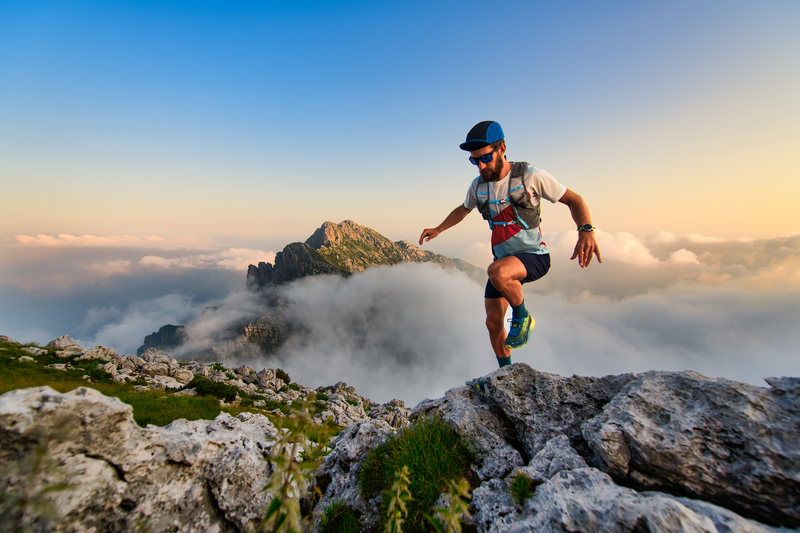
95% of researchers rate our articles as excellent or good
Learn more about the work of our research integrity team to safeguard the quality of each article we publish.
Find out more
ORIGINAL RESEARCH article
Front. Microbiol. , 15 January 2025
Sec. Microorganisms in Vertebrate Digestive Systems
Volume 15 - 2024 | https://doi.org/10.3389/fmicb.2024.1521719
Introduction: Diarrhea is a prevalent disease among calves, which significantly hinders their growth and development, thereby impacting farm productivity and revenue. This study aimed to investigate the impact of diarrhea on calf growth.
Methods: Holstein male calves with similar birth weight (39.5 ± 4.2 kg) were included in this study, and key parameters such as fecal score, diarrhea incidence, and growth performance from birth to weaning were measured. Rectal fecal samples from both diarrheic (n = 24) and healthy calves (n = 24) aged 1–4 weeks were analyzed using 16S rRNA gene sequencing and untargeted metabolomics.
Results: Our findings indicated a high prevalence of diarrhea among calves between 1–4 weeks of age on pasture, which led to a marked decrease in growth performance, including average daily gain. At the genus level, the relative abundance of GCA-900066575 in one-week-old diarrheic calves was significantly higher; Escherichia-Shigella and Pseudoflavonifractor were more abundant in two-week-old calves; while Tyzzerella and Lachnospiraceae_UCG-004 increased significantly in four-week-old calves, and correlated negatively with average daily gain, suggesting that these bacteria may promote the occurrence of diarrhea. Correlation analysis revealed that fecal metabolites such as arachidonic acid, cis-vaccenic acid, oleic acid, choline, creatinine, and others were significantly negatively correlated with calf growth performance and were significantly increased in diarrheic calves. WGNCA identified that dark magenta module metabolites were significantly associated with diarrhea traits from 1–4 weeks. Thirteen metabolites, including glycerophospholipids (such as 1-stearoyl-2-hydroxy-sn-glycero-3-phosphoethanolamine), fatty acids (such as dodecanoic acid), and arachidonic acid, were positively correlated with GCA-900066575, Escherichia-shigella, Tyzzerella, and Clostridium_butyricum, but negatively correlated with UBA1819, Lachnoclostridium_sp_YL32, and Clostridium_scindens.
Discussion: Therefore, GCA-900066575, Escherichia-shigella, Lachnospiraceae_UCG-004, and Tyzzerella are likely key bacterial genera causing diarrhea in calves, while arachidonic acid, glycerol phospholipids, and fatty acids are critical metabolites associated with this condition. These alterations in the fecal microbiota and metabolite composition were found to be the principal contributors to growth retardation in diarrheic calves.
Neonatal calf diarrhea remains a predominant cause of morbidity and mortality, contributing to over 50% of total calf deaths (Cho and Yoon, 2014). This condition severely compromises calf welfare and incurs substantial economic losses within the cattle industry (Pempek et al., 2019). The primary etiological factor is infection by intestinal pathogens, attributed to the immature development of the calf’s gut and its vulnerability to pathogen invasion, which might lead to diarrhea (Cho and Yoon, 2014). Pathogen proliferation in the gastrointestinal tract diminishes beneficial bacteria populations, with pathogens such as Escherichia coli, bovine rotavirus, bovine coronavirus, and Cryptosporidium spp. being closely implicated in neonatal calf diarrhea (Brunauer et al., 2021). Intestinal damage further exacerbates permeability, allowing pathogens to breach the intestinal immune barrier and trigger inflammatory response, ultimately impairing calf growth and development (Ying et al., 2013).
Recent studies have revealed the significance of integrating microbiome and metabolome data to elucidate host–microbe interactions and identify predictive biomarkers for disease (Visconti et al., 2019). Metabolites offer a comprehensive view of metabolic alterations in the gut, enhancing our understanding of the mechanisms by which intestinal dysbiosis precipitates the emergence of diarrhea. Amin et al. (2023) demonstrated a robust correlation between fecal microorganisms, plasma metabolites, and calf growth performance from 42 to 98 days postpartum.
Growing evidence has indicated a significant relationship between gut microbiota and animal health (Esser et al., 2019). For instance, diarrheic calves have been described to exhibit reduced Bacteroides levels and elevated Proteobacteria levels in their feces (Kim E. T. et al., 2021). Moreover, it is known that the gut microbiota of healthy calves promotes the maturation of the intestinal immune system by the production of beneficial metabolites such as short-chain fatty acids (SCFAs) (Shi et al., 2017). For instance, Bacteroides levels are linked to SCFA regulation (Horvath et al., 2022), which in turn stimulates bicarbonate secretion in the intestine (Dohgen et al., 1994). Shi et al. (2023) suggested that calf diarrhea might be associated with gut microbiota imbalance affecting acid–base homeostasis and highlighted the role of microbiota-driven disruptions in purine and arachidonic acid metabolism. Moreover, infection with bovine rotavirus and coronavirus has been shown to alter fecal microbiota composition and metabolites (Cui et al., 2023). Therefore, targeted modulation of the gut microbiota constitutes a potential strategy for preventing and treating calf diarrhea (Shi et al., 2023). However, despite the established link between altered gut microbiota and diarrhea, the microbial and metabolic mechanisms connecting diarrhea to hindered growth and development in calves remain poorly understood.
In the present study, using 16S rRNA gene sequencing and non-targeted metabolomics, differences in fecal metabolites and microbiota between healthy and diarrheic calves aged 1–4 weeks were investigated. The relationship between changes in the intestinal microbiota and metabolites and growth retardation in diarrheic calves was investigated.
Calves included in the present study were sourced from a large-scale dairy farm in Lingwu, Ningxia, China. Sixty one-day-old Holstein male calves showing similar body weight at birth (39.5 ± 4.2 kg, n = 60) were selected and managed according to the farm’s standard feeding protocols. Throughout the trail period, which lasted from birth to weaning for 60 days, calves were isolated from other cows and housed individually on calf islands. No treatments were administered other than routine umbilical cleaning.
Within 6 h of birth, each calf received 2.0 L of colostrum (2.0 L after 2 h and 2.0 L after 4–6 h of birth). On the second day, calves were moved to calf islands and fed regular milk (EKOMILK Bond, Europe) whose composition is detailed in Supplementary Table S1 at a rate of 4 L/day in two feedings. By day 30, daily milk intake was gradually increased to 6 L/day and maintained at this level until weaning on day 60. Clean, fresh water was available from day 3, and hay and calf concentrate supplements (COFCO Feeds, Yinchuan Plant Co., Ltd.) (Supplementary Table S2) were provided from day 7. Fecal scores, color, and status were recorded using a three-point scale (0 = normal feces; 1 = semi-formed feces; 2 = loose feces; 3 = watery feces) at the beginning of the experiment. A diarrhea group and a healthy group were formed to monitor diarrhea incidence calculated based on the below formula.
Feed intake, body length, and size were recorded from birth to weaning (see Supplementary Table S3 for measurement indicators). Calves that were medicated, had other diseases and died during the trial were eliminated.
The study was divided into periods of 4 weeks (week 1, week 2, week 3, and week 4) and based on diarrhea incidence. Each week calves were grouped into diarrhea and healthy groups: (i) D1W (diarrhea during week 1, n = 6) and H1W (healthy during week 1, n = 6); (ii) D2W (diarrhea during week 2 and no diarrhea on week 1, n = 6), H2W (healthy during week 2, n = 6); (iii) D3W (diarrhea during week 3 and no diarrhea on weeks 1 and 2, n = 6) and H3W (healthy during week 3, n = 6); (iv) D4W (diarrhea during week 4 and no diarrhea on weeks 1 to 3, n = 6,) and H4W (healthy during week 4, n = 6). Fifty grams of fecal content were collected from both diarrheic (n = 24) and healthy calves (n = 24) by rectal stimulation using a sterile glove (Figure 1). Samples were placed in frozen storage tubes, quickly transferred to liquid nitrogen tanks, and stored at −80°C. The morphology of fecal samples was observed and recorded as described earlier. Supplementary Table S4 provides details on sample groups and collection methods.
