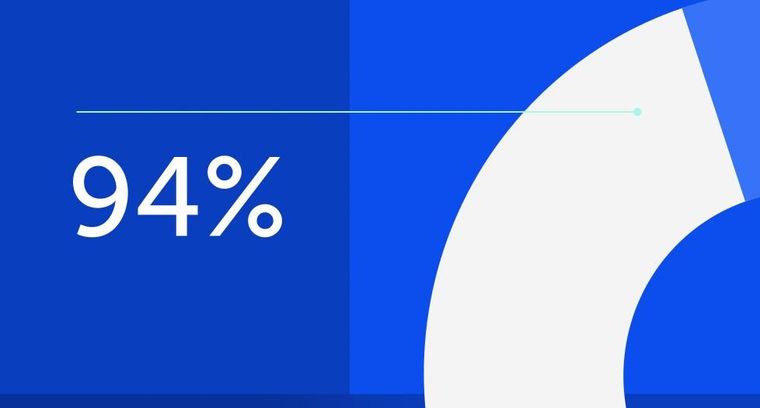
94% of researchers rate our articles as excellent or good
Learn more about the work of our research integrity team to safeguard the quality of each article we publish.
Find out more
REVIEW article
Front. Microbiol., 02 April 2025
Sec. Aquatic Microbiology
Volume 16 - 2025 | https://doi.org/10.3389/fmicb.2025.1504829
This article is part of the Research TopicMitigating Microbial Contamination of Drinking Water SourcesView all 4 articles
Microbial contamination of water sources is a pressing global challenge, disproportionately affecting developing regions with inadequate infrastructure and limited access to safe drinking water. In the Global South, waterborne pathogens such as bacteria, viruses, protozoa, and helminths contribute to diseases like cholera, dysentery, and typhoid fever, resulting in severe public health burdens. Predictive modeling emerges as a pivotal tool in addressing these challenges, offering data-driven insights to anticipate contamination events and optimize mitigation strategies. This review highlights the application of predictive modeling techniques—including machine learning, hydrological simulations, and quantitative microbial risk assessment —to identify contamination hotspots, forecast pathogen dynamics, and inform water resource allocation in the Global South. Predictive models enable targeted actions to improve water safety and lower the prevalence of waterborne diseases by combining environmental, socioeconomic, and climatic factors. Water resources in the Global South are increasingly vulnerability to microbial contamination, and the challenge is exacerbated by rapid urbanization, climate variability, and insufficient sanitation infrastructure. This review underscores the importance of region-specific modeling approaches. Case studies from sub-Saharan Africa and South Asia demonstrated the efficacy of predictive modeling tools in guiding public health actions connected to environmental matrices, from prioritizing water treatment efforts to implementing early-warning systems during extreme weather events. Furthermore, the review explores integrating advanced technologies, such as remote sensing and artificial intelligence, into predictive frameworks, highlighting their potential to improve accuracy and scalability in resource-constrained settings. Increased funding for data collecting, predictive modeling tools, and cross-sectoral cooperation between local communities, non-governmental organizations, and governments are all recommended in the review. Such efforts are critical for developing resilient water systems capable of withstanding environmental stressors and ensuring sustainable access to safe drinking water. By leveraging predictive modeling as a core component of water management strategies, stakeholders can address microbial contamination challenges effectively, safeguard public health, and contribute to achieving the United Nations’ Sustainable Development Goals.
Microbial contamination of water sources is a pressing global issue that poses significant challenges to public health, particularly in the Global South, because their unique socioeconomic and environmental challenges amplify its impact (Erinle et al., 2021; Izah et al., 2023, 2022a,b). High levels of fecal contamination in drinking water, particularly in rural areas of Africa and Southeast Asia, are a significant concern (Ovuru et al., 2023; Sawyer et al., 2023). Inadequate water, sanitation, and hygiene conditions contribute significantly to waterborne diseases, with contaminated water being a key vector. WHO estimates that 5% of all deaths in developing countries stem from water-related diseases, emphasizing the need for effective water quality monitoring and interventions (Prüss-Ustün et al., 2014). The World Health Organization (WHO) estimates that over 2 billion people lack access to safe drinking water, leading to many health risks associated with waterborne pathogens (Ramírez-Castillo et al., 2015). WHO also estimates that 5% of all deaths in developing countries stem from water-related diseases, emphasizing the need for effective water quality monitoring and interventions (Prüss-Ustün et al., 2014).
In parts of the Global South, contaminated water is a major contributor to diseases such as cholera, dysentery, and typhoid fever, which disproportionately affect vulnerable populations in low-income regions (Ramírez-Castillo et al., 2015). The challenges of ensuring safe water supplies in developing countries are exacerbated by inadequate infrastructure, rapid urbanization, and climate change, leading to increased flooding and contamination of water sources (Hering et al., 2013). In many cases, the existing water treatment facilities are outdated or insufficient to handle the growing demand and the complexity of contaminants present in the water supply (Hering et al., 2013). The public health risks associated with microbial contamination are profound (Ben-Eledo et al., 2017; Enaregha et al., 2022; Agedah et al., 2015; Seiyaboh et al., 2020a,b), as contaminated water can lead to severe morbidity and mortality, particularly among children and immunocompromised individuals (Ramírez-Castillo et al., 2015). The disease burden attributable to waterborne pathogens is immense, with millions of cases reported annually, highlighting the urgent need for effective interventions (Ramírez-Castillo et al., 2015). Additionally, the economic implications of waterborne diseases are significant, as they can lead to increased healthcare costs and lost productivity, further straining the limited resources of developing nations (Mekonnen and Hoekstra, 2016).
Many communities in the Global South lack access to improved water sources, and the assumption that these sources present no risk is often misguided (Bain et al., 2014a,b). For instance, studies have shown that even improved water sources can harbor pathogens, particularly in regions with inadequate infrastructure (Poulin et al., 2020; Iyiola et al., 2023a, 2024a,b). This highlights the necessity for comprehensive water safety plans incorporating local knowledge and practices to effectively manage water quality and mitigate risks associated with microbial contamination (Leftwich et al., 2021). Traditional culture-based water testing methods are often impractical in the Global South due to resource constraints and delayed results, underscoring the value of rapid methods like multiplex real-time polymerase chain reaction for timely and accurate pathogen detection (Gemeda et al., 2022; Ogwu and Kosoe, 2024). Socio-economic disparities exacerbate the issue, as many communities lack access to safe water sources, and even “improved” sources may harbor pathogens due to poor infrastructure (Bain et al., 2014b). Comprehensive water safety plans incorporating local knowledge and practices are crucial for managing risks effectively (Leftwich et al., 2021). Environmental factors, including seasonal variations, further complicate microbial contamination dynamics, as seen in studies from Uganda (Sadik et al., 2017; Erhunmwunse et al., 2024). Predictive models must integrate these variables to guide public health strategies and resource allocation. Addressing microbial water contamination in the Global South requires advanced detection technologies, community-driven approaches, and robust public health policies tailored to local contexts to ensure sustainable and impactful solutions.
Predictive modeling emerges as a vital tool in managing water quality, particularly in anticipating contamination events and optimizing mitigation strategies. Predictive modeling involves using mathematical and computational techniques to simulate the behavior of water systems under various conditions, allowing for the identification of potential contamination sources and assessing their impacts (Perelman et al., 2012). This approach is particularly relevant in water quality management, enabling stakeholders to make informed decisions regarding resource allocation and intervention strategies (Perelman et al., 2012). By leveraging predictive modeling, water management authorities can anticipate contamination events, implement timely responses, and allocate resources more effectively, enhancing public health protection (Perelman et al., 2012). The public health risks associated with microbial contamination in water sources are profound (Izah and Ineyougha, 2015; Izah et al., 2021a), as contaminated water can lead to severe morbidity and mortality, particularly among vulnerable populations such as children and immunocompromised individuals (Odiyo et al., 2020). The burden of disease attributable to waterborne pathogens is immense, with millions of cases reported annually, underscoring the urgent need for effective interventions (Prüss-Ustün et al., 2014). Unsafe drinking water is a significant contributor to diarrhea and is responsible for an estimated 10% of global mortality among children under five (Prüss-Ustün et al., 2014). Moreover, the economic implications of waterborne diseases are significant, as they can lead to increased healthcare costs and lost productivity, further straining the limited resources of developing nations (Peletz et al., 2016). The economic burden is compounded by many low-income countries lacking adequate water quality monitoring systems, which can exacerbate the public health crisis (Peletz et al., 2016).
Predictive modeling emerges as a vital tool in managing water quality, particularly in anticipating contamination events and optimizing mitigation strategies. This approach employs mathematical and computational techniques to simulate the behavior of water systems under various conditions, allowing for the identification of potential contamination sources and the assessment of their impacts (Scanlon M. et al., 2022; Bentham and Whiley, 2018). For example, quantitative microbial risk assessment (QMRA) has effectively evaluated the risks associated with microbial contamination in drinking water, providing valuable insights into potential health impacts (Bentham and Whiley, 2018; Abuzerr, 2024). By leveraging predictive modeling, water management authorities can anticipate contamination events, implement timely responses, and allocate resources more effectively, enhancing public health protection (Scanlon B. R. et al., 2022; Bentham and Whiley, 2018). This proactive approach is particularly relevant in regions where waterborne diseases are prevalent, as it enables stakeholders to make informed decisions regarding resource allocation and intervention strategies (Ahmed et al., 2020). Furthermore, the integration of predictive modeling with real-time data collection can significantly improve the responsiveness of water quality management systems. For instance, the use of rapid indicators for microbial contamination can facilitate quicker assessments of water safety, allowing for immediate public health interventions (Colford et al., 2012). This is crucial in areas prone to extreme weather events, exacerbating water quality issues and increasing the risk of waterborne disease outbreaks (Cann et al., 2012). The combination of predictive modeling and real-time monitoring enhances the capacity to manage water quality and fosters a more resilient public health infrastructure capable of addressing the challenges posed by microbial contamination (Cann et al., 2012; Bentham and Whiley, 2018).
This paper aims to explore the application of predictive modeling as a tool to mitigate microbial contamination in water sources, with a specific focus on solutions tailored for developing countries facing resource constraints. Given the unique challenges faced by these regions, it is imperative to develop cost-effective and sustainable modeling approaches that can inform water management practices and enhance the resilience of water supply systems (Mekonnen and Hoekstra, 2016). The scope of this review involves the evaluation of existing modeling frameworks, identifying gaps in current practices, and formulating recommendations for implementing predictive modeling in the context of microbial water contamination.
Microbial contaminants in water sources represent a global public health concern, particularly in developing countries where sanitation and hygiene practices are often inadequate. A complex interplay of environmental, socio-economic, and health factors shapes microbial contaminants in Global South water sources. This region faces significant water quality challenges, directly impacting public health. These contaminants include a variety of microorganisms, such as bacteria, viruses, protozoa, and other pathogens, that can lead to serious health issues when ingested through contaminated water (Table 1). The primary types of microbial contaminants found in water sources include pathogenic bacteria like Escherichia coli, viruses such as norovirus, and protozoa like Giardia and Cryptosporidium (Murphy et al., 2015; Gwimbi et al., 2019). Each of these pathogens has distinct sources and modes of transmission, contributing to the overall burden of waterborne diseases. One of the primary concerns is the prevalence of fecal contamination in drinking water sources. Studies have shown that even improved water sources, such as protected wells and piped supplies, often harbor significant microbial pathogens. Many supposedly improved sources are frequently contaminated, particularly in rural areas of Africa and Southeast Asia, where the risk of waterborne diseases is disproportionately high (Bain et al., 2014a,b). This situation is exacerbated by inadequate sanitation infrastructure and poor hygiene practices, prevalent in many communities across the Global South (Bain et al., 2014b). The health implications of microbial contamination are severe, particularly for vulnerable populations such as children. Waterborne diseases, including cholera, dysentery, and typhoid fever, are major contributors to morbidity and mortality in these regions. For example, Luby et al. (2015) found a direct correlation between microbial contamination in drinking water and the incidence of diarrhea among children in Bangladesh, emphasizing the urgent need for adequate water quality management. Furthermore, as reported by Kumar et al. (2012), the presence of antibiotic-resistant bacteria in water sources raises additional concerns about the effectiveness of treatment options for infections stemming from contaminated water.
Table 1. Microbial contaminants of water sources in the global south that are of global public health concern.
Although the sources of these microbial contaminants in Global South water are multifaceted, the key contributors include sewage discharge, agricultural runoff, and poor sanitation practices. Sewage often introduces a variety of pathogens into water bodies, particularly in areas lacking adequate wastewater treatment facilities (Sibiya and Gumbo, 2013; T’Seole et al., 2022). Agricultural runoff, which may contain fertilizers and animal waste, can also contaminate surface water sources, exacerbating the risk of waterborne diseases (Murphy et al., 2015; Gwimbi et al., 2019). Furthermore, inadequate sanitation infrastructure, particularly in rural and impoverished urban areas, creates conditions conducive to spreading pathogens through water sources (T’Seole et al., 2022; Medeiros and Ferreira, 2020). The interrelationship between these sources and the resultant microbial contamination underscores the need for comprehensive water management strategies addressing water quality and sanitation.
The health impacts of microbial contamination in water are profound, particularly in developing countries with limited access to clean water. Waterborne diseases such as cholera, typhoid fever, and dysentery are prevalent in these regions, leading to significant morbidity and mortality (Jutla et al., 2015; Prüss-Ustün et al., 2014). For instance, cholera outbreaks have been linked to contaminated drinking water sources, with studies indicating that improved sanitation and water purification can drastically reduce the incidence of such diseases (Jutla et al., 2015; Armah et al., 2018). The World Health Organization estimates that inadequate water, sanitation, and hygiene (WASH) contribute to approximately 10% of the global disease burden, with diarrheal diseases alone accounting for a substantial portion of this statistic (McGinnis et al., 2019). In terms of mortality, it is estimated that around 2.2 million people die each year from diarrheal diseases linked to unsafe drinking water and poor sanitation (Prüss-Ustün et al., 2014). Statistics on morbidity and mortality associated with contaminated water highlight the urgent need for improved water quality management. For example, in sub-Saharan Africa, it is estimated that over 300,000 children under five die each year from diarrheal diseases linked to unsafe water and inadequate sanitation (Armah et al., 2018). Moreover, the economic burden of waterborne diseases is significant, with healthcare costs and lost productivity due to illness placing a strain on already limited resources in developing nations (Minh and Hùng, 2011; Patel et al., 2013). The interplay between health impacts and economic factors emphasizes the necessity for investment in water and sanitation infrastructure to improve public health outcomes.
