Embedded algorithms enhance data quality control, while smartphone-integrated microfluidic chips ensure accurate detection by adjusting for environmental variables (Chen et al., 2017). Connectivity also supports collaborative diagnostics, allowing remote consultation with experts and global data-sharing for emerging pathogens (Lau and Botella, 2016), and CRISPR-based devices coupled with geospatial data can predict disease spread to optimize management strategies (Morcia et al., 2023). Data security measures, including encryption and authentication, safeguard agronomic information, while AI models and multi-modal analyses combining external data sources, like satellite imagery and soil profiles, support holistic disease management (Mahlein, 2016).
Flexible connectivity frameworks integrate evolving diagnostic technologies—CRISPR-based tools, synthetic biology biosensors—into existing IoT and cloud platforms, benefiting large-scale and smallholder agriculture. For instance, China’s IoT-based system for crop disease prevention uses ozone sterilization and light-trap technology, transmitting environmental and pathogen data to a central system for real-time alerts and reducing chemical inputs (Wang et al., 2024). Cloud-based analytics platforms like Arable and Semios combine sensor data on plant health, pests, and weather conditions, enabling immediate, data-driven insights and collaboration among farmers, extension services, and researchers. Large-scale epidemiological analysis and predictive modeling help manage infections like Xylella fastidiosa in olive orchards (Hornero et al., 2020) and predict Phytophthora infestans outbreaks in potatoes using machine learning models (Duarte-Carvajalino et al., 2018). This convergence of wireless sensor networks, cloud analytics, and AI-driven modeling fundamentally enhances real-time decision-making, resilience, and sustainable disease control, empowering modern agriculture to confront climate change and evolving pathogen challenges. Figure 15 illustrates the resolution of various plant stress detection techniques.
Figure 15. Schematic representation of multiscale non-invasive spectral and optical techniques for detecting biotic stresses in plant parts, individual plants, and field-level systems.
Smartphone applications for phytopathogen detection have revolutionized agricultural diagnostics, leveraging AI and machine learning to provide real-time disease management solutions. Apps like Plantix are widely used in South Asia and Africa, enabling farmers to diagnose diseases like powdery mildew and rust through image analysis. Plantix’s extensive database covers over 30 crops and 400 diseases, and it also offers a community feature for farmers to share insights, making it a valuable resource in regions with limited access to traditional agricultural support (Samal et al., 2023).
AgroAI targets staple crops such as rice and maize, using CNNs to identify bacterial blight and maize rust while also forecasting disease outbreaks based on environmental data like humidity and temperature (Terentev and Dolzhenko, 2023). AgroAI’s predictive capabilities allow farmers to implement preventive measures, reducing crop losses and improving crop health. In East Africa, iCassava addresses cassava diseases like the Cassava Brown Streak Virus (Enkvetchakul and Surinta, 2022). Developed with TensorFlow’s mobile framework, iCassava, a computer vision dataset, operates offline, essential in remote areas of Uganda and Tanzania. Field tests have demonstrated high accuracy, bolstering efforts to protect this staple crop from devastating diseases (Mwebaze et al., 2019).
DetectPlant uses the EfficientNetV2B2 model to detect diseases in tomatoes and grapes, achieving high accuracy through transfer learning, which adapts pre-trained models to specific crops (Debnath et al., 2023). Meanwhile, CropDoctor is widely adopted in India to identify diseases and nutrient deficiencies across crops like wheat and soybeans. Its AI-powered analysis provides farmers with actionable recommendations, and studies have shown that its guidance can increase crop yields by up to 15% (Siddiqua et al., 1869). LeafDoctor serves researchers and extension officers by quantifying disease severity in crops like wheat and corn. By estimating the percentage of leaf area affected, LeafDoctor helps monitor the effectiveness of treatment strategies, facilitating more precise disease management decisions over time (Maginga et al., 2024). Table 3 summarizes various smartphone applications used in phytopathogen detection.
Portable diagnostic devices have revolutionized disease surveillance by enabling real-time monitoring directly within agricultural settings. These devices integrated into routine farming practices allow for continuous crop monitoring, crucial for early detection and rapid intervention against fast-spreading pathogens.
The data collected by these portable diagnostics is key to data-driven decision-making, enabling precise, targeted disease management. Field-based diagnostic data help farmers and agricultural managers make informed decisions about interventions, such as targeted fungicide application, removal of infected plants, or effective quarantine measures. For example, information on Xylella fastidiosa infections in olive groves informs selective pruning and vector control strategies (Amoia et al., 2023). Additionally, geotagged diagnostic data can be integrated into regional systems, allowing agricultural networks to track and respond to disease trends more effectively. By mapping infection patterns, stakeholders can anticipate future outbreaks and implement preemptive measures.
The advancements in handheld technologies—from LAMP-based assays and lateral flow immunoassays (LFIAs) to microfluidic LOC platforms and smartphone-integrated systems—have transformed plant disease diagnostics. In contrast to traditional laboratory-based methods, these compact devices offer rapid, accurate, and cost-effective on-site identification of pathogens, enabling farmers and agronomists to implement immediate control measures. The text underscores how these novel approaches help mitigate substantial crop losses, strengthen food security, and streamline disease management practices. The discussion underlines the importance of portable diagnostics as a cornerstone of sustainable plant health strategies by connecting recent technological breakthroughs to their practical field applications.
Accurate and timely diagnosis of plant pathogens is critical to minimizing agricultural losses and safeguarding global food security. While reliable, traditional diagnostic methods often require extensive laboratory infrastructure, technical expertise, and significant time, limiting their utility in the field. Advances in portable, rapid, and user-friendly diagnostic tools are revolutionizing the management of major pathogen groups, enabling farmers and agronomists to take swift action at the point of need. These novel approaches leverage cutting-edge technologies such as microfluidic LOC platforms, LFIAs, and smartphone-integrated detection systems to provide accessible and precise solutions for pathogen detection.
Table 4 provides a comprehensive list of significant plant pathogens, categorized by their type and primary target host plants. This summary highlights the diverse pathogens affecting key crops and underscores the need for targeted diagnostic and management strategies.
Bacterial phytopathogens represent a persistent threat to global agriculture, resulting in severe yield losses and economic setbacks. Historically, detection and identification hinged on labor-intensive, laboratory-based methods that were time-consuming and required technical expertise. Recent innovations, however, have ushered in a new era of handheld, easily deployable devices that deliver rapid, sensitive, and accurate on-site detection. Such tools empower farmers and agronomists to respond promptly, curbing the spread of bacterial diseases before they devastate crops.
For instance, the bacterial pathogen Xylella fastidiosa—a severe menace to Southern European olive groves—impedes xylem function, leading to plant wilting and eventual death. Handheld devices employing LAMP assays now enable in-field detection of X. fastidiosa DNA within half an hour. This accelerated process supports immediate decisions regarding containment strategies (Elbeaino et al., 2020). A similar approach addresses Erwinia amylovora, the causative agent of fire blight in apple and pear orchards. LFIAs facilitate real-time pathogen identification through a visible test line analogous to home pregnancy tests. With these simple, cost-effective assays, growers can swiftly engage in targeted pruning or other interventions, averting further spread (Braun-Kiewnick et al., 2011).
Microfluidic LOC platforms further streamline bacterial diagnostics. By integrating sample preparation, DNA amplification, and detection into a single, portable device, these systems yield results within an hour. A microfluidic platform developed for Ralstonia solanacearum, a bacterium responsible for wilt diseases in various crops, exemplifies this technology’s potential to guide proactive management decisions (Chu et al., 2024). Concurrently, the fusion of diagnostic tools with smartphone technology leverages built-in high-resolution cameras, computing power, and connectivity. A smartphone-based fluorescence in situ hybridization (FISH) method for Pseudomonas syringae allows real-time data capture and sharing, streamlining collaborative disease surveillance and rapid response strategies (Rodriguez-Moreno et al., 2008).
Viral pathogens pose a formidable challenge to global crop production, frequently causing significant yield reduction and quality deterioration. Swift detection can prevent irreversible losses, yet traditional diagnostics like PCR-based methods require laboratory infrastructure, delaying timely interventions. The proliferation of portable, field-compatible assays has transformed this landscape by enabling immediate diagnosis and control measures at the outbreak’s origin.
For example, the Tomato Yellow Leaf Curl Virus (TYLCV), disseminated by the whitefly Bemisia tabaci, can result in catastrophic yield losses in tomato crops. Innovative, portable LAMP assays bypass the complexities of thermocycling and deliver accurate results in under 30 min, facilitating immediate removal of infected plants and vector management (Zhou et al., 2022). Similarly, Potato Virus Y (PVY) detection, crucial for maintaining seed quality and ensuring stable potato production, is now possible through LFIAs employing virus-specific antibodies. These user-friendly, on-site tests offer high sensitivity, specificity, and rapid results—ideal for non-specialist operators (Danks and Barker, 2000).
The integration of smartphone technology into viral diagnostics offers further versatility. High-resolution imaging combined with cloud-based data sharing and analytical applications streamlines surveillance efforts. A smartphone-adapted gold nanoparticle colorimetric assay for Cucumber Mosaic Virus (CMV) exemplifies how accessible, field-ready platforms can reliably detect viruses. Immediate data processing and geotagged information enhance disease mapping and resource allocation (Rafidah et al., 2016).
Fungal phytopathogens constitute another substantial impediment to global food production, notorious for persistent soilborne infections and rapid, widespread epidemics. Conventional laboratory-based fungal diagnostics are expensive, slow, and reliant on specialized expertise. The advent of portable detection devices has revolutionized how these threats are managed, ensuring swift identification and targeted countermeasures.
A prominent case is Phytophthora infestans, the pathogen behind late blight in potatoes and tomatoes—famously linked to the Irish Potato Famine. Using immunochromatographic LFIAs, farmers gain on-site confirmation of pathogen presence in minutes, expediting fungicide application or removal of infected material (Lu et al., 2020). Similarly, the soil-borne Fusarium oxysporum, capable of lingering in fields and causing devastating wilt diseases, can be detected through portable LAMP assays. These rapid tests enable growers to enact soil treatments promptly, adjust crop rotations, and mitigate subsequent losses (Katoh et al., 2021).
Microfluidic LOC systems further improve fungal pathogen diagnostics by streamlining sample preparation, nucleic acid amplification, and detection. Devices targeting Sclerotinia sclerotiorum, a widespread fungal pathogen affecting crops like soybeans and canola, provide quick and reliable confirmation of pathogen presence on the spot. Additionally, smartphone-coupled diagnostic tools, such as those for Puccinia striiformis, leverage portable microscopy attachments and image analysis software for immediate, in-field detection and communication of disease data (Zhan et al., 2014).
Field-based phytopathogen diagnostics provide essential advantages over traditional centralized laboratory testing, mainly by reducing reliance on labs, which often have long processing times and require specialized equipment and personnel. On-site diagnostic devices allow rapid pathogen detection at the infection site, enabling swift responses to prevent disease spreading. This capability is precious in agricultural regions with limited lab access, empowering farmers to make informed, timely decisions that positively impact crop health and yield.
