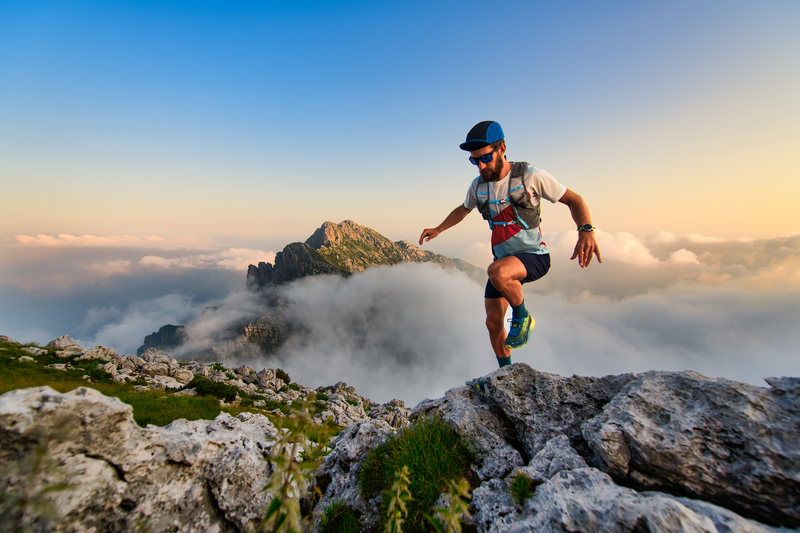
95% of researchers rate our articles as excellent or good
Learn more about the work of our research integrity team to safeguard the quality of each article we publish.
Find out more
REVIEW article
Front. Microbiol.
Sec. Microbiotechnology
Volume 16 - 2025 | doi: 10.3389/fmicb.2025.1507491
The final, formatted version of the article will be published soon.
You have multiple emails registered with Frontiers:
Please enter your email address:
If you already have an account, please login
You don't have a Frontiers account ? You can register here
The demand for early disease detection, treatment monitoring, and personalized medicine is increasing, making it more imperative than ever to create effective, accurate, portable, intelligent, multifunctional diagnostic equipment. Bacteria possess a remarkable perception of their surroundings and have the capacity to adapt by altering the expression of specific genes. Bacteria interact with target substances and produce detectable signals in response to their presence or concentration. This unique property has been harnessed in the development of bacterial biosensors. Due to groundbreaking advancements in synthetic biology, genetic engineering now enables the creation of bacteria tailored with exceptional detecting traits. In addition to meeting a wide range of application needs, this allows quick and precise detection in intricate settings and offers a strong technological basis for early disease diagnosis and treatment monitoring. This article reviews the applications and recent advancements of bacterial biosensors in the medical field and discusses the challenges and obstacles that remain in their research and application.
Keywords: Bacteria, Biosensors, Disease diagnosis, personalized medicine, Real-time monitoring
Received: 07 Oct 2024; Accepted: 24 Mar 2025.
Copyright: © 2025 Liu, Yang, Zhu and Yu. This is an open-access article distributed under the terms of the Creative Commons Attribution License (CC BY). The use, distribution or reproduction in other forums is permitted, provided the original author(s) or licensor are credited and that the original publication in this journal is cited, in accordance with accepted academic practice. No use, distribution or reproduction is permitted which does not comply with these terms.
* Correspondence:
Daojun Yu, Hangzhou First People's Hospital, Hangzhou, 310006, Zhejiang Province, China
Disclaimer: All claims expressed in this article are solely those of the authors and do not necessarily represent those of their affiliated organizations, or those of the publisher, the editors and the reviewers. Any product that may be evaluated in this article or claim that may be made by its manufacturer is not guaranteed or endorsed by the publisher.
Research integrity at Frontiers
Learn more about the work of our research integrity team to safeguard the quality of each article we publish.