In addition to health risks, the variability of microbial contamination due to environmental factors poses further challenges. Seasonal changes can significantly influence pathogen concentrations in water sources, as demonstrated by Sadik et al. (2017), who noted fluctuations in pathogen levels in Kampala, Uganda, during different seasons. This variability necessitates robust monitoring and modeling efforts to effectively predict contamination events and inform public health responses (Sokolova et al., 2012; Wu H. et al., 2021). Moreover, the Global South’s socio-economic context complicates the water quality issue. Many communities rely on untreated surface water or poorly maintained water supply systems, susceptible to contamination from agricultural runoff, industrial discharges, and inadequate waste management practices (Ahmed et al., 2020). The reliance on boiling water as a treatment method, as observed in Sikkim, India, indicates a common practice among communities to mitigate health risks. However, eliminating all pathogens may not always be sufficient (Singh et al., 2019). It is essential to incorporate sophisticated monitoring methods, including microbial source tracking and hydrodynamic modeling, to comprehend and treat the origins of contamination (Sokolova et al., 2012; Wu J. et al., 2021). These methods can improve water safety and public health outcomes in the Global South by assisting in identifying sources of contamination and providing guidance for focused remedies.
Despite recognizing these issues in the Global South, current challenges in controlling waterborne pathogens remain significant. Inadequate water infrastructure and sanitation facilities are prevalent in many regions, particularly in low-income countries where funding for such projects is often insufficient (T’Seole et al., 2022; Minh and Hùng, 2011). This lack of infrastructure not only hampers access to clean water but also increases the risk of contamination from various sources, including untreated sewage and agricultural runoff (Sibiya and Gumbo, 2013; T’Seole et al., 2022). Furthermore, limited access to real-time water quality monitoring and testing exacerbates the problem, as communities may remain unaware of harmful pathogens in their water supply (Nefale et al., 2017). The absence of effective surveillance systems makes it challenging to implement timely interventions to mitigate the risks associated with microbial contamination. Improving water quality and sanitation must also consider the socio-economic factors influencing access to these essential services. Communities with low socio-economic status often face more significant challenges in securing safe drinking water and adequate sanitation facilities, affecting their overall health and well-being. The relationship between poverty, water insecurity, and health outcomes is well-documented, indicating that addressing these disparities is crucial for reducing the burden of waterborne diseases. Additionally, community participation in sanitation initiatives has been shown to enhance the effectiveness of interventions, as local involvement can lead to more sustainable practices and better maintenance of facilities (Olugbamila et al., 2020; O’Reilly, 2015). Some of the world’s most polluted rivers include the Amazon, Ganges, Yangtze, and Niger Rivers, highlighting the shared challenges they face due to industrial, agricultural, and domestic pollution (Fearnside, 2016; Central Pollution Control Board (CPCB)., 2019; Ma et al., 2022). The Amazon River is significantly affected by deforestation and mining, while the Ganges suffers from untreated sewage and industrial discharge, prompting initiatives like the “Namami Gange” program aimed at rejuvenation (Ministry of Water Resources., 2019). The Yangtze River, impacted by industrial waste and ecological changes from the Three Gorges Dam, has seen the implementation of stringent regulations to protect its waters (Ma et al., 2022). Meanwhile, the Niger River faces pollution from oil spills and agricultural runoff, leading to regional cooperation efforts for sustainable practices (Ifelebuegu et al., 2017). This underscores the importance of cross-regional learning, suggesting that successful strategies from one river can inform management practices in others, thereby enhancing the overall understanding of global water pollution and its mitigation.
Predictive modeling in environmental science is a critical tool for understanding and managing microbial water contaminants. These models are designed to forecast the presence and concentration of microbial pathogens in water systems, thereby assisting in public health protection and environmental management (Table 2). The primary purpose of predictive models is to provide insights into the dynamics of microbial contamination, enabling stakeholders to make informed decisions regarding water quality management and risk assessment. By utilizing various data inputs, including environmental variables, land use patterns, and historical contamination data, predictive models can simulate potential contamination scenarios and assess the effectiveness of intervention strategies (Saeidi et al., 2018a; Wu J. et al., 2021).
Several types of predictive models are employed in the context of microbial water contaminants, each with distinct methodologies and applications. Statistical models, such as regression analysis, are commonly used to identify relationships between microbial indicators and environmental factors. For instance, it demonstrated that incorporating land use categories and chemical tracers significantly improved model performance for predicting fecal indicators in urban environments, achieving R2 values greater than 0.69 (Saeidi et al., 2018b). These statistical approaches allow for quantifying the impact of various environmental factors on microbial water quality, providing a basis for targeted interventions. Machine learning techniques have also gained prominence in predictive modeling for microbial contamination. Wu J. et al. (2021) utilized machine learning algorithms to track significant sources of water contamination, highlighting the importance of hydrologic features and land cover in predicting microbial sources. This approach allows for the integration of large datasets and complex interactions between variables, enhancing the accuracy of predictions. Furthermore, machine learning models can adapt to new data, improving their predictive capabilities and providing real-time insights into water quality dynamics.
Hydrological models represent another category of predictive tools for assessing microbial contamination in water bodies. These models simulate the movement of water through the environment, accounting for factors such as precipitation, runoff, and groundwater flow. ’s study on groundwater contamination risks from pit latrines illustrates how hydrological models can be employed to predict microbial contamination based on various environmental parameters, including soil type and groundwater table variations (Hinton, 2023). Stakeholders can better manage water resources and mitigate contamination risks by understanding the hydrological processes that contribute to microbial transport. Developing all-encompassing strategies to combat microbiological water contamination requires integrating different modeling approaches. For instance, combining statistical models with machine learning techniques can enhance predictive accuracy by leveraging the strengths of both methodologies. Additionally, incorporating hydrological modeling into predictive frameworks allows for a more holistic understanding of how microbial contaminants move through ecosystems, ultimately informing management practices to reduce public health risks. The significance of predictive modeling extends beyond academic research; it has practical implications for water quality management and public health protection. For example, predictive models can inform the design of monitoring programs by identifying critical times and locations for sampling, thereby optimizing resource allocation. Moreover, these models can assist in evaluating the effectiveness of interventions, such as implementing best management practices in agricultural settings or establishing buffer zones around water bodies (Holvoet et al., 2012).
Furthermore, predictive modeling is crucial in risk assessment related to microbial water contamination. These models can guide regulatory decisions and public health interventions by estimating the likelihood of contamination events and their potential health impacts. For instance, Atta et al. (2019) highlighted the relationship between drinking water contamination and gastrointestinal illnesses, underscoring the need for practical predictive tools to prevent outbreaks. By quantifying the risks associated with different contamination scenarios, stakeholders can prioritize actions to safeguard public health. In addition to traditional modeling approaches, technological advancements have facilitated the development of innovative predictive tools. For example, remote sensing and geographic information systems (GIS) have enhanced the ability to monitor environmental determinants of microbial contamination in recreational waters. Kotchi et al. (2015) emphasized the importance of integrating Earth observation systems to detect microbial contamination promptly, which is critical for protecting public health during recreational activities. These technological advancements enable more efficient data collection and analysis, ultimately improving the reliability of predictive models. Moreover, the incorporation of climate data into predictive models has become increasingly relevant in the context of microbial water quality. Xu et al. (2019) demonstrated that climate and land use factors significantly influence bacterial levels in stormwater, highlighting the need for models that account for changing environmental conditions. As climate change continues to impact water quality, predictive modeling will be essential for understanding and mitigating the effects of these changes on microbial contamination.
Figure 1 illustrates a conceptual framework for predicting water quality and consumption, leveraging machine learning (ML) and deep learning (DL) models by Rustam et al. (2022). The process typically begins with dataset collection from two sources: a water quality dataset (Kaggle) and a water demand dataset (GitHub). These datasets are split into training (80%) and testing (20%) subsets to build and validate predictive models. The ML techniques employed include Decision Trees (DT), Extra Trees (ET), Random Forests (RF), Support Vector Machines (SVM), Logistic Regression (LR), and Adaptive Boosting (ADA). On the other hand, the DL methods include Convolutional Neural Networks (CNN), Long Short-Term Memory (LSTM), Gated Recurrent Units (GRU), and Artificial Neural Networks (ANN). The trained models are evaluated for water quality prediction using metrics like Accuracy, Precision, Recall, and F1 Score. Simultaneously, water consumption forecasting is assessed using Mean Absolute Error (MAE), Mean Squared Error (MSE), Root Mean Squared Error (RMSE), and R2 Score. This approach effectively monitors water resources, providing actionable insights into quality and consumption patterns. Using advanced algorithms enables the framework to enhance prediction accuracy, facilitating data-driven decision-making for sustainable water resource management. Another relevant framework is a bagging ensemble framework integrating multiple machine learning models for predictive analytics (Figure 2; Chou et al., 2019). This model is essential and suitable for the Global South because it can handle missing data. The process begins with a database where data is bootstrapped to create diverse training datasets. These datasets are fed into different predictive models, including Artificial Neural Networks (ANN), Support Vector Regression (SVR), Linear Regression (LR), and Classification and Regression Trees (CART). Combining these models, the bagging ensemble leverages their strengths and reduces prediction variance. The final predictive value is obtained through a voting mechanism aggregating all models’ outputs, ensuring a robust and accurate prediction. From an application standpoint, this approach is highly beneficial in scenarios requiring high precision, such as water quality monitoring, environmental risk assessments, or industrial process optimization. The ensemble model’s capability to handle diverse data types and mitigate overfitting enhances its adaptability to real-world problems, particularly in dynamic systems with complex variables.
Figure 1. Framework for predicting water quality and consumption using machine learning and deep learning models. Source: Rustam et al. (2022).
Figure 2. Bagging ensemble model for enhanced predictive analytics of microbes in water. Source: Chou et al. (2019).
Diverse LSTM models are widely used for microbial prediction because they process sequential data and capture temporal dependencies. Standard LSTMs predict microbial growth based on time-series inputs like pH, temperature, and nutrient levels, while Bidirectional LSTMs (BiLSTMs) enhance accuracy by analyzing data in forward and backward directions. Stacked LSTMs, with multiple layers, learn complex patterns for dynamic environments or multi-stage processes. Models with attention mechanisms prioritize critical input features, improving predictions under varied conditions. Convolutional LSTMs (ConvLSTMs) handle spatial-temporal data, such as microbial biofilm growth, and hybrid LSTMs combine techniques like SVMs for enhanced accuracy. These models support critical applications in food safety, industrial optimization, and environmental health management. Also, the innovative use of the Hyperconic Multilayer Perceptron (HC-MLP) for predicting microbial growth under varying conditions, such as pH levels and nutrient concentrations, has been experimented with by Murrieta-Dueñas et al. (2021). By leveraging experimental data from Pseudomonas aeruginosa, the HC-MLP offers a novel approach to microbial growth modeling. Its ability to create complex non-linear decision boundaries enhances the accuracy of predictions compared to traditional models. The method supports reduced experimental costs, optimizes the design of bioreactors, and advances real-time control strategies for biological processes, making it particularly valuable for applications in biotechnology, food safety, and pharmaceutical industries? The application of predictive modeling in microbial water quality assessment is not without challenges. One significant issue is the variability in microbial indicators and their relationship with environmental factors. For instance, the presence of fecal coliforms as an indicator of microbial contamination may not always correlate with pathogenic microorganisms, leading to potential misinterpretations of water quality (Sarker et al., 2016). Therefore, ongoing research is necessary to refine predictive models and improve their accuracy in assessing microbial risks.
Developing predictive models for water contamination involves a systematic approach encompassing several critical steps, including data collection and analysis, model design, calibration, and validation (Figure 3). The framework provides a systematic approach to predicting water quality by integrating robust data preprocessing, advanced modeling techniques, and comprehensive evaluation metrics. By incorporating outlier detection and z-score normalization, the framework ensures data integrity and readiness for analysis. Regression models for Water Quality Index prediction and classification models for water quality classification allow for tailored analysis of continuous and categorical outcomes (Table 3). Note that water quality classification thresholds often vary based on regional or institutional standards, though the provided ranges are widely recognized in environmental assessments. However, this classification system is a valuable tool for policymakers and environmental managers to identify water quality issues and prioritize appropriate remedial actions. Additionally, adapting these thresholds to reflect specific socio-economic and ecological contexts is essential for addressing unique regional challenges effectively. The Water Quality Index integrates multiple parameters to evaluate water quality comprehensively. Physical parameters, such as temperature, turbidity, and total dissolved solids, assess water clarity and usability. Chemical parameters, including pH, dissolved oxygen, biological oxygen demand, and contaminants like nitrates and phosphates, measure chemical impacts on ecosystems and health. Microbiological parameters, such as fecal and total coliform, indicate microbial contamination and disease risks. Heavy metals like lead, arsenic, and mercury assess toxicity, while nutrient levels (ammonia, nitrites, phosphorus) highlight risks of eutrophication. Toxic substances, including pesticides and PCBs, trace industrial and agricultural pollutants, while indicators like hardness, alkalinity, and chlorides provide general water quality insights for various uses. Incorporating metrics such as MAE, RMSE, Accuracy, and F1 Score ensures the reliability and validity of the predictions. This structured methodology is particularly valuable in addressing the complexities of water quality assessment, offering insights that can inform public health interventions and resource management. This process is essential for ensuring that the models accurately reflect the complexities of hydrological systems and can effectively predict water quality outcomes.
Figure 3. Standard framework for water quality index prediction- data preprocessing, methodology, and evaluation. Source: Ahmed et al. (2019).
The first step in developing predictive models is comprehensive data collection, which involves gathering various environmental, meteorological, and hydrological data types. This data is the foundation for model development and can include temperature, precipitation, land use, and water quality indicators. For instance, the Soil Water Assessment Tool (SWAT) utilizes extensive datasets related to weather, soil properties, topography, and vegetation to simulate water quantity and quality in complex watersheds (Bacu et al., 2011; Iyiola et al., 2023b). Similarly, machine learning models for predicting Escherichia coli loads integrate hydrometeorological data alongside animal density and grazing patterns, demonstrating the importance of diverse datasets in enhancing predictive accuracy (Abimbola et al., 2020). In addition to raw data, the analysis phase is crucial for understanding the relationships between different variables and identifying patterns that can inform model development. Statistical methods and exploratory data analysis techniques are often employed to discern correlations and trends within the data. For example, regression methods have been utilized to predict E. coli levels based on various environmental factors, highlighting the role of statistical analysis in shaping predictive models (Abimbola et al., 2020). Furthermore, the integration of machine learning techniques allows for the identification of complex non-linear relationships that traditional statistical methods may overlook, thus improving the robustness of predictions (Ahmed et al., 2019).