Successful implementations of field diagnostics illustrate their effectiveness in managing crop diseases. For example, tomato crops face threats from pathogens like Xylella fastidiosa and TYLCV, which can cause severe yield losses if not caught early. Handheld LAMP-based assays for TYLCV have proven effective for rapid, accurate detection in the field, enabling farmers to quickly identify and remove infected plants, thereby reducing the spread of disease. In lettuce cultivation, lab-on-a-chip technologies have been instrumental in managing infections caused by Sclerotinia sclerotiorum, a pathogen that can devastate crops. These portable devices provide results within minutes, allowing targeted fungicide application to protect yields, a clear improvement over traditional lab methods that require days for results (Grabicoski et al., 2020).
Field diagnostics also significantly impact cereal crops, such as wheat, affected by rust caused by Puccinia striiformis. Wheat rust can substantially reduce yields if not promptly controlled. Smartphone-integrated devices allow farmers to detect rust early by capturing and analyzing images of infected leaves via an app, which can also share data with agricultural services for coordinated responses. Real-time analysis and data sharing enhance the effectiveness of interventions like fungicide application and crop rotation, ultimately safeguarding yields. Table 5 presents a performance comparison of various diagnostic devices for plant pathogen detection, detailing their target pathogen types, limits of detection (LOD), response times, and selectivity. This comparison highlights the strengths and applications of different technologies, ranging from rapid detection with high specificity to portable and cost-effective solutions for diverse pathogen types.
Validation of phytopathogen diagnostic devices in resource-poor settings requires robust assessment of specificity, sensitivity, reproducibility, and fitness-for-purpose under field conditions. Resource limitations such as inadequate infrastructure and reagent stability issues necessitate adopting techniques like LAMP, which allows on-site detection without advanced equipment (De Jonghe et al., 2016). Validation begins with stakeholder input to identify pathogens and agroecological constraints, using local sample panels to ensure relevance. For example, validating portable diagnostic devices using local sample panels to detect Ralstonia solanacearum in Kenyan potato fields enhances their relevance and farmer confidence (Okiro et al., 2019). Comparative validation against “gold-standard” methods approved by organizations like IPPC or EPPO is crucial (Conraths and Schares, 2006). A study investigated a nested PCR method against gold-standard techniques, demonstrating its utility for detecting Xanthomonas axonopodis pv. dieffenbachiae, and emphasizing the role of EPPO-endorsed protocols (Chabirand et al., 2013).
A lateral flow device was developed, and PCR-based diagnostic methods were evaluated for Xanthomonas campestris pv. musacearum under field conditions in East Africa, showing high sensitivity and specificity for early disease detection (Hodgetts et al., 2015).
Ruggedness testing is equally vital, ensuring performance under harsh conditions such as fluctuating temperatures or dust. Devices like solar-powered portable PCR kits validated for maize lethal necrosis disease maintained reliability in extreme environments (Snodgrass et al., 2016). Usability testing ensures operational simplicity for users with minimal training, as demonstrated by lateral flow devices (LFDs) for plant pathogen detection, with local technicians in field conditions achieving reliable results through simple operational protocols (Danks and Barker, 2000).
Periodic reassessment of diagnostic tools is crucial to capture pathogen evolution and maintain their robustness. Adaptive frameworks with iterative calibration and field survey feedback ensure diagnostics remain relevant. For example, integrating evolutionary dynamics with immune responses provides insights into pathogen adaptation and highlights iterative calibration’s role in robust management (Restif and Grenfell, 2006). Advances like adaptive sequencing enhance real-time detection and resistance profiling, demonstrating the significance of continuously updated methods (Cheng et al., 2022). Together, these approaches emphasize dynamic, flexible systems essential for addressing evolving pathogen diagnostics and management challenges.
For instance, validated kits for Cassava Brown Streak Virus in Eastern Africa reduced pesticide sprays, improving profitability and reducing environmental harm (Patil et al., 2015). Scalability and sustainability are assessed by evaluating whether diagnostics can be produced locally or maintained with minimal external inputs. Gender-inclusive approaches ensure equitable benefits, particularly for women who play a significant agricultural role (Jost et al., 2015). Integrated validation frameworks that combine diagnostics with data analytics show promise. Handheld LAMP devices paired with smartphone imaging provide real-time geo-referenced disease data for community-wide surveillance, enhancing decision-making and agricultural resilience (Paul et al., 2021). Validated diagnostics in resource-poor settings catalyze sustainable agrarian intensification, resilience to emerging pests, and improved livelihoods.
Furthermore, portable diagnostic devices contribute to environmental sustainability by supporting precision agriculture practices. Accurate, on-site detection allows for targeted treatment of affected plants, reducing the reliance on broad-spectrum pesticide applications. This precision minimizes unnecessary chemical usage, thereby lessening the environmental impact of agricultural activities. Potable diagnostics are vital in advancing sustainable farming methodologies by promoting efficient use of resources and decreasing the potential for harmful side effects on ecosystems. Table 6 compares the three most commonly used portable phytopathogen diagnostic technologies based on their attributes.
While offering rapid, sensitive, and specific diagnostics, portable diagnostic tools for detecting phytopathogens face significant hurdles that limit their effectiveness and adoption. Despite advancements like LFIA and LAMP assays, these devices struggle with sensitivity and specificity under environmental stressors such as temperature and humidity, leading to false positives and negatives. Detecting pathogens at low concentrations remains challenging, hindering their application in early disease detection. Overcoming these technical limitations through enhanced sensitivity and specificity across diverse environments is crucial (Ali et al., 2021; Dey et al., 2023; Archana et al., 2024).
Standardization and quality assurance are equally critical for ensuring the reliability of these tools. A lack of universal testing protocols and calibration procedures undermines result consistency, complicating regulatory approvals and limiting scalability. This inconsistency reduces user confidence, especially among smallholder farmers and agricultural workers, who rely on these devices for on-site diagnostics (Ali et al., 2021). Advances in nanotechnology, molecular diagnostics, and IoT-enabled systems have begun addressing these gaps. For instance, nanotechnology enhances sensitivity and specificity, enabling reliable on-site detection via accessible devices such as smartphones (Li Z. et al., 2020). Molecular diagnostics like real-time PCR and microchip PCR systems integrate built-in quality assurance features such as temperature regulation and amplification monitoring, ensuring dependable pathogen detection in diverse settings (Koo et al., 2013). IoT-enabled tools provide real-time data collection and cloud-based quality assurance, promoting consistent accuracy in field conditions (Buja et al., 2021).
Durability and operational challenges further constrain portable device usability. Harsh field conditions degrade performance, and maintaining devices, including calibration and component replacement, is often infeasible in remote areas. Standardized molecular protocols, like isothermal amplification and next-generation sequencing, reduce variability through uniform sample preparation and device calibration, while user-friendly tools such as biosensors and lateral flow assays improve accessibility for growers and field workers without requiring extensive training (Hariharan and Prasannath, 2021).
Economic barriers compound these challenges, with high upfront costs, recurring consumable expenses, and training requirements making these devices less accessible to smallholder farmers. Inadequate training exacerbates the risk of data misinterpretation, potentially worsening pathogen spread. Additionally, regulatory and ethical considerations, including compliance with regional agricultural laws and data privacy issues linked to cloud-based and smartphone technologies, create further obstacles to widespread adoption. Table 7 illustrates the advantages and limitations of various portable phytopathogen diagnostic devices.
Portable phytopathogen diagnostic tools hold immense commercial potential by mitigating economic losses, enhancing market stability, and driving innovation in agriculture. Early detection of plant diseases minimizes yield losses, stabilizes market supplies, and ensures product quality, addressing challenges from asymptomatic infections and emerging diseases. These tools streamline field applications by eliminating the reliance on centralized laboratories, with real-time PCR systems and nanotechnology-based devices offering rapid and sensitive detection. Their affordability, particularly with smartphone-integrated systems, makes them viable for resource-limited settings, fostering inclusivity in agricultural markets (Paul et al., 2021).
Integration with IoT amplifies their value by linking diagnostics to real-time data management systems, improving disease monitoring and decision-making. Additionally, their applicability extends beyond agriculture to industries like water quality testing, food safety, and environmental monitoring, enabling manufacturers to diversify revenue streams (Islam et al., 2023). These tools also support sustainable agriculture by reducing agrochemical use through precise interventions and meeting consumer demand for eco-friendly, residue-free produce.
Current portable phytopathogen diagnostic devices face significant technical limitations despite miniaturization and field usability advances. A key constraint is throughput; while laboratory methods like high-throughput qPCR or NGS can process large sample volumes efficiently, portable devices are restricted to a few targets per run, limiting their utility for large-scale surveillance. Handheld molecular assays like LAMP improve speed and convenience but are constrained by their inability to handle large sample loads in field conditions, as seen in their limited use for detecting pathogens like Phytophthora infestans and Botrytis cinerea in agricultural settings (Ristaino et al., 2020).
Multiplexing remains another significant hurdle. Detecting multiple pathogens simultaneously is essential for cost-effectiveness but is limited by constraints such as the cross-reactivity of primers and uneven assay performance. Attempts at multiplexing for pathogens like Pseudomonas syringae, Xanthomonas campestris, and Ralstonia solanacearum often result in reduced accuracy, while detecting phylogenetically diverse pathogens (bacteria, fungi, oomycetes, viruses) poses additional biochemical and logistical challenges (Umesha and Avinash, 2015).
Stability of reagents and devices is critical for field deployment. Reagents must withstand environmental fluctuations, but real-world use often shows degradation in sensitivity, as seen in LAMP-based assays for plant viruses requiring cold-chain logistics. Immunoassays also face stability issues, with antibodies losing efficacy under field conditions (Thekisoe et al., 2009).
In West Africa, molecular assays for detecting cassava pathogens like CBSV struggled with reagent stability under high temperatures (Abarshi et al., 2012). Similarly, vineyard surveillance for pathogens like Plasmopara viticola and Erysiphe necator revealed inefficiencies in multiplexed assays and difficulties in managing plant inhibitors in field settings (Possamai and Wiedemann-Merdinoglu, 2022).
Engineering refinements in microfluidics, robust reagent formulations, advanced bioinformatics tools, and integrated digital platforms are essential to address these issues. Emerging methods like CRISPR-based diagnostics show promise but require further validation. Collaboration across disciplines, investment in infrastructure, and regulatory harmonization are necessary to bridge the gap between laboratory capabilities and field requirements, paving the way for more effective integrated pest management systems.
Traditional diagnostic methods, though reliable, are impractical for on-site use, prompting the development of portable systems that integrate molecular biology, engineering, and data analytics. Advances in isothermal amplification techniques like LAMP and recombinase polymerase amplification (RPA) have enabled quick and efficient pathogen detection without requiring complex thermal cycling equipment. These methods have been successfully applied to detect pathogens such as Phytophthora sojae in soybean fields and Ralstonia solanacearum in bacterial wilt cases, providing actionable insights for immediate field interventions (Miles et al., 2015).
