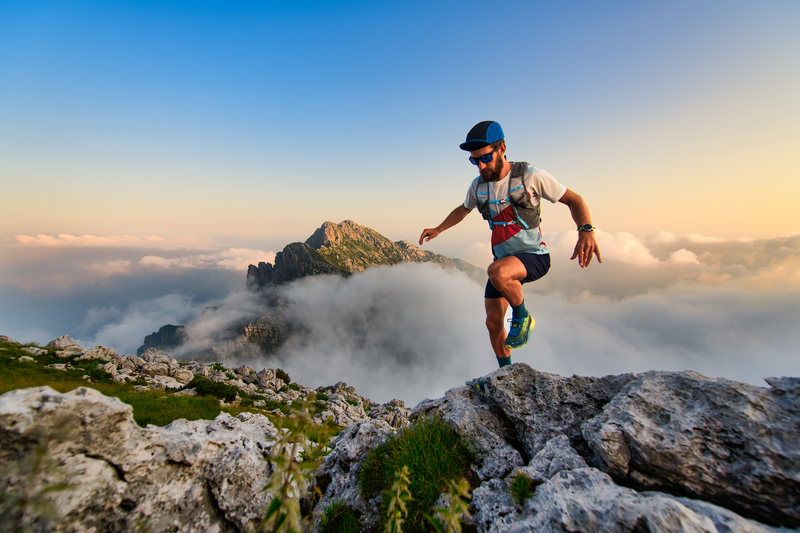
94% of researchers rate our articles as excellent or good
Learn more about the work of our research integrity team to safeguard the quality of each article we publish.
Find out more
ORIGINAL RESEARCH article
Front. Microbiol. , 23 January 2025
Sec. Systems Microbiology
Volume 15 - 2024 | https://doi.org/10.3389/fmicb.2024.1508030
This article is part of the Research Topic Artificial Intelligence in Pathogenic Microorganism Research View all 14 articles
Objective: To develop a machine learning-based prediction model to assist clinicians in accurately determining whether the detection of Klebsiella pneumoniae (KP) in sputum samples indicates an infection, facilitating timely diagnosis and treatment.
Research methods: A retrospective analysis was conducted on 8,318 patients with KP cultures admitted to a tertiary hospital in Northeast China from January 2019 to December 2023. After excluding duplicates, other specimen types, cases with substandard specimen quality, and mixed infections, 286 cases with sputum cultures yielding only KP were included, comprising 67 cases in the colonization group and 219 cases in the infection group. Antimicrobial susceptibility testing was performed on the included strains, and through univariate logistic regression analysis, 15 key influencing factors were identified, including: age > 62 years, ESBL, CRKP, number of positive sputum cultures for KP, history of tracheostomy, use of mechanical ventilation for >96 h, indwelling gastric tube, history of craniotomy, recent local glucocorticoid application, altered consciousness, bedridden state, diagnosed with respiratory infectious disease upon admission, electrolyte disorder, hypoalbuminemia, and admission to ICU (all p < 0.05). These factors were used to construct the model, which was evaluated using accuracy, precision, recall, F1 score, AUC value, and Brier score.
Results: Antimicrobial susceptibility testing indicated that the resistance rates for penicillins, cephalosporins, carbapenems, and quinolones were significantly higher in the infection group compared to the colonization group (all p < 0.05). Six predictive models were constructed using 15 key influencing factors, including Classification and Regression Trees (CART), C5.0, Gradient Boosting Machines (GBM), Support Vector Machines (SVM), Random Forest (RF), and Nomogram. The Random Forest model performed best among all indicators (accuracy 0.93, precision 0.98, Brier Score 0.06, recall 0.72, F1 Score 0.83, AUC 0.99). The importance of each factor was demonstrated using mean decrease in Gini. “Admitted with a diagnosis of respiratory infectious disease” (8.39) was identified as the most important factor in the model, followed by “Hypoalbuminemia” (7.83), then “ESBL” (7.06), “Electrolyte Imbalance” (5.81), “Age > 62 years” (5.24), “The number of Positive Sputum Cultures for KP > 2” (4.77), and being bedridden (4.24). Additionally, invasive procedures (such as history of tracheostomy, use of ventilators for >96 h, and craniotomy) were also significant predictive factors. The Nomogram indicated that CRKP, presence of a nasogastric tube, admission to the ICU, and history of tracheostomy were important factors in determining KP colonization.
Conclusion: The Random Forest model effectively distinguishes between infection and colonization status of KP, while the Nomogram visually presents the predictive value of various factors, providing clinicians with a reference for formulating treatment plans. In the future, the accuracy of infection diagnosis can be further enhanced through artificial intelligence technology to optimize treatment strategies, thereby improving patient prognosis and reducing healthcare burdens.