Once the data has been collected and analyzed, the next step involves designing the predictive model. This phase includes selecting the appropriate modeling framework and algorithms that align with the study’s specific objectives. For instance, hydrodynamic and water quality models such as EPANET and SWAT are commonly used in water contamination studies due to their ability to effectively simulate hydraulic and water quality processes (Cervantes, 2023; Bacu et al., 2011). EPANET has been enhanced with various extensions to improve its capabilities in modeling water quality under different conditions, including pressure-dependent demand scenarios (Seyoum et al., 2013). Calibration is a critical step in model development, wherein the model parameters are adjusted to ensure that the model outputs align with observed data. This process often involves iterative testing and refinement, utilizing historical data to fine-tune the model’s predictive capabilities. For example, the calibration of the SWAT model has been extensively documented, with studies demonstrating its effectiveness in simulating nutrient runoff and sediment yields in various watersheds (Ali et al., 2020). Similarly, the calibration of EPANET models is essential for accurately predicting water quality outcomes, particularly in large distribution networks where computational efficiency is paramount (Davis et al., 2018). Validation follows calibration and assesses the model’s predictive performance against independent datasets. This step is crucial for establishing the model’s credibility and ensuring it can reliably predict future water quality scenarios. Various validation techniques, including cross-validation and hold-out testing, evaluate model accuracy and generalizability (Towett et al., 2020). For instance, the validation of machine learning models for predicting water quality has highlighted the importance of using diverse datasets to ensure that the models perform well across different environmental conditions (Ahmed et al., 2019).
Various predictive models are employed in water contamination studies, each with unique strengths and applications (Table 4). These models provide essential tools for advancing water quality prediction and decision-making in environmental management. Hydrodynamic and water quality models, such as SWAT and EPANET, are widely recognized for their ability to simulate the transport and fate of contaminants in water systems. SWAT, for example, is particularly effective in assessing the impact of land management practices on water quality, making it a valuable tool for watershed management (Bacu et al., 2011; Femeena et al., 2020). EPANET, on the other hand, excels in modeling water distribution systems and has been enhanced with various extensions to improve its functionality in simulating water quality dynamics (Cervantes, 2023; Seyoum et al., 2013). In addition to traditional hydrodynamic models, statistical and machine learning approaches have gained prominence in recent years for predicting pathogen presence and water quality. Machine learning algorithms, such as support vector machines and random forests, have been successfully applied to predict E. coli presence in tap water, demonstrating their effectiveness in handling complex datasets and identifying key predictors of water quality (Kuroki et al., 2023). These models leverage large volumes of data to uncover patterns that may not be immediately apparent through conventional statistical methods, thus providing a more nuanced understanding of water contamination dynamics (Banerjee et al., 2022). Moreover, the integration of machine learning with traditional modeling approaches has led to the development of hybrid models that capitalize on the strengths of both methodologies. For instance, combining hydrodynamic models with machine learning techniques allows for improved water quality predictions under varying environmental conditions, as demonstrated in studies that assess the impact of climate change on water quality (Park et al., 2013). This hybrid approach enhances predictive accuracy and provides valuable insights into the interactions between different environmental factors and water quality outcomes.
Predictive modeling helps forecast microbial contamination in water systems, offering a valuable tool for improving water quality management in developing countries. By providing data-driven insights, these models assist policymakers and public health officials make informed decisions to mitigate contamination risks and safeguard public health. This section focuses on applying predictive modeling to mitigate microbial contamination in developing countries (Table 5).
Table 5. Application of predictive modeling to mitigate microbial contamination in developing countries.
Identifying risk zones for microbial contamination is critical to public health, particularly in developing countries where infrastructure may be inadequate to manage water safety effectively. Predictive models have emerged as a powerful tool in mapping high-risk contamination areas, particularly those near sewage systems and flood-prone regions. Such predictive models leverage historical data and environmental factors to identify areas at heightened risk for microbial contamination, thereby allowing for implementing proactive measures. For instance, studies have shown that regions near sewage discharge points are significantly more susceptible to contamination from fecal indicator bacteria, which can lead to gastrointestinal diseases (Soller et al., 2014; Schoen and Ashbolt, 2010). Furthermore, flood-prone regions often experience runoff that can introduce pathogens into water supplies, necessitating the integration of predictive modeling to assess risk (Viau et al., 2011).
One of the primary challenges in identifying risk zones is the significant variability in microbial contamination across different geographical areas. Studies have highlighted that fecal contamination is more prevalent in rural areas than urban settings, particularly in regions of Africa and Southeast Asia, where many communities rely on unimproved water sources (Bain et al., 2014a,b). This disparity underscores the necessity for targeted interventions in rural zones, where the risk of waterborne diseases is heightened due to inadequate sanitation infrastructure and limited access to safe drinking water (Bain et al., 2014b). Environmental factors also play a crucial role in microbial contamination. For instance, rainfall has been shown to correlate with increased bacterial contamination in groundwater, as microorganisms can be mobilized from contaminated surfaces into water supplies during heavy rains (Bagordo, 2024). This relationship suggests that seasonal variations must be considered when mapping risk zones, as periods of heavy rainfall may exacerbate contamination levels and increase the risk of waterborne disease outbreaks (Bagordo, 2024). Additionally, anthropogenic activities, such as industrial discharges and agricultural runoff, contribute to the microbial load in water bodies, as demonstrated by Posada-Perlaza et al. (2019) who found that human contamination in the Bogotá River significantly altered microbial communities and promoted the spread of antibiotic resistance genes. Moreover, socio-economic factors significantly influence the risk of microbial contamination. Communities with limited resources often lack the infrastructure for effective waste management and water treatment, leading to increased exposure to pathogens (Bain et al., 2014a,b). For example, the reliance on untreated surface water sources in many rural areas heightens the risk of contamination from nearby agricultural or industrial activities. This situation necessitates a multi-faceted approach to risk zone identification that incorporates socio-economic data alongside environmental assessments to effectively target interventions (Bain et al., 2014a,b). Furthermore, advancements in technology and methodologies can enhance the identification of risk zones. Geographic Information Systems (GIS) and remote sensing technologies offer powerful tools for mapping microbial contamination and identifying at-risk areas based on environmental determinants (Kotchi et al., 2015). These technologies can facilitate the integration of various data sources, including hydrological models and microbial community assessments, to provide a comprehensive understanding of contamination dynamics (Kotchi et al., 2015). In conclusion, the Global South’s perspective on identifying risk zones for microbial contamination in water sources emphasizes the need for a holistic approach that integrates environmental, socio-economic, and technological factors. Addressing these challenges is essential for developing effective public health strategies that can mitigate the risks associated with microbial contamination and improve water safety in vulnerable communities.
Integrating spatial data through GIS with microbial risk assessments enhances the ability to visualize and analyze contamination risks effectively. GIS allows for the layering of various data types, such as population density, land use, and historical contamination events, to create comprehensive risk maps (Schijven et al., 2013a,b; Sulistyawati, 2020). This spatial analysis can identify current risk zones and potential future risks based on environmental changes and urban development (Ogwu, 2019). For example, GIS has successfully been employed in dengue control programs to monitor mosquito populations and predict outbreaks, demonstrating its utility in managing infectious diseases (Schijven et al., 2013a,b). Similarly, applying GIS in water safety assessments can provide valuable insights into the dynamics of microbial contamination, enabling targeted interventions in high-risk areas (Sulistyawati, 2020).
Predicting contamination events and outbreaks is another vital area where predictive modeling plays a significant role. Real-time prediction of microbial contamination following extreme weather events, such as floods and droughts, is crucial for timely public health responses. Extreme weather can disrupt sanitation systems and increase runoff, carrying pathogens into water supplies (Awwad et al., 2019). Predictive models incorporating meteorological data can forecast potential contamination events, allowing for preemptive measures, such as issuing boil water advisories or deploying rapid response teams to affected areas (Chang et al., 2021). Early-warning systems based on these predictive models can significantly reduce the incidence of waterborne diseases by facilitating rapid public health interventions (Viau et al., 2011).
Another significant aspect of predicting contamination events is the role of environmental conditions, particularly rainfall and land use. Studies have shown that heavy rain can lead to increased runoff, which often carries pathogens from agricultural and urban areas into water sources (Wu H. et al., 2021; Cann et al., 2012). For instance, Wu J. et al. (2021) utilized machine learning techniques to track significant sources of water contamination, highlighting how hydrological features and land cover influence microbial sources. This approach underscores the necessity of considering local environmental dynamics when predicting contamination risks. Moreover, socio-economic factors play a crucial role in the vulnerability of communities to waterborne diseases. Many areas in the Global South lack adequate sanitation infrastructure, which exacerbates the risk of contamination during extreme weather events (Cann et al., 2012). For example, the reliance on surface water sources, which are more susceptible to contamination, significantly increases the likelihood of outbreaks, particularly in low-income populations (Ahmed et al., 2020). This relationship between socioeconomic conditions and water quality highlights the need for targeted interventions addressing environmental and community-level vulnerabilities. Technological advancements also offer promising avenues for improving predictions of microbial contamination. Applying QMRA can help estimate the likelihood of waterborne disease outbreaks based on various risk factors, including water quality and exposure levels (Ahmed et al., 2020). Additionally, remote sensing and GIS integration can facilitate real-time monitoring and modeling of microbial contamination events, enabling timely public health responses (Wu J. et al., 2021; Weiskerger et al., 2019).
Resource allocation and decision support are essential to effective public health management, particularly in resource-limited settings. Predictive models can prioritize interventions by identifying at-risk populations and areas and optimizing resource allocation. For instance, quantitative microbial risk assessments (QMRA) can be utilized to estimate the health impacts of various intervention strategies, allowing decision-makers to allocate resources more effectively (Cohen et al., 2021; Ahmed et al., 2010). Decision-making tools incorporating predictive modeling can guide community water treatment and sanitation improvements, ensuring that interventions are practical and efficient (Cohen et al., 2021). By leveraging data-driven insights, public health officials can make informed decisions that enhance water safety and reduce the burden of microbial diseases.
Effective resource allocation is essential for addressing the widespread issue of microbial contamination in the Global South. Bain et al. (2014b) highlight that many water sources, including piped systems and wells, are often contaminated, necessitating targeted interventions to improve water quality. The variability in contamination levels across different source types underscores the importance of context-specific strategies prioritizing resources for the most affected communities (Kumpel et al., 2016). For instance, areas with high levels of fecal contamination require immediate attention to mitigate health risks associated with waterborne diseases (Bain et al., 2014a,b). Decision support systems that leverage data and technology can enhance the management of microbial contaminants. Using QMRA provides a framework for understanding the relationship between water quality and health outcomes, enabling stakeholders to make informed decisions regarding resource allocation (Clasen et al., 2015). Additionally, advancements in microbial source tracking can help identify contamination sources, allowing for targeted interventions that address specific risks (Esselman et al., 2018). These tools can inform public health policies and guide investments in water infrastructure and sanitation improvements (Clasen et al., 2015; Wolf et al., 2018). Community engagement is also vital for effective resource allocation and decision-making. Involving local populations in assessing water quality and identifying contamination sources fosters a sense of ownership and accountability, which can enhance the sustainability of interventions (Kumpel et al., 2016). Moreover, education and awareness campaigns can empower communities to adopt better hygiene practices and advocate for improved water management (Prüss-Ustün et al., 2014).
The application of predictive modeling to mitigate microbial contamination in developing countries has gained significant traction, particularly in the context of water safety. This is especially pertinent in regions such as sub-Saharan Africa and parts of Asia, where waterborne diseases like cholera pose substantial public health risks. The success stories of predictive modeling applications in these areas illustrate the potential of technology to enhance water safety and public health outcomes. Similar predictive modeling efforts have been employed in sub-Saharan Africa to address water safety concerns. For example, in sub-Saharan Africa, predictive models have been used to prevent cholera outbreaks by identifying high-risk areas based on environmental and demographic data (Viau et al., 2011; Cohen et al., 2021). These models have enabled health authorities to implement targeted vaccination campaigns and improve sanitation infrastructure in vulnerable communities. By analyzing land use patterns and hydrological features, researchers could predict the presence of fecal contaminants in drinking water sources. This information was crucial for local health officials, who could prioritize water quality testing and remediation efforts in areas identified as high-risk (Wu H. et al., 2021; Ijabadeniyi et al., 2011). Such applications not only enhance the safety of drinking water but also empower communities to take charge of their water quality management. Controlling water pollution and implementing effective remediation strategies are critical for safeguarding aquatic ecosystems and public health. A multifaceted approach is essential, beginning with the establishment of stringent water quality standards and the implementation of monitoring systems to ensure compliance with these standards (Ntsasa et al., 2024). Best management practices (BMPs) in agriculture, such as the creation of riparian buffer strips and the adoption of sustainable land-use practices, can significantly reduce non-point source pollution, which is a major contributor to water quality degradation (Malaj et al., 2014; Tong et al., 2011). Moreover, the integration of advanced technologies, such as IoT-based water quality monitoring systems, can facilitate real-time tracking of pollution levels and enhance response strategies (Pasika and Gandla, 2020). In urban areas, improved stormwater management practices, including green infrastructure and treatment systems, can mitigate runoff and its associated pollutants (Nwokediegwu et al., 2024). Additionally, the use of innovative remediation techniques, such as nanoremediation, has shown promise in effectively removing contaminants from water bodies (El-Ramady et al., 2017). Collaborative efforts among stakeholders, including governments, industries, and local communities, are vital for developing comprehensive water management plans that address both pollution prevention and remediation (Posthuma et al., 2019; Rout et al., 2022). Employing a combination of regulatory measures, technological advancements, and community engagement, it is possible to create sustainable solutions for controlling water pollution and restoring affected ecosystems (Rout et al., 2022). In parts of Asia, predictive modeling has been used to assess the risk of waterborne diseases following monsoon seasons, allowing for timely interventions that significantly reduce disease incidence (Viau et al., 2011; Cohen et al., 2021). By integrating data on rainfall, temperature, and historical cholera cases, models can predict the likelihood of an outbreak occurring in specific regions. This proactive approach has enabled health authorities to allocate resources more effectively and implement timely interventions, such as vaccination campaigns and public health messaging, reducing cholera’s incidence significantly (Wu J. et al., 2021; Ding et al., 2017). The lessons learned from these implementations highlight the challenges and successes associated with predictive modeling in developing countries. One significant challenge is the availability and quality of data. In many regions, especially rural areas, data on water quality, environmental conditions, and health outcomes may be sparse or unreliable. This lack of data can hinder the development of robust predictive models. However, innovative approaches, such as remote sensing and citizen science, have emerged to address these data gaps. For example, mobile applications that allow community members to report water quality issues can provide valuable real-time data for predictive modeling efforts (Árvai and Post, 2011). Another challenge is the integration of predictive models into existing public health frameworks. Successful implementation requires collaboration among various stakeholders, including government agencies, non-governmental organizations, and local communities. Effective communication and training ensure health officials can interpret and apply model outputs in decision-making processes. In some cases, pilot projects have demonstrated the effectiveness of predictive modeling, leading to broader adoption and integration into public health strategies (Árvai and Post, 2011; Ding et al., 2017).