Fecal samples collected from the 48 calves were analyzed using LS-MS untargeted metabolomics sequencing. All sample scans were performed on an LC–MS system according to the manufacturer’s instructions. Samples were separated by ultra-high performance liquid chromatography (Agilent 1290 Infinity LC, China) using a hydrophilic interaction liquid chromatography column. To mitigate the influence of fluctuations in the instrument detection signal, samples were analyzed continuously in random order. Quality control samples were included to monitor system stability and ensure reliability of experimental data.
A mass spectrometer (AB Triple TOF 6600, Basao, China) was used to collect primary and secondary spectra. We used a combination of both positive ion mode (POS) and negative ion mode (NEG) ionization modes in our experiments, which resulted in better metabolite coverage and detection. Data were analyzed using principal component analysis (PCA) (Warnes, 2007) and orthogonal partial least squares discriminant analysis (OPLS-DA) (Thevenot, 2016) using the R package gmodels. We combined multivariate statistical analysis of OPLS-DA for VIP values and univariate statistical analysis of p-values to screen for significantly differentially enriched metabolites between different comparison groups (Saccenti et al., 2014). Thresholds for significant differences were considered based on VIP values ≥1 and p-values <0.05 in the OPLS-DA model. Metabolic function and metabolic pathway analyses of differential metabolites were performed using the KEGG database. Untargeted metabolomics sequencing was carried out by Baseo, Guangzhou, China.
Fecal samples from the 48 calves were analyzed using 16S rRNA gene sequencing. Microbial DNA was extracted using HiPure Soil DNA kits (or HiPure Stool DNA kits) (Magen, Guangzhou, China) according to the manufacturer’s instructions. Samples were stored at −80°C in a refrigerator until library preparation and sequencing could be performed. Amplification of the V3–V4 hypervariable region of the 16 s rRNA gene was performed using an Illumina sequencing platform with index-binding primer pairs 341F (5’-CCTACGGGGNGGCWGCAG-3′) and 806R (5’-GGACTACHVGGGTATCTAAT-3′) (Guo et al., 2017). Agarose gel electrophoresis was performed to verify amplicon sizes. Library quality was assessed using ABI StepOnePlus Real-Time PCR System (Life Technologies, Foster City, United States). Libraries were sequenced on a Novaseq 6000 platform generating 2 × 250 bp paired-end reads. Second-round amplification products were purified using AMPure XP Beads, quantified using the ABI StepOnePlus Real-Time PCR System (Life Technologies, United States), and sequenced on-line according to the PE250 pattern pooling of the Novaseq 6000 platform.
Four distance metrics were calculated based on operational taxonomic unit (OTU) abundance tables. For alpha- and beta-diversity analyses, OTU representative sequences were first aligned using Muscle (version 3.8.31) software (Edgar, 2004), and comparison files were imported into FastTree (version 2.1) software (Price et al., 2010) to construct a phylogenetic tree. Unweighted and weighted UniFrac distance analyses were then performed using the GuniFrac package (version 1.0) (Lozupone and Knight, 2005) in R. To assess alpha-diversity, indices were conducted using QIIME (version 1.9.1) software (Caporaso et al., 2010). Jaccard and Bray-Curtis distance matrices were calculated using R project Vegan package (version 2.5.3) (Oksanen, 2010). Functional annotation of bacterial KEGG pathways was performed using PICRUSt (version 2.1.4) software (Langille et al., 2013), and the abundance of information for each pathway was quantified. 16S sequencing was carried out by Baseo, Guangzhou, China.
WGCNA analysis was performed using the Kidio Cloud platform.1 Low-expression metabolites were filtered out before importing metabolite expression data into R. Co-expression modules were generated using default network construction settings, with a power value of 8 and a minimum module size of 50. The weighted co-expression network model was constructed with “diarrhea” and “health” as traits. KEGG enrichment analysis was applied to metabolites in each module to determine the biological functions associated with the identified co-expression clusters.
Spearman correlation coefficients between fecal microbial species, fecal metabolites, and growth performance traits were calculated using the R language psych package (version 1.8.4). Heatmaps were generated using the pheatmap package to visualize the relationships between fecal metabolomes and 16S rRNA profiles in diarrheic and healthy calves. Data were expressed as mean ± standard deviation, and Welch’s t-test or Wilcoxon rank sum test was used to compare phenotypic differences between groups. Growth performance data were analyzed for significance of differences by one-way ANOVA and t-tests using GraphPad Prism (version 9.3.0) software. All statistical tests were two-tailed, and significance was set at p < 0.05. * indicates p < 0.05 for significant difference, ** indicates p < 0.01, *** indicates p < 0.001, and **** indicates p < 0.0001 for highly significant difference.
Body weight and various growth performance metrics of diarrheic calves were measured, and diarrhea incidence from birth to weaning (1–60 days) was calculated based on fecal scores. Data revealed that the third week (W) was the peak period for the occurrence of pre-weaning calf diarrhea (Figure 2A), with 79.01% of cases occurring between weeks 1 to 4 (Figure 2B).
Figure 2. Diarrhea rate and growth performance of calves. Diarrhea rate of 1–10 w in newborn calves (A). Statistical table of the pie charts (B). Growth performance indices of D1W calves at 14 d (C,G). Growth performance indices of D2W calves at 21 d (D,H). Growth performance indices of D3W calves at 28 d (E,I). Growth performance indices of D4W calves at 36 d (F,J). *p < 0.05,**p < 0.01.
Growth performance indicators for calves aged 1–4 W, measured within 1 week post-diarrhea, included body weight (BW), average daily weight gain, withers height, chest circumference, hip cross height, hip height, hip width, body length, rump length, hucklebone width, and cannon bone circumference (Figures 2C–J). On day 14, D1W calves had significantly lower average daily weight gain and chest circumference compared to H1W calves (Figures 2C,G, p < 0.05). On day 21, BW, average daily weight gain, chest circumference, hip cross height, and body length of D2W calves were significantly lower than those of H2W calves (Figures 2D,H, p < 0.05). On day 28, D3W calves exhibited significantly lower average daily weight gain, body length, and cannon bone circumference compared to H3W calves (Figures 2E,I, p < 0.05). On day 36, BW, average daily weight gain, withers height, chest circumference, and hip height of D4W calves were significantly lower than those of H4W calves (Figures 2F,J, p < 0.05).
Fecal samples from diarrheic and healthy calves were analyzed using 16S rRNA sequencing. Chao1 (Figure 3A), Shannon (Figure 3B), and Simpson (Figure 3C) indices revealed lower microbial diversity in 1- to 4-week-old diarrheic calves compared to healthy calves. Principal coordinate analysis (PCoA) using the Bray-Curtis distance revealed significant differences in beta-diversity in fecal microbial communities between diarrheic and healthy calves (Figures 3D–G).
Figure 3. Differences in rectal microbiota between healthy and diarrheal calves. Chao 1 index (A). Shannon index (B). Simpsons index (C). PCoA-analysis of D1W and H1W fecal flora of calves (D). PCoA-analysis of D2W and H2W fecal flora of calves (E). PCoA-analysis of D3W and H3W fecal flora of calves (F). PCoA-analysis of D4W and H4W fecal flora of calves (G). Histogram shows the top 10 relative abundances of the phylum in this taxa (H). Histogram shows the top 10 relative abundances of the genus in this taxa (I).
At the phylum level, Firmicutes, Proteobacteria, and Bacteroidota were predominant in both groups, with Firmicutes being the most abundant, accounting for 52.14 and 50.30% of the total microbial population in diarrheic and healthy calves, respectively (Figure 3H). Specifically, Firmicutes were more abundant in D1W calves than that in H1W calves, whereas Bacteroidota were less abundant. The abundance of Proteobacteria was significantly higher in the feces of D2W, D3W, and D4W calves compared to H2W, H3W, and H4W calves.
At the genus level, Bacteroides, Faecalibacterium, Escherichia-shigella, and Lactobacillus were the dominant genera in the fecal microbiota of both diarrheic and healthy calves (Figure 3I). Escherichia-shigella was the primary genus in D1W and D2W calves, accounting for 32.83 and 16.17% of total bacteria in the gut, respectively. It was also the main genus in H1W calves, comprising 30.5% of the total gut microbiota. Conversely, Lactobacillus was the most dominant genus in D3W and D4W calves, accounting for 17.68 and 22.80% of total bacteria in the gut, respectively (Figure 3I). Faecalibacterium was the predominant genus in H2W, H3W, and H4W calves, representing 24.08, 23.38, and 17.82% of the bacterial population in the gut, respectively (Figure 3I).
The Wilcoxon rank sum test was used to analyze differences in the fecal microbiota of 1–4 W diarrheic and healthy calves. At the phylum level, Firmicutes were significantly increased in D1W compared to H1W calves (Figure 4A, p < 0.05), while Proteobacteria were significantly higher in D2W calves (Figure 4B, p < 0.05). Compared with H3W, Proteobacteria in the fecal microbiota of D3W calves were also significantly higher (Figure 4C, p < 0.05). No significant change was found in the fecal microbiota of D4W calves compared to H4W (p > 0.05).
Figure 4. Significant differences in rectal flora between healthy and diarrheal calves. Wilcoxon rank sum test of D1W and H1W fecal flora of calves at Phylum level (A). Wilcoxon rank sum test of D2W and H2W fecal flora of calves at the Phylum level (B). Wilcoxon rank sum test of D4W and H4W fecal flora of calves at the Phylum level (C). Wilcoxon rank sum test of D1W and H1W fecal flora of calves at genus level (D). Wilcoxon rank sum test of D2W and H2W fecal flora of calves at genus level (E). Wilcoxon rank sum test of D4W and H4W fecal flora of calves at genus level (F).LEfSE analysis of fecal flora of D1W and H1W, D2W and H2W, D3W and H3W, D4W and H4W calves (G).