Klebsiella pneumoniae (commonly referred to as KP) is a frequently encountered opportunistic pathogen in clinical settings, typically colonizing the human gut and upper respiratory tract (Podschun and Ullmann, 1998). KP colonization can be detected in more than 40% of the population (Guilhen et al., 2019). At the same time, this bacterium is the third most common bacterial cause of hospital-acquired pneumonia (Jones, 2010). KP infections are also common in ventilator-associated pneumonia (VAP) (Alnimr, 2023). With the emergence of multi-drug resistant (MDR) strains, it has been classified by the World Health Organization (WHO) as a priority pathogen for which urgent new therapies are needed (Antimicrobial resistance: global report on surveillance, n.d.). Sputum culture is an important method for identifying respiratory tract infection pathogens in clinical practice; however, for the opportunistic pathogen KP, clinicians often find it challenging to distinguish between colonization and infection. Colonization refers to the adherence and growth of microorganisms on the surface of host tissues, while infection describes the process by which microorganisms invade host cells or tissues, leading to pathological conditions. Colonization and infection are closely related (Vornhagen et al., 2024). When the host’s immune response is suppressed or there are other risk factors present, colonizing Klebsiella pneumoniae may begin to proliferate and invade surrounding tissues, leading to severe infection (Cai et al., 2024). Misclassifying colonization as infection can lead to overtreatment in clinical settings, increasing the incidence of adverse reactions and promoting the development of antibiotic resistance. This includes mechanisms such as enzymatic antibiotic inactivation and modification (e.g., ESBLs, AmpC, and NDM), spread of resistance genes, absence of outer membrane porin expression, and overexpression of active efflux pump systems, among others (Li et al., 2023) (as shown in Figure 1, which illustrates the main resistance mechanisms of KP). Conversely, misclassifying infection as colonization can delay clinical treatment and increase patient mortality rates. Therefore, there is a need for a tool to assist clinicians in better differentiating between KP colonization and infection.
Figure 1. Major resistance mechanisms of KP. In clinical practice, sputum culture is an important tool for diagnosing respiratory tract infections. However, misinterpreting colonizing Klebsiella pneumoniae as an infectious pathogen may lead to unnecessary antimicrobial treatment. In such cases, this could contribute to the development of resistance in Klebsiella pneumoniae. We have illustrated several common resistance mechanisms of Klebsiella pneumoniae in the figure.
Previous studies have typically used logistic regression analysis to identify risk factors associated with infection and colonization (Wang X. et al., 2024). However, logistic regression struggles to capture complex nonlinear relationships between features and lacks intuitive clarity. In recent years, artificial intelligence and machine learning have shown great potential in the diagnosis and treatment of bacterial infections. They can effectively handle complex data, integrate various patient characteristics to construct predictive models, and help doctors understand more intuitively how different clinical features influence the occurrence of diseases, providing more reliable support for clinical decision-making (Deo, 2015; Jiang et al., 2022; Seyer Cagatan et al., 2022). Therefore, the aim of this study is to develop a predictive model that can accurately identify colonization versus infection of KP in sputum cultures. This model is intended to provide clinicians with scientific evidence to optimize treatment strategies and reduce the risk of misdiagnosis and inappropriate use of antimicrobial agents.
A retrospective analysis was conducted on 8,318 patients with microbiological cultures identified as KP admitted to a tertiary hospital in Northeast China from January 2019 to December 2023. After excluding duplicate cases, other types of specimens, cases with substandard specimen quality, and mixed infections, a total of 286 cases were included, which consisted of patients with sputum cultures yielding only Klebsiella pneumoniae. Among these, 67 cases were classified as the colonization group and 219 cases as the infection group (Figure 2). This study has been reviewed and approved by the Ethics Committee of the First Affiliated Hospital of China Medical University [Ethics ID(2023)2023-142-2]. Infectious disease specialists confirmed the diagnosis of KP infection or colonization (diagnostic criteria are shown in Table 1). (In Figure 3 we show the CT lung images and sputum cultures of a relatively typical case of pneumonia due to KP).
Clinical data for the included cases were collected through the hospital’s electronic medical record system. Using a case–control study design, a retrospective analysis of the clinical data from all patients was conducted. Antimicrobial susceptibility testing was performed on the KP (KP) strains obtained from the included cases. Identification of all pathogens and antimicrobial susceptibility testing were carried out using the VITEK 2 automated bacterial identification and VITEK 2 Compact antimicrobial susceptibility testing system from bioMérieux, France. The results of the antimicrobial susceptibility tests were categorized as susceptible, intermediate, and resistant according to the standards set by the Clinical and Laboratory Standards Institute (CLSI) (CLSI, 2023; Vornhagen et al., 2024). Extended spectrum beta-lactamase (ESBL) strains were detected through double disk synergy tests and phenotypic confirmation tests (antimicrobial susceptibility test disks were purchased from Oxoid, United Kingdom). In this study, carbapenem-resistant bacteria were defined as those resistant to either imipenem or meropenem.