CRISPR-Cas systems have further revolutionized diagnostics, offering unparalleled specificity through programmable Cas12 and Cas13 proteins, enabling rapid pathogen identification from simple samples. Such technologies are adaptable and promise applications for various plant diseases (Li et al., 2022; Prasad et al., 2022). Rapid and efficient insulated isothermal PCR method for detecting Fusarium oxysporum directly from banana crop samples, supporting early diagnosis and containment efforts (Chang et al., 2022).
Emerging technologies such as nanopore sequencing are also transforming field diagnostics. Handheld devices like the Oxford Nanopore MinION deliver real-time pathogen identification, offering strain-level precision even in remote locations, although they currently require technical expertise (Player et al., 2020). Smartphones have further enhanced accessibility by integrating high-resolution imaging and cloud-based data sharing for real-time pathogen detection and geotagged disease monitoring. Smartphone-based fluorescence detectors exemplify the potential for user-friendly diagnostic tools that empower farmers with timely insights (Zhang et al., 2023).
The future of portable diagnostics lies in integrating multiplexed detection, machine learning, and user-friendly interfaces to democratize agricultural disease management. Innovations in synthetic biology, biosensors, and nanotechnology are expected to enhance diagnostic accuracy and accessibility further, empowering farmers to mitigate threats with minimal delay and ensuring sustainable agricultural practices in a changing climate. Figure 15 visualizes various phytodiagnostic techniques used in agriculture.
Scalable manufacturing and cost-reduction strategies for portable phytopathogen detection devices focus on optimizing materials, modular designs, and streamlined production processes. Paper-based lateral flow assays are cost-effective due to their simplicity, compatibility with point-of-need applications, and reliance on mass-produced cellulose membranes (Sena-Torralba et al., 2022). Polymers like cyclic olefin copolymer (COC) and poly(methyl methacrylate) (PMMA), used in microfluidic chip fabrication, offer scalable manufacturing via injection molding, while low-cost sensors, such as LEDs and smartphone cameras, reduce reliance on specialized hardware (Liu et al., 2024). Modular designs enable standardization of components, such as interchangeable reagent cartridges, enhancing scalability and reducing per-unit costs.
Reagent stabilization and simplified sample preparation are critical for scalability. Lyophilized primers and isothermal amplification methods like LAMP and RPA enable ambient storage and reduce cold-chain dependence (Carter et al., 2017). Integrated sample-to-answer systems employing miniaturized nucleic acid extraction modules have been successfully used to detect pathogens like Phytophthora infestans in potato fields, empowering smallholder farmers with rapid diagnostic capabilities (Paul et al., 2021). Bulk purchasing of reagents, automated assembly lines, and robotic manufacturing streamline production while maintaining reliability (Ortiz et al., 2017).
Collaborative approaches play a vital role in cost reduction. Partnerships between public and private sectors, supported by agencies like the International Plant Protection Convention (IPPC), facilitate technology transfer, standardization, and large-scale production (Boyer et al., 2016). Domestic manufacturing reduces import costs and enhances local availability in low- and middle-income countries, while philanthropic and governmental support ensures affordability. Automated quality control measures using standardized reference strains, assuring product reliability across regions (Adiga et al., 2018).
Real-world examples highlight these strategies. Mass-produced PCR-based lateral flow kits for Cassava Brown Streak Virus in East Africa demonstrated reduced costs through local manufacturing and smartphone-based detection (Munguti et al., 2024). Similarly, a low-cost lateral flow device for field detection of Xanthomonas campestris pv. musacearum in banana plantations aids in rapid outbreak mitigation and reduces losses (Hodgetts et al., 2015). Such efforts create a sustainable ecosystem for innovation, enabling widespread deployment of portable phytopathogen detection devices and bolstering global food security.
Linking portable phytopathogen diagnostics to global surveillance and early warning systems integrates advanced molecular detection, sensor technologies, and data-driven networks for rapid on-site disease assessment. Portable tools, such as isothermal amplification techniques like LAMP, enable quick and reliable detection without laboratory infrastructure, producing results within 30–60 min (Parida et al., 2008). CRISPR-based detection platforms add specificity and sensitivity, making pathogen identification highly precise and accessible. Smartphone imaging and biosensor systems enhance portability by integrating high-resolution cameras, image recognition, and cloud-based analysis, enabling real-time data sharing with global networks. These advancements align with global initiatives like the IPPC and FAO’s surveillance frameworks, ensuring that diagnostic data feed into platforms like the International Phytosanitary Portal and GPPIS for immediate access by plant health authorities (International Plant Protection Convention (IPPC), 2016). For example, LAMP kits reduce instances of Phytophthora spp. and Phytophthora cactorum in strawberry fields (Siegieda et al., 2021) and rapid diagnostics curtailing downy mildew in vineyards through timely fungicide application (Radhey et al., 2024). As climate shifts alter pathogen distributions, portable diagnostics ensure real-time disease mapping and swift interventions, fortifying global capacity to mitigate threats (Buja et al., 2021).
Empowering local networks through training in assay interpretation, data logging, and sample handling builds decentralized surveillance systems capable of early pathogen detection. Public-private partnerships can improve kit affordability and innovation, while international agencies ensure cross-regional data accuracy through proficiency testing (Markell et al., 2020). Emerging technologies, such as drones and satellites equipped with diagnostic tools, offer the potential for real-time infection mapping and outbreak prediction through integrated data systems. Such innovations advance global preparedness, fostering resilient and collaborative responses to safeguard food security and biodiversity (Abbas et al., 2023).
The development of portable phytopathogen detection devices offers significant potential for modern agriculture by enabling rapid and accurate disease diagnosis to reduce crop losses. To ensure their successful adoption, robust policies, and stakeholder engagement are crucial. Governments should incentivize research and development through grants and tax benefits while fostering public-private partnerships to accelerate prototyping and testing. Clear regulatory guidelines are essential to guarantee safety, reliability, and data security, while privacy policies should balance farmer confidentiality with the benefits of aggregated data for disease monitoring and early warning systems. Market accessibility can be enhanced by subsidies, reduced tariffs, and microcredit systems, particularly for smallholder farmers in low-income regions.
Active stakeholder involvement is critical throughout the development and deployment processes. Farmers, as primary users, must be included in co-design efforts to ensure the devices meet real-world needs. Research institutions and agricultural organizations are instrumental in tailoring detection mechanisms to region-specific pathogens and validating device performance. Collaboration with agrarian technology companies supports mass production and ensures alignment with market demands, while engagement with policymakers facilitates streamlined regulatory approvals and integration with agricultural health priorities. International organizations like the FAO can provide technical assistance, advocate for standardization, and support deployment in underserved areas. Additionally, NGOs can enhance access in remote farming communities.
Building capacity among agricultural extension workers ensures that the devices are correctly utilized, maximizing their effectiveness. Cost barriers and adoption resistance can be addressed through subsidized manufacturing, NGO partnerships, training programs, and demonstration projects to build trust and demonstrate value. Interoperability issues can be resolved with open data standards and modular designs, enhancing device usability across different systems and regions. A cohesive approach integrating strong policies with comprehensive stakeholder engagement is vital for the widespread adoption of these diagnostic technologies. Such efforts will strengthen agricultural productivity and resilience, ensuring these innovations fulfill their potential to combat crop diseases and enhance global food security.
Portable diagnostic technologies for plant pathogens present a transformative opportunity in agriculture by enabling rapid, on-site detection that bridges the gap between disease identification and response. These devices offer significant benefits, particularly in their capacity to reduce dependency on centralized laboratory facilities, thus democratizing access to timely and precise diagnostic tools. Current advancements have integrated smartphone technologies, IoT, and machine learning, underscoring the potential of portable diagnostics to become indispensable tools in modern agricultural practices. By fostering rapid pathogen detection, these technologies directly support sustainable agriculture, enhancing food security through improved plant health management. Portable diagnostic tools contribute to reduced chemical usage and allow for more precise interventions, thereby minimizing environmental impacts and supporting long-term ecological balance.
For stakeholders, including researchers, policymakers, and industry players, the potential of portable diagnostics in agriculture calls for coordinated efforts to address existing challenges such as standardization, cost barriers, and regulatory considerations. Researchers should focus on improving the sensitivity and specificity of these devices and on developing robust, field-deployable technologies that can withstand diverse agricultural conditions. Policymakers play a crucial role in supporting frameworks that encourage the adoption of portable diagnostic tools while maintaining data privacy and security standards. Meanwhile, industry players are encouraged to invest in and develop cost-effective, scalable solutions accessible to small-scale farmers and large agricultural operations.
Looking ahead, the future of portable diagnostics in agriculture is promising. Emerging technologies, such as nanotechnology and microfluidic systems, will enhance these devices’ precision and utility, allowing for early detection of pathogens even at low concentrations. As agriculture increasingly embraces digital transformation, integrating portable diagnostics with predictive analytics, precision farming, and real-time data-sharing platforms could revolutionize how plant diseases are managed. This shift towards a more connected, data-driven approach holds the potential to mitigate crop losses and bolster global food security by enabling proactive, tailored disease management solutions across diverse agricultural landscapes.
AY: Conceptualization, Writing – original draft, Writing – review & editing. KY: Conceptualization, Writing – review & editing.
The author(s) declare that no financial support was received for the research, authorship, and/or publication of this article.
The authors declare that the research was conducted in the absence of any commercial or financial relationships that could be construed as a potential conflict of interest.
The authors declare that no Gen AI was used in the creation of this manuscript.
All claims expressed in this article are solely those of the authors and do not necessarily represent those of their affiliated organizations, or those of the publisher, the editors and the reviewers. Any product that may be evaluated in this article, or claim that may be made by its manufacturer, is not guaranteed or endorsed by the publisher.
Abarshi, M. M., Mohammed, I. U., Jeremiah, S. C., Legg, J. P., Kumar, P. L., Hillocks, R. J., et al. (2012). Multiplex RT-PCR assays for the simultaneous detection of both RNA and DNA viruses infecting cassava and the common occurrence of mixed infections by two cassava brown streak viruses in East Africa. J. Virol. Methods 179, 176–184. doi: 10.1016/j.jviromet.2011.10.020
Abbas, A., Zhang, Z., Zheng, H., Alami, M. M., Alrefaei, A. F., Abbas, Q., et al. (2023). Drones in plant disease assessment, efficient monitoring, and detection: a way forward to Smart agriculture. Agronomy 13:1524. doi: 10.3390/agronomy13061524
Abd Rahman, S. F., Yusof, N. A., Md Arshad, M. K., Hashim, U., Md Nor, M. N., and Hamidon, M. N. (2022). Fabrication of silicon nanowire sensors for highly sensitive pH and DNA hybridization detection. Nanomaterials 12:2652. doi: 10.3390/nano12152652
Adiga, R., Al-Adhami, M., Andar, A., Borhani, S., Brown, S., Burgenson, D., et al. (2018). Point-of-care production of therapeutic proteins of good-manufacturing-practice quality. Nat. Biomed. Eng. 2, 675–686. doi: 10.1038/s41551-018-0259-1
Ahmad-Nejad, P., Ashavaid, T., Vacaflores Salinas, A., Huggett, J., Harris, K., Linder, M. W., et al. (2021). Current and future challenges in quality assurance in molecular diagnostics. Clin. Chim. Acta 519, 239–246. doi: 10.1016/j.cca.2021.05.004
Alfozan, S. S., and Hassan, M. M. (2021). MajraDoc an image based disease detection app for agricultural plants using deep learning techniques. Proceedings of the CS & IT Conference Proceedings.