Moreover, the sustainability of predictive modeling initiatives is a critical consideration. Many projects face funding constraints and may rely on external support. To address this, some researchers advocate for developing local capacity through training programs that empower communities to independently maintain and operate predictive modeling systems. This approach enhances local ownership and ensures that predictive modeling efforts can continue to evolve and adapt to changing environmental and health conditions (Árvai and Post, 2011). In addition to these challenges, the successes of predictive modeling applications in developing countries underscore the importance of interdisciplinary collaboration. Integrating environmental science, public health, and data science expertise is crucial for developing effective predictive models. Collaborative efforts can lead to more comprehensive approaches considering the multifaceted nature of water safety and public health (Wu H. et al., 2021; Wu J. et al., 2021; Árvai and Post, 2011). Furthermore, the role of technology in enhancing predictive modeling capabilities cannot be overstated. Advances in artificial intelligence and machine learning have significantly improved the accuracy and efficiency of predictive models. For instance, using neural networks and time series analysis has enabled researchers to develop models that can predict water quality changes with greater precision, considering various environmental factors (Wu et al., 2023). These technological advancements hold promise for further enhancing the effectiveness of predictive modeling in addressing microbial contamination in water supplies.
Modeling AMR genes in water is a critical and growing aspect of health surveillance, particularly given the increasing prevalence of antimicrobial-resistant bacteria (ARB) in aquatic environments. The presence of antimicrobial resistance genes (ARGs) in water bodies poses significant public health risks, as these genes can be transferred among bacteria, leading to the emergence of multi-drug resistant pathogens. This phenomenon is exacerbated by anthropogenic activities, including the discharge of untreated or inadequately treated wastewater into rivers and lakes, which serve as reservoirs for these resistance genes (Guerra et al., 2022; Cutrupi et al., 2024; Waseem et al., 2017). Recent studies have highlighted the importance of monitoring ARGs in various water sources, including urban drinking water and sewage systems. For instance, Guerra et al. (2022) demonstrated the occurrence of both antimicrobial residues and ARGs in urban drinking water and sewage in Southern Brazil, emphasizing the need for improved sewage treatment and monitoring systems. Similarly, Bueno et al. (2021) employed spatial mapping to quantify and predict the presence of antimicrobials and ARGs in Minnesota’s water bodies, underscoring the role of environmental factors in the spread of AMR. These findings indicate that water bodies can act as significant reservoirs for ARGs, facilitating their dissemination into broader ecosystems (Ogura et al., 2020).
Metagenomic approaches have emerged as powerful tools for advancing the understanding of AMR in aquatic environments. Ottesen et al. (2022) utilized metagenomic and quasimetagenomic methods to analyze surface waters, revealing a complex resistome that is influenced by local anthropogenic activities, such as proximity to hospitals. This aligns with the findings of Hendriksen et al. (2019) who conducted metagenomic analyses of urban sewage, highlighting the critical role of wastewater in the global spread of AMR. Such methodologies not only provide insights into the diversity of ARGs present but also help in assessing the potential health risks associated with exposure to contaminated water sources. The environmental persistence of ARGs is further complicated by the selective pressure exerted by the presence of antimicrobials in water. Studies have shown that exposure to disinfectants like chlorine can enhance the expression of resistance genes in microbial populations, thereby increasing the survival and proliferation of ARB in treated water (Karumathil et al., 2014; Hayward et al., 2020). This phenomenon is particularly concerning in wastewater treatment plants (WWTPs), which are often hotspots for horizontal gene transfer among bacteria (Kotlarska et al., 2014). The implications of these findings are profound, as they suggest that even treated water can harbor significant levels of AMR, posing risks to human health and the environment.
Developing countries face significant challenges in data collection and technological infrastructure, which hinder effective environmental management and decision-making. Limited access to reliable data, inadequate technological tools, and lack of skilled personnel contribute to difficulties in addressing ecological issues. Some the key data and technological challenges in developing countries are outlined in Table 6.
In low-resource settings, the issues surrounding incomplete or unreliable water quality data are profound and multifaceted. The lack of comprehensive data hampers effective decision-making and policy formulation, leading to adverse public health outcomes. For instance, highlight that incomplete water event data significantly undermines the management of transboundary river basins, which is critical for ensuring water quality and availability in China and neighboring countries (Wang and Lv, 2022). Similarly, the study emphasizes that chronic environmental contamination, such as that from per- and poly-fluoroalkyl substances, exacerbates community stress and complicates public health responses due to inadequate data on contamination levels (Calloway et al., 2020). This lack of reliable data affects immediate public health interventions and undermines long-term strategies for sustainable water resource management. To address these data gaps, innovative approaches to data collection are essential. Mobile-based reporting systems have emerged as a viable solution, enabling real-time data collection and reporting from communities directly affected by water quality issues. Such systems can enhance the accuracy and timeliness of data, as demonstrated in various pilot projects across developing regions. Community involvement is another critical component; engaging local populations in monitoring efforts can foster a sense of ownership and responsibility toward water resources. Phungela et al. (2022) argued that effective water quality monitoring is vital for identifying contributors to water quality variations, which can inform integrated water resource management strategies. By leveraging local knowledge and participation, data collection efforts can become more robust and reflective of actual conditions on the ground.
Moreover, integrating community-driven data collection with mobile technology can enhance the reliability of water quality assessments. This approach democratizes data collection and empowers communities to actively safeguard their water resources. The use of participatory methods in data collection has been shown to yield more accurate and contextually relevant information, thereby improving the overall quality of data available for public health decision-making (Zhang and Wang, 2022). As such, addressing data gaps through innovative and community-focused strategies is crucial for mitigating the impacts of microbial contamination in developing countries.
Technological limitations pose significant challenges to the practical application of predictive modeling in resource-constrained environments. Limited access to advanced technologies and computational infrastructure restricts the ability of public health officials and researchers to develop and implement sophisticated models that can predict water quality and contamination levels. For instance, the reliance on outdated technologies can lead to inefficiencies in data processing and analysis, as highlighted by the findings of Helly et al. (2021), who noted that even in relatively developed regions, uncertainties in data quality can hinder effective decision-making. This situation is exacerbated in low-resource settings where access to modern computational tools is often severely limited. To overcome these technological barriers, strategies such as cloud computing and the use of open-source platforms can be employed. Cloud computing offers a scalable solution that allows for the storage and processing of large datasets without the need for significant local infrastructure investment. This approach can facilitate collaborative research efforts and enable access to advanced analytical tools that would otherwise be unavailable in resource-limited settings. Furthermore, open-source platforms can democratize access to modeling tools, allowing local researchers and public health officials to adapt and utilize these resources according to their specific needs. Zhang and Wang (2022) emphasize the importance of building social resilience in public health governance, which includes leveraging technology to enhance data collection and analysis capabilities. Additionally, adapting existing modeling tools to fit the constraints of low-resource environments is crucial. This may involve simplifying models to reduce computational demands while providing valuable insights into water quality dynamics. Integrating local knowledge and expertise into these models can also enhance their relevance and applicability. By fostering an environment where local researchers can contribute to model development, the predictive capabilities of these tools can be significantly improved, leading to better-informed public health interventions (Bergeron et al., 2017). Ultimately, addressing technological limitations through innovative strategies is essential for enhancing the effectiveness of predictive modeling in mitigating microbial contamination in developing countries.
Building local expertise in predictive modeling and data interpretation is paramount for addressing the challenges of microbial contamination in water resources. The importance of training and capacity-building initiatives cannot be overstated, as they empower local public health officials, water authorities, and community members to utilize data and modeling tools effectively. As highlighted by Galway et al. (2016), interdisciplinary research capacity is essential for addressing complex public health challenges, including those related to water quality. Without adequate training, local stakeholders may struggle to interpret data accurately or apply predictive models effectively, leading to suboptimal decision-making. Capacity-building programs should be tailored to the needs of public health officials and water authorities in developing countries. These programs can include workshops, online courses, and hands-on training sessions focusing on predictive modeling techniques, data analysis, and interpretation. For instance, Meredith (2023) emphasized the need for facilitated online learning to build strategic public health skills, which can be particularly beneficial in resource-constrained environments. By enhancing the skills of local practitioners, these initiatives can foster a more informed and capable workforce better equipped to tackle water quality issues.
Moreover, community involvement in capacity-building efforts is crucial for ensuring the sustainability of these initiatives. Engaging local communities in training programs can enhance their understanding of water quality issues and empower them to monitor and manage their water resources actively. This participatory approach builds local capacity and fosters a sense of ownership and responsibility toward water quality management. The findings highlight the importance of building capability within the community and public health workforce to ensure sustainable public health interventions (Adams and Dickinson, 2010).
Integrating predictive models with public health surveillance systems is paramount for enhancing disease surveillance and control, particularly in waterborne diseases. Predictive modeling leverages historical data and real-time information to forecast disease outbreaks, enabling public health officials to implement timely interventions. For instance, the application of artificial intelligence (AI) in public health surveillance systems has shown significant promise in identifying and containing infectious diseases, particularly waterborne pathogens (Adefemi, 2023). By utilizing predictive analytics, health authorities can anticipate outbreaks based on environmental factors, historical trends, and population movements, optimizing resource allocation and response strategies. Moreover, integrating predictive models into public health frameworks can guide waterborne disease outbreak response strategies. The systematic review by Wang and Yang (2019) highlighted the effectiveness of remote sensing techniques in monitoring water quality, which is crucial for predicting potential outbreaks of waterborne diseases. Such monitoring systems can provide comprehensive data on water quality indicators, allowing for proactive management measures. This proactive approach is essential in developing countries where inadequate surveillance systems and limited resources often exacerbate the burden of waterborne diseases. By combining predictive modeling with public health surveillance, authorities can enhance their capacity to respond to outbreaks, ultimately reducing morbidity and mortality associated with waterborne diseases. The role of community engagement in improving disease surveillance cannot be overstated. Cohen et al. (2019) emphasized the importance of social capital in a community’s recovery during emergencies, suggesting that community engagement skills among emergency responders can significantly improve public health outcomes. By fostering trust and communication within communities, public health officials can enhance the effectiveness of surveillance systems, ensuring that individuals are more likely to report illnesses and adhere to public health recommendations. This community-centered approach is particularly vital in developing countries, where cultural factors and trust in health systems can significantly influence disease reporting and response efforts.
Predictive modeling plays a critical role in long-term water quality monitoring and sustainable management, particularly in the context of achieving the Sustainable Development Goals (SDGs). SDG 6 emphasizes the importance of ensuring the availability and sustainable management of water and sanitation for all. The Integrated Monitoring Initiative for SDG 6 (IMI-SDG 6) provides a comprehensive framework for monitoring water quality indicators, including ambient water quality, which is essential for assessing progress toward this goal (Wu, 2023). By employing predictive models, stakeholders can identify trends in water quality over time, enabling them to implement targeted interventions to mitigate pollution and improve water management practices. Additionally, predictive tools can facilitate the achievement of water-related SDGs by providing insights into the interlinkages between water quality and other sustainable development objectives. For example, the research by Alcamo (2019) highlighted the synergies between water quality and various SDGs, suggesting that improvements in water quality can positively impact health, education, and economic development. By integrating predictive modeling into water management strategies, policymakers can better understand these interlinkages and develop comprehensive approaches that address multiple SDGs simultaneously. The importance of effective water governance is also underscored in the context of sustainable water management. Improved water governance supports social, economic, and environmental objectives, as highlighted by (Bertule et al., 2018). By utilizing predictive models to assess governance progress, stakeholders can identify areas for improvement and implement strategies that enhance the resilience of water systems. This is particularly relevant in developing countries, where governance challenges often hinder effective water management and contribute to water quality issues.
Using predictive models to empower communities with information on water safety and contamination risks is essential for promoting community health and resilience. By providing communities with real-time data on water quality, stakeholders can enhance public awareness and encourage proactive measures to mitigate contamination risks. For instance, Imani et al. (2021) demonstrated the feasibility of integrating historical data and machine learning techniques to develop resilience predictive models for water quality. Such models can inform communities about potential risks, enabling them to take preventive actions and improve their resilience to waterborne diseases. Building resilient water systems is crucial for protecting vulnerable populations in developing countries. The research by Allen et al. (2021) emphasized the importance of integrating public health considerations into water infrastructure planning, particularly in climate change and its impacts on water resources. By adopting a holistic approach that considers the interconnections between water infrastructure, public health, and community resilience, stakeholders can develop better systems to withstand environmental stresses and respond to public health emergencies. Furthermore, community resilience is closely linked to the availability and accessibility of healthcare services. Cohen et al. (2020) highlighted the role of healthcare facilities in building community resilience, particularly during emergencies. When communities have confidence in the availability of health services, they are more likely to engage in preventive health behaviors and report health issues, ultimately enhancing community resilience. This underscores the need for integrated approaches that combine water management, public health, and community engagement to foster resilience in vulnerable populations.
Integrating predictive modeling in water management is increasingly recognized as critical for effective governance and sustainable resource management. This integration is heavily influenced by policy frameworks that provide the necessary support and guidance for adopting and implementing predictive modeling techniques. Such frameworks are essential for establishing standards, facilitating data sharing, and promoting collaboration among various stakeholders involved in water management. The importance of these policy frameworks cannot be overstated, as they align the interests of different organizations, ensuring that predictive modeling is adopted and effectively utilized to address the challenges posed by microbial contaminants in water systems.