Statistical analysis was conducted using SPSS 27.0 software. Categorical data were expressed as counts and percentages. The Chi-square test was used to compare the differences in resistance rates between the KP colonization group and the infection group (p < 0.05 was considered statistically significant). Univariate binary logistic regression analysis was performed to identify factors with statistically significant differences between the two groups (p < 0.05 was considered statistically significant), and these factors were included as predictive variables in the model. Predictive models to distinguish between KP colonization and infection were constructed using R 4.4.1 software, including Classification and Regression Trees (CART), C5.0, Gradient Boosting Machine (GBM), Support Vector Machine (SVM), Random Forest (RF), and Nomogram models. The function set.seed(42) was used to ensure the reproducibility of the random process, allowing the same data to yield identical training and test set divisions. The trainControl function was employed to set the model training control parameters for 10-fold cross-validation. The performance of each model was evaluated using accuracy, precision, recall, F1 Score, AUC value, and Brier score.
Antimicrobial susceptibility testing was performed on strains isolated from sputum cultures of the final 286 enrolled cases (Figure 4). Compared with the colonization group, the infection group exhibited generally higher resistance rates to various antibiotics, with all differences being statistically significant (p < 0.05) (see Supplementary Table S1 for detailed results). A total of 141 CRKP strains were isolated, including 17 strains from the colonization group and 124 strains from the infection group.
Figure 4. Comparison of drug resistance rates. It shows the rates of resistance to common antimicrobial agents in the colonized and infected groups. The X-axis represents the antimicrobial agents (Ampicillin, Cefuroxime, Ceftazidime, Ceftriaxone, Cefepime, Ampicillin/Sulbactam, Piperacillin/Tazobactam, Aztreonam, Imipenem, Meropenem, Gentamicin, Amikacin, Levofloxacin, Nitrofurantoin, Sulfamethoxazole/Trimethoprim), and the Y-axis represents the resistance rate. Yellow indicates the colonized group and green indicates the infected group. Detailed information can be found in Supplementary Table S1.
The clinical data of the included cases were collected (Table 2), and univariate logistic regression analysis revealed the following important factors influencing colonization and infection: age > 62 years, ESBL positivity, CRKP positivity, number of positive sputum cultures for KP, history of tracheostomy, use of ventilator for >96 h, presence of indwelling gastric tube, craniotomy, recent history of topical glucocorticoid use, altered consciousness, bedridden status, diagnosis of respiratory infectious disease upon admission, electrolyte disturbances, low serum protein levels, and admission to ICU (all p < 0.05).
Table 2. Clinical characteristics of cases with KP colonization and infection and univariate logistic regression analysis.
The 15 important influencing factors obtained from univariate logistic regression analysis were used to construct models including Classification and Regression Trees (CART), C5.0, Gradient Boosting Machine (GBM), Support Vector Machine (SVM), Random Forest (RF), and Nomogram. These models were designed to differentiate between colonization and infection status of KP. The performance of the models was evaluated using several metrics, including accuracy, precision, recall, F1 Score, Area Under the Curve (AUC), and Brier Score.
In this study, the predictive models were evaluated, and the Random Forest model demonstrated the best performance across all metrics: accuracy of 0.93, precision of 0.98, Brier Score of 0.06, recall of 0.72, and F1 Score of 0.83, indicating excellent predictive performance. The C5 model also performed well, with an accuracy of 0.87, precision of 0.92, and an F1 Score of 0.65, suggesting its reliability in predicting KP infections. The CART model achieved an accuracy of 0.85, precision of 0.76, and an F1 Score of 0.61, showing performance similar to that of the C5 model. Although both C5 and CART effectively distinguished between colonization and infection, their recall rates were relatively low, and their Brier Scores were higher, indicating slightly insufficient predictive performance. The SVM model had an accuracy of 0.84 and effectively identified infection samples, reducing the risk of false negatives; however, its overall performance was still inferior to that of the C5 and CART models. The GBM model performed relatively weakly across all metrics, particularly in recall (0.35) and F1 Score (0.46), suggesting a higher probability of missed detections. The AUC (Area Under the Curve) is an important metric for measuring a model’s discriminative ability. In this study, the Random Forest model achieved an AUC of 0.99, while the Nomogram model also exhibited a high AUC of 0.85. In contrast, the AUC values for the CART, GBM, and C5 models ranged from 0.75 to 0.80, indicating moderate discrimination ability. In summary, the Random Forest model performed best in distinguishing between colonization and infection of KP, while the Nomogram model also proved to be a reliable choice, suitable for the accurate identification of colonization and infection states in clinical applications (see Figure 5 and Table 3).
Figure 5. ROC curves for the six models. Here are the ROC (receiver operating characteristic) curves for six different models, with the horizontal axis of each plot representing specificity, the vertical axis representing sensitivity, and the diagonal as the baseline for random guesses. AUC is an important indicator of ROC curve, which is used to evaluate the classification performance of the model. The values of AUC range from 0 to 1 and are explained as follows: AUC = 1: perfect. 0.7 ≤ AUC < 0.8: good. 0.6 ≤ AUC < 0.7: general. AUC < 0.6 was considered poor. In the figure, the closer the curve is to the upper left corner, the higher the AUC value, indicating better model performance (the specific AUC values can be found in Table 3).