Ali, Q., Ahmar, S., Sohail, M. A., Kamran, M., Ali, M., Saleem, M. H., et al. (2021). Research advances and applications of biosensing technology for the diagnosis of pathogens in sustainable agriculture. Environ. Sci. Pollut. Res. Int. 28, 9002–9019. doi: 10.1007/s11356-021-12419-6
Ali, Z., Sanchez, E., Tehseen, M., Mahas, A., Marsic, T., Aman, R., et al. (2022). Bio-SCAN: A CRISPR/dCas9-based lateral flow assay for rapid, specific, and sensitive detection of SARS-CoV-2. ACS Synth. Biol. 11, 406–419. doi: 10.1021/acssynbio.1c00499
Alzahrani, M. S., and Alsaade, F. W. (2023). Transform and deep learning algorithms for the early detection and recognition of tomato leaf disease. Agronomy 13:1184. doi: 10.3390/agronomy13051184
Amoia, S. S., Loconsole, G., Ligorio, A., Pantazis, A. K., Papadakis, G., Gizeli, E., et al. (2023). A colorimetric LAMP detection of Xylella fastidiosa in crude alkaline sap of olive trees in Apulia as a field-based tool for disease containment. Agriculture 13:448. doi: 10.3390/agriculture13020448
Archana, T. S., Kumar, D., Ghosh, P., and Kumar, V. (2024). Plant pathogen detection: exploring modern techniques and future directions. Indian Phytopathol. 77, 573–585. doi: 10.1007/s42360-024-00762-w
Asani, E. O., Osadeyi, Y. P., Adegun, A. A., Viriri, S., Ayoola, J. A., and Kolawole, E. A. (2023). mPD-APP: a mobile-enabled plant diseases diagnosis application using convolutional neural network toward the attainment of a food secure world. Front. Artif. Intell. 6:1227950. doi: 10.3389/frai.2023.1227950
Aslam, S., Tahir, A., Aslam, M. F., Alam, M. W., Shedayi, A. A., and Sadia, S. (2017). Recent advances in molecular techniques for the identification of phytopathogenic fungi—a mini review. J. Plant Interact. 12, 493–504. doi: 10.1080/17429145.2017.1397205
Attaluri, S., and Dharavath, R. (2023). Novel plant disease detection techniques-a brief review. Mol. Biol. Rep. 50, 9677–9690. doi: 10.1007/s11033-023-08838-y
Avila-Huerta, M. D., Ortiz-Riano, E. J., Mancera-Zapata, D. L., and Morales-Narvaez, E. (2020). Real-time photoluminescent biosensing based on graphene oxide-coated microplates: a rapid pathogen detection platform. Anal. Chem. 92, 11511–11515. doi: 10.1021/acs.analchem.0c02200
Ayaz, M., Ammad-Uddin, M., Sharif, Z., Mansour, A., and Aggoune, E.-H. M. (2019). Internet-of-things (IoT)-based Smart agriculture: toward making the fields talk. IEEE Access 7, 129551–129583. doi: 10.1109/access.2019.2932609
Baek, S. H., Lee, S. W., Kim, E. J., Shin, D. H., Lee, S. W., and Park, T. J. (2017). Portable agrichemical detection system for enhancing the safety of agricultural products using aggregation of gold nanoparticles. ACS Omega 2, 988–993. doi: 10.1021/acsomega.6b00477
Banerjee, R., and Jaiswal, A. (2018). Recent advances in nanoparticle-based lateral flow immunoassay as a point-of-care diagnostic tool for infectious agents and diseases. Analyst 143, 1970–1996. doi: 10.1039/c8an00307f
Barman, U., Sarma, P., Rahman, M., Deka, V., Lahkar, S., Sharma, V., et al. (2024). ViT-SmartAgri: vision transformer and smartphone-based plant disease detection for smart agriculture. Agronomy 14:327. doi: 10.3390/agronomy14020327
Bawa, A., Baath, G., Juneja, P., and Brar, J. (2024). “Drone mapping for agricultural sustainability: applications and benefits” in Sustainable development using geospatial techniques, Wiley, USA: Scrivener Publishing. 411–436.
Bawingan, P. A., Montevirgen, E. M. Jr., Canuto, P. P. L., Lucas, L. E., and Pumaras, J. C. (2024). Plant identification mobile apps: users’ difficulties and impressions. Asian J. Biol. Educ. 16, 15–21. doi: 10.57443/ajbe.16.0_15
Bergwerff, A. A., and van Knapen, F. (2006). Surface plasmon resonance biosensors for detection of pathogenic microorganisms: strategies to secure food and environmental safety. J. AOAC Int. 89, 826–831. doi: 10.1093/jaoac/89.3.826
Bobrinetskiy, I., Radovic, M., Rizzotto, F., Vizzini, P., Jaric, S., Pavlovic, Z., et al. (2021). Advances in nanomaterials-based electrochemical biosensors for foodborne pathogen detection. Nanomaterials 11:2700. doi: 10.3390/nano11102700
Boonham, N., Kreuze, J., Winter, S., van der Vlugt, R., Bergervoet, J., Tomlinson, J., et al. (2014). Methods in virus diagnostics: from ELISA to next generation sequencing. Virus Res. 186, 20–31. doi: 10.1016/j.virusres.2013.12.007
Boukabouya, R. A., Moussaoui, A., and Berrimi, M. (2022). Vision transformer based models for plant disease detection and diagnosis. Proceedings of the 2022 5th International Symposium on Informatics and its Applications (ISIA). 1–6.
Boyer, E. J., Van Slyke, D. M., and Rogers, J. D. (2016). An empirical examination of public involvement in public-private partnerships: qualifying the benefits of public involvement in PPPs. J. Public Adm. Res. Theory 26, 45–61. doi: 10.1093/jopart/muv008
Braun-Kiewnick, A., Altenbach, D., Oberhansli, T., Bitterlin, W., and Duffy, B. (2011). A rapid lateral-flow immunoassay for phytosanitary detection of Erwinia amylovora and on-site fire blight diagnosis. J. Microbiol. Methods 87, 1–9. doi: 10.1016/j.mimet.2011.06.015
Buja, I., Sabella, E., Monteduro, A. G., Chiriaco, M. S., De Bellis, L., Luvisi, A., et al. (2021). Advances in plant disease detection and monitoring: from traditional assays to in-field diagnostics. Sensors 21:2129. doi: 10.3390/s21062129
Calle, J. P. R., and Böckmann, E.. (2020). D3.5: Report on case studies. WP3 – Benchmarking methodologies and technologies. SmartProtech Consortium, Eropean Comission.
Carter, C., Akrami, K., Hall, D., Smith, D., and Aronoff-Spencer, E. (2017). Lyophilized visually readable loop-mediated isothermal reverse transcriptase nucleic acid amplification test for detection Ebola Zaire RNA. J. Virol. Methods 244, 32–38. doi: 10.1016/j.jviromet.2017.02.013
Cetin, A. E., Coskun, A. F., Galarreta, B. C., Huang, M., Herman, D., Ozcan, A., et al. (2014). Handheld high-throughput plasmonic biosensor using computational on-chip imaging. Light Sci. Appl. 3:e122. doi: 10.1038/lsa.2014.3
Cetin, A. E., Kocer, Z. A., Topkaya, S. N., and Yazici, Z. A. (2021). Handheld plasmonic biosensor for virus detection in field-settings. Sens. Actuators B 344:130301. doi: 10.1016/j.snb.2021.130301
Chabirand, A., Jouen, E., Pruvost, O., Chiroleu, F., Hostachy, B., Bergsma-Vlami, M., et al. (2013). Comparative and collaborative studies for the validation of a nested PCR for the detection of Xanthomonas axonopodis pv. dieffenbachiae from Anthurium samples. Plant Pathol. 63, 20–30. doi: 10.1111/ppa.12083
Chakraborty, B., and Chakraborty, U. (2021). Molecular detection of fungal pathogens and induction of phytoimmunity using bioinoculants. Indian Phytopathol. 74, 307–322. doi: 10.1007/s42360-021-00351-1
Chang, T. D., Huang, L. N., Lin, Y. J., Wu, Z. B., Tsai, S. H., and Lin, Y. H. (2022). Rapid detection of Fusarium oxysporum using insulated isothermal PCR and a rapid, simple DNA preparation protocol. Int. J. Mol. Sci. 23:13253. doi: 10.3390/ijms232113253
Chauhan, N., and Pundir, C. S. (2011). An amperometric biosensor based on acetylcholinesterase immobilized onto iron oxide nanoparticles/multi-walled carbon nanotubes modified gold electrode for measurement of organophosphorus insecticides. Anal. Chim. Acta 701, 66–74. doi: 10.1016/j.aca.2011.06.014
Chen, C.-J., Li, Y.-S., Tai, C.-Y., Chen, Y.-C., and Huang, Y.-M. (2022). Pest incidence forecasting based on Internet of Things and long short-term memory network. Appl. Soft Comput. 124:108895. doi: 10.1016/j.asoc.2022.108895
Chen, W., Yu, H., Sun, F., Ornob, A., Brisbin, R., Ganguli, A., et al. (2017). Mobile platform for multiplexed detection and differentiation of disease-specific nucleic acid sequences, using microfluidic loop-mediated isothermal amplification and smartphone detection. Anal. Chem. 89, 11219–11226. doi: 10.1021/acs.analchem.7b02478
Chen, C. A., Yuan, H., Chen, C. W., Chien, Y. S., Sheng, W. H., and Chen, C. F. (2021). An electricity- and instrument-free infectious disease sensor based on a 3D origami paper-based analytical device. Lab Chip 21, 1908–1915. doi: 10.1039/d1lc00079a
Cheng, H., Sun, Y., Yang, Q., Deng, M., Yu, Z., Zhu, G., et al. (2022). A rapid bacterial pathogen and antimicrobial resistance diagnosis workflow using Oxford Nanopore adaptive sequencing method. Brief. Bioinform. 23:bbac453. doi: 10.1093/bib/bbac453
Chu, L. T., Laxman, D., Abdelhamed, J., Pirlo, R. K., Fan, F., Wagner, N., et al. (2024). Development of a tomato xylem-mimicking microfluidic system to study Ralstonia pseudosolanacearum biofilm formation. Front. Bioeng. Biotechnol. 12:1395959. doi: 10.3389/fbioe.2024.1395959
Conraths, F. J., and Schares, G. (2006). Validation of molecular-diagnostic techniques in the parasitological laboratory. Vet. Parasitol. 136, 91–98. doi: 10.1016/j.vetpar.2005.12.004
Crocombe, R. A. (2018). Portable spectroscopy. Appl. Spectrosc. 72, 1701–1751. doi: 10.1177/0003702818809719
Currie, E. H. (2021). “PSoC creator” in Mixed-signal embedded systems design: a hands-on guide to the cypress PSoC (Cham: Springer), 225–290.
