- 1G. Oppenheimer Center for Neurobiology of Stress and Resilience at UCLA, Los Angeles, CA, United States
- 2UCLA Vatche and Tamar Manoukian Division of Digestive Diseases, Los Angeles, CA, United States
- 3David Geffen School of Medicine at UCLA, Los Angeles, CA, United States
- 4Goodman Luskin Microbiome Center at UCLA, Los Angeles, CA, United States
- 5Department of Medicine, University of California, Los Angeles, Los Angeles, CA, United States
- 6Department of Community Health Sciences Fielding School of Public Health, University of California, Los Angeles, Los Angeles, CA, United States
- 7California Center for Population Research, UCLA, Los Angeles, CA, United States
Background: Discrimination is a recognized psychosocial stressor that has been linked to various negative health outcomes. This study explored the impact of discrimination on gut health, specifically focusing on microbiome changes, predicted metagenomic differences, transcriptomic profiles, and the potential for using a multi-omic approach to predict discrimination to identify discrimination status for an individual. Methods: We conducted a comprehensive investigation involving male and premenopausal female participants, using the Everyday Discrimination Scale to classify them into either high or low discrimination. Multiple questionnaires were administered to evaluate participants’ physiological, psychological, and perceived stressors. Two diet questionnaires were also administered. Stool samples were collected for microbiome analysis and RNA sequencing. Microbial composition changes were analyzed using the Shannon index and Chao1 richness estimator for alpha diversity and the Aitchison distance metric for beta diversity. Differential abundance was evaluated using MaAsLin2, followed by metatranscriptomics sequencing and annotation. A multi-omic approach utilizing random forest was used to assess the predictability of discrimination.
Results: The study results showed that high discrimination was linked to higher gut microbiome species richness (Chao1, p = 0.02) and significant beta diversity differences (p = 0.04). Prevotella and Ruminococcaceae were both less abundant in the high discrimination group. High discrimination participants also reported higher levels of depression, anxiety, perceived stress, early life adversity, visceral sensitivity, and neuroticism than those in the low discrimination group. Gene expression analysis revealed distinctive patterns, with significant changes in genes associated with environmental sensing (two-component system) and metabolic pathways. In a plot comparing gene transcription to DNA content, certain genes showed higher expression levels in participants who experienced both high and low levels of discrimination. Our random forest classifier demonstrated the capability to accurately differentiate individuals with high and low discrimination in our training cohort (AUC = 0.91).
Conclusion: These findings illuminate the substantial impact of discrimination on gut health, encompassing microbiome composition, gene expression, and functional pathways. These findings suggest that discrimination is associated with internal biological changes that can be associated with negative health outcomes, opening research to examine novel pathways that can be used to mitigate the negative health effects of discrimination.
Introduction
Discrimination, or the differential treatment of individuals based on existing or perceived membership to a specific identity, plays a significant role in the social structures that perpetuate inequality and injustices (Schmitt and Branscombe, 2002; Slopen and Williams, 2014). The role of discrimination and one’s place in either an “advantaged” or “disadvantaged” group has clear implications for their prospective healthcare outcomes (Berger and Sarnyai, 2015). However, the biological pathways by which discrimination impacts mental and physical health remain unclear.
Previous research has consistently revealed that belonging to disadvantaged groups is directly associated with poorer health outcomes (Melchior et al., 2007; Banerjee, 2016; Killen et al., 2016). Several pathways have been described to link discrimination to health, with the most popular being the stress response provoked by discrimination via the activation of the hypothalamic–pituitary–adrenal (HPA) axis. Physical arousal that results from the experience of discrimination can activate the HPA axis, resulting in the release of cortisol (Keller et al., 2017). Cortisol release as a result of dysregulation of the HPA axis has been implicated in the pathophysiology of anxiety and depression (Tetel et al., 2018; Lyte et al., 2020; Foster et al., 2017).
Recent studies, however, have pushed for an investigation of the gut microbiome in influencing the psychosocial stress response via the gut–brain axis as well (De Palma et al., 2015; Moussaoui et al., 2017). The gut microbiome and the brain communicate through various pathways, such as the HPA axis and the immune system (Dong et al., 2023; Cryan et al., 2019; Marcondes Ávila et al., 2020). Stress can dysregulate these pathways, interrupting the communication between the gut and the brain. It is well documented that stress-induced changes to the brain can result in a compromised brain–gut signaling network, leading to alterations in the gut microbiome, increased intestinal permeability, microbial dysbiosis, and changes to microbial gene expression (Keita and Söderholm, 2010; Moreira et al., 2016; Ritter et al., 2012; Vetter et al., 2010; Higginson and McNamara, 2016; Van Dyken and Lacoste, 2018). These changes can subsequently result in immune system activation and thus an inflammatory response by the host (Sarubbo et al., 2022; Chakrabarti et al., 2022). Despite emerging evidence linking stress, the gut–brain axis, and health outcomes, there is still a significant gap in understanding how discrimination specifically impacts these biological pathways. Understanding these effects is crucial in revealing novel biomarkers of discrimination-related stress and providing insights into targeted interventions to mitigate adverse health impacts.
The purpose of this study was to understand the exact impact of discrimination on the gut microbiome and its correlation with healthcare outcomes. To comprehensively understand how discrimination can affect biological pathways linked to outcomes in health, we performed a detailed analysis of the effects of discrimination on the gut microbiome in a racially diverse population, specifically focusing on changes to the gut microbiome composition, predicted metagenome differences, and transcriptomic profiles. These alterations were also examined in correlation with health outcomes such as depression, anxiety, and perceived stress. Using these results, we evaluated the potential for using a random forest classifier to predict discrimination to identify discrimination status for an individual.
Materials and methods
Ethics approval and consent to participate
The procedures performed were approved by the Institutional Review Board at the University of California, Los Angeles, Office of Protection for Research Subjects. All participants provided written informed consent.
Study cohort
Individuals were recruited from clinics and communities in Los Angeles, California. To be eligible for the study, participants had to be at least 18 years old. We excluded individuals who took antibiotics or probiotics in the 3 months preceding the study. Because this part of the study was part of a larger study involving magnetic resonance scanning, participants were also excluded if they used analgesic drugs or medications that interfered with the central nervous system, weighed over 400 pounds (due to magnetic resonance imaging scanning weight limits), or were lefthanded (since there is variability in brain scans due to hand dominance). The initial cohort consisted of 165 adults, and the final sample consisted of 154 adults. Participant data included the following: blood samples for genetic expression, stool samples for microbial and transcriptomic analysis, anthropometrics, diet history, and verified medical survey questionnaires. Participants self-reported race/ethnicity (Asian American, Black, Hispanic, or White). For female participants, only premenopausal individuals were enrolled, and biological samples were collected in the follicular phase of their menstruation cycle, as determined by the reported date of their last menstrual period. This approach aimed to minimize any hormonal influence on the biological and microbiome samples.