To better understand the relationship between the model and the data, we visually analyzed the best-performing Random Forest model using Mean Decrease Gini. The X-axis represents the “Mean Decrease Accuracy” values for each feature; higher values indicate a more significant decline in model performance when that feature is removed. The influencing factors are ranked according to their importance, with features at the top contributing the most to the model. The most important factor in the model is “Admitted with a diagnosis of respiratory infectious disease” (8.39). The second most important feature is “Hypoalbuminemia” (7.83), followed by “ESBL” (7.06), “Electrolyte Imbalance” (5.81), “Age” (5.24), and “The number of Positive Sputum Cultures for KP > 2” (4.77). In contrast, factors such as “Craniotomy” (1.97), “Nasogastric Tube Placement” (2.62), and “ICU Admission” (2.99) contributed relatively little to determining whether the sputum culture for KP indicated infection (see Figure 6).
Figure 6. Evaluation of feature importance in the random forest model. This bar chart shows the importance of different clinical factors in the random forest model. The X-axis represents the “Mean Decrease Gini,” while the Y-axis lists the clinical factors, arranged in order of their importance to the outcome from high to low. ≥ 8.0, High; 5.0–7.9, Medium-High; 3.0–4.9, Medium; 1.0–2.9, Low; 0.0–0.9, Very Low or None.
Due to the good performance of the Nomogram, which is more intuitive than the Random Forest model, we also presented the drawn Nomogram (Figure 7). It clearly shows that “Admitted with a diagnosis of respiratory infectious disease” is the most important factor, followed by “bedridden status,” “the number of positive sputum cultures for KP > 2,” ESBL, and craniotomy. Additionally, CRKP, nasogastric tube placement, ICU admission, and tracheostomy are all important factors for determining KP colonization.
Figure 7. Nomogram model. The Y-axis of the nomogram represents various clinical factors, with each axis line corresponding to an input variable. The figure 0 on the axis indicates colonization, while the figure 1 indicates infection. The scale value on the X-axis represents the points or measurements of that clinical factors, and the corresponding point can be found to determine the contribution of that factor in the total score.
In this study, the resistance rates of various antimicrobial drugs in the infection group were generally higher than those in the colonization group, with statistically significant differences (p < 0.05). The proportion of ESBL and CRKP in the infection group was significantly higher than that in the colonization group, indicating that the infection status is highly likely associated with the extensive use of antimicrobial drugs. Previous studies have shown that the use of broad-spectrum antimicrobial agents and carbapenems are independent risk factors for the occurrence of ESBL and CRKP (Lou et al., 2022; Zhu et al., 2023; Lopera et al., 2024). High resistance rates will place significant pressure on the selection of antimicrobial agents in clinical practice. Infection status not only increases the demand for antimicrobial drugs among patients but may also accelerate the proliferation and transmission of resistant strains. Previous studies have indicated that colonizing strains of KP (KP) have a high degree of homology with infecting isolates, with 50% of KP infections arising from the patient’s own microbiota (Gorrie et al., 2017). However, this study found that there was a significant difference in the resistance rates of the strains isolated from the colonization group and the infection group. From the host’s perspective (Gonzalez-Ferrer et al., 2021), during an infection, the patient’s immune system may be compromised, and the transmission of resistant strains through cross-infection in healthcare environments, such as hospitals (Córdova-Espinoza et al., 2023), may result in the strains in the infection group being more resistant. In contrast, the strains in the colonization group may not experience a significant increase in resistance due to the pressure from antimicrobial agents, as they exist in a relatively stable colonization state. From a microbiological perspective (Gomez-Simmonds and Uhlemann, 2017), Colonized strains may convert to infection by acquiring additional resistance genes through mechanisms such as horizontal gene transfer, gene mutation, and so on. In summary, the resistance rates in the infection group are significantly higher than those in the colonization group, indicating that we should enhance the monitoring and research of resistant strains in clinical practice. Particularly, preventing and controlling the spread of resistant bacteria will be an important focus for future research and practice.
In this study, we constructed Classification and Regression Trees (CART), C5.0, Gradient Boosting Machine (GBM), Support Vector Machine (SVM), Random Forest (RF), and Nomogram models through univariate logistic regression analysis. All six models achieved AUC values above 0.75, indicating that our research can provide a reference for differentiating between KP colonization and infection in clinical settings. The Random Forest model performed the best across all metrics, with an AUC of 0.99, an accuracy of 0.93, effectively distinguishing between KP colonization and infection status while significantly reducing the probability of misclassification. The precision was 0.98, indicating that nearly all samples predicted as infections were correct, thus reducing the occurrence of false positives. The Brier Score was 0.06, reflecting excellent predictive performance. In many practical scenarios, Random Forest has demonstrated outstanding performance, and this powerful ensemble learning algorithm is particularly well-suited for clinical data analysis and prediction (Jin et al., 2024; Mendapara, 2024; Wang Y. et al., 2024).