Governmental and international organizations play a vital role in fostering an environment conducive to data sharing and collaboration. By establishing policies that encourage transparency and cooperation among stakeholders, these entities can enhance the effectiveness of predictive modeling initiatives. For instance, as Dewulf et al. (2011 discussed), collaborative frameworks emphasize connecting various actors’ perspectives to effectively address complex water governance issues. Establishing such frameworks is crucial in overcoming barriers to collaboration, which can often stem from fragmented governance structures and differing organizational priorities (Widmer et al., 2019). Moreover, international organizations usually provide the technical expertise and financial resources necessary for implementing predictive modeling projects, thereby facilitating the sharing of best practices and lessons learned across borders (Feng, 2023). Institutional collaboration is another critical aspect of effectively implementing predictive modeling in water management. Partnerships between governments, research institutions, and non-governmental organizations (NGOs) can significantly enhance data collection, analysis, and interpretation capacity. These partnerships allow for the pooling of resources and expertise, which is particularly important in addressing the multifaceted challenges of microbial contamination in water systems. For example, the collaborative governance model proposed by Widmer et al. (2019) highlighted the importance of social-ecological fit in managing water quality across different jurisdictions. This model underscores the need for interconnected governance arrangements that can adapt to the complexities of water management, particularly in transboundary contexts where multiple stakeholders are involved.
International agencies also play a vital role in providing technical and financial support for predictive modeling initiatives. These agencies often facilitate capacity-building efforts, enabling local governments and organizations to develop the necessary skills and knowledge to implement predictive modeling effectively. The strategic vision for international collaboration outlined by Feng (2023) emphasized the importance of establishing partnerships and sharing experiences to enhance resource management capabilities. Furthermore, global organizations can assist in developing common standards and guidelines that promote consistency and reliability in predictive modeling practices, fostering stakeholder trust and encouraging broader participation in collaborative efforts (Boer et al., 2016). Integrating predictive modeling within policy frameworks requires a concerted effort to address the various barriers that may hinder collaboration. As highlighted by Turek et al. (2022), understanding the dynamics of stakeholder engagement is crucial for fostering effective partnerships. This understanding can be achieved through comprehensive stakeholder analyses that identify common interests and facilitate aligning goals among different organizations. Additionally, the role of community management in rural water supplies, as discussed by Opare (2011), illustrated the potential for local communities to engage in collaborative water management efforts, provided they receive adequate support and resources from external agencies. This local engagement is essential for ensuring that predictive modeling efforts are grounded in the realities of the communities they aim to serve.
Integrating emerging technologies such as artificial intelligence (AI) and big data analytics into predictive modeling for water contamination presents a transformative opportunity for water management. These technologies enable the processing and analysis of vast datasets, facilitating real-time monitoring and predictive analytics that can significantly enhance decision-making processes in water quality management (Li et al., 2019). For instance, AI algorithms can identify patterns and predict potential contamination events by analyzing historical water quality data alongside environmental variables (Liu and Yang, 2018). The ability to harness big data analytics allows for integrating diverse datasets, including meteorological, hydrological, and anthropogenic factors, which are crucial for understanding complex water systems (Ma et al., 2020a). Moreover, interdisciplinary research combining water science, public health, and data science is essential for developing robust predictive models that address the multifaceted nature of water contamination. The collaboration between these fields can lead to innovative approaches that predict water quality issues and assess their implications for public health (Golembiewski et al., 2018). For example, integrating public health data with water quality models can help identify vulnerable populations and inform targeted interventions (Zhang et al., 2023). This interdisciplinary approach is vital for creating comprehensive solutions addressing water quality challenges’ environmental and health dimensions (Piffer et al., 2021).
Scaling successful predictive modeling approaches across different regions requires strategic planning and adaptation to local contexts, particularly in the developing world. One effective strategy involves customizing models to account for regional variability in water quality challenges. This necessitates a thorough understanding of local hydrological conditions, pollution sources, and socio-economic factors influencing water quality (Ma et al., 2020b). For instance, models developed in urban settings may not directly apply to rural areas due to differences in land use, population density, and pollution sources (Garaba et al., 2015). Therefore, localized data collection and model calibration are critical for ensuring the accuracy and relevance of predictive models in diverse contexts (Galway et al., 2016). Additionally, addressing regional variability involves engaging local stakeholders in the modeling process. Collaborative efforts with local communities, government agencies, and non-governmental organizations can enhance the applicability of predictive models by incorporating local knowledge and priorities (Pugas, 2023). This participatory approach fosters ownership of the modeling outcomes and ensures that the solutions developed are culturally and contextually appropriate (Su et al., 2022). Furthermore, leveraging technology such as mobile applications for data collection can facilitate community involvement and enhance the richness of the data used in predictive modeling (Aminia et al., 2021).
Ensuring the sustainability of predictive modeling efforts beyond initial implementation is critical for water quality management. This involves establishing frameworks for continuously monitoring, evaluating, and adapting models to reflect changing environmental conditions and emerging challenges (Islam and Repella, 2015). Long-term sustainability can be achieved by integrating predictive modeling into existing water management policies and practices, ensuring that these tools are not viewed as standalone solutions but as integral components of a broader water governance framework (Fefferman, 2023). Moreover, measuring the long-term impacts of modeling solutions on water quality and public health is essential for assessing the effectiveness of predictive modeling initiatives. Longitudinal studies that track changes in water quality and health outcomes over time can provide valuable insights into the efficacy of interventions guided by predictive models (Kemp and Nurius, 2015). Such studies should establish causal relationships between modeling outputs and real-world outcomes, reinforcing the importance of data-driven decision-making in water management (Richter et al., 2021).
The role of predictive modeling in mitigating microbial contamination of water sources in developing countries has emerged as a critical component in addressing public health challenges associated with waterborne diseases. The findings from various studies underscore the effectiveness of predictive models in assessing microbial water quality, identifying contamination sources, and informing water management strategies. For instance, it demonstrated that incorporating land use patterns and chemical tracers significantly enhances the predictive performance of models to forecast microbial fecal indicators, thereby facilitating urban development planning that prioritizes human health protection. Similarly, research illustrates how predictive models can perform empirical risk assessments, allowing for targeted interventions before contamination occurs. These insights highlight the need for advanced modeling techniques to safeguard water quality in vulnerable regions. The broader implications of predictive modeling extend beyond immediate microbial contamination concerns, significantly impacting public health and development. By improving water safety, predictive modeling reduces waterborne diseases, disproportionately affecting children and marginalized populations in developing countries. For example, conducted a systematic review that revealed a strong correlation between fecal contamination in drinking water and the prevalence of waterborne diseases, emphasizing the urgent need for effective monitoring and intervention strategies (Bain et al., 2014a,b). Furthermore, the work indicates that using deep tube wells, less susceptible to surface contamination, has led to a notable decline in childhood diarrhea rates in Bangladesh (Escamilla et al., 2011). These findings collectively reinforce the notion that predictive modeling is not merely a technical tool but a vital strategy for enhancing public health outcomes and fostering sustainable development. Considering these findings, there is an urgent call to action for increased investment in predictive modeling technologies and capacity-building initiatives. Collaborative efforts among governments, NGOs, and local communities are essential to protect vulnerable populations from microbial water contamination. As highlighted by Onda et al. (2012), integrating household water treatment strategies can significantly mitigate health risks associated with contaminated water sources. Moreover, establishing water safety plans, as advocated by Leftwich et al. (2021), is crucial for ensuring the continuous functionality and safety of water supply systems. By prioritizing these investments and collaborative approaches, stakeholders can effectively address the pressing challenges of microbial contamination and enhance the resilience of water systems in developing countries.
SI: Conceptualization, Data curation, Formal Analysis, Funding acquisition, Investigation, Methodology, Project administration, Resources, Software, Supervision, Validation, Visualization, Writing – original draft, Writing – review and editing. MO: Conceptualization, Data curation, Formal Analysis, Funding acquisition, Investigation, Methodology, Project administration, Resources, Software, Supervision, Validation, Visualization, Writing – original draft, Writing – review and editing.
The author(s) declare that no financial support was received for the research and/or publication of this article.
The authors declare that the research was conducted in the absence of any commercial or financial relationships that could be construed as a potential conflict of interest.
The authors declare that no Generative AI was used in the creation of this manuscript.
All claims expressed in this article are solely those of the authors and do not necessarily represent those of their affiliated organizations, or those of the publisher, the editors and the reviewers. Any product that may be evaluated in this article, or claim that may be made by its manufacturer, is not guaranteed or endorsed by the publisher.
Abimbola, O., Mittelstet, A., Messer, T., Berry, Bartelt-Hunt, S., and Hansen, S. (2020). Predicting Escherichia coli loads in cascading dams with machine learning: An integration of hydrometeorology, animal density and grazing pattern. Sci. Total Environ. 722:137894. doi: 10.1016/j.scitotenv.2020.137894
Abuzerr, S. (2024). Quantitative microbial risk assessment for Escherichia Coli O157: h7 via drinking water in the Gaza Strip, Palestine. SAGE Open Med. 12:20503121241258071. doi: 10.1177/20503121241258071
Adams, J., and Dickinson, P. (2010). Evaluation training to build capability in the community and public health workforce. Am. J. Eval. 31, 421–433. doi: 10.1177/1098214010366586
Adefemi, A. (2023). Artificial intelligence in environmental health and public safety: A comprehensive review of usa strategies. World J. Adv. Res. Rev. 20, 1420–1434. doi: 10.30574/wjarr.2023.20.3.2591
Adler, B., and de la Peña Moctezuma, A. (2010). Leptospira and leptospirosis. Vet. Microbiol. 140, 287–296. doi: 10.1016/j.vetmic.2009.03.012
Agedah, E. C., Ineyougha, E. R., Izah, S. C., and Orutugu, L. A. (2015). Enumeration of total heterotrophic bacteria and some physico-chemical characteristics of surface water used for drinking sources in Wilberforce Island. Nigeria. J. Environ. Treat. Tech. 3, 28–34.
Ahmed, J., Wong, L., Chua, Y., Channa, N., Mahar, R., Yasmin, A., et al. (2020). Quantitative microbial risk assessment of drinking water quality to predict the risk of waterborne diseases in primary-school children. Int. J. Environ. Res. Public Health 17:2774. doi: 10.3390/ijerph17082774
Ahmed, U., Mumtaz, R., Anwar, H., Shah, A. A., Irfan, R., and García-Nieto, J. (2019). Efficient water quality prediction using supervised machine learning. Water 11:2210. doi: 10.3390/w11112210
Ahmed, W., Vieritz, A., Goonetilleke, A., and Gardner, T. (2010). Health risk from the use of roof-harvested rainwater in Southeast Queensland, Australia, as potable or nonpotable water, determined using quantitative microbial risk assessment. Appl Environ Microbiol. 76, 7382–7391. doi: 10.1128/AEM.00944-10
Alcamo, J. (2019). Water quality and its interlinkages with the sustainable development goals. Curr. Opin. Environ. Sustainabil. 36, 126–140. doi: 10.1016/j.cosust.2018.11.005
Ali, W., Chen, N., Umar, W., Sundas, A., Nisa, Z., and Mahfuzur, R. (2020). Assessment of runoff, sediment yields and nutrient loss using the swat model in upper indus basin of pakistan. J. Geosci. Environ. Protection 8, 62–81. doi: 10.4236/gep.2020.89004
Allen, T., Behr, J., Bukvic, A., Calder, R., Caruson, K., Connor, C., et al. (2021). Anticipating and adapting to the future impacts of climate change on the health, security and welfare of low elevation coastal zone (lecz) communities in southeastern USA. J. Mar. Sci. Eng. 9:1196. doi: 10.3390/jmse9111196
Aminia, S., Ponta, J., van der Sterrena, M., and Freebairnb, A. (2021). “Lessons learned using dsednet plug-in within a water supply catchment for greater Sydney,” in Proceedings of the 24th International Congress on Modelling and Simulation, (Sydney, NS), doi: 10.36334/modsim.2021.f7.amini
Armah, F., Ekumah, B., Yawson, D., Odoi, J., Afitiri, A., and Nyieku, F. (2018). Access to improved water and sanitation in sub-Saharan Africa in a quarter century. Heliyon 4:e00931. doi: 10.1016/j.heliyon.2018.e00931
Árvai, J., and Post, K. (2011). Risk management in a developing country context: Improving decisions about point-of-use water treatment among the rural poor in Africa. Risk Anal. 32, 67–80. doi: 10.1111/j.1539-6924.2011.01675.x
Atta, M., Amer, W., Ali, G., and Zamzam, A. (2019). Relation between drinking water contamination and gastroenteritis Egyptian. J. Med. Microbiol. 28, 17–24. doi: 10.21608/ejmm.2019.282950
Awwad, A., Gobran, M., Kamal, R., and Boraey, M. (2019). A 3d risk assessment model based on gis layers technique. Int. J. Performability Eng. 15:2563. doi: 10.23940/ijpe.19.10.p1.25632569
Bacu, V., Mihon, D., Rodila, D., Stefănut, T., and Gorgan, D. (2011). Gswat Platform for Grid Based Hydrological Model Calibration and Execution. Piscataway, NJ: IEEE, doi: 10.1109/ispdc.2011.52
Bagordo, F. (2024). Factors influencing microbial contamination of groundwater: A systematic review of field-scale studies. Microorganisms 12:913. doi: 10.3390/microorganisms12050913
Bain, R., Cronk, R., Hossain, R., Bonjour, S., Onda, K., Wright, J., et al. (2014a). Global assessment of exposure to faecal contamination through drinking water based on a systematic review. Trop. Med. Int. Health 19, 917–927. doi: 10.1111/tmi.12334
Bain, R., Cronk, R., Wright, J., Yang, H., Slaymaker, T., and Bartram, J. (2014b). Fecal contamination of drinking-water in low- and middle-income countries: A systematic review and meta-analysis. PLoS Med. 11:e1001644. doi: 10.1371/journal.pmed.1001644
Banerjee, K., Bali, V., Nawaz, N., Bali, S., Mathur, S., Mishra, R., et al. (2022). A machine-learning approach for prediction of water contamination using latitude, longitude, and elevation. Water 14:728. doi: 10.3390/w14050728
Ben-Eledo, V., Kigigha, L., Izah, S., and Eledo, B. (2017). Bacteriological quality assessment of epie creek, niger delta region of Nigeria. Int. J. Ecotoxicol. Ecobiol. 2, 102–108.