At the genus level, UBA1819 in the fecal microbiota of D1W calves was significantly decreased and GCA-900066575 was significantly increased compared with H1W (Figure 4D, p < 0.05). Compared to H2W, Escherichia-shigella was found in significantly higher amounts in the fecal microbiota of D2W calves while Pseudoflavonifractor was found in significantly lower amounts (Figure 4E, p < 0.05). No significant changes were found in the fecal microbiota of D3W and H3W calves (p > 0.05). Tyzzerella and Lachnospiraceae_UCG-004 in the fecal microflora of D4W calves were found significantly increased compared to H4W, while Butyricimonas, Eubacterium, and Flavonifractor were significantly decreased (Figure 4F, p < 0.05).
LEfSE analysis revealed that Lachnoclostridium_sp_YL32 had the highest LDA score in the fecal microbiota of H1W calves (LDA = 3.68, Figure 4G, p < 0.05), whereas Firmicutes had the highest LDA score in the fecal microbiota of D1W calves (LDA = 4.87, Figure 4G, p < 0.05). In H2W calves, Clostridium_asparagiforme_DSM_15981 had the highest LDA score (LDA = 4.52, Figure 4G, p < 0.05), whereas Escherichia_shigella had the highest LDA score in D2W calves (LDA = 4.62, Figure 4G, p < 0.05). Bifidobacterium_pseudocatenulatum_DSM_20438_JCM_ 1200_LMG_10505 had the highest LDA score in H3W calves (LDA = 4.18, Figure 4G, p < 0.05), whereas Proteobacteria showed the highest LDA score in D3W calves (LDA = 5.07, Figure 4G, p < 0.05). Butyricimonas had the highest LDA score in H4W calves (LDA = 3.59, Figure 4G, p < 0.05), whereas Tyzzerella had the highest LDA score in D4W calves (LDA = 3.70, Figure 4G, p < 0.05).
Thus, Escherichia_shigella, Tyzzerella, and Lachnospiraceae_UCG-004 and other differential microorganisms were significantly increased in diarrheic calves, suggesting that these microorganisms may be key contributors to promoting diarrhea. In contrast, Eubacterium_coprostanoligenes_group, Pseudoflavonifractor, Butyricimonas, and Flavonifractor were more prevalent in healthy calves and were found significantly reduced in diarrheic calves, indicating that these microorganisms may promote intestinal health and help prevent diarrhea in calves.
KEGG pathway enrichment analysis revealed the enrichment of several pathways, including C5-branched dibasic acid metabolism, biotin metabolism, citrate cycle (TCA cycle), and lipopolysaccharide biosynthesis in both D1W and H1W calves (Figure 5A, p < 0.05). Moreover, pathways including aminoacyl-tRNA biosynthesis, lysine biosynthesis, cysteine and methionine metabolism, and histidine metabolism were significantly enriched in both D2W and H2W calves, but significantly reduced in D2W calves compared to H2W calves (Figure 5B, p < 0.05). Additionally, bacterial invasion of epithelial cells and shigellosis pathways were notably increased in D2W calves compared to H2W calves (Figure 5B, p < 0.05). In D3W and H3W calves, RNA polymerase, caprolactam degradation, and carotenoid biosynthesis were enriched, with RNA polymerase and carotenoid biosynthesis pathways significantly reduced in D3W calves compared to H3W calves, with caprolactam degradation increased (Figure 5C, p < 0.05). Moreover, non-homologous end-joining and carotenoid biosynthesis were enriched, but significantly reduced in D4W calves compared with H4W calves (Figure 5D, p < 0.05).
Figure 5. Functional analysis of intestinal microbiota difference between healthy and diarrheal calves. KEGG enrichment analysis of fecal metabolites in D1W and H1W calves (A). KEGG enrichment analysis of fecal metabolites in D2W and H2W calves (B). KEGG enrichment analysis of fecal metabolites in D3W and H3W calves (C). KEGG enrichment analysis of fecal metabolites in D4W and H4W calves (D).
Spearman correlation analysis was used to explore the relationship between differential fecal microbes and growth performance traits in calves at various time points during pre-weaning. The findings revealed that the average daily weight gain of D1W and H1W calves was significantly negatively associated with the relative abundances of GCA-900066575 and Firmicutes, and significantly positively associated with Morganellaceae (Figure 6A, p < 0.05). For D2W and H2W calves, body weight and height were significantly negatively correlated with Enterobacteriaceae, Escherichia_shigella, Gammaproteobacteria, Proteobacteria, and Clostridium_butyricum (Figure 6B, p < 0.05), but significantly positively correlated with Clostridium_asparagiforme_DSM_ 15981, Burkholderiales, and Allobaculum (Figure 6B, p < 0.05). In D3W and H3W calves, BW, chest circumference, and withers height were significantly negatively correlated with Proteobacteria, Gammaproteobacteria, Enterococcus_cecorum, and Methylobacterium_sp_PC3044, but were significantly positively correlated with Bifidobacterium_pseudocatenulatum_DSM_20438 (Figure 6C, p < 0.05). For D4W and H4W calves, growth performance was significantly negatively correlated with Tyzzerella, Anaerotignum_lactatifermentans, Gallibacterium_genomosp_3, phascolarctobacterium_succinatutens_YIT_12067 and Lachnospiraceae_UCG-004, but significantly positively correlated with Butyricimonas, Oscillospiraceae, and Lachnoclostridium_sp_YL32 (Figure 6D, p < 0.05). Moreover, the relative abundance of GCA-900066575, Escherichia_shigella, Enterococcus_cecorum, and Tyzzerella were significantly negatively correlated with calf growth performance, suggesting that these bacterial groups may inhibit growth in diarrheic calves.
Figure 6. Relationship between fecal bacterial of calves with diarrhea and growth performance after 1 week of birth. Correlation of differential fecal bacterial in diarrheic calves at 1st week of age with 14 d growth performance of calves (A). Correlation of differential fecal bacterial in diarrheic calves at 2 weeks of age with 21 d of calf growth (B). Correlation of differential fecal bacterial in diarrheic calves at 3 weeks of age with calf growth at 28 d of age (C). Correlation of differential fecal bacterial in diarrheic calves at 4 weeks of age with calf performance at 36 d (D). *p < 0.05, **p < 0.01, ***p < 0.001.
OPLS-DA was used to analyze fecal metabolites of 1–4 W diarrheic and healthy calves. The results of PCA plots (Supplementary Figures S2A–D) and OPLS-DA plots (Supplementary Figures S2I–L) indicated significant differences in fecal metabolites between the two groups. A Q2 regression line generated with alignment tests (Supplementary Figures S2I–L), demonstrating that the OPLS-DA model provided a good fit for distinguishing the fecal metabolite profiles of diarrheic and healthy calves.
A differential analysis of fecal metabolites between 1 and 4 W diarrheic and healthy calves revealed 136 differential metabolites in D1W versus H1W calves, of which 49 were up-regulated and 87 were down-regulated (Figure 7A; Supplementary Table S5, VIP > 2, p < 0.05). For D2W versus H2W, 182 metabolites were differentially expressed, of which 76 were up-regulated and 106 down-regulated (Figure 7B; Supplementary Table S6, VIP > 2, p < 0.05). A total of 156 differential metabolites were found between D3W and H3W calves, of which 54 up-regulated and 102 down-regulated (Figure 7C; Supplementary Table S7, VIP > 2, p < 0.05). Finally, for D4W and H4W calves, 172 differential metabolites were found (Figure 7D; Supplementary Table S8, VIP > 2, p < 0.05), of which 102 up-regulated and 70 down-regulated.
Figure 7. Differential expression of metabolites in feces between healthy and diarrheal calves. Volcano maps of differential metabolites in D1W and H1W calf feces (A). Volcano maps of differential metabolites in D2W and H2W calf feces (B). Volcano maps of differential metabolites in D3W and H3W calf feces (C). Volcano maps of metabolite differences in D4W and H4W calf feces (D). Bubble diagram of KEGG metabolic pathways with significant enrichment of differential metabolites in D1W and H1W calf feces (E). Bubble diagram of KEGG metabolic pathways with significant enrichment of differential metabolites in D2W and H2W calf feces (F). Bubble diagram of KEGG metabolic pathways with significant enrichment of differential metabolites in D3W and H3W calf feces (G). Bubble diagram of KEGG metabolic pathways with significant enrichment of differential metabolites in D4W and H4W calf feces (H).
KEGG enrichment analysis was performed on differential metabolites for selected calf fecal samples. It was found that metabolites from D1W and H1W fecal samples were significantly enriched in pathways related to amino sugar and nucleotide sugar metabolism, fatty acid biosynthesis, phosphotransferase system (PTS), glycerophospholipid metabolism, two-component system, cutin, suberine and wax biosynthesis, biosynthesis of unsaturated fatty acids, cysteine and methionine metabolism, and other metabolic pathways (Figure 7E, p < 0.05). Metabolites from D2W and H2W calves were significantly enriched in pathways related to fatty acid biosynthesis, eicosanoids, and taurine and hypotaurine metabolism (Figure 7F, p < 0.05). Differential metabolites in D3W and H3W calves were significantly enriched in pathways related to ethylbenzene degradation, amino sugar and nucleotide sugar metabolism, degradation of aromatic compounds (Figure 7G, p < 0.05). Moreover, differential metabolites in D4W and H4W calves were significantly enriched in pathways related to fatty acid biosynthesis, caffeine metabolism, glycerophospholipid metabolism, choline metabolism in cancer, cutin, suberine and wax biosynthesis, ABC transporters, pathogenic E. coli infection, eicosanoids, glycine, serine and threonine metabolism, alcoholism, biosynthesis of unsaturated fatty acids, aminoacyl-tRNA biosynthesis, and prolactin signaling pathway (Figure 7H, p < 0.05).