To better understand the relationship between the model and the data, we performed a visual analysis of the Random Forest model, which exhibited the best performance, by examining the importance of different factors using Mean Decrease Gini. These factors hold significant clinical relevance. Specifically, “Admitted with a diagnosis of respiratory infectious disease” is identified as the primary predictor of KP infection. The significant increase in infection risk for patients diagnosed with respiratory infectious diseases upon admission highlights the importance of early infection identification. This is particularly crucial as a large proportion of KP infections originate from the community, and studies have shown a strong correlation between KP-CAP (KP Community-Acquired Pneumonia) infections and higher, earlier mortality rates (Grosjean et al., 2024). This suggests that clinicians should promptly conduct pathogen detection and identification when seeing patients with respiratory infection symptoms, ensuring early detection, diagnosis, and treatment. Hypoalbuminemia, electrolyte imbalance, ESBL presence, and bedridden status are all important influencing factors for KP infection, closely related to the patient’s overall health status and immune function. Being bedridden indicates that the patient’s mobility is limited, which may lead to a series of health issues, such as muscle atrophy, venous thromboembolism, and complications like pneumonia. Hypoalbuminemia can result in malnutrition, reduced immunity, and poor healing capacity (Wang et al., 2023). This highlights the potential contribution of a patient’s nutritional and metabolic status to the risk of infection. For bedridden patients, it is crucial to regularly monitor serum albumin and electrolyte levels. An age greater than 62 years has been identified as an important risk factor, which is consistent with previously published studies indicating that nearly half of KP infections (45.7%) occur in the elderly population (Liu and Guo, 2019). This may be related to the decreased immune function and the prevalence of underlying diseases in the elderly population. The number of positive sputum cultures for KP (greater than 2) indicates a higher probability of infection, suggesting that multiple cultures are crucial for improving diagnostic accuracy in clinical practice. Invasive procedures such as tracheostomy and the placement of nasogastric tubes are also significant factors in KP infections. These invasive interventions can partially disrupt the normal structure of the upper respiratory tract, affecting the cleanliness and protective functions of the airway, making infections more likely. When the duration of mechanical ventilation exceeds 96 h, the risk of infection significantly increases. This may be related to the formation of respiratory biofilms, which can serve as breeding grounds for bacteria and elevate the risk of infection (Mishra et al., 2024). Craniotomy is also an important influencing factor, which may be because craniotomy is often difficult and lasting for a long time, and some patients are critically ill and need immediate surgery. Inadequate preoperative preparation and non-standard use of prophylactic antibiotics can lead to an increased probability of infection (He et al., 2024). Recent use of local corticosteroids can suppress local immune responses, reducing the body’s defenses against infections and making it easier for infections to develop. This immunosuppressive effect can lead to an increased risk of KP infections, particularly in patients who may already be vulnerable due to other underlying health conditions or invasive procedures (Fernández Peláez et al., 2000). Patients with disorders of consciousness usually lack the cough reflex to effectively clear respiratory secretions, and at the same time, the probability of aspiration is increased, which increases the risk of KP infection (Kobata, 2023).
Although the Random Forest model demonstrated good overall performance, it is not very intuitive. In contrast, a Nomogram is an intuitive visual tool used to display the risks or outcomes of multivariable prediction models. It is easy to use and understand, and it clearly illustrates the relative contributions of various factors to the outcome, making it particularly suitable for personalized decision-making in clinical practice (Yang et al., 2022; Gao et al., 2024; Luo et al., 2024). This study found that certain factors can serve as important criteria for assessing whether KP is colonized. When a patient’s sputum culture results indicate CRKP (Ceftazidime-Resistant KP), or if the patient has a nasogastric tube in place, is undergoing tracheostomy, or is in the ICU, we must exercise greater caution and thoroughness in assessment and judgment rather than blindly initiating antimicrobial therapy. Only by comprehensively considering the patient’s specific circumstances can clinicians develop more rational treatment plans, significantly improving the targeting and effectiveness of treatment while minimizing the misuse of medications and the emergence of resistance.
Although this study provides important insights into the diagnosis and management of KP infections, several limitations remain, which we hope to overcome in future research. First, the study included only 286 cases of KP positivity, and the data were sourced from a single center. Future studies should aim to expand the sample size and conduct multi-center collaborative research to enhance the generalizability and reliability of the findings. Moreover, the determination of colonization and infection relies on clinicians’ judgments and experiences, which may vary among different doctors and affect the accuracy of the results. We look forward to the development of more standardized clinical guidelines to reduce subjective differences among physicians and improve diagnostic consistency. Although this study identified 15 influencing factors, the occurrence of infections in clinical practice may be affected by other potential factors, such as the composition of the microbiome, environmental factors, and the psychological state of the patient. Additionally, molecular mechanisms, such as the development of bacterial resistance and the host’s immune response, are also important influencing factors. However, due to limitations in laboratory conditions and funding, we were unable to include these factors in this study. We hope that future research can develop a more comprehensive clinical model to provide clinicians with a more scientific and accurate basis for their judgments. Additionally, we anticipate integrating our constructed model into a clinical decision support system (CDSS) in the future. Regular evaluation of the model’s performance, along with the collection of feedback from healthcare professionals and patients, will be critical. This will allow us to update the data and retrain the model, continuously improving its usability and accuracy.