Questionnaires
The following validated questionnaires were used to assess the cohort members: Everyday Discrimination Scale (EDS), Connor Davidson Resilience Scale (CD-RISC), Early Traumatic Inventory (ETI), Hospital Anxiety and Depression (HAD) scale, International Personality Item Pool (IPIP), Perceived Stress Scale (PSS), State–Trait Anxiety Inventory (STAI), Visceral Sensitivity Index (VSI), Socioeconomic Status (SES), Patient Health Questionnaire-15 (PHQ-15), and Short Form Health Survey (SF12). All participants also participated in a Diet History Questionnaire (DHQ) III and the UCLA Diet Checklist (Lenhart et al., 2022).
EDS was used to assess discrimination. It measures routine and chronic experiences of unjust treatment, categorizing subjects into a high EDS level or a low EDS (Michaels et al., 2019). The questionnaire has been widely used in diverse populations, consisting of nine-item questions to assess how frequently someone perceives discrimination in their day-to-day life with frequency rate options of ‘never,’ ‘less than once a year,’ ‘a few times a year,’ ‘a few times a month,’ ‘at least once a week,’ and ‘almost every day.’ These answers are assigned a score on a Likert scale, with ‘never’ equating to zero and ‘almost every day’ equating to a five and a maximum score of 50. Follow-up questions are asked, querying the responder to speculate on the main reason for these experiences if they answered a few times a year or more frequently to any initial questions.
The CD-RISC, ETI, HAD, IPIP, PSS, STAI, and VSI were used as psychological and symptom instruments. The CD-RISC was used to measure stress-coping ability by assessing resilience (Connor and Davidson, 2003). It includes 25 items, each scored on a scale from 0 to 4, with higher scores reflecting greater resilience in respondents. The ETI was used to measure self-reported childhood trauma, including questions from general, physical, emotional, and sexual trauma, as well as additional questions for serious trauma (Jeon et al., 2012). The HAD scale assessed anxiety and depression symptoms in the participants (Edelstein et al., 2010). Respondents were asked to rate 14 different items on a 4-point severity scale, producing results for both anxiety and depression. The IPIP was used to assess personality, categorizing an individual into the big five personality traits (Ypofanti et al., 2015). The PSS was used to measure one’s perception of stress based on their evaluation of the degree to which specific situations are categorized as stressful (Nater and Ali, 2020). The STAI was used to assess anxiety in cohort members (Carmin and Ownby, 2010). The questionnaire includes four separate dimensions of “state” and “trait” anxiety, including feelings of tension, worry, and more. The VSI was used to measure gastrointestinal symptom-specific anxiety (Trieschmann et al., 2022). The questionnaire consists of 15 items ranked on the same Likert scale described previously, with examples of items including statements such as “I worry that abdominal pain might be due to a serious illness” and “I am afraid that when I experience stomach discomfort, it will get worse.”
The SF12 was used to assess an individual’s quality of life by quantifying the impact of health on daily life (Huo et al., 2018). Both the DHQ-III and UCLA Diet Checklist were used for the evaluation of participants’ diets.
Statistical analyses
Differences in baseline demographic characteristics were assessed using Student’s t-test for continuous variables and the chi-squared test for categorical variables. For the purposes of our analyses, we dichotomized EDS to either “high discrimination” (EDI > =6) or “low discrimination” (EDI < 6) using the median score of our study population. This process determined a cutoff using the study population similar to previously published studies (Chang et al., 2018).
Changes to microbial composition were analyzed using several metrics. Raw microbiome reads were processed through DADA2 (Callahan et al., 2016). The microbiome dataset was rarefied to a standardized sequence depth of 34,222 reads. Alpha diversity—the diversity within a sample—was computed using the Shannon (a measure of species evenness) and Chao1 (a measure of species richness) indices through QIIME2. The statistical significance of the Shannon and Chao1 indices was determined using analysis of variance (ANOVA) using the aov function in R. Beta diversity—the variability in composition among the community—was analyzed using the robust Aitchison distance metric within the DEICODE package of QIIME2 (Sawyer et al., 2012). Significance in beta diversity was evaluated using permutational multivariate analysis of variance, specifically employing the ‘adonis’ package in R (Version 4.1.2), adjusting for sex, age, body mass index (BMI), and diet. Differential abundance analysis of genera was computed using MaAsLin2 (Mallick et al., 2021).
Fecal samples were sent to Viome Life Sciences, Inc., for RNA extraction, metatranscriptomics sequencing, and annotation (Hatch et al., 2019). Functional annotation of genes using metatranscriptomics allowed for the identification and comparison of genes expressed by the high and low discrimination groups. RNA extraction was performed via bead beating; DNase was used to degrade DNA, and subtractive hybridization was used to deplete 16S/23S ribosomal RNA. The resulting RNA was used to prepare sequencing libraries. The libraries were processed through 150×2 paired-end sequencing on Illumina NovaSeq. Sequence reads were aligned to a precomputed unique k-mers database. To perform functional annotation, the sequence reads were aligned to the MetaHIT Consortium integrated gene catalog (MetaHIT Consortium, 2014).
PICRUst2, a reputable tool used to attain metagenomic data from 16S rRNA compositional data, was used to infer metagenomic data from the 16S rRNA sequencing data of each sample. The 16S rRNA sequencing data were inputted into the PICRUst2 program and normalized by copy number. Default parameters were used. The Kyoto Encyclopedia of Genes and Genomes (KEGG) database was used to categorize subsequent metagenes by function. DESeq2 was used to identify differences in predicted metagenes by discrimination, with p-values adjusted for multiple hypothesis testing.
Using a random forest classifier, a model incorporating 16S data, transcriptomic data, and metagenomic data was created using the variables that had at least a p-value of <0.1 to classify participants as either high or low discrimination. Random forest analysis was conducted utilizing the `randomForest` package in R. The analysis was executed with default settings, including a forest size of 1,000 trees. To assess the model’s performance and generalization ability, k-fold cross-validation was employed, with k set to a value of 10. This procedure ensures robustness and minimizes overfitting by partitioning the dataset into k equally sized folds, where each fold serves as a validation set while the remaining data are utilized for training. The process is repeated k times, with each fold serving once as the validation set. This approach allows for an unbiased estimation of the model’s predictive performance.
Clinical measures as evaluated by the abovementioned questionnaires and demographic data were correlated with discrimination using analysis of variance for continuous variables and the chi-square tests for categorical variables.
Results
Participant characteristics
Of the 154 participants, 141 submitted fecal samples for analysis. The participants consisted of 30 Asian individuals (13 high EDS and 17 low EDS), 18 Black individuals (11 high EDS and 7 low EDS), 57 Hispanic individuals (32 high EDS and 25 low EDS), and 36 White individuals (19 high EDS and 17 low EDS) (Table 1).
No significant differences were observed in the proportion of missing microbiome or metabolomics data between the groups (p = 0.20).