Danks, C., and Barker, I. (2000). On-site detection of plant pathogens using lateral-flow devices. Eppo Bull. 30, 421–426. doi: 10.1111/j.1365-2338.2000.tb00922.x
de Almeida, C., Panhoca, E., Bonfante, G. F. J., De Carvalho Paulino, J. F., De Deus, B. C., Patr Icio, F., et al. (2021). Precise assessment of angular leaf spot severity using the leaf doctor app for common beans. Afr. J. Biotechnol. 20, 169–174. doi: 10.5897/AJB2021.17324
de Campos da Costa, J. P., Bastos, W. B., da Costa, P. I., Zaghete, M. A., Longo, E., and Carmo, J. P. (2019). Portable laboratory platform with electrochemical biosensors for immunodiagnostic of hepatitis C virus. IEEE Sensors J. 19, 10701–10709. doi: 10.1109/jsen.2019.2930957
De Jonghe, K., De Roo, I., and Maes, M. (2016). Fast and sensitive on-site isothermal assay (LAMP) for diagnosis and detection of three fruit tree phytoplasmas. Eur. J. Plant Pathol. 147, 749–759. doi: 10.1007/s10658-016-1039-y
Dean, R., Van Kan, J. A., Pretorius, Z. A., Hammond-Kosack, K. E., Di Pietro, A., Spanu, P. D., et al. (2012). The top 10 fungal pathogens in molecular plant pathology. Mol. Plant Pathol. 13, 414–430. doi: 10.1111/j.1364-3703.2011.00783.x
Debnath, A., Hasan, M. M., Raihan, M., Samrat, N., Alsulami, M. M., Masud, M., et al. (2023). A smartphone-based detection system for tomato leaf disease using EfficientNetV2B2 and its explainability with artificial intelligence (AI). Sensors 23:8685. doi: 10.3390/s23218685
DeShields, J. B., Bomberger, R. A., Woodhall, J. W., Wheeler, D. L., Moroz, N., Johnson, D. A., et al. (2018). On-site molecular detection of soil-borne phytopathogens using a portable real-time PCR system. J. Vis. Exp. 132:e56891. doi: 10.3791/56891
Dey, M. K., Iftesum, M., Devireddy, R., and Gartia, M. R. (2023). New technologies and reagents in lateral flow assay (LFA) designs for enhancing accuracy and sensitivity. Anal. Methods 15, 4351–4376. doi: 10.1039/d3ay00844d
Dholu, M., and Ghodinde, K. A. (2018). Internet of Things (IoT) for precision agriculture application. Proceedings of the 2018 2nd International Conference on Trends in Electronics and Informatics (ICOEI). 339–342.
Di Nardo, F., Alladio, E., Baggiani, C., Cavalera, S., Giovannoli, C., Spano, G., et al. (2019). Colour-encoded lateral flow immunoassay for the simultaneous detection of aflatoxin B1 and type-B fumonisins in a single test line. Talanta 192, 288–294. doi: 10.1016/j.talanta.2018.09.037
Dimov, I. K., Garcia-Cordero, J. L., O’Grady, J., Poulsen, C. R., Viguier, C., Kent, L., et al. (2008). Integrated microfluidic tmRNA purification and real-time NASBA device for molecular diagnostics. Lab Chip 8, 2071–2078. doi: 10.1039/b812515e
Dou, Y., Su, J., Chen, S., Li, T., Wang, L., Ding, X., et al. (2022). A smartphone-based three-in-one biosensor for co-detection of SARS-CoV-2 viral RNA, antigen and antibody. Chem. Commun. 58, 6108–6111. doi: 10.1039/d2cc01297a
Duarte-Carvajalino, J. M., Alzate, D. F., Ramirez, A. A., Santa-Sepulveda, J. D., Fajardo-Rojas, A. E., and Soto-Suárez, M. (2018). Evaluating late blight severity in potato crops using unmanned aerial vehicles and machine learning algorithms. Remote Sens. 10:1513. doi: 10.3390/rs10101513
Elbeaino, T., Incerti, O., Dakroub, H., Valentini, F., and Huang, Q. (2020). Development of an FTP-LAMP assay based on TaqMan real-time PCR and LAMP for the specific detection of Xylella fastidiosa De Donno and mulberry strains in both plants and insect vectors. J. Microbiol. Methods 175:105992. doi: 10.1016/j.mimet.2020.105992
Enkvetchakul, P., and Surinta, O. (2022). Effective data augmentation and training techniques for improving deep learning in plant leaf disease recognition. Appl. Sci. Eng. Prog. 15:3810. doi: 10.14416/j.asep.2021.01.003
Fan, Y., Li, J., Guo, Y., Xie, L., and Zhang, G. (2021). Digital image colorimetry on smartphone for chemical analysis: a review. Measurement 171:108829. doi: 10.1016/j.measurement.2020.108829
Fujikawa, T., and Iwanam, T. (2012). Sensitive and robust detection of citrus greening bacterium “Candidatus Liberibacter asiaticus” by DNA extraction-free loop-mediated isothermal amplification method. Applied Microbiology and Biotechnology. 94, 529–539.
Foudeh, A. M., Fatanat Didar, T., Veres, T., and Tabrizian, M. (2012). Microfluidic designs and techniques using lab-on-a-chip devices for pathogen detection for point-of-care diagnostics. Lab Chip 12, 3249–3266. doi: 10.1039/c2lc40630f
Fountas, S., Espejo-García, B., Kasimati, A., Mylonas, N., and Darra, N. (2020). The future of digital agriculture: technologies and opportunities. IT Prof. 22, 24–28. doi: 10.1109/MITP.2019.2963412
Gawade, D. R. (2022). “Development of a low-power, battery-less near field communication sensor transponder for wireless sensing applications” in MRes thesis (Cork: University College Cork).
Gilligan, C. A. (2024). Developing predictive models and early warning Systems for Invading Pathogens: wheat rusts. Annu. Rev. Phytopathol. 62, 217–241. doi: 10.1146/annurev-phyto-121423-041956
Gonzalez-Gonzalez, E., Trujillo-de Santiago, G., Lara-Mayorga, I. M., Martinez-Chapa, S. O., and Alvarez, M. M. (2020). Portable and accurate diagnostics for COVID-19: combined use of the miniPCR thermocycler and a well-plate reader for SARS-CoV-2 virus detection. PLoS One 15:e0237418. doi: 10.1371/journal.pone.0237418
Grabicoski, E. M. G., Jaccoud-Filho, D. S., Lee, D., Henneberg, L., and Pileggi, M. (2020). Real-time quantitative and ion-metal Indicator LAMP-based assays for rapid detection of Sclerotinia sclerotiorum. Plant Dis. 104, 1514–1526. doi: 10.1094/PDIS-07-19-1455-RE
Gudkov, S. V., Matveeva, T. A., Sarimov, R. M., Simakin, A. V., Stepanova, E. V., Moskovskiy, M. N., et al. (2023). Optical methods for the detection of plant pathogens and diseases. AgriEngineering 5, 1789–1812. doi: 10.3390/agriengineering5040110
Gutiérrez-Aguirre, I., Hodnik, V., Glais, L., Rupar, M., Jacquot, E., Anderluh, G., et al. (2014). Surface plasmon resonance for monitoring the interaction of Potato virus Y with monoclonal antibodies. Anal. Biochem. 447, 74–81. doi: 10.1016/j.ab.2013.10.032
Hariharan, G., and Prasannath, K. (2021). Recent advances in molecular diagnostics of fungal plant pathogens: a mini review. Front. Cell. Infect. Microbiol. 10:600234. doi: 10.3389/fcimb.2020.600234
Harper, S. J., Ward, L. I., and Clover, G. R. (2010). Development of LAMP and real-time PCR methods for the rapid detection of Xylella fastidiosa for quarantine and field applications. Phytopathology 100, 1282–1288. doi: 10.1094/PHYTO-06-10-0168
Hodgetts, J., Karamura, G., Johnson, G., Hall, J., Perkins, K., Beed, F., et al. (2015). Development of a lateral flow device for in-field detection and evaluation of PCR-based diagnostic methods for Xanthomonas campestris pv. musacearum, the causal agent of banana Xanthomonas wilt. Plant Pathol. 64, 559–567. doi: 10.1111/ppa.12289
Hohenstein, J., O’Dell, D., Murnane, E. L., Lu, Z., Erickson, D., and Gay, G. (2017). Enhancing the usability of an optical reader system to support point-of-care rapid diagnostic testing: an iterative design approach. JMIR Hum. Factors 4:e29. doi: 10.2196/humanfactors.8621
Hornero, A., Hernández-Clemente, R., North, P. R. J., Beck, P. S. A., Boscia, D., Navas-Cortes, J. A., et al. (2020). Monitoring the incidence of Xylella fastidiosa infection in olive orchards using ground-based evaluations, airborne imaging spectroscopy and Sentinel-2 time series through 3-D radiative transfer modelling. Remote Sens. Environ. 236:111480. doi: 10.1016/j.rse.2019.111480
Hsieh, K., Ferguson, B. S., Eisenstein, M., Plaxco, K. W., and Soh, H. T. (2015). Integrated electrochemical microsystems for genetic detection of pathogens at the point of care. Acc. Chem. Res. 48, 911–920. doi: 10.1021/ar500456w
Hu, B., Li, J., Mou, L., Liu, Y., Deng, J., Qian, W., et al. (2017). An automated and portable microfluidic chemiluminescence immunoassay for quantitative detection of biomarkers. Lab Chip 17, 2225–2234. doi: 10.1039/c7lc00249a
Huang, X., Xu, D., Chen, J., Liu, J., Li, Y., Song, J., et al. (2018). Smartphone-based analytical biosensors. Analyst 143, 5339–5351. doi: 10.1039/c8an01269e
Ijaz, S., Haq, I. U., Mukhtar, S., and Habib, Z. (2022). “Molecular phytopathometry” in Trends in plant disease assessment (Singapore: Springer), 167–201.
International Plant Protection Convention (IPPC). (2016). 2016 IPPC Annual Report. Rome, Italy: Food and Agriculture Organization of the United Nations.
Islam, S., Hasan, M. Z., and Kabir, S. A. (2023). Smart agriculture system for plant disease detection and irrigation management using machine learning and IoT. In Proceedings of the 2023 5th International Conference on Sustainable Technologies for Industry 5.0 (STI). 1–6.