In this study, we successfully constructed six predictive models. Among them, the Random Forest model performed the best, achieving an area under the curve (AUC) of 0.99, with an accuracy of 0.93 and a precision of 0.98. This model effectively distinguishes between KP (KP) infection and colonization status, significantly reducing the rate of misjudgment. The Brier Score was 0.06, further validating the model’s excellent predictive performance. Key factors such as being diagnosed with respiratory infections upon admission and the number of positive sputum cultures underscore the importance of early diagnosis and repeated cultures. Additionally, factors closely related to the overall health status of patients, such as being bedridden, electrolyte imbalances, and hypoalbuminemia, significantly impact the risk of KP infection. To enhance the model’s applicability in clinical practice, we employed an intuitive nomogram, making it easier for clinicians to understand and utilize multivariable predictions. When faced with patients whose sputum cultures test positive for CRKP, or who have nasogastric tubes, tracheostomies, or are receiving ICU treatment, it is crucial to conduct a more cautious evaluation rather than initiating antimicrobial therapy blindly. This approach allows for more precise decision-making in patient management. We look forward to applying artificial intelligence technologies in future research to improve the accuracy of infection diagnoses, optimize antimicrobial treatment strategies, reduce unnecessary use of antibiotics, and thereby enhance patient outcomes and decrease the burden on healthcare systems.
The original contributions presented in the study are included in the article/Supplementary material, further inquiries can be directed to the corresponding authors.
The studies involving humans were approved by the Ethics Committee of the First Affiliated Hospital of China Medical University [KLS(2023)2023-142-2]. The studies were conducted in accordance with the local legislation and institutional requirements. The human samples used in this study were acquired from primarily isolated as part of your previous study for which ethical approval was obtained. Written informed consent for participation was not required from the participants or the participants’ legal guardians/next of kin in accordance with the national legislation and institutional requirements.
XiaZ: Conceptualization, Data curation, Methodology, Software, Validation, Visualization, Writing – original draft, Writing – review & editing. XifZ: Conceptualization, Data curation, Methodology, Software, Validation, Visualization, Writing – original draft, Writing – review & editing. DZ: Conceptualization, Data curation, Formal analysis, Visualization, Writing – review & editing. JX: Conceptualization, Formal analysis, Methodology, Visualization, Writing – review & editing. JZ: Methodology, Resources, Writing – original draft, Writing – review & editing. XinZ: Methodology, Resources, Writing – original draft, Writing – review & editing.
The author(s) declare that financial support was received for the research, authorship, and/or publication of this article. This study was supported by the Community Infectious Diseases Research Capacity Building Program (J202201E026).
The images in this article are drawn with the help of BioRender and WPS office.
The authors declare that the research was conducted in the absence of any commercial or financial relationships that could be construed as a potential conflict of interest.
The author(s) declare that no Gen AI was used in the creation of this manuscript.
All claims expressed in this article are solely those of the authors and do not necessarily represent those of their affiliated organizations, or those of the publisher, the editors and the reviewers. Any product that may be evaluated in this article, or claim that may be made by its manufacturer, is not guaranteed or endorsed by the publisher.
The Supplementary material for this article can be found online at: https://www.frontiersin.org/articles/10.3389/fmicb.2025.1508030/full#supplementary-material
Alnimr, A. (2023). Antimicrobial resistance in ventilator-associated pneumonia: predictive microbiology and evidence-based therapy. Infect. Dis. Ther. 12, 1527–1552. doi: 10.1007/s40121-023-00820-2
Antimicrobial resistance: global report on surveillance (n.d.). Available at: https://www.who.int/publications/i/item/9789241564748 (accessed July 23, 2024).
Cai, S., Wang, Z., Han, X., Hu, H., Quan, J., Jiang, Y., et al. (2024). The correlation between intestinal colonization and infection of carbapenem-resistant Klebsiella pneumoniae: a systematic review. J. Glob. Antimicrob. Resist. 38, 187–193. doi: 10.1016/j.jgar.2024.04.013
Chinese Medical Association, Chinese Medical Association Journal, Chinese Medical Association General Medicine Society, et al. (2019). Community-acquired pneumonia in adults basic guidelines (practice edition, 2018) [J]. Chin. J. Gen. Pract. 19, 127–133. doi: 10.3760/cma.j.issn.1671-7368.2019.02.006
CLSI (2023). Performance standards for antimicrobial susceptibility testing. 33rd ed.CLSI supplement M100. Clinical and Laboratory Standards Institute. Available at: https://iacld.com/UpFiles/Documents/672a1c7c-d4ad-404e-b10e-97c19e21cdce.pdf (Accessed April 7, 2024).