Of the 154 participants, 80 were classified as high discrimination and 74 were classified as low discrimination. Compared to the low discrimination group, the high discrimination group presented with higher levels of depression (p = 0.009), anxiety (p = 0.009), perceived stress (p = 0.001), early life adversity (p = 0.009), visceral sensitivity (p < 0.001), neuroticism (p = 0.01), and overall poorer scores for physical (p = 0.02) and mental (p = 0.01) health. No significant differences were observed in age, BMI, education, marital status, or diet between the high and the low discrimination groups.
Discrimination introduces changes to microbiome composition
Analysis of the gut microbiome between the high and low discrimination groups indicates that discrimination is associated with clear microbiome changes. Both the Chao1 richness estimator and Shannon indices were used to measure alpha diversity for the high and low discrimination groups. Those with high levels of discrimination had higher levels of species richness (Figure 1A). A similar trend was seen with the Shannon index (p-value = 0.05) (Figure 1B). Beta diversity showed a statistically significant difference between the two groups (p-value = 0.04) (Figure 1C).
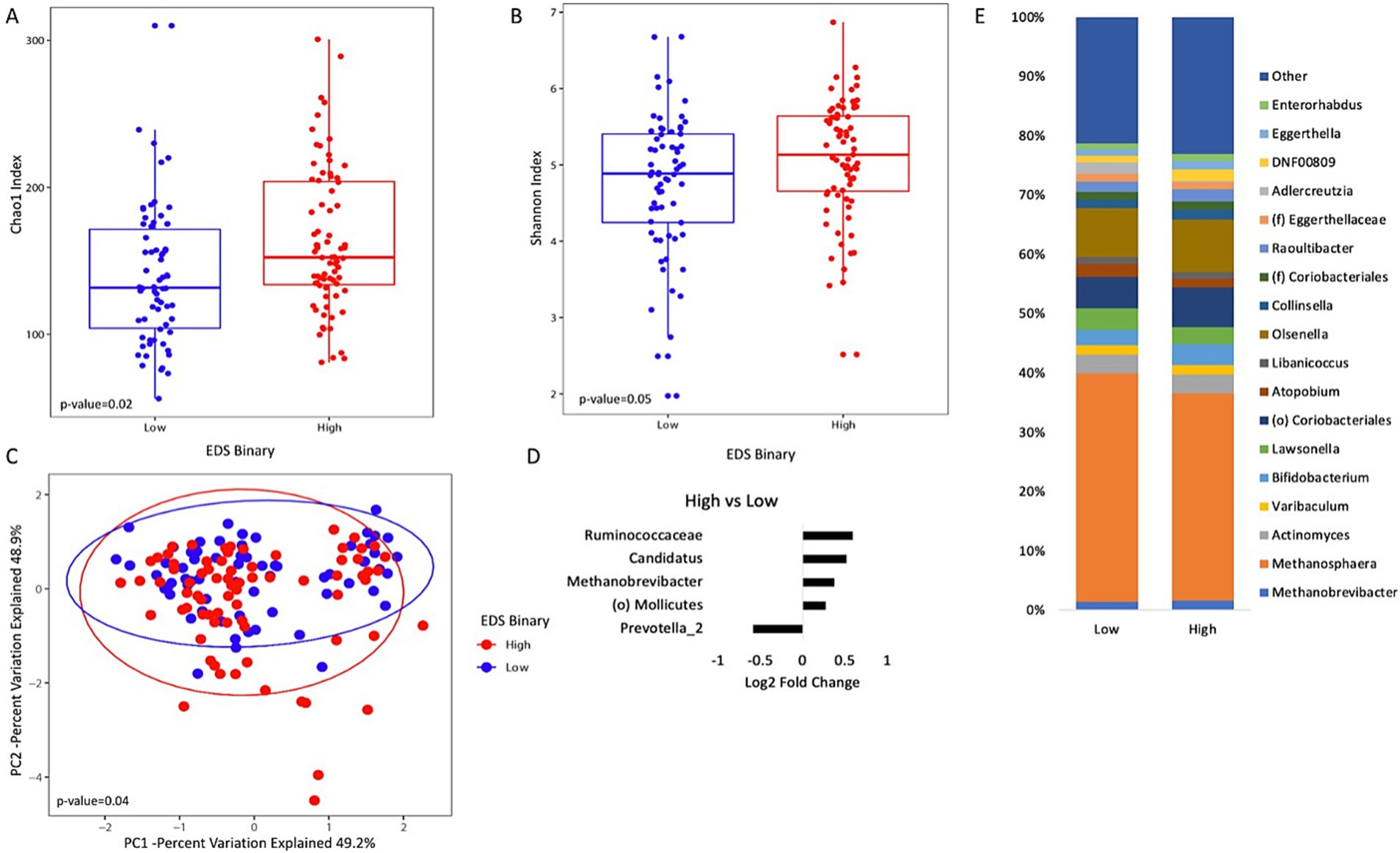
Figure 1. Analysis of gut microbiome diversity and taxonomic composition in the high and low discrimination groups. (A) Box plot of Chao1 index (species richness) and (B) Shannon index (species evenness). (C) Principal coordinate analysis plot of the microbiome composition by the high and low discrimination groups as measured by the Everyday Discrimination Scale (EDS). (D) MaAsLin2 output that shows which genera were differentially abundant in the high discrimination group compared to the low discrimination group. (E) Taxonomic plots of all genera by discrimination status. Only genera with a relative abundance of >1% are represented.
Using MAASLIN2 to assess the abundance of specific genera revealed that the abundance of Prevotella specifically was lower in the high discrimination group than in the low discrimination group (Figure 1D). Genera belonging to Ruminococcaceae, Candidatus, Methanobrevibacter, and the order Mollicutes were higher in the high discrimination group than the low discrimination group. A taxonomic plot summarizing major genera is shown in Figure 1E.
Analysis of predicted metagenomic and transcriptomic profiles reveals potential biomarkers for high discrimination group
Both the predicted metagenome and transcriptomics show no significant differences between the high and low discrimination groups based on principal component analysis (Figures 2A,B). Differential abundance testing of the predicted metagenome showed that there were higher amounts of electrochemical potential-driven transporters (K11689)—proteins that rely on electrochemical gradients to facilitate secondary active transport—and micrococcal nuclease (K01174)—enzymes that cleave regions between nucleosomes—in the high discrimination group than in the low discrimination group (Figure 2C). Differential abundance testing of the transcriptomic data showed less antigen processing and presentation (K01369) and nucleotide excision repair (K03657) in the high discrimination group than in the low discrimination group (Figure 2D). Generally, the pattern of RNA relative abundances to the DNA relative abundance between the high and low discrimination groups was similar but not identical (Figures 2E,F).