In total, 136, 182, 156, and 172 fecal metabolites were identified between D1W versus H1W, D2W versus H2W, D3W versus H3W, and D4W versus H4W, respectively, which could be considered key metabolites contributing to the onset of diarrhea in calves, and may serve as biomarkers for the condition.
Spearman correlation analysis revealed significant relationships between fecal differential metabolites and growth performance traits (e.g., BW, average daily gain, body height, chest circumference, cross section height, and body slant length) in 1–4 W diarrheic calves (Figure 8). The results showed that the average daily gain of diarrheic calves on the first week of birth was significantly positively correlated with acridone, pantothenate, guanidinoethyl sulfonate, and pyruvate (Figure 8A, p < 0.05), but significantly negatively correlated with arachidonic acid, 1, 2-dioleoyl-SN-glycero-3-PC, and cis-vaccenic acid (Figure 8A, p < 0.05). On the second week, chest circumference and body length of diarrheic calves were significantly negatively correlated with arachidonic acid, oleic acid, pantothenate and cis-vaccenic acid, choline, creatinine, palmitic acid, His-Ser, glycerol 3-phosphate, α-ergocryptine and 1-stearoyl-sn-glycerol 3-phosphocholine [LPC(18:0)] (Figure 8B, p < 0.05), but significantly positively correlated with butanoic acid, acridone, cholesteryl sulfate, His-Ser, saccharin, pantothenate, and glycerol 3-phosphate (Figure 8B, p < 0.05). Growth performance of diarrheic calves on the third week of birth was significantly negatively correlated with choline, LPC (18:0), arachidonic acid, 1-stearoyl-2-hydroxy-sn-glycero-3-PE, gamma-muricholic acid, α-ergocryptine, and creatinine (Figure 8C), while average daily weight gain and body length were significantly positively correlated with butanoic acid, biphenylindanone A, pantothenate, and valeric acid (Figure 8C, p < 0.05). Finally, on the fourth week of birth, average daily weight gain, withers height, and hip height of diarrheic calves were significantly negatively correlated with Cis-vaccenic acid, oleic acid, choline, fahfa 36:1, palmitic acid, LPC (18:0), α-ergocryptine and creatinine (Figure 8D, p < 0.05), while growth performance was significantly positively correlated with sphingosine, saccharin, cholesteryl sulfate, deoxycholic acid, His-Ser and Ile-Pro (Figure 8D).
Figure 8. Relationship between fecal metabolites and growth performance of calves with diarrhea. Correlation between differential fecal metabolites of D1W and H1W calves and 14d growth performance of calves (A). Correlation between D2W and H2W calf fecal differential metabolites and 21-day growth performance of calves (B). Correlation between D3W and H3W calf fecal differential metabolites and 28-day growth performance of calves (C). Differential fecal metabolites of D4W and H4W calves were correlated with 36-day growth performance of calves (D). *p < 0.05, **p < 0.01, ***p < 0.001.
Thus, it was found that the above fecal metabolites were positively correlated with growth performance traits in calves during the first 28 days birth. In contrast, metabolites such as arachidonic acid, Cis-vaccenic acid, choline, creatinine, α-ergocryptine, and LPC (18:0) were negatively correlated with growth performance in calves. Hence, these metabolites may be considered key factors promoting diarrhea and hindering growth in calves during the first 28 days post-birth.
WGCNA was performed on fecal metabolites of 1–4 W diarrheic and healthy calves, and four groups of data were created considering diarrheic and healthy experimental groups, with diarrhea (D) and health (H) as traits.
A total of 15,728 metabolites were identified in the original data. Using a selected power of 8, a weighted co-expression network model was constructed. These 15,728 metabolites were categorized into 20 modules, with the gray module consisting of metabolites that could not be assigned to any specific group (Figure 9A). Spearman correlation algorithm was used to calculate the correlation coefficients and p-values between characteristic metabolites and disease traits, generating a correlation heat map. The results indicated that the darkmagenta module was significantly positively correlated with disease traits (Figure 9B, p < 0.05). Cluster analysis of the metabolite expression within the module revealed substantial differences in fecal metabolite expression between diarrheic and healthy calves (Figure 9C). Furthermore, the regulatory network diagram of the darkmagenta module revealed fecal metabolites such as fluazifop-butyl, dehydrocurvularin, and 4′,5-dihydroxy-7-methoxyflavanone as hub components (Figure 9D). KEGG enrichment analysis of fecal metabolites from this module revealed significant enrichment in pathways related to protein digestion and absorption, aminoacyl-tRNA biosynthesis, and lysine biosynthesis (Figure 9E).
Figure 9. WGCNA analysed the changes of fecal metabolites of diarrheal and healthy cows. Weighted co-expression network (A). Metabolite module association heat map (B). Correlation heat map of character modules (C). Metabolite regulatory network map (D). KEGG enrichment analysis (E). Venn diagrams (F).
Furthermore, a Venn diagram comparing differential fecal metabolites in the darkmagenta module and 1–4 W diarrheic calves was drawn. In total, 37 fecal metabolites were commonly found in 1 W diarrheic and healthy calves; 53 in 2 W calves; 35 in 3 W calves; and 48 in 4 W calves. Considering these, it was found that 13 differential fecal metabolites were consistently shared across all group comparisons (Figure 9F; Table 1).
Table 1. Common metabolites of the darkmagenta module with diarrhoea and healthy calves at 1–4 weeks of age.
Subsequently, KEGG enrichment analysis of these 13 fecal metabolites revealed that, compared with the whole metabolome, these metabolites were mainly significantly enriched in fatty acid biosynthesis, linoleic acid metabolism, amoebiasis, and GnRH signaling pathway. These findings suggest that these 13 differential fecal metabolites may play a key role in promoting diarrhea in calves.
Spearman correlation analysis was performed to explore the relationship between fecal microorganisms in diarrheic calves and the 13 common differential metabolites identified using WGCNA. The results showed that Firmicutes, Actinomycetaceae, GCA-900066575 and Phascolarctobacterium_succinatutens_YIT_12067 in D1W vs. HIW calves were positively correlated with the identified 13 differential fecal metabolites, while Lachnoclostridium_sp_YL32, Morganellaceae, Candidatus_hamiltonella and UBA1819 were negatively correlated (Figure 10A). Clostridium_asparagiforme_DSM_15981, Allobaculum, Burkholderiales and Pseudoflavonifractor in D2W vs. H2W calves were negatively correlated with the 13 fecal metabolites, while Escherichia-shigella, Enterobacteriaceae, Gammaproteobacteria, Proteobacteria and Clostridium_butyricum were positively correlated (Figure 10B). Bifidobacterium_pseudocatenulatum_DSM_20438 in D3W vs. H3W calves was negatively correlated with these 13 fecal metabolites, while Proteobacteria, Gammaproteobacteria, Methylobacterium_sp_PC3044 and Enterococcus_cecorum were positively correlated (Figure 10C). Butyricimonas, Oscillospiraceae, and Eubacterium_coprostanoligenes_group in D4W vs. H4W calves were negatively correlated with these 13 fecal metabolites, while Tyzzerella, Lachnospiraceae_UCG-004, Anaerotignum_lactatifermentans, Phascolarctobacterium_succinatutens_YIT_12067 and Gallibacterium_genomosp_3 were positively correlated (Figure 10D).
Figure 10. Correlation analysis of intestinal microorganisms and fecal metabolites in calves with diarrhea. Correlation between differential fecal microbes and differential metabolites in D1W and H1W calves (A). Correlation between different fecal microorganisms and different metabolites in D2W and H2W calves (B). Correlation between differential fecal microbes and differential metabolites in D3W and H3W calves (C). Correlation between different fecal microbes and different metabolites in D4W and H4W calves (D). *p < 0.05, **p < 0.01, ***p < 0.001.
Hence, GCA-900066575, Escherichia-shigella, Enterococcus_cecorum, Methylobacterium_sp_PC3044, Lachnospiraceae_UCG-004, and Tyzzerella in calf feces within 1–4 W of birth were positively correlated with these 13 fecal metabolites, suggesting that these microorganisms may be key in promoting diarrhea in calves during this critical period.
Diarrhea is a critical factor contributing to the disruption of the intestinal microbiota in calves (He et al., 2022). Previous studies have shown that diarrhea induces dysbiosis in the intestinal microbiota of calves, altering its structure and composition compared to healthy calves (Gomez et al., 2017). Once the intestinal barrier is damaged, pathogenic bacteria can colonize the intestinal tract, triggering inflammation (Qin et al., 2010). Diarrheic calves often exhibit a higher abundance of opportunistic pathogens, particularly members of Proteobacteria and Fusobacteriota, which are frequently associated with the dysregulation of the intestinal microbiota (Fan et al., 2021; Zeineldin et al., 2018). It has been shown that the main bacteria associated with high incidence of diarrhea belong the phyla Firmicutes (78.93%), Fusobacteriota (7.75%), Actinobacteriota (5.99%), and Proteobacteria (5.15%), with Firmicutes and Bacteroidetes being dominant in pre-weaning diarrhea calves, accounting for 80% (Kim E. T. et al., 2021). In the present study, it was found that Firmicutes (52.14%), Proteobacteria (26.74%), and Bacteroidota (17.09%) were the most prevalent bacteria in calves with diarrhea, which was consistent with the findings of previous studies. In particular, Firmicutes were the predominant phylum in 1–4 W diarrheic calves accounting for 52.14 and 50.30% of total bacteria in diarrheic calves and healthy calves, respectively.