Bentham, R., and Whiley, H. (2018). Quantitative microbial risk assessment and opportunist waterborne infections are there too many gaps to fill? Int. J. Environ. Res. Public Health 15:1150. doi: 10.3390/ijerph15061150
Bergeron, K., Abdi, S., DeCorby, K., Mensah, G., Rempel, B., and Manson, H. (2017). Theories, models and frameworks used in capacity building interventions relevant to public health: A systematic review. BMC Public Health 17:914. doi: 10.1186/s12889-017-4919-y
Bertule, M., Glennie, P., Bjørnsen, P., Lloyd, G., Kjellén, M., Dalton, J., et al. (2018). Monitoring water resources governance progress globally: Experiences from monitoring sdg indicator 6.5.1 on integrated water resources management implementation. Water 10:1744. doi: 10.3390/w10121744
Bethony, J., Brooker, S., Albonico, M., Geiger, S., Loukas, A., Diemert, D., et al. (2006). Soil-transmitted helminth infections: Ascariasis, trichuriasis, and hookworm. Lancet 367, 1521–1532. doi: 10.1016/S0140-6736(06)68653-4
Blaser, M. J., Duncan, D. J., and Li, H. (2008). Campylobacter jejuni and related species. Clin. Microbiol. Rev. 21, 213–229. doi: 10.1128/CMR.00066-06
Boer, C., Kruijf, J., Özerol, G., and Bressers, H. (2016). Collaborative water resource management: What makes up a supportive governance system? Environ. Policy Governance 26, 229–241. doi: 10.1002/eet.1714
Bueno, I., Beaudoin, A., Arnold, W., Kim, T., Frankson, L., LaPara, T., et al. (2021). Quantifying and predicting antimicrobials and antimicrobial resistance genes in waterbodies through a holistic approach: A study in Minnesota. United States. Sci. Rep. 11:18747. doi: 10.1038/s41598-021-98300-5
Calloway, E., Chiappone, A., Schmitt, H., Sullivan, D., Gerhardstein, B., Tucker, P., et al. (2020). Exploring community psychosocial stress related to per- and poly-fluoroalkyl substances (PFAS) contamination: Lessons learned from a qualitative study. Int. J. Environ. Res. Public Health 17:8706. doi: 10.3390/ijerph17238706
Cann, K., Thomas, D., Salmon, R., Wyn-Jones, A., and Kay, D. (2012). Extreme water-related weather events and waterborne disease. Epidemiol. Infect. 141, 671–686. doi: 10.1017/S0950268812001653
Central Pollution Control Board (CPCB). (2019). Ganga River Water Quality Assessment. New Delhi: Government of India.
Cervantes, D. (2023). Epanet inp code for incomplete mixing model in cross junctions for water distribution networks. Water 15:4253. doi: 10.3390/w15244253
Chang, C., Li, C., and Wang, C. (2021). A multi-step-ahead markov conditional forward model with cube perturbations for extreme weather forecasting. Proc. AAAI Conf. Artificial Intell. 35, 6948–6955. doi: 10.1609/aaai.v35i8.16856
Chou, J.-S., Yu, C.-P., Truong, D.-N., Susilo, B., Hu, A., and Sun, Q. (2019). Predicting microbial species in a river based on physicochemical properties by bio-inspired metaheuristic optimized machine learning. Sustainability 11:6889. doi: 10.3390/su11246889
Clasen, T., Alexander, K., Sinclair, D., Boisson, S., Peletz, R., Chang, H., et al. (2015). Interventions to improve water quality for preventing diarrhoea. Cochrane Database Syst Rev. 2015:CD004794. doi: 10.1002/14651858.CD004794.pub3
Cohen, J., Fink, D., and Zuckerberg, B. (2021). Extreme winter weather disrupts bird occurrence and abundance patterns at geographic scales. Ecography 44, 1143–1155. doi: 10.1111/ecog.05495
Cohen, O., Mahagna, A., Shamia, A., and Slobodin, O. (2020). Health-care services as a platform for building community resilience among minority communities: An israeli pilot study during the COVID-19 outbreak. Int. J. Environ. Res. Public Health 17:7523. doi: 10.3390/ijerph17207523
Cohen, O., Shapira, S., Aharonson-Daniel, L., and Shamian, J. (2019). Confidence in health-services availability during disasters and emergency situations-does it matter-lessons learned from an israeli population survey. Int. J. Environ. Res. Public Health 16:3519. doi: 10.3390/ijerph16193519
Colford, J., Schiff, K., Griffith, J., Yau, V., Arnold, B., Wright, C., et al. (2012). Using rapid indicators for Enterococcus to assess the risk of illness after exposure to urban runoff contaminated marine water. Water Res. 46, 2176–2186. doi: 10.1016/j.watres.2012.01.033
Colley, D., Bustinduy, A., Secor, W., and King, C. (2014). Human schistosomiasis. Lancet 383, 2253–2264. doi: 10.1016/S0140-6736(13)61949-2
Crump, J., Luby, S., and Mintz, E. D. (2004). The global burden of typhoid fever. Bull. World Health Organ. 82, 346–353.
Cutrupi, F., Osińska, A., Rahmatika, I., Afolayan, J., Vystavna, Y., Mahjoub, O., et al. (2024). Towards monitoring the invisible threat: A global approach for tackling amr in water resources and environment. Front. Water 6:1362701. doi: 10.3389/frwa.2024.1362701
Davis, M., Janke, R., and Taxon, T. (2018). Mass imbalances in EPANET water-quality simulations. Drink Water Eng. Sci. 11, 25–47. doi: 10.5194/dwes-11-25-2018
Dewulf, A., Mancero, M., Cárdenas, G., and Sucozhañay, D. (2011). Fragmentation and connection of frames in collaborative water governance: A case study of river catchment management in southern ecuador. Int. Rev. Adm. Sci. 77, 50–75. doi: 10.1177/0020852310390108
Ding, X., Zhang, J., Jiang, G., and Zhang, S. (2017). Early warning and forecasting system of water quality safety for drinking water source areas in three gorges reservoir area, china. Water 9:465. doi: 10.3390/w9070465
El-Ramady, H., Alshaal, T., El-Henawy, A., Abdalla, N., Taha, H., Elmahrouk, M., et al. (2017). Environmental nanoremediation under changing climate. Environ. Biodiver. Soil Security 1, 190–200. doi: 10.21608/jenvbs.2017.1550.1009
Enaregha, E., Omotete, T., Izah, S., and Odubo, T. (2022). Comparison of total viable bacteria counts and in situ characteristics in drinking water sources in Sagbama town, Bayelsa state, Nigeria. Int. J. Pathogen Res. 11, 79–87.
Erhunmwunse, N. O., Pajiah, T. J., and Ogwu, M. C. (2024). “Microplastics as water pollutants and sustainable management strategies,” in Water Crises and Sustainable Management in the Global South, eds S. C. Izah, M. C. Ogwu, A. Loukas, and H. Hamidifar (Singapore: Springer), doi: 10.1007/978-981-97-4966-9_8
Erinle, K. O., Ogwu, M. C., Evivie, S. E., Zaheer, M. S., Ogunyemi, S. O., and Adeniran, S. O. (2021). Impacts of COVID-19 on agriculture and food security in developing countries: Potential mitigation strategies. CAB Rev. 16, 1–16. doi: 10.1079/PAVSNNR202116016
Escamilla, V., Wagner, B., Yunus, M., Streatfield, P., van Geen, A., and Emch, M. (2011). Effect of deep tube well use on childhood diarrhoea in Bangladesh. Bull. World Health Organ. 89, 521–527. doi: 10.2471/BLT.10.085530
Esselman, P., Jiang, S., Peller, H., Buck, D., and Wainwright, J. (2018). Landscape drivers and social dynamics shaping microbial contamination risk in three maya communities in southern belize, central america. Water 10:1678. doi: 10.3390/w10111678
Estes, M. K., and Kapikian, A. Z. (2007). “Rotaviruses,” in Fields Virology, 5th Edn, eds D. M. Knipe and P. M. Howley (Philadelphia: Lippincott Williams and Wilkins), 1917–1974.
Fearnside, P. M. (2016). Deforestation of the Amazon rainforest: A global perspective. Environ. Sci. Policy 61, 1–10.
Fefferman, N. (2023). A new paradigm for pandemic preparedness. Curr. Epidemiol. Rep. 10, 240–251. doi: 10.1007/s40471-023-00336-w
Femeena, P., Chaubey, I., Aubeneau, A., McMillan, S., Wagner, P., and Fohrer, N. (2020). An improved process-based representation of stream solute transport in the soil and water assessment tools. Hydrol. Processes 34, 2599–2611. doi: 10.1002/hyp.13751
Feng, Y. (2023). International Collaboration and Cooperation Strategy for Comprehensive Natural Resource Surveys. Amsterdam: Atlantis press, 177–183. doi: 10.2991/978-94-6463-260-6_23
Galway, L., Parkes, M., Allen, D., and Takaro, T. (2016). Building interdisciplinary research capacity: A key challenge for ecological approaches in public health. AIMS Public Health 3, 389–406. doi: 10.3934/publichealth.2016.2.389
Garaba, S., Friedrichs, A., Voß, D., and Zielinski, O. (2015). Classifying natural waters with the forel-ule colour index system: Results, applications, correlations and crowdsourcing. Int. J. Environ. Res. Public Health 12, 16096–16109. doi: 10.3390/ijerph121215044
Gemeda, S., Soboksa, N., Tefera, Y., Desta, A., and Gari, S. R. (2022). PCR-based detection of pathogens in improved water sources: A scoping review protocol of the evidence in low-income and middle-income countries. BMJ Open 12:e057154. doi: 10.1136/bmjopen-2021-057154
Golembiewski, E., Holmes, A., Jackson, J., Brown-Podgorski, B., and Menachemi, N. (2018). Interdisciplinary dissertation research among public health doctoral trainees, 2003-2015. Public Health Rep. 133, 182–190. doi: 10.1177/0033354918754558
Guerra, R., Mezzomo, L., Machado, W., Hein, C., Müller, C., Silva, T., and Martins, A. (2022). The occurrence of antimicrobial residues and antimicrobial resistance genes in urban drinking water and sewage in southern brazil. Braz. J. Microbiol. 53, 1483–1489. doi: 10.1007/s42770-022-00786-2
Gwimbi, P., George, M., and Ramphalile, M. (2019). Bacterial contamination of drinking water sources in rural villages of Mohale Basin, Lesotho: Exposures through neighbourhood sanitation and hygiene practices. Environ. Health Prev. Med. 24:33. doi: 10.1186/s12199-019-0790-z
Hayward, C., Ross, K., Brown, M., and Whiley, H. (2020). Water as a source of antimicrobial resistance and healthcare-associated infections. Pathogens 9:667. doi: 10.3390/pathogens9080667
Helly, J., Cayan, D., Corringham, T., Stricklin, J., and Hillaire, T. (2021). Patterns of water use in california. San Francisco Estuary Watershed Sci. 19, 1–38. doi: 10.15447/sfews.2021v19iss4art2
Hendriksen, R., Munk, P., Njage, P., van Bunnik, B., McNally, L., Lukjancenko, O., et al. (2019). Global monitoring of antimicrobial resistance based on metagenomics analyses of urban sewage. Nat. Commun. 10:1124. doi: 10.1038/s41467-019-08853-3
Hering, J., Waite, T., Luthy, R., Drewes, J., and Sedlak, D. L. A. (2013). changing framework for urban water systems. Environ. Sci. Technol. 47, 10721–10726. doi: 10.1021/es4007096
Hinton, R. (2023). Spatial model of groundwater contamination risks from pit-latrines to 2070: Case study malawi. Water Res. 267, 122734. doi: 10.21203/rs.3.rs-3604573/v1
Holvoet, K., Jacxsens, L., Sampers, I., and Uyttendaele, M. (2012). Insight into the prevalence and distribution of microbial contamination to evaluate water management in the fresh produce processing industry. J. Food Prot. 75, 671–681. doi: 10.4315/0362-028X.JFP-11-175
Hopkins, D., Ruiz-Tiben, E., Downs, P., Withers, P., and Roy, S. (2008). Dracunculiasis eradication: Neglected no longer. Am. J. Trop. Med. Hyg. 79, 474–479.
Ifelebuegu, A., Ukpebor, J., Ahukannah, A., Nnadi, E., and Theophilus, S. (2017). Environmental effects of crude oil spill on the physicochemical and hydrobiological characteristics of the Nun River, Niger Delta. Environ. Monit. Assess. 189:173. doi: 10.1007/s10661-017-5882-x
Ijabadeniyi, O., Debusho, L., Vanderlinde, M., and Buys, E. (2011). Irrigation water as a potential preharvest source of bacterial contamination of vegetables. J. Food Saf. 31, 452–461. doi: 10.1111/j.1745-4565.2011.00321.x
Imani, M., Hasan, M., Bittencourt, L., McClymont, K., and Kapelan, Z. A. (2021). novel machine learning application: Water quality resilience prediction Model. Sci. Total Environ. 768:144459. doi: 10.1016/j.scitotenv.2020.144459
Islam, S., and Repella, A. (2015). Water diplomacy: A negotiated approach to manage complex water problems. J. Contemp. Water Res. Educ. 155, 1–10. doi: 10.1111/j.1936-704x.2015.03190.x
Iyiola, A. O., Afolabi, O. A., Alimi, S. K., Akingba, O. O., Izah, S. C., and Ogwu, M. C. (2024a). “Climate change and water crisis in the global south,” in Water Crises and Sustainable Management in the Global South, eds S. C. Izah, M. C. Ogwu, A. Loukas, and H. Hamidifar (Singapore: Springer), doi: 10.1007/978-981-97-4966-9_4
Iyiola, A. O., Izah, S. C., Morya, S., Akinsorotan, A., and Ogwu, M. C. (2023a). Owalla reservoir in south-western Nigeria: Assessment of fish distribution, biological diversity, and water quality index. Indonesian J. Agric. Res. 6, 106–125. doi: 10.32734/injar.v6i2.12207
Iyiola, A. O., Kolawole, A. S., Ajayi, F. O., Ogidi, O. I., and Ogwu, M. C. (2023b). “Sustainable water use and management for agricultural transformation in Africa,” in Water Resources Management for Rural Development, eds S. Madhav, A. L. Srivastav, S. C. Izah, and E. van Hullebusch (Amsterdam: Elsevier), 287–299. doi: 10.1016/B978-0-443-18778-0.00014-3
Iyiola, A. O., Kolawole, A. S., Setufe, S. B., Bilikoni, J., Ofori, E., and Ogwu, M. C. (2024b). “Fish as a sustainable biomonitoring tool in aquatic environments,” in Biomonitoring of Pollutants in the Global South, eds S. C. Izah, M. C. Ogwu, and H. Hamidifar (Singapore: Springer), doi: 10.1007/978-981-97-1658-6_12
Izah, S., and Ineyougha, E. (2015). A review of the microbial quality of potable water sources in Nigeria. J. Adv. Biol. Basic Res. 1, 12–19.