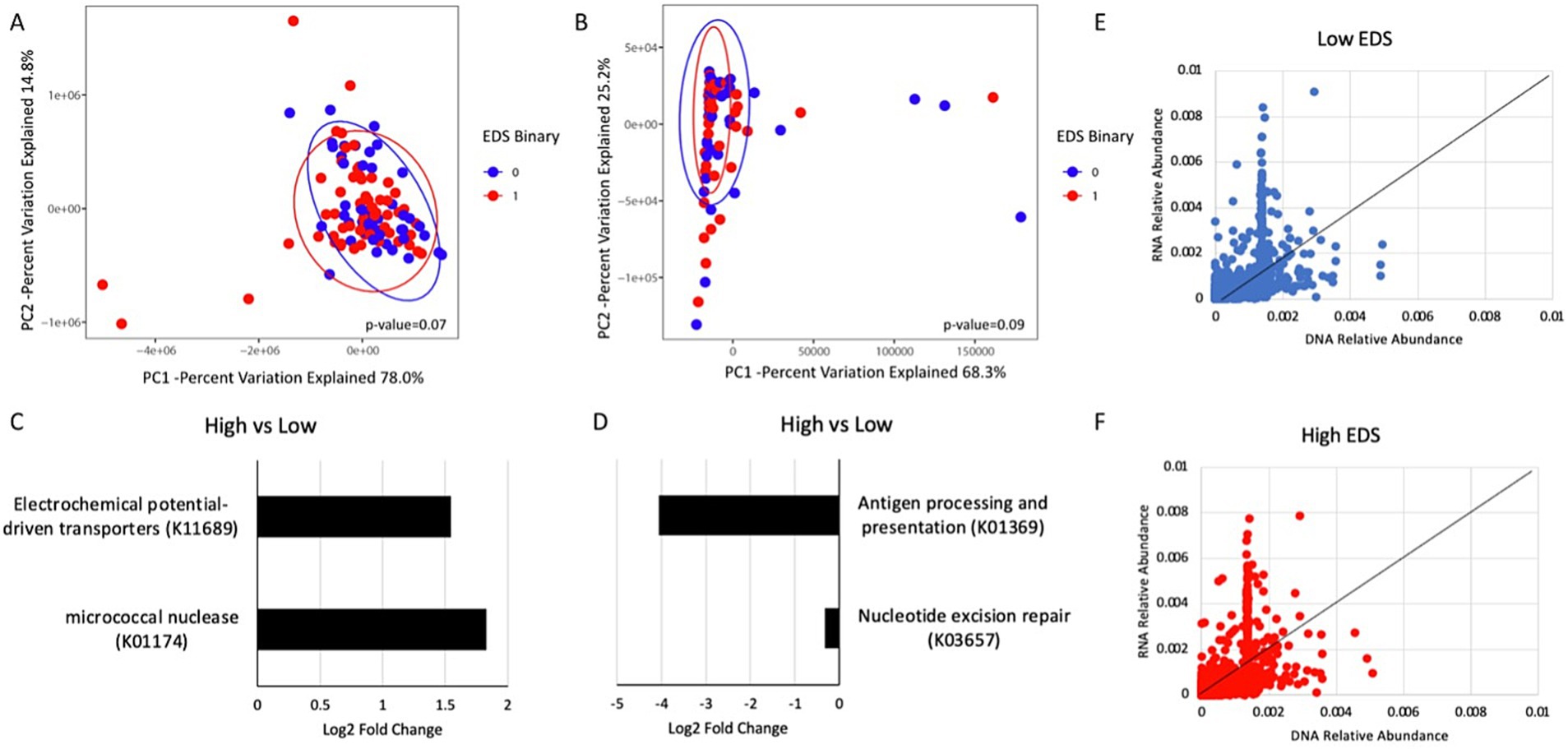
Figure 2. Analysis of predicted metagenomic and transcriptomic profiles in the high and low discrimination groups. (A) Principal component analysis plot of predicted metagenomic data and (B) transcriptomic data by discrimination. (C) DESeq2 analysis showing differential abundant predicted metagenes in the high discrimination group compared to the low discrimination group. (D) DESeq2 analysis showing differential abundant transcripts between the high discrimination group and the low discrimination group. (E) DNA relative abundance to RNA relative abundance of each gene in the participants with low discrimination and (F) high discrimination.
A closer analysis of gene transcription relative to DNA content in participants from the low and high discrimination groups revealed that several genes appear to be transcribed at significantly different levels between the two groups (Figure 3A). Seven genes—K13924 (bacterial chemotaxis), K07795 (two-component system), K00998 (biosynthesis and metabolic pathways), K01069 (pyruvate metabolism), K01029 (metabolic pathways), K07678 (two-component system), and K01758 (biosynthesis and metabolic pathways)—had higher transcription rates in individuals with high discrimination levels. Six genes—K12983 (lipopolysaccharide biosynthesis), K07670 (two-component system), K01659 (metabolic pathways), K05881 (metabolic pathways), K02771 (fructose and mannose metabolism), and K10742 (DNA replication)—had lower transcription rates in individuals with high discrimination levels.
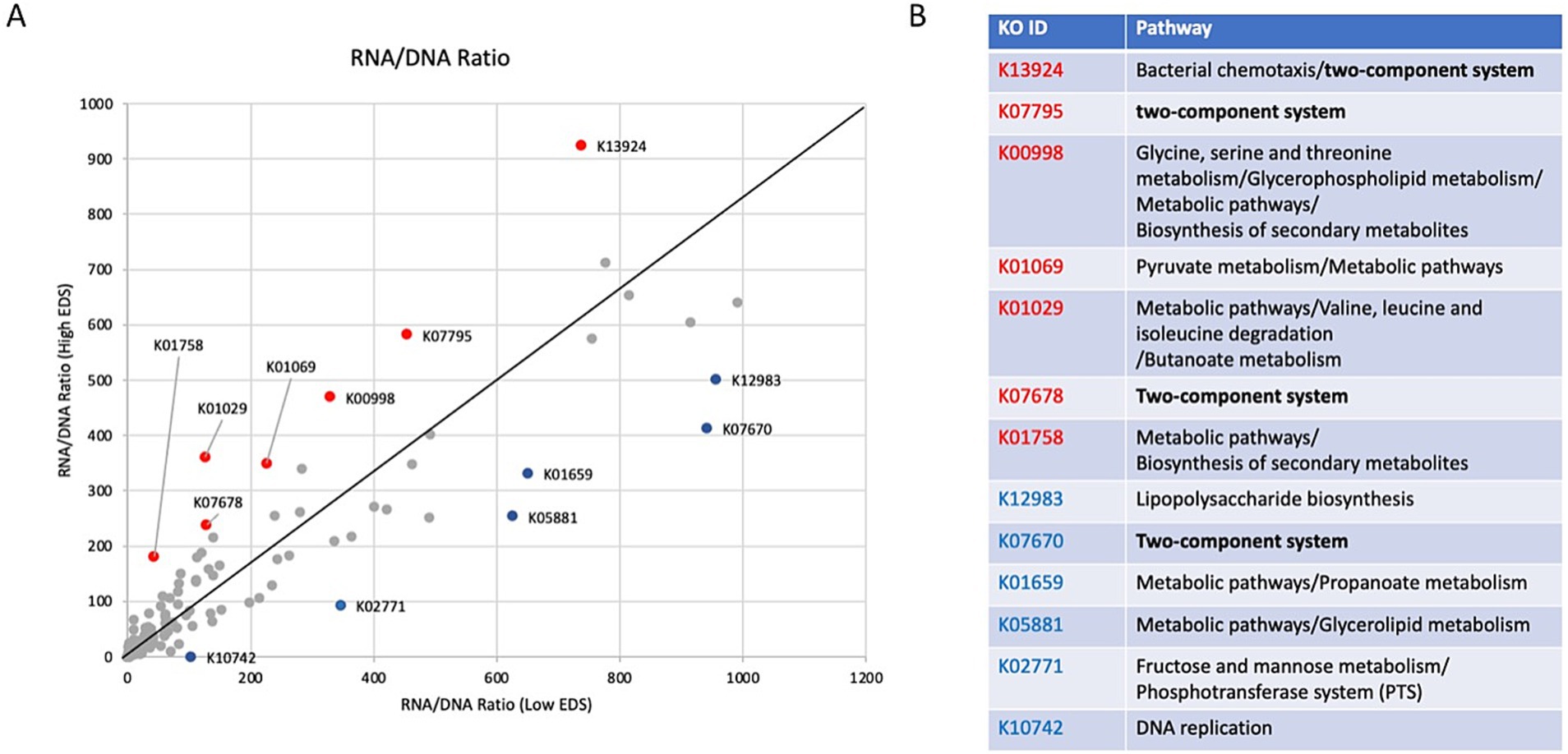
Figure 3. Comparative analysis of RNA-to-DNA ratios and functional descriptions of discrimination-associated genes. (A) Graphical comparison of RNA-to-DNA ratios between the low and high discrimination groups. The genes above the line indicate higher transcription rates than those with high discrimination levels, while those below the line represent higher transcription levels in the low discrimination group. Of those genes, the ones highlighted in red and blue represent transcription rates two standard deviations greater than the baseline in the high and low discrimination groups. (B) Descriptions of functions for genes indicated to be significantly different between the high and low discrimination groups in figure.