As calves mature, on the day before weaning, Bacteroidota levels increase in the colon and cecum (Dias et al., 2018). It has been shown that Alloprevotella can be used as a probiotic to reduce or prevent diarrhea in calves (Rey et al., 2014). In the present study, Alloprevotella first appeared in H2W calves and its levels gradually increased, suggesting that this bacterial species can decelerate the occurrence of diarrhea in calves. Enterobacteriaceae was the dominant bacterial family in all fecal samples of calves (Klein-Jobstl et al., 2019). In particular, Enterobacteriaceae was found at 3–5 weeks of age in calves, but disappeared after the fifth week and was no longer detected in the subsequent weeks (Uyeno et al., 2010). Notably, in the present study, Enterobacteriaceae was detected in 2 W calves and was significantly increased in D2W diarrheic calves.
Changes in microbial dynamics can predict early-life diarrhea and enhance calf health (Bartels et al., 2010; Kim H. S. et al., 2021). Therefore, instability and low microbial diversity serve as potential biomarkers for predicting diarrhea in calves. Nutritional metabolic disorders, often linked to microecological disturbances, are marked by significant downregulation in gene expression related to ribosome translation, amino acid metabolism, and carbohydrate metabolism (Zeineldin et al., 2018). In particular, changes in amino acid metabolism have been identified as an important factor associated with calf diarrhea (Kim E. T. et al., 2021).
Energy metabolism is essential for body growth and development. When the energy levels cannot meet bodily needs, feed conversion and growth performance will be negatively impacted. Energy metabolism has been found to be positively correlated with the relative abundance of Prevotella, Bacteroides, and Sutterella in the gut (Lu et al., 2022). Prevotella can degrade starch, monosaccharides, and other non-cellulosic polysaccharides, produce volatile fatty acids to provide energy for the body and promote rumen development (Purushe et al., 2010). Similarly, Bacteroides can synthesize SCFAs. However, when the abundance of Bacteroidota in the gut is low, animals begin to produce δ-aminolevulinic acid with the support of the TCA cycle using glycine and succinyl coenzyme A as substrates. Thus, δ-aminolevulinic acid can be used as a marker to identify an increase in the abundance of Proteobacteria, which can lead to microecological dysregulation (Gomez et al., 2017).
Firmicutes are considered one of the primary producers of SCFAs, being more effective than Bacteroidota in promoting nutrient absorption (Li et al., 2021). Both Firmicutes and Bacteroidota participate in the degradation of complex polysaccharides, which allows the metabolization of carbohydrates, sugars, and fatty acids. However, a reduction in their abundance can impair these functions (Jami et al., 2013). Moreover, a significant negative correlation has been described between the abundance of Lachnospiraceae and the duration of diarrhea in calves. Lachnospiraceae, belonging to the phylum Firmicutes, can produce large amounts of SCFAs (Chen et al., 2017; Reichardt et al., 2014) and other metabolites (such as tryptophan), which help regulate immune and inflammatory responses (Arpaia et al., 2013; Smith et al., 2013). These metabolites decrease the levels of pro-inflammatory cytokines, such as tumor necrosis factor-α, interleukin-6, and interferon-γ, while increasing the production of anti-inflammatory factors in calves.
The Firmicutes/Bacteroidetes ratio is crucial for maintaining intestinal homeostasis in calves, and an imbalance in this ratio is linked to the onset of certain diseases (Cui et al., 2013; Stojanov et al., 2020). In particular, a significant increase in the Firmicutes/Bacteroidetes ratio leads to disturbances in the intestinal microbiota (Wang J. et al., 2019). In the present study, Firmicutes were found significantly increased in D1W diarrheic calves. At the species level, Enterococcus_cecorum within the phylum Firmicutes was found significantly increased in D3W diarrheic calves. At the genus level, Lachnospiraceae_UCG-004 and Tyzzerella, and Anaerotignum_lactatifermentans at the species level, all belonging to the phylum Firmicutes, were found significantly increased in D4W diarrheic calves. Thus, the increase in the above taxa may be the primary contributors to diarrhea in D4W calves in this specific farm. Furthermore, the above findings revealed that the abundance of Firmicutes in the feces of diarrheic calves may be the primary cause of disturbances in the intestinal microbiota of calves, and could be considered the main cause of diarrhea of calves.
Conversely, Clostridium_sp_YL32 belonging to the family Lachnospiraceae was found significantly decreased in D1W and D4W diarrheic calves, while Clostridium_scindens was significantly decreased in D4W diarrhea calves. Thus, these taxa can be considered beneficial bacteria that might contribute to hinder the onset of diarrhea in calves. Based on these observations, we speculated that the rapid immune response in calves during the initial stages of diarrhea may involve regulating inflammation through the modulation of Lachnospiraceae levels in the gut.
Ruminococcaceae, another family of butyrate-producing bacteria, can be used to predict and potentially prevent colic, with the Firmicutes (particularly Lachnospiraceae and Ruminococcaceae)/Proteobacteria ratio serving as a potential indicator (Weese et al., 2015). Although certain members of the family Ruminococcaceae are associated with diarrhea, others, such as UBA1819, a beneficial species belonging to the phylum Firmicutes, family Rumenococcaceae, have been linked to calf health, highlighting its potential to be used as probiotic strains in this particular commercial farm with this specific microbial colonization pattern (Chen et al., 2022). In the present study, compared with D2W, UBA1819 was significantly increased in H1W calves, which indicated that UBA1819 could be used as beneficial bacteria to promote calf growth. However, GCA_900066575, belonging to the phylum Firmicutes, was significantly increased in D1W diarrheic calves. Taken together, these findings highlight that Firmicutes played a pivotal role in disrupting the intestinal microbiota in calves.
In this study, Escherichia-shigella and Gammaproteobacteria were found to the main causes of diarrhea in D2W calves. Escherichia-shigella has been described to be associated with diarrhea-related diseases in calves in various studies (Akashi et al., 2017; Qu et al., 2012). Escherichia-shigella alter the intestinal environment of calves, promoting anaerobic microbial colonization and reducing the abundance of beneficial bacteria (Mayer et al., 2012). Intestinal colonization by Escherichia-shigella in calves likely increases the susceptibility of calves to E. coli-induced diarrhea, which is a well-known pathogen responsible for diarrhea in calves (Huang et al., 2020).
Proteobacteria, known for the production of lipopolysaccharide (LPS) that trigger inflammation, was the most abundant bacterial phylum in the intestinal microbiota of calves suffering from moist heat diarrhea (Mukhopadhya et al., 2012). However, the pathogenesis of Proteobacteria in the aggravation of diarrhea in calves is still unclear. In the present study, Proteobacteria was found to be the main phylum causing diarrhea in D2W calves, being significantly increased in D2W and D3W diarrheic calves, with Escherichia-shigella and Gammaproteobacteria identified as major contributors. Proteobacteria was the main bacterial group responsible for inflammation in D2W and D3W diarrheic calves, especially Enterococcus_cecorum, Gammaproteobacteria, and Methylobacterium_sp_PC3044.
Bifidobacterium detected in healthy calves is an important core and beneficial physiological flora in the intestinal tract of humans and animals, which has a significant impact on the early development of the body. Notably, Bifidobacterium_pseudocatenulatum_DSM_20438_=_JCM_1200_=_LMG_10505 was significantly decreased in D3W diarrheic calves, which reinforces its role as a beneficial bacterium hindering the onset of diarrhea and promoting growth in calves.
Polysaccharides, a key carbon source for intestinal microorganisms, are fermented into SCFAs including acetic acid, propionic acid, butanoic acid, valeric acid, and hexanoic acid, which play a critical role in maintaining mucosal integrity, metabolism, and immunity in vivo, thus promoting the growth of beneficial microorganisms in the gut. A reduction in SCFA-producing microbial species in the gut leads to compromised immune function (Angelini et al., 2014). For instance, propionic acid exerts immunomodulatory properties, inducing the expression of regulatory T cells (Duscha et al., 2020; Wang J. J. et al., 2019). The decrease in certain SCFA-producing bacteria, such as Parabacteroides and Ruminococcus, has been closely linked to reduced levels of acetic acid, whereas a decrease in Parabacteroides, Ruminococcus, Fournierella, and Rikenellaceae_RC9_gut_group has been shown to be closely related to a decrease in isocaproic acid content (Cui et al., 2023).
Our findings revealed that the contents of propionic acid, butanoic acid (D1W, D2W and D3W), and valeric acid (D3W group) were significantly lower in the feces of 1–4 W diarrheic calves compared to those in healthy calves. In addition, propionic acid and isobutyric acid were found to be significantly positively correlated with Collinsella and Bifidobacterium. It has been shown that changes in gut microbiota composition, especially related to a decrease in butyrate-producing bacteria, can lead to diarrhea in calves during the first week of birth (Gomez et al., 2017; Oikonomou et al., 2013). Acetate produced by Bacteroidota can be utilized by other bacteria (e.g., Bactericoccus and Megamonas) to produce butyrate and propionate (Trachsel et al., 2018), both of which are main sources of energy for intestinal epithelial cells in calves. Butyrate can also inhibit the signaling pathway of pro-inflammatory factors and enhance intestinal barrier function by increasing mucin secretion and strengthening tight junctions (Jonkers et al., 2012; Morgan et al., 2012).
Butyricimonas is a beneficial SCFA-producing bacterium that helps reduce inflammation. Our findings revealed that the concentration of Butyricimonas was significantly reduced in diarrheic calves with 4 weeks of age, while butanoic acid was significantly decreased in D1W, D2W, and D3W diarrheic calves. Moreover, butanoic acid was significantly negatively correlated with Proteobacteria in D3W diarrheic calves, and with Gammaproteobacteria. In addition, butanoic acid was significantly negatively correlated with Proteobacteria in D3W diarrheic calves and with Gammaproteobacteria in Proteobacteria; butanoic acid was negatively correlated with Escherichia-shigella within the class Gammaproteobacteria in D3W calves, as well as with growth performance traits in calves (e.g., BW, chest circumference, etc.).