Córdova-Espinoza, M. G., Giono-Cerezo, S., Sierra-Atanacio, E. G., Escamilla-Gutiérrez, A., Carrillo-Tapia, E., Carrillo-Vázquez, L. I., et al. (2023). Isolation and identification of multidrug-resistant Klebsiella pneumoniae clones from the hospital environment. Pathogens 12:634. doi: 10.3390/pathogens12050634
Deo, R. C. (2015). Machine learning in medicine. Circulation 132, 1920–1930. doi: 10.1161/CIRCULATIONAHA.115.001593
Fernández Peláez, J. M., Sánchez Martín, E., Polo Romero, F. J., and Sáez Méndez, L. (2000). Lung infection caused by aspergillus fumigatus and Nocardia asteroides as complication of glucocorticoid treatment. Med. Clin. (Barc.) 114:358. doi: 10.1016/s0025-7753(00)71294-2
Gao, Y., Chen, L., Wen, Z., Jiang, H., and Feng, J. (2024). Risk factors for multidrug resistance in patients infected with Carbapenem-resistant Klebsiella pneumoniae: a nomogram. Infect. Drug Resist. 17, 4833–4841. doi: 10.2147/IDR.S479374
Gomez-Simmonds, A., and Uhlemann, A.-C. (2017). Clinical implications of genomic adaptation and evolution of Carbapenem-resistant Klebsiella pneumoniae. J. Infect. Dis. 215, S18–S27. doi: 10.1093/infdis/jiw378
Gonzalez-Ferrer, S., Peñaloza, H. F., Budnick, J. A., Bain, W. G., Nordstrom, H. R., Lee, J. S., et al. (2021). Finding order in the Chaos: outstanding questions in Klebsiella pneumoniae pathogenesis. Infect. Immun. 89, e00693–e00620. doi: 10.1128/IAI.00693-20
Gorrie, C. L., Mirceta, M., Wick, R. R., Edwards, D. J., Thomson, N. R., Strugnell, R. A., et al. (2017). Gastrointestinal carriage is a major reservoir of Klebsiella pneumoniae infection in intensive care patients. Clin. Infect. Dis. 65, 208–215. doi: 10.1093/cid/cix270
Grosjean, V., Gressens, S. B., Pham, T., Gaudry, S., Ait-Oufella, H., De Prost, N., et al. (2024). Community-acquired Klebsiella pneumoniae pneumonia in ICU: a multicenter retrospective study. Ann. Intensive Care 14:69. doi: 10.1186/s13613-024-01269-3
Guilhen, C., Miquel, S., Charbonnel, N., Joseph, L., Carrier, G., Forestier, C., et al. (2019). Colonization and immune modulation properties of Klebsiella pneumoniae biofilm-dispersed cells. NPJ Biofilms Microbiomes 5:25. doi: 10.1038/s41522-019-0098-1
He, K., Li, Y., and Liu, H. (2024). Risk and protective factors associated with wound infection after neurosurgical procedures: a meta-analysis. Int. Wound J. 21:e14699. doi: 10.1111/iwj.14699
Jiang, Y., Luo, J., Huang, D., Liu, Y., and Li, D.-D. (2022). Machine learning advances in microbiology: a review of methods and applications. Front. Microbiol. 13:925454. doi: 10.3389/fmicb.2022.925454
Jin, J., Lu, J., Su, X., Xiong, Y., Ma, S., Kong, Y., et al. (2024). Development and validation of an ICU-venous thromboembolism prediction model using machine learning approaches: a multicenter study. Int. J. Gen. Med. 17, 3279–3292. doi: 10.2147/IJGM.S467374
Jones, R. N. (2010). Microbial etiologies of hospital-acquired bacterial pneumonia and ventilator-associated bacterial pneumonia. Clin. Infect. Dis. 51, S81–S87. doi: 10.1086/653053
Kobata, H. (2023). Differential diagnosis and management of disturbance of consciousness: approach to comatose patients. No Shinkei Geka 51, 1009–1020. doi: 10.11477/mf.1436204847
Li, Y., Kumar, S., Zhang, L., Wu, H., and Wu, H. (2023). Characteristics of antibiotic resistance mechanisms and genes of Klebsiella pneumoniae. Open Med. (Wars) 18:20230707. doi: 10.1515/med-2023-0707
Liu, C., and Guo, J. (2019). Hypervirulent Klebsiella pneumoniae (hypermucoviscous and aerobactin positive) infection over 6 years in the elderly in China: antimicrobial resistance patterns, molecular epidemiology and risk factor. Ann. Clin. Microbiol. Antimicrob. 18:4. doi: 10.1186/s12941-018-0302-9
Lopera, C., Monzó, P., Aiello, T. F., Chumbita, M., Peyrony, O., Gallardo-Pizarro, A., et al. (2024). Prevalence and impact of multidrug-resistant bacteria in solid cancer patients with bloodstream infection: a 25-year trend analysis. Microbiol. Spectr. 12:e0296123. doi: 10.1128/spectrum.02961-23
Lou, T., Du, X., Zhang, P., Shi, Q., Han, X., Lan, P., et al. (2022). Risk factors for infection and mortality caused by carbapenem-resistant Klebsiella pneumoniae: a large multicentre case-control and cohort study. J. Infect. 84, 637–647. doi: 10.1016/j.jinf.2022.03.010
Luo, Y., Cui, X., Zhou, J., Zhuang, Y., Zheng, C., Su, Q., et al. (2024). Development and validation of a clinical nomogram for predicting complications from pediatric multiple magnet ingestion: a large retrospective study. Am. J. Gastroenterol. doi: 10.14309/ajg.0000000000002983