In general, the genes with the most significant changes were associated with environmental sensing (two-component system) and metabolic pathways (Figure 3B).
Prediction of discrimination status using a multi-omic approach
Using a random forest classifier, we showed that a dataset based on the gut microbiome composition, metagenome, and transcriptome could accurately identify participants in the cohort as either in the high or low discrimination group. The model’s receiver operating characteristic (ROC) curve demonstrated a high accuracy, with an area under the curve (AUC) of 0.91 (Figure 4). A model with only microbiome data had an AUC of 0.79, a model with only predicted metagenome data had an AUC of 0.89, and a model with only transcriptomic data had an AUC of 0.86.
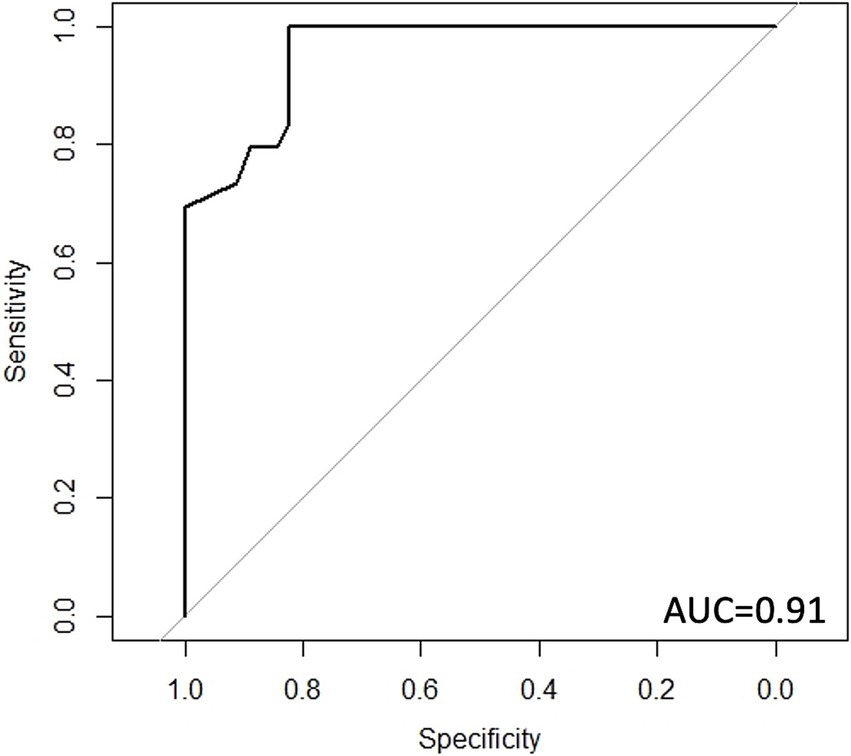
Figure 4. Receiver operating characteristic (ROC) curve for discrimination status classification. ROC curve for random forest classifier using microbiome data, predicted metagenomic data, and transcriptomic data. AUC: area under the curve.
Discussion
Discrimination is associated with significant changes in the gut microbiome profile affecting both the composition of the gut microbiome and its gene expression. A model that encompasses microbiome, metagenomic, and transcriptomic data has a high accuracy for classifying those with high discrimination.
Discrimination impacts psychological and physical symptoms
Discrimination has been increasingly recognized as a psychosocial stressor with implications for health outcomes (Sawyer et al., 2012). In our study, individuals in the high discrimination group not only exhibited significantly higher levels of adverse psychological traits, including depression, anxiety, and perceived stress, but also reported higher occasions of life adversity and neuroticism. The high discrimination group also had poorer scores overall in physical and mental health. These findings align with existing literature that highlights the deleterious effects of stressors such as discrimination on physical and mental health (Sawyer et al., 2012; Brandt et al., 2022). For example, past studies have linked discrimination to a higher incidence of anxiety and obesity (Chang et al., 2018; MacIntyre et al., 2023; Panza et al., 2019).
The association between visceral sensitivity and discrimination specifically reflects the relationship between psychological stress and physiological response. Visceral sensitivity is the experience of discomfort in an individual’s internal organs (Zhou and Verne, 2011). Chronic stressors, such as discrimination, are considered capable of dysregulating the body’s HPA axis and autonomic nervous system—both critical to the human stress response (Herman et al., 2016; Stephens and Wand, 2012). In our study, the high discrimination group reported heightened visceral sensitivity compared to the low discrimination group. Such physiological changes may contribute to compromised gastrointestinal function.