Glycerophospholipids were a major component of the cell membrane and can help alleviate diarrhea-related diseases in calves (Akashi et al., 2017; Kalenyak et al., 2019). Phosphocholines (PCs) are involved in glycerophospholipid metabolism. In this experiment, 13 common differential metabolites associated with calf diarrhea traits were identified by WGCNA. The contents of glycerophospholipids (e.g., 1-palmitoyl-2-linoleoyl-sn-glycero-3-PC, 1-stearoyl-2-arachidonyl-sn-glycero-3-PC, 1-oleoyl-2-myristoyl-sn-glycero-3-phosphocholine, 1-palmitoyl-sn-glycero-3-PC, and 1-stearoyl-2-hydroxy-sn-glycero-3-phosphoethanolamine) were significantly elevated in diarrheic calves across all weeks, and were enriched in glycerol phospholipid metabolic pathway. The elevated levels of PC indicate that oxidative stress occurs in calves (Amin et al., 2023), and damaged intestinal mucosa triggers the release of arachidonic acid, which further promotes the release of reactive of oxygen species (ROS) and aggravates oxidative stress (Sanchez, 2018). In particular, lysophosphatidyl ethanolamine (LysoPE) and lysophosphatidylcholine (LysoPC), which belong to the class of lysophospholipids, are considered important components of lipid droplet monolayers in mammalian cells. Arachidonic acid has been considered a key unsaturated fatty acid associated with various diseases including diarrhea (Burns et al., 2018; Hua et al., 2020; Naito et al., 2015; Shi et al., 2023). Herein, the content of arachidonic acid (peroxide free) was associated with diarrhea traits and significantly increased in the first 4 weeks of age in calves. Correlation analysis revealed a positive correlation between elevated fecal metabolites and harmful intestinal microorganisms, along with a negative correlation with growth performance.
The correlation between arachidonic acid (peroxide free) and growth performance traits in calves, such as average daily gain, chest circumference, and hip height showed a significant negative correlation. This suggests that the increase in arachidonic acid (peroxide free) may be a contributing factor to the stunted growth observed in diarrheic calves. Further analysis revealed a positive correlation between arachidonic acid (peroxide free) and harmful fecal metabolites and microorganisms such as GCA_900066575, Gammaproteobacteria, and Proteobacteria, while showing a significant negative correlation with beneficial bacteria Lachnoclostridium_sp_YL32 and Clostridium_scindens. Studies have found that Escherichia-shigella was significantly correlated with metabolites involved in the metabolism of arachidonic acid and α-linolenic acid such as phosphatidylcholine and lecithin (Yan et al., 2022). Our findings also revealed that Escherichia-shigella was positively correlated with arachidonic acid (peroxide free). It can be speculated that arachidonic acid (peroxide free) is associated with an increase in Gammaproteobacteria and Proteobacteria, which results in the production of pro-inflammatory factors and may have stunted the growth and development of calves.
Herein, 7-keto-8-aminopelargonic acid was found in D1W diarrheic calves, which results in the production of demercaptobiotin after an ATP-dependent carboxylation reaction. Biotin is a cofactor of carboxylase, and is produced by various gut bacteria, being considered essential for glucose, amino acid, and fatty acid metabolism (Bi et al., 2016; Yoshii et al., 2019). It has also anti-inflammatory properties by inhibiting nuclear factor kappa-B activity (Elahi et al., 2018). Free biotin is essential for the growth and survival of the microbiota, and biotin deficiency will affect the composition of the intestinal microbiota in calves, leading to dysbiosis (Hayashi et al., 2017), which may be the reason for the significant decrease in metabolites related to biotin metabolism, TCA cycle, and lipopolysaccharide biosynthesis in diarrheic calves. Finally, a strong correlation was found between the excessive abundance of Fusobacterium and the loss of certain potentially beneficial bacterial genera (Yoshii et al., 2019), which might play a role that biotin deficiency contributes to intestinal microbiota disruption.
In the present study, it was found that diarrhea significantly reduced average daily gain and BW in calves during the first 4 weeks after birth. Significant changes were found in fecal microbiota composition and metabolite profiles in diarrheic calves, characterized by an increased abundance of intestinal pathogenic bacteria and imbalanced metabolite levels. Key bacteria such as Enterococcus_cecorum, Lachnospiraceae_UCG-004, Tyzzerella, Clostridium_butyricum Anaerotignum_lactatifermentans, and Escherichia-shigella were identified as primary contributors to diarrhea in calves. The main underlying mechanism leading to reduced growth and development in diarrheic calves could be associated to an increase in certain metabolites such as arachidonic acid (peroxide free), fatty acids, and glycerophospholipids. Conversely, beneficial bacteria such as Lachnoclostridium_sp_YL32, Bifidobacterium_pseudocatenulatum_DSM_20438_=_JCM_1200_=_LMG_10505, UBA1819, and Clostridium_scindens could potentially aid in preventing and controlling diarrhea in calves. These findings provide potential biomarkers for the targeted regulation of intestinal microbiota and metabolites, offering insights into strategies for preventing and controlling diarrhea in calves.
The datasets presented in this study can be found in online repositories. The names of the repository/repositories and accession number(s) can be found in the article/Supplementary material.
The animal study was approved by Animal Experiment Committee of Ningxia University. The study was conducted in accordance with the local legislation and institutional requirements.
PC: Conceptualization, Formal analysis, Writing – original draft. CH: Data curation, Software, Writing – review & editing. ML: Data curation, Software, Writing – review & editing. YA: Project administration, Writing – review & editing. XF: Project administration, Writing – review & editing. XM: Project administration, Writing – review & editing. DW: Methodology, Validation, Writing – review & editing. ZS: Methodology, Validation, Writing – review & editing. GJ: Methodology, Validation, Writing – review & editing. DY: Methodology, Validation, Writing – review & editing. QM: Methodology, Validation, Writing – review & editing. WY: Methodology, Validation, Writing – review & editing. JD: Methodology, Validation, Writing – review & editing. HZ: Methodology, Validation, Writing – review & editing. YM: Methodology, Validation, Writing – review & editing. YFM: Funding acquisition, Project administration, Writing – review & editing.
The author(s) declare that financial support was received for the research, authorship, and/or publication of this article. This work was supported by grants from the Ningxia Key Research and Development Plan Projects (No. 2023BCF01034, Yinchuan, China), Ningxia Ruminant Nutrition Science and Technology Innovation Team (No. 2024CXTD008, Yinchuan, China), Yinchuan Dairy Cattle Efficient and Healthy Breeding Research and Innovation Team (No. 2023CXTD32, Yinchuan, China).
We really appreciate all the supports from the funding agencies and all the participants.
H.Y.An and Z.D.Wang were employed by Ningxia Borui Technology Co., Ltd. Q.Ma and F.W.Yang were employed by Ningxia Xin’ao Agriculture and Animal Husbandry Co., Ltd.
The remaining authors declare that the research was conducted in the absence of any commercial or financial relationships that could be construed as a potential conflict of interest.
The authors declare that no Generative AI was used in the creation of this manuscript.
All claims expressed in this article are solely those of the authors and do not necessarily represent those of their affiliated organizations, or those of the publisher, the editors and the reviewers. Any product that may be evaluated in this article, or claim that may be made by its manufacturer, is not guaranteed or endorsed by the publisher.