Ivanov, A. V., Safenkova, I. V., Zherdev, A. V., and Dzantiev, B. B. (2020). Nucleic acid lateral flow assay with recombinase polymerase amplification: solutions for highly sensitive detection of RNA virus. Talanta 210:120616. doi: 10.1016/j.talanta.2019.120616
Ivanov, A. V., Safenkova, I. V., Zherdev, A. V., and Dzantiev, B. B. (2021). The potential use of isothermal amplification assays for in-field diagnostics of plant pathogens. Plants 10:2424. doi: 10.3390/plants10112424
Jones, J. T., Haegeman, A., Danchin, E. G., Gaur, H. S., Helder, J., Jones, M. G., et al. (2013). Top 10 plant-parasitic nematodes in molecular plant pathology. Mol. Plant Pathol. 14, 946–961. doi: 10.1111/mpp.12057
Jost, C., Kyazze, F., Naab, J., Neelormi, S., Kinyangi, J., Zougmore, R., et al. (2015). Understanding gender dimensions of agriculture and climate change in smallholder farming communities. Clim. Dev. 8, 133–144. doi: 10.1080/17565529.2015.1050978
Kamoun, S., Furzer, O., Jones, J. D., Judelson, H. S., Ali, G. S., Dalio, R. J., et al. (2015). The top 10 oomycete pathogens in molecular plant pathology. Mol. Plant Pathol. 16, 413–434. doi: 10.1111/mpp.12190
Karadurmus, L., Kaya, S. I., and Ozkan, S. A. (2021). Recent advances of enzyme biosensors for pesticide detection in foods. J. Food Meas. Charact. 15, 4582–4595. doi: 10.1007/s11694-021-01032-3
Kashyap, P. L., Kumar, S., and Srivastava, A. K. (2016a). Nanodiagnostics for plant pathogens. Environ. Chem. Lett. 15, 7–13. doi: 10.1007/s10311-016-0580-4
Kashyap, P. L., Rai, P., Sharma, S., Chakdar, H., Kumar, S., Pandiyan, K., et al. (2016b). “Nanotechnology for the detection and diagnosis of plant pathogens” in Nanoscience in food and agriculture 2. Sustainable agriculture reviews. eds. S. Ranjan, N. Dasgupta, and E. Lichtfouse (Cham: Springer), 253–276.
Katoh, H., Yamazaki, S., Fukuda, T., Sonoda, S., Nishigawa, H., and Natsuaki, T. (2021). Detection of Fusarium oxysporum f. sp. fragariae by using loop-mediated isothermal amplification. Plant Dis. 105, 1072–1079. doi: 10.1094/PDIS-03-20-0590-RE
Kaur, J., and Singh, P. K. (2020). Enzyme-based optical biosensors for organophosphate class of pesticide detection. Phys. Chem. Chem. Phys. 22, 15105–15119. doi: 10.1039/d0cp01647k
Khuwaja, K., Aliza, A., Mukhtiar, N., Tarcă, R., Noje, D., Juman, M., et al. (2023). Sustainable agriculture: an IoT-based solution for early disease detection in greenhouses. Proceedings of the 2023 17th International Conference on Engineering of Modern Electric Systems (EMES). 1–4.
Koo, C., Malapi-Wight, M., Kim, H. S., Cifci, O. S., Vaughn-Diaz, V. L., Ma, B., et al. (2013). Development of a real-time microchip PCR system for portable plant disease diagnosis. PLoS One 8:e82704. doi: 10.1371/journal.pone.0082704
Kou, X., Tong, L., Shen, Y., Zhu, W., Yin, L., Huang, S., et al. (2020). Smartphone-assisted robust enzymes@MOFs-based paper biosensor for point-of-care detection. Biosens. Bioelectron. 156:112095. doi: 10.1016/j.bios.2020.112095
Kumar, C. V., Saritha, K., Reddy, M. V. S. S., and Pai, H. A. (2023) IoT based smart agriculture for the detection of plant decay. Proceedings of the 2023 7th International Conference on Computing Methodologies and Communication (ICCMC). 1376–1379.
Lau, H. Y., and Botella, J. R. (2016). Advanced DNA-based point-of-care diagnostic methods for plant diseases detection. Front. Plant Sci. 8:2016. doi: 10.3389/fpls.2017.02016
Lei, K. F., Lee, K.-F., and Yang, S.-I. (2012). Fabrication of carbon nanotube-based pH sensor for paper-based microfluidics. Microelectron. Eng. 100, 1–5. doi: 10.1016/j.mee.2012.07.113
Li, L., Duan, C., Weng, J., Qi, X., Liu, C., Li, X., et al. (2022). A field-deployable method for single and multiplex detection of DNA or RNA from pathogens using Cas12 and Cas13. Sci. China Life Sci. 65, 1456–1465. doi: 10.1007/s11427-021-2028-x
Li, Z., Paul, R., Ba Tis, T., Saville, A. C., Hansel, J. C., Yu, T., et al. (2019). Non-invasive plant disease diagnostics enabled by smartphone-based fingerprinting of leaf volatiles. Nat. Plants 5, 856–866. doi: 10.1038/s41477-019-0476-y
Li, X., Tian, J., Garnier, G., and Shen, W. (2010). Fabrication of paper-based microfluidic sensors by printing. Colloids Surf. B 76, 564–570. doi: 10.1016/j.colsurfb.2009.12.023
Li, Z., Yu, T., Paul, R., Fan, J., Yang, Y., and Wei, Q. (2020). Agricultural nanodiagnostics for plant diseases: recent advances and challenges. Nanoscale Adv. 2, 3083–3094. doi: 10.1039/c9na00724e
Li, D., Zhang, P., Chen, T., and Qin, W. (2020). Recent development and challenges in spectroscopy and machine vision technologies for crop nitrogen diagnosis: a review. Remote Sens. 12:2578. doi: 10.3390/rs12162578
Liu, X., Sun, A., Brodsky, J., Gablech, I., Lednicky, T., Voparilova, P., et al. (2024). Microfluidics chips fabrication techniques comparison. Sci. Rep. 14:28793. doi: 10.1038/s41598-024-80332-2
Lu, X., Zheng, Y., Zhang, F., Yu, J., Dai, T., Wang, R., et al. (2020). A rapid, equipment-free method for detecting Phytophthora infestans in the field using a lateral flow strip-based recombinase polymerase amplification assay. Plant Dis. 104, 2774–2778. doi: 10.1094/PDIS-01-20-0203-SC
Maginga, T. J., Masabo, E., Bakunzibake, P., Kim, K. S., and Nsenga, J. (2024). Using wavelet transform and hybrid CNN - LSTM models on VOC & ultrasound IoT sensor data for non-visual maize disease detection. Heliyon 10:e26647. doi: 10.1016/j.heliyon.2024.e26647
Mahlein, A.-K. (2016). Plant disease detection by imaging sensors-parallels and specific demands for precision agriculture and plant phenotyping. Plant Dis. 100, 241–251. doi: 10.1094/PDIS-03-15-0340-FE
Mandal, R., Lohar, P., and Wakalkar, P. (2022). CROP DOCTOR A prediction model using machine learning algorithm. Proceedings of the 4th International Conference on Communication & Information Processing (ICCIP).
Mansfield, J., Genin, S., Magori, S., Citovsky, V., Sriariyanum, M., Ronald, P., et al. (2012). Top 10 plant pathogenic bacteria in molecular plant pathology. Mol. Plant Pathol. 13, 614–629. doi: 10.1111/j.1364-3703.2012.00804.x
Markell, S. G., Tylka, G. L., Anderson, E. J., and van Esse, H. P. (2020). Developing public-private partnerships in plant pathology extension: case studies and opportunities in the United States. Annu. Rev. Phytopathol. 58, 161–180. doi: 10.1146/annurev-phyto-030320-041359
Martinez, A. W., Phillips, S. T., Whitesides, G. M., and Carrilho, E. (2010). Diagnostics for the developing world: microfluidic paper-based analytical devices. Anal. Chem. 82, 3–10. doi: 10.1021/ac9013989
Meno, L., Escuredo, O., Abuley, I. K., and Seijo, M. C. (2023). Predicting daily aerobiological risk level of potato late blight using C5.0 and random forest algorithms under field conditions. Sensors 23:3818. doi: 10.3390/s23083818
METOS. (2005). Available at: https://metos.global/en/fieldclimate/. (Accessed October 19, 2005)
Miles, T. D., Martin, F. N., and Coffey, M. D. (2015). Development of rapid isothermal amplification assays for detection of Phytophthora spp. in plant tissue. Phytopathology 105, 265–278. doi: 10.1094/PHYTO-05-14-0134-R
Milson, S., and Kevin, C. (2023). Lab-on-a-chip technologies: transforming diagnostics with microfluidics, 2516–2314.
Morcia, C., Piazza, I., Ghizzoni, R., Terzi, V., Carrara, I., Bolli, G., et al. (2023). Molecular diagnostics in tomato: chip digital PCR assays targeted to identify and quantify Clavibacter michiganensis subsp. michiganensis and Ralstonia solanacearum in planta. Horticulturae 9:553. doi: 10.3390/horticulturae9050553
Moreau, A. L., Janissen, R., Santos, C. A., Peroni, L. A., Stach-Machado, D. R., de Souza, A. A., et al. (2012). Highly-sensitive and label-free indium phosphide biosensor for early phytopathogen diagnosis. Biosens. Bioelectron. 36, 62–68. doi: 10.1016/j.bios.2012.03.038
Mrisho, L. M., Mbilinyi, N. A., Ndalahwa, M., Ramcharan, A. M., Kehs, A. K., McCloskey, P. C., et al. (2020). Accuracy of a smartphone-based object detection model, PlantVillage Nuru, in identifying the foliar symptoms of the viral diseases of cassava-CMD and CBSD. Front. Plant Sci. 11:590889. doi: 10.3389/fpls.2020.590889
Munguti, F. M., Kilalo, D. C., Yegon, H. K., Macharia, I., Seal, S. E., Mwango’mbe, A. W., et al. (2024). Real-time reverse transcription recombinase polymerase amplification (RT-RPA) assay for detection of cassava brown streak viruses. Sci. Rep. 14:12438. doi: 10.1038/s41598-024-62249-y
Mwebaze, E., Gebru, T., Frome, A., Nsumba, S., and Tusubira, J.. (2019). iCassava 2019 fine-grained visual categorization challenge. arXiv. Available at: https://arxiv.org/abs/1908.02900. [Epub ahead of preprint].