Discrimination impacts the gut microbiome
Analyses of the gut microbiome revealed significant differences between the high and low discrimination groups, indicating the potential role of discrimination on the composition of the gut microbiome. One of the most notable observations from these analyses was that the median value for the Chao1 richness estimator was higher for the high discrimination group than for the low discrimination group, suggesting that the high discrimination group has greater species richness. Alpha diversity is used as a common indicator for assessing gut microbiota health and can be closely associated with disease status (Gong et al., 2016). While the exact relationship between higher species richness and inflammation still remains unclear, several studies imply that a higher alpha diversity is considered a biomarker for high-stress environments (Kelly et al., 2021). In this context, a higher Chao1 index implies higher species richness as a response to environmental stressors that can be associated with discriminatory experiences. Taxonomic analysis of the gut microbiome showed that the most notable difference was a decrease in the abundance of Prevotella in individuals experiencing high levels of discrimination. Prevotella species are associated with the production of short-chain fatty acids and the stimulation of regulatory T-cell production by the immune system. These regulatory T cells play a significant role in suppressing excessive inflammation and maintaining immune homeostasis (Larsen, 2017). Prevotella is also linked to various metabolic processes, suggesting that its alteration in individuals with high discrimination may disrupt the host’s health more broadly.
From the microbial gene expression analysis, the differential expression of environmental sensing genes in the high discrimination group is suggestive of an adaptive response by the microbiome to maintain homeostasis in a high-stress environment (Karl et al., 2018; Wu and Wu, 2012). The high discrimination group had less antigen processing and presentation, indicating a weaker host immune response, and less expression of nucleotide excision repair, indicating a potentially weakened DNA damage response (Cheong and Nagel, 2022). The high discrimination group also had significantly higher amounts of micrococcal nuclease (K01174). Micrococcal nuclease catalyzes the hydrolysis of nucleic acids (Alexander et al., 1961). An increased abundance of micrococcal nucleases in the high discrimination group may reflect a greater need for DNA repair or nucleic acid turnover. This enhanced nuclease activity is potentially indicative of a greater need for DNA repair mechanisms due to discriminatory stressors that induce inflammation, thereby increasing the likelihood of DNA damage (Nastasi et al., 2020).
Prediction of discrimination status
The multi-omic approach, one that combines metagenomic, transcriptomic, and microbiome data, reflected high accuracy in identifying the discrimination status of participants in the trained cohort. This implies that these changes in the gut microbiome are highly linked to discrimination status. While this study has many strengths, such as the use of transcriptomic analysis and comprehensive microbiome analysis, there are several limitations. Because of the sample size, we were unable to split the cohort into a training and validation cohort. While the model was internally validated using k-fold cross-validation, an external cohort will be needed to validate these results. Furthermore, the cross-sectional nature of this study cannot imply causality. Longitudinal studies will be needed to examine the effects of early and late-stage discrimination on the effects of microbiome development.
Conclusion and clinical implications
Overall, the findings in this study confirm that there is an association between the gut microbiome and its gene expression to discrimination status. The trends revealed in this study emphasize the need for further research to identify the exact mechanisms underlying these relationships. This study provides an initial step toward understanding how inequality manifests into a whole-body experience. Empowering individuals with knowledge about the potential impact of discrimination on their gut microbiome and, more largely, their health could aid in promoting preventative stress and health management strategies. Individuals coping with the physiological and psychological consequences of discrimination could also be supported by actively modulating their gut microbiota through dietary and probiotic interventions tailored to their individual microbiome profiles. By gaining an understanding of how discriminatory experiences shape the microbiome, we can develop targeted interventions that aim to mitigate health inequities as a result of discriminatory experiences.
Data availability statement
Original datasets are available in a publicly accessible repository: 10.5281/zenodo.13921371.
Ethics statement
The studies involving humans were approved by the Institutional Review Board at the University of California, Los Angeles, Office of Protection for Research Subjects. All participants provided written informed consent. The studies were conducted in accordance with the local legislation and institutional requirements. The participants provided their written informed consent to participate in this study.
Author contributions
TD: Conceptualization, Formal analysis, Methodology, Supervision, Visualization, Writing – original draft, Writing – review & editing. SS: Visualization, Writing – original draft, Writing – review & editing. KP: Visualization, Writing – original draft, Writing – review & editing. GG: Writing – original draft, Writing – review & editing. HB-S: Writing – original draft, Writing – review & editing. MW: Writing – original draft, Writing – review & editing. LK: Writing – original draft, Writing – review & editing. XZ: Data curation, Resources, Writing – original draft, Writing – review & editing. JL: Data curation, Resources, Writing – original draft, Writing – review & editing. AV: Data curation, Resources, Writing – original draft, Writing – review & editing. AC: Conceptualization, Data curation, Formal analysis, Funding acquisition, Methodology, Resources, Supervision, Visualization, Writing – original draft, Writing – review & editing.
Funding
The author(s) declare that financial support was received for the research, authorship, and/or publication of this article. This research was supported by grants from the National Institutes of Health including R01 MD015904 (AC), K23 DK106528 (AC), R03 DK121025 (AC), T32 DK07180 (TD), and ULTR001881/DK041301 [UCLA CURE/CTSI Pilot and Feasibility Study (AC)].
Acknowledgments
We would like to acknowledge the assistance of the Bioinformatics and Statistics Core, Microbiome Core, and the Biorepository Core of the UCLA Goodman-Luskin Microbiome Center for their assistance with various processing, storage, and analyses of the current manuscript.
Conflict of interest
AC was a Scientific Consultant to Yamaha.
The remaining authors declare that the research was conducted in the absence of any commercial or financial relationships that could be construed as a potential conflict of interest.
The author(s) declared that they were an editorial board member of Frontiers, at the time of submission. This had no impact on the peer review process and the final decision.
Publisher’s note
All claims expressed in this article are solely those of the authors and do not necessarily represent those of their affiliated organizations, or those of the publisher, the editors and the reviewers. Any product that may be evaluated in this article, or claim that may be made by its manufacturer, is not guaranteed or endorsed by the publisher.
References
Alexander, M., Heppel, L. A., and Hurwitz, J. (1961). The purification and properties of Micrococcal nuclease. J. Biol. Chem. 236, 3014–3019. doi: 10.1016/S0021-9258(19)76420-5
Banerjee, P. A. (2016). A systematic review of factors linked to poor academic performance of disadvantaged students in science and maths in schools. Cogent Educ. 3:1178441. doi: 10.1080/2331186X.2016.1178441
Berger, M., and Sarnyai, Z. (2015). “More than skin deep”: stress neurobiology and mental health consequences of racial discrimination. Stress 18, 1–10. doi: 10.3109/10253890.2014.989204
Brandt, L., Liu, S., Heim, C., and Heinz, A. (2022). The effects of social isolation stress and discrimination on mental health. Transl. Psychiatry 12:398. doi: 10.1038/s41398-022-02178-4
Callahan, B. J., McMurdie, P. J., Rosen, M. J., Han, A. W., Johnson, A. J. A., and Holmes, S. P. (2016). DADA2: high-resolution sample inference from Illumina amplicon data. Nat. Methods 13, 581–583. doi: 10.1038/nmeth.3869
Carmin, C., and Ownby, R. L. (2010). “Assessment of anxiety in older adults” In Handbook of assessment in clinical gerontology 2nd Edn. ed. P. A. Lichtenberg (Elsevier), 45–60.