The Supplementary material for this article can be found online at: https://www.frontiersin.org/articles/10.3389/fmicb.2024.1521719/full#supplementary-material
Akashi, T., Muto, A., Takahashi, Y., and Nishiyama, H. (2017). Enteral formula containing egg yolk lecithin improves diarrhea. J. Oleo Sci. 66, 1017–1027. doi: 10.5650/jos.ess17007
Amin, N., Schwarzkopf, S., Troscher-Mussotter, J., Camarinha-Silva, A., Danicke, S., Huber, K., et al. (2023). Host metabolome and faecal microbiome shows potential interactions impacted by age and weaning times in calves. Anim. Microbiome. 5:12. doi: 10.1186/s42523-023-00233-z
Angelini, R., Vortmeier, G., Corcelli, A., and Fuchs, B. (2014). A fast method for the determination of the pc/lpc ratio in intact serum by maldi-tof ms: an easy-to-follow lipid biomarker of inflammation. Chem. Phys. Lipids 183, 169–175. doi: 10.1016/j.chemphyslip.2014.07.001
Arpaia, N., Campbell, C., Fan, X., Dikiy, S., van der Veeken, J., DeRoos, P., et al. (2013). Metabolites produced by commensal bacteria promote peripheral regulatory t-cell generation. Nature 504, 451–455. doi: 10.1038/nature12726
Bartels, C. J., Holzhauer, M., Jorritsma, R., Swart, W. A., and Lam, T. J. (2010). Prevalence, prediction and risk factors of enteropathogens in normal and non-normal faeces of young Dutch dairy calves. Prev. Vet. Med. 93, 162–169. doi: 10.1016/j.prevetmed.2009.09.020
Bi, H., Zhu, L., Jia, J., and Cronan, J. E. (2016). A biotin biosynthesis gene restricted to helicobacter. Sci. Rep. 6:21162. doi: 10.1038/srep21162
Brunauer, M., Roch, F. F., and Conrady, B. (2021). Prevalence of worldwide neonatal calf diarrhoea caused by bovine rotavirus in combination with bovine coronavirus, escherichia coli k99 and cryptosporidium spp.: a meta-analysis. Animals 11:1014. doi: 10.3390/ani11041014
Burns, J. L., Nakamura, M. T., and Ma, D. (2018). Differentiating the biological effects of linoleic acid from arachidonic acid in health and disease. Prostaglandins Leukot. Essent. Fatty Acids 135, 1–4. doi: 10.1016/j.plefa.2018.05.004
Caporaso, J. G., Kuczynski, J., Stombaugh, J., Bittinger, K., Bushman, F. D., Costello, E. K., et al. (2010). Qiime allows analysis of high-throughput community sequencing data. Nat. Methods 7, 335–336. doi: 10.1038/nmeth.f.303
Chen, H., Liu, Y., Huang, K., Yang, B., Zhang, Y., Yu, Z., et al. (2022). Fecal microbiota dynamics and its relationship to diarrhea and health in dairy calves. J. Anim. Sci. Biotechnol. 13:132. doi: 10.1186/s40104-022-00758-4
Chen, L., Wilson, J. E., Koenigsknecht, M. J., Chou, W. C., Montgomery, S. A., Truax, A. D., et al. (2017). Nlrp12 attenuates colon inflammation by maintaining colonic microbial diversity and promoting protective commensal bacterial growth. Nat. Immunol. 18, 541–551. doi: 10.1038/ni.3690
Cho, Y. I., and Yoon, K. J. (2014). An overview of calf diarrhea – infectious etiology, diagnosis, and intervention. J. Vet. Sci. 15, 1–17. doi: 10.4142/jvs.2014.15.1.1
Cui, S., Guo, S., Zhao, Q., Li, Y., Ma, Y., and Yu, Y. (2023). Alterations of microbiota and metabolites in the feces of calves with diarrhea associated with rotavirus and coronavirus infections. Front. Microbiol. 14:1159637. doi: 10.3389/fmicb.2023.1159637
Cui, C., Shen, C. J., Jia, G., and Wang, K. N. (2013). Effect of dietary bacillus subtilis on proportion of bacteroidetes and firmicutes in swine intestine and lipid metabolism. Genet. Mol. Res. 12, 1766–1776. doi: 10.4238/2013.May.23.1
Dias, J., Marcondes, M. I., Motta, D. S. S., Cardoso, D. M. E. S., Fontes, N. M., Tassinari, R. R., et al. (2018). Bacterial community dynamics across the gastrointestinal tracts of dairy calves during preweaning development. Appl. Environ. Microbiol. 84. doi: 10.1128/AEM.02675-17
Dohgen, M., Hayahshi, H., Yajima, T., and Suzuki, Y. (1994). Stimulation of bicarbonate secretion by luminal short-chain fatty acid in the rat and human colon in vitro. Jpn. J. Physiol. 44, 519–531. doi: 10.2170/jjphysiol.44.519
Duscha, A., Gisevius, B., Hirschberg, S., Yissachar, N., Stangl, G. I., Dawin, E., et al. (2020). Propionic acid shapes the multiple sclerosis disease course by an immunomodulatory mechanism. Cell 180, 1067–1080.e16. doi: 10.1016/j.cell.2020.02.035
Edgar, R. C. (2004). Muscle: multiple sequence alignment with high accuracy and high throughput. Nucleic Acids Res. 32, 1792–1797. doi: 10.1093/nar/gkh340
Elahi, A., Sabui, S., Narasappa, N. N., Agrawal, S., Lambrecht, N. W., Agrawal, A., et al. (2018). Biotin deficiency induces th1- and th17-mediated proinflammatory responses in human cd4(+) t lymphocytes via activation of the mtor signaling pathway. J. Immunol. 200, 2563–2570. doi: 10.4049/jimmunol.1701200
Esser, D., Lange, J., Marinos, G., Sieber, M., Best, L., Prasse, D., et al. (2019). Functions of the microbiota for the physiology of animal metaorganisms. J. Innate Immun. 11, 393–404. doi: 10.1159/000495115
Fan, P., Kim, M., Liu, G., Zhai, Y., Liu, T., Driver, J. D., et al. (2021). The gut microbiota of newborn calves and influence of potential probiotics on reducing diarrheic disease by inhibition of pathogen colonization. Front. Microbiol. 12:772863. doi: 10.3389/fmicb.2021.772863
Gomez, D. E., Arroyo, L. G., Costa, M. C., Viel, L., and Weese, J. S. (2017). Characterization of the fecal bacterial microbiota of healthy and diarrheic dairy calves. J. Vet. Intern. Med. 31, 928–939. doi: 10.1111/jvim.14695
Guo, M., Wu, F., Hao, G., Qi, Q., Li, R., Li, N., et al. (2017). Bacillus subtilis improves immunity and disease resistance in rabbits. Front. Immunol. 8:354. doi: 10.3389/fimmu.2017.00354
Hayashi, A., Mikami, Y., Miyamoto, K., Kamada, N., Sato, T., Mizuno, S., et al. (2017). Intestinal dysbiosis and biotin deprivation induce alopecia through overgrowth of lactobacillus murinus in mice. Cell Rep. 20, 1513–1524. doi: 10.1016/j.celrep.2017.07.057
He, L., Wang, C., Simujide, H., Aricha, H., Zhang, J., Liu, B., et al. (2022). Effects of pathogenic escherichia coli infection on the flora composition, function, and content of short-chain fatty acids in calf feces. Animals 12:959. doi: 10.3390/ani12080959
Horvath, T. D., Ihekweazu, F. D., Haidacher, S. J., Ruan, W., Engevik, K. A., Fultz, R., et al. (2022). Bacteroides ovatus colonization influences the abundance of intestinal short chain fatty acids and neurotransmitters. Iscience 25:104158. doi: 10.1016/j.isci.2022.104158
Hua, Y. L., Ma, Q., Zhang, X. S., Jia, Y. Q., Peng, X. T., Yao, W. L., et al. (2020). Pulsatilla decoction can treat the dampness-heat diarrhea rat model by regulating glycerinphospholipid metabolism based lipidomics approach. Front. Pharmacol. 11:197. doi: 10.3389/fphar.2020.00197
Huang, M. Z., Cui, D. A., Wu, X. H., Hui, W., Yan, Z. T., Ding, X. Z., et al. (2020). Serum metabolomics revealed the differential metabolic pathway in calves with severe clinical diarrhea symptoms. Animals 10:769. doi: 10.3390/ani10050769
Jami, E., Israel, A., Kotser, A., and Mizrahi, I. (2013). Exploring the bovine rumen bacterial community from birth to adulthood. ISME J. 7, 1069–1079. doi: 10.1038/ismej.2013.2
Jonkers, D., Penders, J., Masclee, A., and Pierik, M. (2012). Probiotics in the management of inflammatory bowel disease: a systematic review of intervention studies in adult patients. Drugs 72, 803–823. doi: 10.2165/11632710-000000000-00000
Kalenyak, K., Heilmann, R. M., van de Lest, C., Brouwers, J. F., and Burgener, I. A. (2019). Comparison of the systemic phospholipid profile in dogs diagnosed with idiopathic inflammatory bowel disease or food-responsive diarrhea before and after treatment. PLoS One 14:e215435:e0215435. doi: 10.1371/journal.pone.0215435
Kim, E. T., Lee, S. J., Kim, T. Y., Lee, H. G., Atikur, R. M., Gu, B. H., et al. (2021). Dynamic changes in fecal microbial communities of neonatal dairy calves by aging and diarrhea. Animals 11:1113. doi: 10.3390/ani11041113
Kim, H. S., Whon, T. W., Sung, H., Jeong, Y. S., Jung, E. S., Shin, N. R., et al. (2021). Longitudinal evaluation of fecal microbiota transplantation for ameliorating calf diarrhea and improving growth performance. Nat. Commun. 12:161. doi: 10.1038/s41467-020-20389-5
Klein-Jobstl, D., Quijada, N. M., Dzieciol, M., Feldbacher, B., Wagner, M., Drillich, M., et al. (2019). Microbiota of newborn calves and their mothers reveals possible transfer routes for newborn calves' gastrointestinal microbiota. PLoS One 14:e220554. doi: 10.1371/journal.pone.0220554
Langille, M. G., Zaneveld, J., Caporaso, J. G., McDonald, D., Knights, D., Reyes, J. A., et al. (2013). Predictive functional profiling of microbial communities using 16s rrna marker gene sequences. Nat. Biotechnol. 31, 814–821. doi: 10.1038/nbt.2676
Li, A., Yang, Y., Qin, S., Lv, S., Jin, T., Li, K., et al. (2021). Microbiome analysis reveals gut microbiota alteration of early-weaned yimeng black goats with the effect of milk replacer and age. Microb. Cell Factories 20:78. doi: 10.1186/s12934-021-01568-5
Lozupone, C., and Knight, R. (2005). Unifrac: a new phylogenetic method for comparing microbial communities. Appl. Environ. Microbiol. 71, 8228–8235. doi: 10.1128/AEM.71.12.8228-8235.2005
Lu, M., Hu, F., Bi, Y., Ma, T., Diao, Q., Jiang, L., et al. (2022). Effects of anemoside b4 on diarrhea incidence, serum indices, and fecal microbial of suckling calves. Front. Vet. Sci. 9:851865. doi: 10.3389/fvets.2022.851865
Mayer, M., Abenthum, A., Matthes, J. M., Kleeberger, D., Ege, M. J., Holzel, C., et al. (2012). Development and genetic influence of the rectal bacterial flora of newborn calves. Vet. Microbiol. 161, 179–185. doi: 10.1016/j.vetmic.2012.07.023
Morgan, X. C., Tickle, T. L., Sokol, H., Gevers, D., Devaney, K. L., Ward, D. V., et al. (2012). Dysfunction of the intestinal microbiome in inflammatory bowel disease and treatment. Genome Biol. 13:R79. doi: 10.1186/gb-2012-13-9-r79
Mukhopadhya, I., Hansen, R., El-Omar, E. M., and Hold, G. L. (2012). Ibd-what role do proteobacteria play? Nat. Rev. Gastroenterol. Hepatol. 9, 219–230. doi: 10.1038/nrgastro.2012.14
Naito, Y., Ji, X., Tachibana, S., Aoki, S., Furuya, M., Tazura, Y., et al. (2015). Effects of arachidonic acid intake on inflammatory reactions in dextran sodium sulphate-induced colitis in rats. Br. J. Nutr. 114, 734–745. doi: 10.1017/S000711451500224X
Oikonomou, G., Teixeira, A. G., Foditsch, C., Bicalho, M. L., Machado, V. S., and Bicalho, R. C. (2013). Fecal microbial diversity in pre-weaned dairy calves as described by pyrosequencing of metagenomic 16s rdna. Associations of faecalibacterium species with health and growth. Plos One 8:e63157. doi: 10.1371/journal.pone.0063157
Oksanen, J. (2010). Vegan: Community ecology package. Available at: http://vegan.r-forge.r-project.org/ (Accessed September 23, 2010).