Izah, S., Iyiola, A., and Richard, G. (2023). “Impacts of pollution on the hydrogeochemical and microbial community of aquatic ecosystems in Bayelsa State, Southern Nigeria,” in Hydrogeochemistry of Aquatic Ecosystems, eds S. Madhav, V. Singh, M. Kumar, and S. Singh (Hoboken, NJ: John Wiley and Sons Ltd), 283–305. doi: 10.1002/9781119870562.ch13
Izah, SC., Ngun, CT., and Richard, G. (2022a). Microbial quality of groundwater in the Niger Delta region of Nigeria: Health implications and effective treatment technologies. in Urban Water Crisis and Management: Strategies for Sustainable Development. Current Directions in Water Scarcity Research, eds AL Srivastav, S Madhav, and AK Bhardwaj (Amsterdam: Elsevier), 149–172. doi: 10.1016/B978-0-323-91838-1.00010-5
Izah, S. C., Aigberua, A., and Srivastav, A. (2022b). “Factors influencing the alteration of microbial and heavy metal characteristics of river systems in the Niger Delta region of Nigeria,” in Ecological Significance of River Ecosystem: Challenges and Management, eds S. Madhav, S. Kanhaiya, A. L. Srivastav, V. B. Singh, and P. Singh (Amsterdam: Elsevier), 51–78. doi: 10.1016/B978-0-323-85045-2.00005-4
Izah, S. C., Chakrabarty, S., and Srivastava, A. K. (2021a). Microbial water quality improvements through predictive modeling in Nigeria. J. Environ. Health 16, 123–135. doi: 10.1016/j.envint.2020.106841
Izah, S., Richard, G., and Sawyer, W. (2021b). Distribution of Fungi density and diversity in a Surface water of Epie Creek in Yenagoa Metropolis, Nigeria. Arch. Epidemiol. Public Health 3, 2–5. doi: 10.15761/AEPH.1000121x
Jacobsen, K., and Wiersma, S. (2010). Hepatitis A virus seroprevalence by age and world region, 1990 and 2005. Vaccine 28, 6653–6657. doi: 10.1016/j.vaccine.2010.08.037
Jutla, A., Akanda, A., Unnikrishnan, A., Huq, A., and Colwell, R. (2015). Predictive time series analysis linking bengal cholera with terrestrial water storage measured from gravity recovery and climate experiment sensors. Am. J. Trop. Med. Hyg. 93, 1179–1186. doi: 10.4269/ajtmh.14-0648
Karumathil, D., Yin, H., Kollanoor-Johny, A., and Venkitanarayanan, K. (2014). Effect of chlorine exposure on the survival and antibiotic gene expression of multidrug resistant Acinetobacter baumannii in water. Int. J. Environ. Res. Public Health 11, 1844–1854. doi: 10.3390/ijerph110201844
Kemp, S., and Nurius, P. (2015). Preparing emerging doctoral scholars for transdisciplinary research: A developmental approach. J. Teach. Soc. Work. 35, 131–150. doi: 10.1080/08841233.2014.980929
Kotchi, S., Brazeau, S., Turgeon, P., Pelcat, Y., Legare, J., Lavigne, M., et al. (2015). Evaluation of earth observation systems for estimating environmental determinants of microbial contamination in recreational waters. IEEE J. Select. Top. Appl. Earth Observ. Remote Sens. 8, 3730–3741. doi: 10.1109/jstars.2015.2426138
Kotlarska, E., Łuczkiewicz, A., Pisowacka, M., and Burzyński, A. (2014). Antibiotic resistance and prevalence of class 1 and 2 integrons in Escherichia coli isolated from two wastewater treatment plants, and their receiving waters (Gulf of Gdansk, Baltic Sea, Poland). Environ. Sci. Pollut. Res. Int. 22, 2018–2030. doi: 10.1007/s11356-014-3474-7
Kotloff, K., Nataro, J., Blackwelder, W., Nasrin, D., Farag, T., Panchalingam, S., et al. (2013). Burden and aetiology of diarrhoeal disease in infants and young children in developing countries (the Global Enteric Multicenter Study, GEMS): A prospective, case-control study. Lancet 382, 209–222. doi: 10.1016/S0140-6736(13)60844-2
Kumar, S., Tripathi, V., and Garg, S. (2012). Antibiotic resistance and genetic diversity in water-borne enterobacteriaceae isolates from recreational and drinking water sources. Int. J. Environ. Sci. Technol. 10, 789–798. doi: 10.1007/s13762-012-0126-7
Kumpel, E., Peletz, R., Bonham, M., and Khush, R. (2016). Assessing drinking water quality and water safety management in sub-saharan africa using regulated monitoring data. Environ. Sci. Technol. 50, 10869–10876. doi: 10.1021/acs.est.6b02707
Kuroki, S., Ogata, R., and Sakamoto, M. (2023). Predicting the presence of e. coli in tap water using machine learning in Nepal. Water Environ. J. 37, 402–411. doi: 10.1111/wej.12844
Leftwich, B., Opoku, S., Yin, J., and Adhikari, A. (2021). Assessing hotel employee knowledge on risk factors and risk management procedures for microbial contamination of hotel water distribution systems. Int. J. Environ. Res. Public Health 18:3539. doi: 10.3390/ijerph18073539
Li, H., Smith, C., Wang, L., Li, Z., Xiong, C., and Zhang, R. (2019). Combining spatial analysis and a drinking water quality index to evaluate monitoring data. Int. J. Environ. Res. Public Health 16:357. doi: 10.3390/ijerph16030357
Liu, Z., and Yang, H. (2018). The impacts of spatiotemporal landscape changes on water quality in Shenzhen. China. Int. J. Environ. Res. Public Health 15:1038. doi: 10.3390/ijerph15051038
Luby, S., Halder, A., Huda, T., Unicomb, L., Islam, M., Arnold, B., et al. (2015). Microbiological contamination of drinking water associated with subsequent child diarrhea. Am. J. Trop. Med. Hyg. 93, 904–911. doi: 10.4269/ajtmh.15-0274
Ma, T., Sun, S., Fu, G., Hall, J., Ni, Y., He, L., et al. (2020a). Pollution exacerbates China’s water scarcity and its regional inequality. Nat. Commun. 11:650. doi: 10.1038/s41467-020-14532-5
Ma, T., Zhao, N., Ni, Y., Yi, J., Wilson, J., He, L., et al. (2020b). China’s improving inland surface water quality since 2003. Sci. Adv. 6:eaau3798. doi: 10.1126/sciadv.aau3798
Ma, Y., Chai, Y., Xu, Y., Li, Z., and Zheng, S. (2022). Spatial and temporal changes of sand mining in the yangtze river basin since the establishment of the three gorges dam. Int. J. Environ. Res. Public Health 19:16712. doi: 10.3390/ijerph192416712
Malaj, E., Ohe, P. C., Grote, M., Kühne, R., Mondy, C. P., Usseglio-Polatera, P., et al. (2014). Organic chemicals jeopardize the health of freshwater ecosystems on the continental scale. Proc. Natl. Acad. Sci. U S A. 111, 9549–9554. doi: 10.1073/pnas.1321082111
McGinnis, S., Marini, D., Amatya, P., and Murphy, H. (2019). Bacterial contamination on latrine surfaces in community and household latrines in kathmandu. Nepal. Int. J. Environ. Res. Public Health 16:257. doi: 10.3390/ijerph16020257
Medeiros, R., and Ferreira, T. (2020). The impact of inefficient and inadequate sanitation diseases on the average cost of hospital admissions in Brazilian municipalities. Rev. Estudo Debate 27, 89–107. doi: 10.22410/issn.1983-036x.v27i2a2020.2489
Mekonnen, M., and Hoekstra, A. (2016). Four billion people facing severe water scarcity. Sci. Adv. 2:e1500323. doi: 10.1126/sciadv.1500323
Meredith, G. (2023). Facilitated asynchronous online learning to build public health strategic skills. J. Public Health Manag. Pract. 30, 56–65. doi: 10.1097/PHH.0000000000001813
Minh, H., and Hùng, N. (2011). Economic aspects of sanitation in developing countries. Environ. Health Insights 5, 63–70. doi: 10.4137/EHI.S8199
Ministry of Water Resources. (2019). Namami Gange Programme: A Comprehensive Approach to Clean Ganga. New Delhi: Government of India.
Murphy, H., Thomas, M., Schmidt, P., Medeiros, D., McFadyen, S., and Pintar, K. (2015). Estimating the burden of acute gastrointestinal illness due to Giardia, Cryptosporidium, Campylobacter, E. coli O157 and norovirus associated with private wells and small water systems in Canada. Epidemiol. Infect. 144, 1355–1370. doi: 10.1017/S0950268815002071
Murrieta-Dueñas, R., Serrano-Rubio, J. P., López-Ramírez, V., Segovia-Dominguez, I., and Cortez-González, J. (2022). Prediction of microbial growth via the hyperconic neural network approach. Chem. Eng. Res. Design 186, 525–540. doi: 10.1016/j.cherd.2022.08.021
Nefale, A., Kamika, I., Obi, C., and Momba, M. (2017). The limpopo non-metropolitan drinking water supplier response to a diagnostic tool for technical compliance. Int. J. Environ. Res. Public Health 14:810. doi: 10.3390/ijerph14070810
Ntsasa, N., Mkhohlakali, A., Mogashane, T., Tshilongo, J., and Letsoalo, M. R. (2024). Trends in systematic techniques for pollutants monitoring in the environmental water systems. London: IntechOpen. doi: 10.5772/intechopen.1007099
Nwokediegwu, Z., Ugwuanyi, E., Dada, M., Majemite, M., and Obaigbena, A. (2024). Urban water management: A review of sustainable practices in the usa. Eng. Sci. Technol. J. 5, 517–530. doi: 10.51594/estj.v5i2.829
O’Reilly, K. (2015). From toilet insecurity to toilet security: Creating safe sanitation for women and girls. Wiley Interdisciplinary Rev. Water 3, 19–24. doi: 10.1002/wat2.1122
Odiyo, J., Mathoni, M., and Makungo, R. (2020). Health risks and potential sources of contamination of groundwater used by public schools in Vhuronga 1, limpopo province, South Africa. Int. J. Environ. Res. Public Health 17:6912. doi: 10.3390/ijerph17186912
Ogura, Y., Ueda, T., Nukazawa, K., Hiroki, H., Xie, H., Arimizu, Y., et al. (2020). The level of antimicrobial resistance of sewage isolates is higher than that of river isolates in different Escherichia coli lineages. Sci. Rep. 10:17880. doi: 10.1038/s41598-020-75065-x
Ogwu, M. C. (2019). “Towards sustainable development in Africa: The challenge of urbanization and climate change adaptation,” in The Geography of Climate Change Adaptation in Urban Africa, eds P. B. Cobbinah and M. Addaney (Switzerland: Springer), 29–55. doi: 10.1007/978-3-030-04873-0_2
Ogwu, M. C., and Kosoe, E. A. (2024). “Place of cultural diversity in sustainable water resource management in Ghana,” in Water Crises and Sustainable Management in the Global South, eds S. C. Izah, M. C. Ogwu, A. Loukas, and H. Hamidifar (Singapore: Springer), doi: 10.1007/978-981-97-4966-9_14
Olugbamila, O., Adeyinka, S., Odunsi, O., Olowoyo, S., Isola, O., and Adanlawo, T. (2020). Community participation in the provision of environmental sanitation infrastructure in Akure, Nigeria. Environ. Soc. Econ. Stud. 8, 48–59. doi: 10.2478/environ-2020-0017
Onda, K., LoBuglio, J., and Bartram, J. (2012). Global access to safe water: Accounting for water quality and the resulting impact on MDG progress. Int. J. Environ. Res. Public Health 9, 880–894. doi: 10.3390/ijerph9030880
Opare, S. (2011). Sustaining water supply through a phased community management approach: Lessons from ghana’s “oats” water supply scheme. Environ. Dev. Sustainabil. 13, 1021–1042. doi: 10.1007/s10668-011-9303-y
Ottesen, A., Kocurek, B., Ramachandran, P., Reed, E., Commichaux, S., Engelbach, G., et al. (2022). Advancing antimicrobial resistance monitoring in surface waters with metagenomic and quasimetagenomic methods. PLoS Water 1:e0000067. doi: 10.1101/2022.04.22.489054
Ovuru, K., Izah, S., Ogidi, O., Imarhiagbe, O., and Ogwu, M. (2023). Slaughterhouse facilities in developing nations: Sanitation and hygiene practices, microbial contaminants and sustainable management system. Food Sci. Biotechnol. 33, 519–537. doi: 10.1007/s10068-023-01406-x
Park, J., Park, G., and Kim, S. (2013). Assessment of future climate change impact on water quality of Chung Ju lake, South Korea, using wasp coupled with swat. Jawra J. Am. Water Resour. Assoc. 49, 1225–1238. doi: 10.1111/jawr.12085
Pasika, S., and Gandla, S. (2020). Smart water quality monitoring system with cost-effective using IoT. Heliyon 6:e04096. doi: 10.1016/j.heliyon.2020.e04096
Patel, M. M., Hall, A. J., Vinjé, J., and Parashar, U. D. (2008). Noroviruses: A comprehensive review. J. Clin. Virol. 44, 1-8. doi: 10.1016/j.jcv.2008.06.002
Patel, R., Stoklosa, H., Shitole, S., Shitole, T., Sawant, K., Nanarkar, M., et al. (2013). The high cost of diarrhoeal illness for urban slum households-a cost-recovery approach: A cohort study. BMJ Open 3:e002251. doi: 10.1136/bmjopen-2012-002251
Peletz, R., Kumpel, E., Bonham, M., Rahman, Z., and Khush, R. (2016). To What extent is drinking water tested in sub-Saharan Africa? A comparative analysis of regulated water quality monitoring. Int. J. Environ. Res. Public Health 13:275. doi: 10.3390/ijerph13030275
Perelman, L., Arad, J., Housh, M., and Ostfeld, A. (2012). Event detection in water distribution systems from multivariate water quality time series. Environ. Sci. Technol. 46, 8212–8219. doi: 10.1021/es3014024
Phungela, T., Maphanga, T., Chidi, B., Madonsela, B., and Shale, K. (2022). The impact of wastewater treatment effluent on crocodile river quality in Ehlanzeni district, Mpumalanga province, south africa. S. Afr. J. Sci. 118, 1–12. doi: 10.17159/sajs.2022/12575
Piffer, P., Tambosi, L., Ferraz, S., Metzger, J., and Uriarte, M. (2021). Native forest cover safeguards stream water quality under a changing climate. Ecol. Appl. 31:e02414. doi: 10.1002/eap.2414