Chakrabarti, A., Geurts, L., Hoyles, L., Iozzo, P., Kraneveld, A. D., la Fata, G., et al. (2022). The microbiota–gut–brain axis: pathways to better brain health. Perspectives on what we know, what we need to investigate and how to put knowledge into practice. Cell. Mol. Life Sci. 79:80. doi: 10.1007/s00018-021-04060-w
Chang, Y., Brown, C., Bromberger, J. T., and Matthews, K. A. (2018). Everyday discrimination and metabolic syndrome incidence in a racially/ethnically diverse sample: study of Women’s health across the nation. Psychosom. Med. 80, 114–121. doi: 10.1097/PSY.0000000000000516
Cheong, A., and Nagel, Z. D. (2022). Human variation in DNA repair, immune function, and Cancer risk. Front. Immunol. 13:899574. doi: 10.3389/fimmu.2022.899574
Connor, K. M., and Davidson, J. R. T. (2003). Development of a new resilience scale: the Connor-Davidson resilience scale (CD-RISC). Depress. Anxiety. 18, 76–82. doi: 10.1002/da.10113
Cryan, J. F., O'Riordan, K. J., Cowan, C. S. M., Sandhu, K. V., Bastiaanssen, T. F. S., Boehme, M., et al. (2019). The microbiota-gut-brain Axis. Physiol. Rev. 99, 1877–2013. doi: 10.1152/physrev.00018.2018
De Palma, G., Blennerhassett, P., Lu, J., Deng, Y., Park, A. J., Green, W., et al. (2015). Microbiota and host determinants of behavioural phenotype in maternally separated mice. Nat. Commun. 6:7735. doi: 10.1038/ncomms8735
Dong, T. S., Gee, G. C., Beltran-Sanchez, H., Wang, M., Osadchiy, V., Kilpatrick, L. A., et al. (2023). How discrimination gets under the skin: biological determinants of discrimination associated with dysregulation of the brain-gut microbiome system and psychological symptoms. Biol. Psychiatry 94, 203–214. doi: 10.1016/j.biopsych.2022.10.011
Edelstein, B. A., Drozdick, L. W., and Ciliberti, C. M. (2010). “Chapter 1 - Assessment of Depression and Bereavement in Older Adults” in Lichtenberg, Handbook of Assessment in Clinical Gerontology (Second Edition) (Elsevier: Academic Press), 3–43. doi: 10.1016/B978-0-12-374961-1.10001-6
Foster, J. A., Rinaman, L., and Cryan, J. F. (2017). Stress & the gut-brain axis: regulation by the microbiome. Neurobiol. Stress 7, 124–136. doi: 10.1016/j.ynstr.2017.03.001
Gong, D., Gong, X., Wang, L., Yu, X., and Dong, Q. (2016). Involvement of reduced microbial diversity in inflammatory bowel disease. Gastroenterol. Res. Pract. 2016, 1–7. doi: 10.1155/2016/6951091
Hatch, A., Horne, J., Toma, R., Twibell, B. L., Somerville, K. M., Pelle, B., et al. (2019). A robust Metatranscriptomic Technology for Population-Scale Studies of diet, gut microbiome, and human health. Int. J. Genomics 2019, 1–9. doi: 10.1155/2019/1718741
Herman, J. P., McKlveen, J. M., Ghosal, S., Kopp, B., Wulsin, A., Makinson, R., et al. (2016). “Regulation of the Hypothalamic-Pituitary-Adrenocortical Stress Response Compr Physiol. 6, 603–21. doi: 10.1002/cphy.c150015
Higginson, A. D., and McNamara, J. M. (2016). An adaptive response to uncertainty can lead to weight gain during dieting attempts. Evol. Med. Public Health 2016, 369–380. doi: 10.1093/emph/eow031
Huo, T., Guo, Y., Shenkman, E., and Muller, K. (2018). Assessing the reliability of the short form 12 (SF-12) health survey in adults with mental health conditions: a report from the wellness incentive and navigation (WIN) study. Health Qual. Life Outcomes 16:34. doi: 10.1186/s12955-018-0858-2
Jeon, J.-R., Lee, E. H., Lee, S. W., Jeong, E. G., Kim, J. H., Lee, D., et al. (2012). The early trauma inventory self report-short form: psychometric properties of the Korean version. Psychiatry Investig. 9, 229–235. doi: 10.4306/pi.2012.9.3.229
Karl, J. P., Hatch, A. M., Arcidiacono, S. M., Pearce, S. C., Pantoja-Feliciano, I. G., Doherty, L. A., et al. (2018). Effects of psychological, environmental and physical stressors on the gut microbiota. Front. Microbiol. 9:2013. doi: 10.3389/fmicb.2018.02013
Keita, Å. V., and Söderholm, J. D. (2010). The intestinal barrier and its regulation by neuroimmune factors: intestinal barrier and neuroimmune regulation. Neurogastroenterol. Motil. 22, 718–733. doi: 10.1111/j.1365-2982.2010.01498.x
Keller, J., Gomez, R., Williams, G., Lembke, A., Lazzeroni, L., Murphy, G. M. Jr., et al. (2017). HPA axis in major depression: cortisol, clinical symptomatology and genetic variation predict cognition. Mol. Psychiatry 22, 527–536. doi: 10.1038/mp.2016.120
Kelly, L. S., Apple, C. G., Gharaibeh, R., Pons, E. E., Thompson, C. W., Kannan, K. B., et al. (2021). Stress-related changes in the gut microbiome after trauma. J. Trauma Acute Care Surg. 91, 192–199. doi: 10.1097/TA.0000000000003209
Killen, M., Rutland, A., and Yip, T. (2016). Equity and justice in developmental science: discrimination, social exclusion, and intergroup attitudes. Child Dev. 87, 1317–1336. doi: 10.1111/cdev.12593
Larsen, J. M. (2017). The immune response to Prevotella bacteria in chronic inflammatory disease. Immunology 151, 363–374. doi: 10.1111/imm.12760
Lenhart, A., Dong, T., Joshi, S., Jaffe, N., Choo, C., Liu, C., et al. (2022). Effect of exclusion diets on symptom severity and the gut microbiota in patients with irritable bowel syndrome. Clin. Gastroenterol. Hepatol. Off. Clin. Pract. J. Am. Gastroenterol. Assoc. 20, e465–e483. doi: 10.1016/j.cgh.2021.05.027
Lyte, J. M., Gheorghe, C. E., Goodson, M. S., Kelley-Loughnane, N., Dinan, T. G., Cryan, J. F., et al. (2020). Gut-brain axis serotonergic responses to acute stress exposure are microbiome-dependent. Neurogastroenterol. Motil. 32:e13881. doi: 10.1111/nmo.13881
MacIntyre, M. M., Zare, M., and Williams, M. T. (2023). Anxiety-related disorders in the context of racism. Curr. Psychiatry Rep. 25, 31–43. doi: 10.1007/s11920-022-01408-2
Mallick, H., Rahnavard, A., McIver, L. J., Ma, S., Zhang, Y., Nguyen, L. H., et al. (2021). Multivariable association discovery in population-scale meta-omics studies. PLoS Comput. Biol. 17:e1009442. doi: 10.1371/journal.pcbi.1009442