Pempek, J. A., Watkins, L. R., Bruner, C. E., and Habing, G. G. (2019). A multisite, randomized field trial to evaluate the influence of lactoferrin on the morbidity and mortality of dairy calves with diarrhea. J. Dairy Sci. 102, 9259–9267. doi: 10.3168/jds.2019-16476
Price, M. N., Dehal, P. S., and Arkin, A. P. (2010). Fasttree 2--approximately maximum-likelihood trees for large alignments. PLoS One 5:e9490. doi: 10.1371/journal.pone.0009490
Purushe, J., Fouts, D. E., Morrison, M., White, B. A., Mackie, R. I., Coutinho, P. M., et al. (2010). Comparative genome analysis of prevotella ruminicola and prevotella bryantii: insights into their environmental niche. Microb. Ecol. 60, 721–729. doi: 10.1007/s00248-010-9692-8
Qin, J., Li, R., Raes, J., Arumugam, M., Burgdorf, K. S., Manichanh, C., et al. (2010). A human gut microbial gene catalogue established by metagenomic sequencing. Nature 464, 59–65. doi: 10.1038/nature08821
Qu, M., Deng, Y., Zhang, X., Liu, G., Huang, Y., Lin, C., et al. (2012). Etiology of acute diarrhea due to enteropathogenic bacteria in Beijing, China. J. Infect. 65, 214–222. doi: 10.1016/j.jinf.2012.04.010
Reichardt, N., Duncan, S. H., Young, P., Belenguer, A., McWilliam, L. C., Scott, K. P., et al. (2014). Phylogenetic distribution of three pathways for propionate production within the human gut microbiota. ISME J. 8, 1323–1335. doi: 10.1038/ismej.2014.14
Rey, M., Enjalbert, F., Combes, S., Cauquil, L., Bouchez, O., and Monteils, V. (2014). Establishment of ruminal bacterial community in dairy calves from birth to weaning is sequential. J. Appl. Microbiol. 116, 245–257. doi: 10.1111/jam.12405
Saccenti, E., Hoefsloot, H. C. J., Smilde, A. K., Westerhuis, J. A., and Hendriks, M. M. W. B. (2014). Reflections on univariate and multivariate analysis of metabolomics data. Metabolomics 10, 361–374. doi: 10.1007/s11306-013-0598-6
Sanchez, B. (2018). Bile acid-microbiota crosstalk in gastrointestinal inflammation and carcinogenesis: a role for bifidobacteria and lactobacilli? Nat. Rev. Gastroenterol. Hepatol. 15:205. doi: 10.1038/nrgastro.2018.23
Shi, N., Li, N., Duan, X., and Niu, H. (2017). Interaction between the gut microbiome and mucosal immune system. Military Med. Res. 4:14. doi: 10.1186/s40779-017-0122-9
Shi, Z., Wang, Y., Yan, X., Ma, X., Duan, A., Hassan, F., et al. (2023). Metagenomic and metabolomic analyses reveal the role of gut microbiome-associated metabolites in diarrhea calves. Msystems 8:e0058223. doi: 10.1128/msystems.00582-23
Smith, P. M., Howitt, M. R., Panikov, N., Michaud, M., Gallini, C. A., Bohlooly-Y, M., et al. (2013). The microbial metabolites, short-chain fatty acids, regulate colonic treg cell homeostasis. Science 341, 569–573. doi: 10.1126/science.1241165
Stojanov, S., Berlec, A., and Strukelj, B. (2020). The influence of probiotics on the firmicutes/bacteroidetes ratio in the treatment of obesity and inflammatory bowel disease. Microorganisms 8:1715. doi: 10.3390/microorganisms8111715
Thevenot, E. A. (2016). (Pca, pls(−da) and opls(−da) for multivariate analysis and feature selection of omics data.
Trachsel, J., Humphrey, S., and Allen, H. K. (2018). Butyricicoccus porcorum sp. Nov., a butyrate-producing bacterium from swine intestinal tract. Int. J. Syst. Evol. Microbiol. 68, 1737–1742. doi: 10.1099/ijsem.0.002738
Uyeno, Y., Sekiguchi, Y., and Kamagata, Y. (2010). Rrna-based analysis to monitor succession of faecal bacterial communities in Holstein calves. Lett. Appl. Microbiol. 51, 570–577. doi: 10.1111/j.1472-765X.2010.02937.x
Visconti, A., Le Roy, C. I., Rosa, F., Rossi, N., Martin, T. C., Mohney, R. P., et al. (2019). Interplay between the human gut microbiome and host metabolism. Nat. Commun. 10:4505. doi: 10.1038/s41467-019-12476-z
Wang, J., Feng, W., Zhang, S., Chen, L., Sheng, Y., Tang, F., et al. (2019). Ameliorative effect of atractylodes macrocephala essential oil combined with panax ginseng total saponins on 5-fluorouracil induced diarrhea is associated with gut microbial modulation. J. Ethnopharmacol. 238:111887. doi: 10.1016/j.jep.2019.111887
Wang, J. J., Zhang, Q. M., Ni, W. W., Zhang, X., Li, Y., Li, A. L., et al. (2019). Modulatory effect of lactobacillus acidophilus klds 1.0738 on intestinal short-chain fatty acids metabolism and gpr41/43 expression in beta-lactoglobulin-sensitized mice. Microbiol. Immunol. 63, 303–315. doi: 10.1111/1348-0421.12723
Weese, J. S., Holcombe, S. J., Embertson, R. M., Kurtz, K. A., Roessner, H. A., Jalali, M., et al. (2015). Changes in the faecal microbiota of mares precede the development of post partum colic. Equine Vet. J. 47, 641–649. doi: 10.1111/evj.12361
Yan, Z., Zhang, K., Zhang, K., Wang, G., Wang, L., Zhang, J., et al. (2022). Integrated 16s rdna gene sequencing and untargeted metabolomics analyses to investigate the gut microbial composition and plasma metabolic phenotype in calves with dampness-heat diarrhea. Front. Vet. Sci. 9:703051. doi: 10.3389/fvets.2022.703051
Ying, L., Qianqian, X. U., Xiaoyang, X., Jun, C., Jingjing, X., Ligong, C., et al. (2013). Effect of anemonin on intestinal mucosa repair factors egfr and tgfβ1 of diarrheal mice. Chin. J. Anim. Vet. Sci. 44, 475–480.
Yoshii, K., Hosomi, K., Sawane, K., and Kunisawa, J. (2019). Metabolism of dietary and microbial vitamin b family in the regulation of host immunity. Front. Nutr. 6:48. doi: 10.3389/fnut.2019.00048
Keywords: diarrhea, Holstein male calves, 16S rRNA, untargeted metabolomics, growth performance
Citation: Cao PP, Hu CL, Li MJ, An YH, Feng X, Ma XH, Wang DZ, Song ZH, Ji GS, Yang D, Ma Q, Yang WF, Dong JN, Zhang HR, Ma Y and Ma YF (2025) 16S rRNA and metabolomics reveal the key microbes and key metabolites that regulate diarrhea in Holstein male calves. Front. Microbiol. 15:1521719. doi: 10.3389/fmicb.2024.1521719
Received: 04 November 2024; Accepted: 27 December 2024;
Published: 15 January 2025.
Edited by:
Kun Li, Nanjing Agricultural University, ChinaReviewed by:
Hao Lizhuang, Qinghai University, ChinaCopyright © 2025 Cao, Hu, Li, An, Feng, Ma, Wang, Song, Ji, Yang, Ma, Yang, Dong, Zhang, Ma and Ma. This is an open-access article distributed under the terms of the Creative Commons Attribution License (CC BY). The use, distribution or reproduction in other forums is permitted, provided the original author(s) and the copyright owner(s) are credited and that the original publication in this journal is cited, in accordance with accepted academic practice. No use, distribution or reproduction is permitted which does not comply with these terms.
*Correspondence: Y. F. Ma, bWF5ZkBueHUuZWR1LmNu
Disclaimer: All claims expressed in this article are solely those of the authors and do not necessarily represent those of their affiliated organizations, or those of the publisher, the editors and the reviewers. Any product that may be evaluated in this article or claim that may be made by its manufacturer is not guaranteed or endorsed by the publisher.
Research integrity at Frontiers
Learn more about the work of our research integrity team to safeguard the quality of each article we publish.