Mendapara, K. (2024). Development and evaluation of a chronic kidney disease risk prediction model using random forest. Front. Genet. 15:1409755. doi: 10.3389/fgene.2024.1409755
Mishra, A., Tabassum, N., Aggarwal, A., Kim, Y.-M., and Khan, F. (2024). Artificial intelligence-driven analysis of antimicrobial-resistant and biofilm-forming pathogens on biotic and abiotic surfaces. Antibiotics (Basel) 13:788. doi: 10.3390/antibiotics13080788
Podschun, R., and Ullmann, U. (1998). Klebsiella spp. as nosocomial pathogens: epidemiology, taxonomy, typing methods, and pathogenicity factors. Clin. Microbiol. Rev. 11, 589–603. doi: 10.1128/CMR.11.4.589
Seyer Cagatan, A., Taiwo Mustapha, M., Bagkur, C., Sanlidag, T., and Ozsahin, D. U. (2022). An alternative diagnostic method for C. neoformans: preliminary results of deep-learning based detection model. Diagnostics (Basel) 13:81. doi: 10.3390/diagnostics13010081
Subspecialty Group of Infectious Diseases, Respiratory Society, Chinese Medical Association (2018). Chinese guidelines for the diagnosis and treatment of hospital-acquired pneumonia and ventilator-associated pneumonia in adults (2018 edition) [J]. Chin. J. Tubere. Respir. Dis. 41, 255–280. doi: 10.3760/cma.j.issn.1001-0939.2018.04.006
Vornhagen, J., Rao, K., and Bachman, M. A. (2024). Gut community structure as a risk factor for infection in Klebsiella pneumoniae-colonized patients. mSystems 9, e00786–e00724. doi: 10.1128/msystems.00786-24
Wang, X., Liu, J., and Li, A. (2024). Incidence and risk factors for subsequent infections among rectal carriers with carbapenem-resistant Klebsiella pneumoniae: a systematic review and meta-analysis. J. Hosp. Infect. 145, 11–21. doi: 10.1016/j.jhin.2023.12.002
Wang, Q.-R., Long, J., Wang, C.-C., Hu, J.-L., Lin, N., and Tang, S.-H. (2023). Case report of atypical undernutrition of hypoproteinemia type. Open Life Sci. 18:20220766. doi: 10.1515/biol-2022-0766
Wang, Y., Yao, H.-X., Liu, Z.-Y., Wang, Y.-T., Zhang, S.-W., Song, Y.-Y., et al. (2024). Design of Machine Learning Algorithms and Internal Validation of a kidney risk prediction model for type 2 diabetes mellitus. Int. J. Gen. Med. 17, 2299–2309. doi: 10.2147/IJGM.S449397
Yang, L., Wang, Y., He, X., Liu, X., Sui, H., Wang, X., et al. (2022). Development and validation of a prognostic dynamic nomogram for in-hospital mortality in patients with Stanford type B aortic dissection. Front. Cardiovasc. Med. 9:1099055. doi: 10.3389/fcvm.2022.1099055
Zhu, L., Liang, L., Hui, J., Lu, J., Yang, R., He, Q., et al. (2023). Relationship between antibiotic exposure and carbapenem-resistant Klebsiella pneumoniae infection within four types of control patients: a systematic review and meta-analysis. J. Glob. Antimicrob. Resist. 33, 137–151. doi: 10.1016/j.jgar.2023.02.020
Keywords: Klebsiella pneumoniae, colonization, infection, sputum culture, risk factors, machine learning, clinical prediction model
Citation: Zhang X, Zhang X, Zhang D, Xu J, Zhang J and Zhang X (2025) The clinical prediction model to distinguish between colonization and infection by Klebsiella pneumoniae. Front. Microbiol. 15:1508030. doi: 10.3389/fmicb.2024.1508030
Received: 08 October 2024; Accepted: 30 December 2024;
Published: 23 January 2025.
Edited by:
Yu-Dong Yao, Stevens Institute of Technology, United StatesReviewed by:
Lu Liu, Temple University, United StatesCopyright © 2025 Zhang, Zhang, Zhang, Xu, Zhang and Zhang. This is an open-access article distributed under the terms of the Creative Commons Attribution License (CC BY). The use, distribution or reproduction in other forums is permitted, provided the original author(s) and the copyright owner(s) are credited and that the original publication in this journal is cited, in accordance with accepted academic practice. No use, distribution or reproduction is permitted which does not comply with these terms.
*Correspondence: Jingping Zhang, empwODA5MzAyQDE2My5jb20=; Xin Zhang, emhhbmd4aW44MDA3MDVAMTYzLmNvbQ==
†These authors have contributed equally to this work and share first authorship
‡These authors have contributed equally to this work
Disclaimer: All claims expressed in this article are solely those of the authors and do not necessarily represent those of their affiliated organizations, or those of the publisher, the editors and the reviewers. Any product that may be evaluated in this article or claim that may be made by its manufacturer is not guaranteed or endorsed by the publisher.
Research integrity at Frontiers
Learn more about the work of our research integrity team to safeguard the quality of each article we publish.