Nanduri, V., Sorokulova, I. B., Samoylov, A. M., Simonian, A. L., Petrenko, V. A., and Vodyanoy, V. (2007). Phage as a molecular recognition element in biosensors immobilized by physical adsorption. Biosens. Bioelectron. 22, 986–992. doi: 10.1016/j.bios.2006.03.025
Neethirajan, S., Kobayashi, I., Nakajima, M., Wu, D., Nandagopal, S., and Lin, F. (2011). Microfluidics for food, agriculture and biosystems industries. Lab Chip 11, 1574–1586. doi: 10.1039/c0lc00230e
Nguyen, H. A., Anh Thi, N. P., Thien Trang, N. P., Ho, T.-T., Trinh, T. N. D., Tran, N. K. S., et al. (2024). Recent advances in biosensors for screening plant pathogens. Anal. Methods 16, 4485–4495. doi: 10.1039/D4AY00766B
Okiro, L. A., Tancos, M. A., Nyanjom, S. G., Smart, C. D., and Parker, M. L. (2019). Comparative evaluation of LAMP, qPCR, conventional PCR, and ELISA to detect Ralstonia solanacearum in Kenyan potato fields. Plant Dis. 103, 959–965. doi: 10.1094/PDIS-03-18-0489-RE
Oliveira, R., Braga, R., and Oliveira, C. (2020). Plante: an intelligent platform for monitoring and controlling of agricultural environments. Proceedings of the 8th International Workshop on ADVANCEs in ICT Infrastructures and Services (ADVANCE 2020). 1–8.
Ortiz, L., Pavan, M., McCarthy, L., Timmons, J., and Densmore, D. M. (2017). Automated robotic liquid handling assembly of modular DNA devices. J. Vis. Exp. 130:e54703. doi: 10.3791/54703
Ozalp, V. C., Bayramoglu, G., Erdem, Z., and Arica, M. Y. (2015). Pathogen detection in complex samples by quartz crystal microbalance sensor coupled to aptamer functionalized core-shell type magnetic separation. Anal. Chim. Acta 853, 533–540. doi: 10.1016/j.aca.2014.10.010
Pan, S., and Arnold, M. A. (1996). Selectivity enhancement for glutamate with a nafion/glutamate oxidase biosensor. Talanta 43, 1157–1162. doi: 10.1016/0039-9140(95)01854-9
Parida, M., Sannarangaiah, S., Dash, P. K., Rao, P. V., and Morita, K. (2008). Loop mediated isothermal amplification (LAMP): a new generation of innovative gene amplification technique; perspectives in clinical diagnosis of infectious diseases. Rev. Med. Virol. 18, 407–421. doi: 10.1002/rmv.593
Parihar, A., Choudhary, N. K., Sharma, P., and Khan, R. (2023). MXene-based aptasensor for the detection of aflatoxin in food and agricultural products. Environ. Pollut. 316:120695. doi: 10.1016/j.envpol.2022.120695
Parihar, A., and Khan, R. (2024). Yttrium functionalized reduced graphene oxide nanocomposite-based aptasensor for ultrasensitive detection of a breast cancer biomarker. ACS Appl. Nano Mater. 7, 18207–18218. doi: 10.1021/acsanm.3c03234
Parihar, A., Singhal, A., Kumar, N., Khan, R., Khan, M. A., and Srivastava, A. K. (2022). Next-generation intelligent MXene-based electrochemical aptasensors for point-of-care cancer diagnostics. Nanomicro Lett. 14:100. doi: 10.1007/s40820-022-00845-1
Parkula, V., Berto, M., Diacci, C., Patrahau, B., Di Lauro, M., Kovtun, A., et al. (2020). Harnessing selectivity and sensitivity in electronic biosensing: a novel lab-on-chip multigate organic transistor. Anal. Chem. 92, 9330–9337. doi: 10.1021/acs.analchem.0c01655
Pascual, R. M., Canonigo, S. G., Cruz, L. R., Roque, M., and Nobleza, J. (2020). Development of mobile application for automatic identification of biotic diseases in Oryza sativa using image processing techniques. Proceedings of the 2020 International Symposium on Computer, Consumer and Control (IS3C). 480–483.
Patil, B. L., Legg, J. P., Kanju, E., and Fauquet, C. M. (2015). Cassava brown streak disease: a threat to food security in Africa. J. Gen. Virol. 96, 956–968. doi: 10.1099/vir.0.000014
Paul, R., Ostermann, E., Chen, Y., Saville, A. C., Yang, Y., Gu, Z., et al. (2021). Integrated microneedle-smartphone nucleic acid amplification platform for in-field diagnosis of plant diseases. Biosens. Bioelectron. 187:113312. doi: 10.1016/j.bios.2021.113312
Perdikaris, A., Vassilakos, N., Yiakoumettis, I., Kektsidou, O., and Kintzios, S. (2011). Development of a portable, high throughput biosensor system for rapid plant virus detection. J. Virol. Methods 177, 94–99. doi: 10.1016/j.jviromet.2011.06.024
Pham, D., Sivalingam, V., Tang, H. M., Montgomery, J. M., Chen, S. C., and Halliday, C. L. (2024). Molecular diagnostics for invasive fungal diseases: current and future approaches. J. Fungi 10:447. doi: 10.3390/jof10070447
Player, R., Verratti, K., Staab, A., Bradburne, C., Grady, S., Goodwin, B., et al. (2020). Comparison of the performance of an amplicon sequencing assay based on Oxford Nanopore technology to real-time PCR assays for detecting bacterial biodefense pathogens. BMC Genomics 21:166. doi: 10.1186/s12864-020-6557-5
Possamai, T., and Wiedemann-Merdinoglu, S. (2022). Phenotyping for QTL identification: a case study of resistance to Plasmopara viticola and Erysiphe necator in grapevine. Front. Plant Sci. 13:930954. doi: 10.3389/fpls.2022.930954
Praneeth, M. (2023). Perceived effectiveness of the mobile applications in agriculture in terms of availability, acceptance, usage and satisfaction. Asian J. Agric. Ext. Econ. Sociol. 41, 19–28. doi: 10.9734/ajaees/2023/v41i112257
Prasad, D., Mani, N. K., and Pandey, D. M. (2022). CRISPR/Cas technology: opportunities for phytopathogenic viruses detection. J. Biotechnol. 360, 211–217. doi: 10.1016/j.jbiotec.2022.11.010
Radhey, N. S., Chandra, C. M., Abhiram, V., Humesh, R., Kumar, M., and Kumar, S. (2024). A comparative analysis of grape plant leaf disease detection-methods and challenges. Proceedings of the 2024 5th International Conference for Emerging Technology (INCET). 1–6
Rafidah, A. R., Faridah, S., Shahrul, A. A., Mazidah, M., and Zamri, I. (2016). Chronoamperometry measurement for rapid cucumber mosaic virus detection in plants. Procedia Chem. 20, 25–28. doi: 10.1016/j.proche.2016.07.003
Raguraman, P., Meghana, A., Navya, Y., Karishma, S., and Iswarya, S. (2021). Color detection of RGB images using Python and OpenCv. Int. J. Sci. Res. Comput. Sci. Eng. Inform. 7, 109–112. doi: 10.32628/cseit217119
Rajwade, J. M., Chikte, R. G., and Paknikar, K. M. (2020). Nanomaterials: new weapons in a crusade against phytopathogens. Appl. Microbiol. Biotechnol. 104, 1437–1461. doi: 10.1007/s00253-019-10334-y
Ray, M. K., Mishra, A. K., Mohanta, Y. K., Mahanta, S., Chakrabartty, I., Kungwani, N. A., et al. (1856). Nanotechnology as a promising tool against phytopathogens: a futuristic approach to agriculture. Agriculture 2023:13. doi: 10.3390/agriculture13091856
Razo, S. C., Panferov, V. G., Safenkova, I. V., Varitsev, Y. A., Zherdev, A. V., and Dzantiev, B. B. (2018). Double-enhanced lateral flow immunoassay for potato virus X based on a combination of magnetic and gold nanoparticles. Anal. Chim. Acta 1007, 50–60. doi: 10.1016/j.aca.2017.12.023
Restif, O., and Grenfell, B. T. (2006). Integrating life history and cross-immunity into the evolutionary dynamics of pathogens. Proc. Biol. Sci. 273, 409–416. doi: 10.1098/rspb.2005.3335
Ricciardi, C., Canavese, G., Castagna, R., Digregorio, G., Ferrante, I., Marasso, S. L., et al. (2010). Online portable microcantilever biosensors for Salmonella enterica serotype Enteritidis detection. Food Bioprocess Technol. 3, 956–960. doi: 10.1007/s11947-010-0362-0
Ristaino, J. B., Saville, A. C., Paul, R., Cooper, D. C., and Wei, Q. (2020). Detection of Phytophthora infestans by loop-mediated isothermal amplification, real-time LAMP, and droplet digital PCR. Plant Dis. 104, 708–716. doi: 10.1094/PDIS-06-19-1186-RE
Roda, A., Cevenini, L., Michelini, E., and Branchini, B. R. (2011). A portable bioluminescence engineered cell-based biosensor for on-site applications. Biosens. Bioelectron. 26, 3647–3653. doi: 10.1016/j.bios.2011.02.022
Rodriguez-Moreno, L., Pineda, M., Soukupova, J., Macho, A. P., Beuzon, C. R., Baron, M., et al. (2008). Early detection of bean infection by Pseudomonas syringae in asymptomatic leaf areas using chlorophyll fluorescence imaging. Photosynth. Res. 96, 27–35. doi: 10.1007/s11120-007-9278-6
Russell, P. F., McOwen, N., Bohannon, S., Amato, M. A., and Bohannon, R. (2015). Rapid on-site detection of the huanglongbing/citrus greening causal agent “Candidatus Liberibacter asiaticus” by Amplify RP, a novel rapid isothermal nucleic acid amplification platform. Acta Hortic. 1065, 905–912. doi: 10.17660/ActaHortic.2015.1065.112
Ryo, K., Nadine Yumi, K., Adam Isamu, M., Anne, M. A., Mark, A. S., Caitilyn, A., et al. (2009). Engineering a real-time disposable platform for discrimination of sub-populations of Ralstonia solanacearum. Proceedings of the 2009. June 21–24, 2009: Reno, Nevada.
Safavieh, M., Ahmed, M. U., Sokullu, E., Ng, A., Braescu, L., and Zourob, M. (2014). A simple cassette as point-of-care diagnostic device for naked-eye colorimetric bacteria detection. Analyst 139, 482–487. doi: 10.1039/c3an01859h
Samal, I., Bhoi, T. K., Pradhan, A. K., and Mahanta, D. K. (2023). Plantix app: a success story of artificial intelligence in plant protection. Van Sangyan, 10, 24–44.