Posada-Perlaza, C., Ramírez-Rojas, A., Porras, P., Adu-Oppong, B., Botero-Coy, A., Hernández, F., et al. (2019). Bogotá River anthropogenic contamination alters microbial communities and promotes spread of antibiotic resistance genes. Sci. Rep. 9:11764. doi: 10.1038/s41598-019-48200-6
Posthuma, L., Munthe, J., Gils, J., Altenburger, R., Müller, C., Slobodnıìk, J., and Brack, W. (2019). A holistic approach is key to protect water quality and monitor, assess and manage chemical pollution of european surface waters. Environ. Sci. Europe 31. doi: 10.1186/s12302-019-0243-8
Poulin, C., Peletz, R., Ercumen, A., Pickering, A., Marshall, K., Boehm, A., et al. (2020). What environmental factors influence the concentration of fecal indicator bacteria in groundwater? Insights from explanatory modeling in Uganda and Bangladesh. Environ. Sci. Technol. 54, 13566–13578. doi: 10.1021/acs.est.0c02567
Prüss-Ustün, A., Bartram, J., Clasen, T., Colford, J., Cumming, O., Curtis, V., et al. (2014). Burden of disease from inadequate water, sanitation and hygiene in low- and middle-income settings: A retrospective analysis of data from 145 countries. Trop. Med. Int. Health 19, 894–905. doi: 10.1111/tmi.12329
Pugas, A. (2023). The hunt for data: Obstacles faced by researchers in the search for accurate information on precipitation in Brazil. Int. J. Hydrol. 7, 88–91. doi: 10.15406/ijh.2023.07.00344
Ramírez-Castillo, F., Loera-Muro, A., Jacques, M., Garneau, P., Avelar-González, F., Harel, J., et al. (2015). Waterborne pathogens: Detection methods and challenges. Pathogens 4, 307–334. doi: 10.3390/pathogens4020307
Richter, D., Goeppert, N., Zindler, B., and Goldscheider, N. (2021). Spatial and temporal dynamics of suspended particles and e. coli in a complex surface-water and karst groundwater system as a basis for an adapted water protection scheme, northern vietnam. Hydrogeol. J. 29, 1965–1978. doi: 10.1007/s10040-021-02356-6
Rout, A. K., Dixit, S., Dey, S., Parida, P. K., Bhattacharya, M., Pradhan, S. K., et al. (2022). “Role of modern biotechnology in the era of river water pollution,” in River Health and Ecology in South Asia, eds B. C. Patra, P. K. Shit, G. S. Bhunia, and M. Bhattacharya (Berlin: Springer), 63–79. doi: 10.1007/978-3-030-83553-8_4
Rustam, F., Ishaq, A., Kokab, S. T., de la Torre Diez, I., Mazón, J. L. V., Rodríguez, C. L., et al. (2022). An artificial neural network model for water quality and water consumption prediction. Water 14:3359. doi: 10.3390/w14213359
Sadik, N., Uprety, S., Nalweyiso, A., Kiggundu, N., Banadda, N., Shisler, J., et al. (2017). Quantification of multiple waterborne pathogens in drinking water, drainage channels, and surface water in Kampala, Uganda, during seasonal variation. Geohealth 1, 258–269. doi: 10.1002/2017GH000081
Saeidi, N., Gu, X., Tran, N., Goh, S., Kitajima, M., Kushmaro, A., et al. (2018a). Occurrence of traditional and alternative fecal indicators in tropical Urban environments under different land use patterns. Appl. Environ. Microbiol. 84:e0287–18. doi: 10.1128/AEM.00287-18
Saeidi, N., Montas, H., and Chu, M. L. (2018b). Predicting fecal indicator bacteria in urban watersheds using land use patterns and chemical tracers. Environ. Monit. Assess. 190, 1–15. doi: 10.1007/s10661-018-6965-2
Sarker, A., Dash, S., Hoque, M., Ahmed, S., and Shaheb, R. (2016). Assessment of microbial quality of water in popular restaurants in sylhet city of bangladesh. Bangladesh J. Agric. Res. 41, 115–125. doi: 10.3329/bjar.v41i1.27677
Sawyer, W. E., Izah, S. C., and Ogwu, M. C. (2023). Rural posting in public health practices: Principles and strategies. J. Commun. Med. Health Care 8, 1–10.
Scanlon, B. R., Faunt, C. C., Longuevergne, L., Reedy, R. C., Alley, W. M., McGuire, V. L., et al. (2022). Machine learning models for predicting groundwater contamination in rural Bangladesh. Water Resour. Res. 58:e2021WR031423. doi: 10.1029/2021WR031423
Scanlon, M., Gordon, J., Tonozzi, A., and Griffin, S. (2022). Reducing the risk of healthcare associated infections from legionella and other waterborne pathogens using a water management for construction (WMC) Infection control risk assessment (ICRA) tool. Infect. Dis. Rep. 14, 341–359. doi: 10.3390/idr14030039
Schijven, J., Bouwknegt, M., Husman, A., Rutjes, S., Sudre, B., Suk, J., et al. (2013a). A decision support tool to compare waterborne and foodborne infection and/or illness risks associated with climate change. Risk Anal. 33, 2154–2167. doi: 10.1111/risa.12077
Schijven, J., Hoogenboezem, W., Hassanizadeh, S. M., and Brouwer, G. (2013b). Mapping high-risk contamination areas using GIS-based microbial risk assessment models. J. Hydrol. 490, 130–140. doi: 10.1016/j.jhydrol.2013.03.036
Schoen, M., and Ashbolt, N. (2010). Assessing pathogen risk to swimmers at non-sewage impacted recreational beaches. Environ. Sci. Technol. 44, 2286–2291. doi: 10.1021/es903523q
Seiyaboh, E. I., Youkparigha, F. O., Izah, S., and Daniels, I. (2020b). Bacteriological quality of groundwater in imiringi Town, Bayelsa State, Nigeria. J. Biotechnol. Biomed. Sci. 2, 34–40.
Seiyaboh, E. I., Youkparigha, F. O., Izah, S., and Mientei, K. (2020a). Assessment of bacteriological characteristics of surface water of Taylor Creek in Bayelsa state, Nigeria. Noble Int. J. Sci. Res. 4, 25–30.
Seyoum, A., Tanyimboh, T., and Siew, C. (2013). Assessment of water quality modelling capabilities of epanet multiple species and pressure-dependent extension models. Water Sci. Technol. Water Supply 13, 1161–1166. doi: 10.2166/ws.2013.118
Sibiya, J., and Gumbo, J. (2013). Knowledge, attitude and practices (KAP) survey on water, sanitation and hygiene in selected schools in Vhembe District, Limpopo, South Africa. Int. J. Environ. Res. Public Health 10, 2282–2295. doi: 10.3390/ijerph10062282
Singh, A., Das, S., Singh, S., Pradhan, N., Gajamer, V., Kumar, S., et al. (2019). Physicochemical parameters and alarming coliform count of the potable water of eastern Himalayan State Sikkim: An Indication of severe fecal contamination and immediate health risk. Front. Public Health 7:174. doi: 10.3389/fpubh.2019.00174
Sokolova, E., Aström, J., Pettersson, T., Bergstedt, O., and Hermansson, M. (2012). Estimation of pathogen concentrations in a drinking water source using hydrodynamic modelling and microbial source tracking. J. Water Health 10, 358–370. doi: 10.2166/wh.2012.183
Soller, J., Schoen, M., Varghese, A., Ichida, A., Boehm, A., Eftim, S., et al. (2014). Human health risk implications of multiple sources of faecal indicator bacteria in a recreational waterbody. Water Res. 66, 254–264. doi: 10.1016/j.watres.2014.08.026
Su, H., Zou, R., Zhang, X., Liang, Z., Ye, R., and Liu, Y. (2022). Exploring the type and strength of nonlinearity in water quality responses to nutrient loading reduction in shallow eutrophic water bodies: Insights from a large number of numerical simulations. J. Environ. Manage 313:115000. doi: 10.1016/j.jenvman.2022.115000
Sulistyawati, S. (2020). Measuring the dengue risk area using geographic information system: A review. Insights Public Health J. 1:36. doi: 10.20884/1.iphj.2020.1.1.3012
Thompson, R., and Monis, P. (2012). Giardia–from genome to proteome. Adv. Parasitol. 78, 57–95. doi: 10.1016/B978-0-12-394303-3.00003-7
Tian, Y., Wang, J., Xie, T., Li, X., and Sun, W. (2020). Bayesian network-based probabilistic risk assessment of microbial contamination in drinking water distribution systems. J. Water Resour. Plann. Manag. 146:04020066. doi: 10.1061/(ASCE)WR.1943-5452.0001242
Tong, Y., Deng, Z., and Gang, D. (2011). Nonpoint source pollution. Water Environ. Res. 83, 1683-1703. doi: 10.2175/106143011x13075599870054
Towett, E., Drake, L., Acquah, G., Haefele, S., McGrath, S., and Shepherd, K. (2020). Comprehensive nutrient analysis in agricultural organic amendments through non-destructive assays using machine learning. PLoS One 15:e0242821. doi: 10.1371/journal.pone.0242821
T’Seole, N., Mindu, T., Kalinda, C., and Chimbari, M. (2022). Barriers and facilitators to water, sanitation and hygiene (WaSH) practices in Southern Africa: A scoping review. PLoS One 17:e0271726. doi: 10.1371/journal.pone.0271726
Turek, J., Bansal, V., Tekin, A., Singh, S., Deo, N., Sharma, M., et al. (2022). Lessons from a rapid project management exercise in the time of pandemic: Methodology for a global COVID-19 virus registry database. JMIR Res. Protoc. 11:e27921. doi: 10.2196/27921
Viau, E., Lee, D., and Boehm, A. (2011). Swimmer risk of gastrointestinal illness from exposure to tropical coastal waters impacted by terrestrial dry-weather runoff. Environ. Sci. Technol. 45, 7158–7165. doi: 10.1021/es200984b
Wang, L., and Lv, A. (2022). Identification and diagnosis of transboundary river basin water management in China and neighboring countries. Sustainability 14:12360. doi: 10.3390/su141912360
Wang, X., and Yang, W. (2019). Water quality monitoring and evaluation using remote sensing techniques in China: A systematic review. Ecosyst. Health Sustainabil. 5, 47–56. doi: 10.1080/20964129.2019.1571443
Waseem, H., Williams, M., Stedtfeld, R., and Hashsham, S. (2017). Antimicrobial resistance in the environment. Water Environ. Res. 89, 921–941. doi: 10.2175/106143017X15023776270179
Weiskerger, C., Brandão, J., Ahmed, W., Aslan, A., Avolio, L., Badgley, B., et al. (2019). Impacts of a changing earth on microbial dynamics and human health risks in the continuum between beach water and sand. Water Res. 162, 456–470. doi: 10.1016/j.watres.2019.07.006
Widmer, A., Herzog, L., Möser, A., and Ingold, K. (2019). Multilevel water quality management in the international rhine catchment area: how to establish social-ecological fit through collaborative governance. Ecology and Society, 24. doi: 10.5751/es-11087-240327
Wolf, J., Hunter, P., Freeman, M., Cumming, O., Clasen, T., Bartram, J., et al. (2018). Impact of drinking water, sanitation and handwashing with soap on childhood diarrhoeal disease: Updated meta-analysis and meta-regression. Trop. Med. Int. Health 23, 508–525. doi: 10.1111/tmi.13051
Wu, H., Zhang, H., Guo, S., Wang, X., and Li, H. (2021). Using hydrological models to trace the sources and transport of microbial contamination in a rural catchment. Hydrol. Res. 52, 893–904. doi: 10.2166/nh.2021.065
Wu, J., Song, C., Dubinsky, E., and Stewart, J. (2021). Tracking major sources of water contamination using machine learning. Front. Microbiol. 11:616692. doi: 10.3389/fmicb.2020.616692
Wu, J., Zhang, J., Tan, W., Lan, H., Zhang, S., Xiao, K., et al. (2023). Application of time serial model in water quality predicting. Comput. Mater. Continua 74, 67–82. doi: 10.32604/cmc.2023.030703
Wu, Y. (2023). Inspiring citizen science innovation for sustainable development goal 6 in water quality monitoring in China. Front. Environ. Sci. 11:1234966. doi: 10.3389/fenvs.2023.1234966
Xu, K., Valeo, C., He, J., and Xu, Z. (2019). Climate and land use influences on bacteria levels in stormwater. Water 11:2451. doi: 10.3390/w11122451
Zhang, C., and Wang, M. A. (2022). Study on the evaluation of the public health governance in countries along the belt and road initiative (BRI). Int. J. Environ. Res. Public Health 19:14993. doi: 10.3390/ijerph192214993
Keywords: microbial contamination, predictive modeling, public health, environmental health, waterborne diseases, water safety, sustainable water management
Citation: Izah SC and Ogwu MC (2025) Modeling solutions for microbial water contamination in the global south for public health protection. Front. Microbiol. 16:1504829. doi: 10.3389/fmicb.2025.1504829
Received: 01 October 2024; Accepted: 06 March 2025;
Published: 02 April 2025.
Edited by:
Jesus L. Romalde, University of Santiago de Compostela, SpainReviewed by:
Ajaya Kumar Rout, Rani Lakshmi Bai Central Agricultural University, IndiaCopyright © 2025 Izah and Ogwu. This is an open-access article distributed under the terms of the Creative Commons Attribution License (CC BY). The use, distribution or reproduction in other forums is permitted, provided the original author(s) and the copyright owner(s) are credited and that the original publication in this journal is cited, in accordance with accepted academic practice. No use, distribution or reproduction is permitted which does not comply with these terms.
*Correspondence: Matthew Chidozie Ogwu, b2d3dW1jQGFwcHN0YXRlLmVkdQ==
Disclaimer: All claims expressed in this article are solely those of the authors and do not necessarily represent those of their affiliated organizations, or those of the publisher, the editors and the reviewers. Any product that may be evaluated in this article or claim that may be made by its manufacturer is not guaranteed or endorsed by the publisher.
Research integrity at Frontiers
Learn more about the work of our research integrity team to safeguard the quality of each article we publish.