Marcondes Ávila, P. R., Fiorot, M., Michels, M., Dominguini, D., Abatti, M., Vieira, A., et al. (2020). Effects of microbiota transplantation and the role of the vagus nerve in gut–brain axis in animals subjected to chronic mild stress. J. Affect. Disord. 277, 410–416. doi: 10.1016/j.jad.2020.08.013
Melchior, M., Moffitt, T. E., Milne, B. J., Poulton, R., and Caspi, A. (2007). Why do children from socioeconomically disadvantaged families suffer from poor health when they reach adulthood? A life-course study. Am. J. Epidemiol. 166, 966–974. doi: 10.1093/aje/kwm155
MetaHIT Consortium (2014). An integrated catalog of reference genes in the human gut microbiome. Nat. Biotechnol. 32, 834–841. doi: 10.1038/nbt.2942
Michaels, E., Thomas, M., Reeves, A., Price, M., Hasson, R., Chae, D., et al. (2019). Coding the everyday discrimination scale: implications for exposure assessment and associations with hypertension and depression among a cross section of mid-life African American women. J. Epidemiol. Community Health 73, 577–584. doi: 10.1136/jech-2018-211230
Moreira, C. G., Russell, R., Mishra, A. A., Narayanan, S., Ritchie, J. M., Waldor, M. K., et al. (2016). Bacterial adrenergic sensors regulate virulence of enteric pathogens in the gut. MBio 7, e00826–e00816. doi: 10.1128/mBio.00826-16
Moussaoui, N., Jacobs, J. P., Larauche, M., Biraud, M., Million, M., Mayer, E., et al. (2017). Chronic early-life stress in rat pups alters basal corticosterone, intestinal permeability, and fecal microbiota at weaning: influence of sex. J. Neurogastroenterol. Motil. 23, 135–143. doi: 10.5056/jnm16105
Nastasi, C., Mannarino, L., and D’Incalci, M. (2020). DNA Damage Response and Immune Defense. Int. J. Mol. Sci. 21:7504. doi: 10.3390/ijms21207504
Nater, U. M., and Ali, N. (2020). Alpha-Amylase. Encycl. Behav. Med. 87–89.doi: 10.1007/978-3-030-39903-0
Panza, G. A., Puhl, R. M., Taylor, B. A., Zaleski, A. L., Livingston, J., and Pescatello, L. S. (2019). Links between discrimination and cardiovascular health among socially stigmatized groups: a systematic review. PLoS One 14:e0217623. doi: 10.1371/journal.pone.0217623
Ritter, S., Vetter, M. L., and Sarwer, D. B. (2012). Lifestyle modifications and surgical options in the treatment of patients with obesity and type 2 diabetes mellitus. Postgrad. Med. 124, 168–180. doi: 10.3810/pgm.2012.07.2578
Sarubbo, F., Cavallucci, V., and Pani, G. (2022). The influence of gut microbiota on neurogenesis: evidence and hopes. Cells 11:382. doi: 10.3390/cells11030382
Sawyer, P. J., Major, B., Casad, B. J., Townsend, S. S. M., and Mendes, W. B. (2012). Discrimination and the stress response: psychological and physiological consequences of anticipating prejudice in interethnic interactions. Am. J. Public Health 102, 1020–1026. doi: 10.2105/AJPH.2011.300620
Schmitt, M. T., and Branscombe, N. R. (2002). The meaning and consequences of perceived discrimination in disadvantaged and privileged social groups. Eur. Rev. Soc. Psychol. 12, 167–199. doi: 10.1080/14792772143000058
Slopen, N., and Williams, D. R. (2014). Discrimination, other psychosocial stressors, and self-reported sleep duration and difficulties. Sleep 37, 147–156. doi: 10.5665/sleep.3326
Stephens, M. A. C., and Wand, G. (2012). Stress and the HPA axis: role of glucocorticoids in alcohol dependence. Alcohol Res. Curr. Rev. 34, 468–483.
Tetel, M. J., De Vries, G. J., Melcangi, R. C., Panzica, G., and O’Mahony, S. M. (2018). Steroids, stress and the gut microbiome-brain axis. J. Neuroendocrinol. 30:e12548. doi: 10.1111/jne.12548
Trieschmann, K., Chang, L., Park, S., Naliboff, B., Joshi, S., Labus, J. S., et al. (2022). The visceral sensitivity index: a novel tool for measuring GI-symptom-specific anxiety in inflammatory bowel disease. Neurogastroenterol. Motil. 34:e14384. doi: 10.1111/nmo.14384
Van Dyken, P., and Lacoste, B. (2018). Impact of metabolic syndrome on Neuroinflammation and the blood–brain barrier. Front. Neurosci. 12:930. doi: 10.3389/fnins.2018.00930
Vetter, M. L., Faulconbridge, L. F., Webb, V. L., and Wadden, T. A. (2010). Behavioral and pharmacologic therapies for obesity. Nat. Rev. Endocrinol. 6, 578–588. doi: 10.1038/nrendo.2010.121
Wu, H.-J., and Wu, E. (2012). The role of gut microbiota in immune homeostasis and autoimmunity. Gut Microbes 3, 4–14. doi: 10.4161/gmic.19320
Ypofanti, M., Zisi, V., Zourbanos, N., Mouchtouri, B., Tzanne, P., Theodorakis, Y., et al. (2015). Psychometric properties of the international personality item Pool big-five personality questionnaire for the Greek population. Health Psychol. Res. 3:2206. doi: 10.4081/hpr.2015.2206
Keywords: discrimination, psychosocial stressor, gut microbiome, Prevotella , transcriptome
Citation: Dong TS, Shera S, Peters K, Gee GC, Beltrán-Sánchez H, Wang MC, Kilpatrick LA, Zhang X, Labus JS, Vaughan A and Church A (2024) Experiences of discrimination are associated with microbiome and transcriptome alterations in the gut. Front. Microbiol. 15:1457028. doi: 10.3389/fmicb.2024.1457028
Edited by:
Zheng Ruan, Nanchang University, ChinaReviewed by:
Luca Carnevali, University of Parma, ItalyFlorentin Constancias, ETH Zürich, Switzerland
Copyright © 2024 Dong, Shera, Peters, Gee, Beltrán-Sánchez, Wang, Kilpatrick, Zhang, Labus, Vaughan and Church. This is an open-access article distributed under the terms of the Creative Commons Attribution License (CC BY). The use, distribution or reproduction in other forums is permitted, provided the original author(s) and the copyright owner(s) are credited and that the original publication in this journal is cited, in accordance with accepted academic practice. No use, distribution or reproduction is permitted which does not comply with these terms.
*Correspondence: Tien S. Dong, VFNEb25nQG1lZG5ldC51Y2xhLmVkdQ==; Arpana Church, QXJwYW5hQ2h1cmNoQG1lZG5ldC51Y2xhLmVkdQ==