Sarker, B. K., Shrestha, R., Singh, K. M., Lombardi, J., An, R., Islam, A., et al. (2023). Label-free neuropeptide detection beyond the Debye length limit. ACS Nano 17, 20968–20978. doi: 10.1021/acsnano.3c02537
Savary, S., and Willocquet, L. (2020). Modeling the impact of crop diseases on global food security. Annu. Rev. Phytopathol. 58, 313–341. doi: 10.1146/annurev-phyto-010820-012856
Sena-Torralba, A., Alvarez-Diduk, R., Parolo, C., Piper, A., and Merkoci, A. (2022). Toward next generation lateral flow assays: integration of nanomaterials. Chem. Rev. 122, 14881–14910. doi: 10.1021/acs.chemrev.1c01012
Siddiqua, A., Kabir, M. A., Ferdous, T., Ali, I. B., and Weston, L. A. (1869). Evaluating plant disease detection mobile applications: quality and limitations. Agronomy 12:12. doi: 10.3390/agronomy12081869
Siegieda, D. G., Panek, J., and Frąc, M. (2021). “Shining a LAMP” (Loop-Mediated Isothermal Amplification) on the molecular detection of phytopathogens Phytophthora spp. and Phytophthora cactorum in strawberry fields. Pathogens 10:1453. doi: 10.3390/pathogens10111453
Siskandar, R., Fadhil, M. A., Kusumah, B. R., Irmansyah, I., and Irzaman, I. (2020). Internet of Things: automatic plant watering system using android. J. Tek. Pertanian Lampung 9, 297–310. doi: 10.23960/jtep-l.v9i4.297-310
Snodgrass, R., Gardner, A., Jiang, L., Fu, C., Cesarman, E., and Erickson, D. (2016). KS-detect-validation of solar thermal PCR for the diagnosis of Kaposi’s sarcoma using pseudo-biopsy samples. PLoS One 11:e0147636. doi: 10.1371/journal.pone.0147636
Song, X., and Knotts, M. (2008). Time-resolved luminescent lateral flow assay technology. Anal. Chim. Acta 626, 186–192. doi: 10.1016/j.aca.2008.08.006
Srinivasan, B., and Tung, S. (2015). Development and applications of portable biosensors. J. Lab. Autom. 20, 365–389. doi: 10.1177/2211068215581349
Ssengo, J., Wasswa, P., Mukasa, S., Okiror, A., and Kyamanywa, S. (2020). Portable PCR field-based detection of sweetpotato viruses. Afr. Crop. Sci. J. 28, 363–374. doi: 10.4314/acsj.v28i3.3
Studholme, D. J., Glover, R. H., and Boonham, N. (2011). Application of high-throughput DNA sequencing in phytopathology. Annu. Rev. Phytopathol. 49, 87–105. doi: 10.1146/annurev-phyto-072910-095408
Terentev, A., and Dolzhenko, V. (2023). Can metabolomic approaches become a tool for improving early plant disease detection and diagnosis with modern remote sensing methods? A review. Sensors 23:5366. doi: 10.3390/s23125366
The AgroScout. (2024). Available at: https://agro-scout.com/. (Accessed October 19, 2024)
Thekisoe, O. M., Bazie, R. S., Coronel-Servian, A. M., Sugimoto, C., Kawazu, S., and Inoue, N. (2009). Stability of loop-mediated isothermal amplification (LAMP) reagents and its amplification efficiency on crude trypanosome DNA templates. J. Vet. Med. Sci. 71, 471–475. doi: 10.1292/jvms.71.471
Trilles, S., Torres-Sospedra, J., Belmonte, Ó., Zarazaga-Soria, F. J., González-Pérez, A., and Huerta, J. (2020). Development of an open sensorized platform in a smart agriculture context: a vineyard support system for monitoring mildew disease. Sustain. Comput.: Inform. Syst. 28:100309. doi: 10.1016/j.suscom.2019.01.011
Uddin, M. A., Mansour, A., Jeune, D. L., and Aggoune, E. H. M. (2017). Agriculture Internet of Things: AG-IoT. Proceedings of the 2017 27th International Telecommunication Networks and Applications Conference (ITNAC). 1–6.
Umesha, S., and Avinash, P. (2015). Multiplex PCR for simultaneous identification of Ralstonia solanacearum and Xanthomonas perforans. 3 Biotech 5, 245–252. doi: 10.1007/s13205-014-0223-z
Venbrux, M., Crauwels, S., and Rediers, H. (2023). Current and emerging trends in techniques for plant pathogen detection. Front. Plant Sci. 14:1120968. doi: 10.3389/fpls.2023.1120968
Wang, Y., Knoll, W., and Dostalek, J. (2012). Bacterial pathogen surface plasmon resonance biosensor advanced by long range surface plasmons and magnetic nanoparticle assays. Anal. Chem. 84, 8345–8350. doi: 10.1021/ac301904x
Wang, Z., Qiao, X., Wang, Y., Yu, H., and Mu, C. (2024). IoT-based system of prevention and control for crop diseases and insect pests. Front. Plant Sci. 15:1323074. doi: 10.3389/fpls.2024.1323074
Wang, T. M., and Yang, J. T. (2019). Visual DNA diagnosis of tomato yellow leaf curl virus with integrated recombinase polymerase amplification and a gold-nanoparticle probe. Sci. Rep. 9:15146. doi: 10.1038/s41598-019-51650-7
Wright, D., Hammond, N., Thomas, G., MacLeod, B., and Abbott, L. K. (2018). The provision of pest and disease information using information communication tools (ICT); an Australian example. Crop Prot. 103, 20–29. doi: 10.1016/j.cropro.2017.08.023
Wu, K., Chugh, V. K., di Girolamo, A., Liu, J., Saha, R., Su, D., et al. (2021). A portable magnetic particle spectrometer for future rapid and wash-free bioassays. ACS Appl. Mater. Interfaces 13, 7966–7976. doi: 10.1021/acsami.0c21040
Xie, T., Wang, P., Wu, L., Sun, B., Zhao, Q., and Li, G. (2021). A hand-powered microfluidic system for portable and low-waste sample discretization. Lab Chip 21, 3429–3437. doi: 10.1039/d1lc00448d
Xin, J., Harmon, C. L., Vergot, P. III, and Jin, H. (2009). Tracking pests and plant diseases through space and time using geo-tagged digital images. Proceedings of the 7th World Congress on Computers in Agriculture Conference Proceedings. June 22–24, 2009, Reno, Nevada. 711P0409e.
Xu, W., Chen, L., Hu, X., Zhang, L., Huang, D., Li, J., et al. (2024). Botanic signal monitor: advanced wearable sensor for plant health analysis. Adv. Funct. Mater. 34:2410544. doi: 10.1002/adfm.202410544
Yao, J. (2024). “Multi-label deep learning for plant leaf disease classification” in Final thesis (Hobart, TAS, Australia: University of Tasmania).
Ymeti, A., Subramaniam, V., Beumer, T. A., and Kanger, J. S. (2007). An ultrasensitive young interferometer handheld sensor for rapid virus detection. Expert Rev. Med. Devices 4, 447–454. doi: 10.1586/17434440.4.4.447
Zeng, C., Huang, X., Xu, J., Li, G., Ma, J., Ji, H. F., et al. (2013). Rapid and sensitive detection of maize chlorotic mottle virus using surface plasmon resonance-based biosensor. Anal. Biochem. 440, 18–22. doi: 10.1016/j.ab.2013.04.026
Zhan, G., Tian, Y., Wang, F., Chen, X., Guo, J., Jiao, M., et al. (2014). A novel fungal hyperparasite of Puccinia striiformis f. sp. tritici, the causal agent of wheat stripe rust. PLoS One 9:e111484. doi: 10.1371/journal.pone.0111484
Zhang, Y., Guo, Y., Liu, G., Zhou, S., Su, R., Ma, Q., et al. (2024). Portable all-in-one microfluidic system for CRISPR-Cas13a-based fully integrated multiplexed nucleic acid detection. Lab Chip 24, 3367–3376. doi: 10.1039/d4lc00326h
Zhang, D., and Liu, Q. (2016). Biosensors and bioelectronics on smartphone for portable biochemical detection. Biosens. Bioelectron. 75, 273–284. doi: 10.1016/j.bios.2015.08.037
Zhang, Y., Peng, A., Jie, X., Lv, Y., Wang, X., and Tian, Z. (2017). A BODIPY-based fluorescent probe for detection of subnanomolar phosgene with rapid response and high selectivity. ACS Appl. Mater. Interfaces 9, 13920–13927. doi: 10.1021/acsami.7b02013
Zhang, T., Zeng, Q., Ji, F., Wu, H., Ledesma-Amaro, R., Wei, Q., et al. (2023). Precise in-field molecular diagnostics of crop diseases by smartphone-based mutation-resolved pathogenic RNA analysis. Nat. Commun. 14:4327. doi: 10.1038/s41467-023-39952-x
Zhao, F., He, J., Li, X., Bai, Y., Ying, Y., and Ping, J. (2020). Smart plant-wearable biosensor for in-situ pesticide analysis. Biosens. Bioelectron. 170:112636. doi: 10.1016/j.bios.2020.112636
Zhao, C., and Liu, X. (2016). A portable paper-based microfluidic platform for multiplexed electrochemical detection of human immunodeficiency virus and hepatitis C virus antibodies in serum. Biomicrofluidics 10:024119. doi: 10.1063/1.4945311
Zhao, X., Zhai, L., Chen, J., Zhou, Y., Gao, J., Xu, W., et al. (2024). Recent advances in microfluidics for the early detection of plant diseases in vegetables, fruits, and grains caused by bacteria, fungi, and viruses. J. Agric. Food Chem. 72, 15401–15415. doi: 10.1021/acs.jafc.4c00454
Zhou, Y., Zheng, H. Y., Jiang, D. M., Liu, M., Zhang, W., and Yan, J. Y. (2022). A rapid detection of tomato yellow leaf curl virus using recombinase polymerase amplification-lateral flow dipstick assay. Lett. Appl. Microbiol. 74, 640–646. doi: 10.1111/lam.13611
Zhu, H., Fohlerova, Z., Pekarek, J., Basova, E., and Neuzil, P. (2020). Recent advances in lab-on-a-chip technologies for viral diagnosis. Biosens. Bioelectron. 153:112041. doi: 10.1016/j.bios.2020.112041
Keywords: portable diagnostics, point-of-care testing, plant pathogens, portable biosensors, lab-on-a-chip
Citation: Yadav A and Yadav K (2025) Portable solutions for plant pathogen diagnostics: development, usage, and future potential. Front. Microbiol. 16:1516723. doi: 10.3389/fmicb.2025.1516723
Received: 24 October 2024; Accepted: 14 January 2025;
Published: 31 January 2025.
Edited by:
Ravinder Kumar, Indian Agricultural Research Institute (ICAR), IndiaReviewed by:
Florence Lagarde, Université Claude Bernard Lyon 1, FranceCopyright © 2025 Yadav and Yadav. This is an open-access article distributed under the terms of the Creative Commons Attribution License (CC BY). The use, distribution or reproduction in other forums is permitted, provided the original author(s) and the copyright owner(s) are credited and that the original publication in this journal is cited, in accordance with accepted academic practice. No use, distribution or reproduction is permitted which does not comply with these terms.
*Correspondence: Anurag Yadav, YW51cmFneWFkYXYxMjNAZ21haWwuY29t
Disclaimer: All claims expressed in this article are solely those of the authors and do not necessarily represent those of their affiliated organizations, or those of the publisher, the editors and the reviewers. Any product that may be evaluated in this article or claim that may be made by its manufacturer is not guaranteed or endorsed by the publisher.
Research integrity at Frontiers
Learn more about the work of our research integrity team to safeguard the quality of each article we publish.