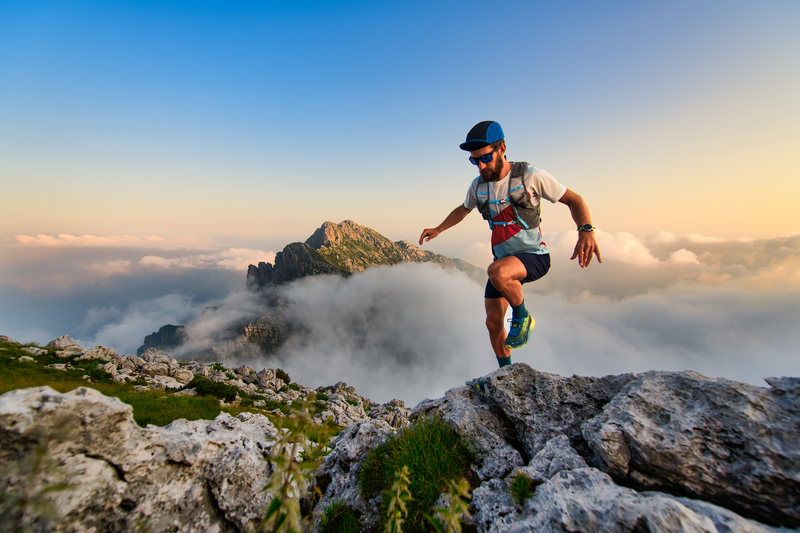
95% of researchers rate our articles as excellent or good
Learn more about the work of our research integrity team to safeguard the quality of each article we publish.
Find out more
ORIGINAL RESEARCH article
Front. Microbiol. , 29 October 2024
Sec. Terrestrial Microbiology
Volume 15 - 2024 | https://doi.org/10.3389/fmicb.2024.1442026
This article is part of the Research Topic Forest Microbiome: Dynamics and Interactions in the Anthropocene Era View all 19 articles
Understanding how different tree species affect soil microbial communities is crucial for sustainable forestry and ecosystem management practices. Despite Black walnut (Juglans nigra L.) forestry having a rich history, the overall comprehension of how this hardwood species influences soil remains incomplete. In earlier studies, we examined the effects of hardwood plantations on soil chemical properties and their interaction with microbial biomass, however, we highlight the importance of studying the soil microbial communities and their relationship with soil properties in greater depth. Building on this foundation, our research focused on evaluating microbiome compositions beneath J. nigra and another hardwood, Northern red oak (Quercus rubra L.) after a decade of establishment. We uncovered intriguing patterns within the soil bacterial/archaeal and fungal structures by conducting meticulous analyses utilizing amplicon sequencing alongside soil chemical properties. Our findings underscore that tree species play a pivotal role in shaping soil microbial structures, a role that surpasses even seasonal and depth influences. Most notably, J. nigra stands out for its ability to enhance microbial diversity, as evidenced by increased alpha-diversity indices compared to baseline values. Conversely, Q. rubra tends to decrease these indices. Significant disparities in microbial composition between the two tree species were evident, with J. nigra exhibiting enrichment in certain taxa such as Nitrospira, Geobacter, and Bacillus while Q. rubra showed enrichment in others like Acidobacteriota and ectomycorrhizal fungi. Furthermore, we also observed differences in co-occurrence networks by delving deeper into the interconnections within the soil microbiota. In both fungal and bacterial/archaeal communities, J. nigra and Q. rubra notably decreased the number of connections within their networks, while Q. rubra increased some, suggesting a more interconnected network. These differences were further highlighted by network metrics with Q. rubra displaying a higher mean degree and clustering coefficient. Additionally, our analysis revealed that tree species influence soil chemical properties, either directly or indirectly, thereby affecting soil bacterial and fungal communities. In conclusion, our study elucidates the intricate interplay between tree species and soil microbiota, emphasizing the need to consider these relationships in forestry and ecosystem management practices.
The soil on the planet Earth teems with life and harbors a remarkable diversity of microorganisms which constitute over 15% of Earth’s biomass (Wall et al., 2019). These microorganisms, collectively known as microbiota, are involved in vital ecological processes such as biogeochemical cycles, carbon (C) mineralization, and plant nutrient acquisition (Fierer, 2017). These complex relationships are intricately intertwined with the diverse biological components of soil communities (Tecon and Or, 2017). Various environmental factors like pH, substrate quality, temperature, and water availability sculpt the intricacies of microbial interactions. Moreover, the dynamic nature of microbial community composition spans from short-term seasonal fluctuations to long-term changes over years or decadal scales, adding another layer of complexity to the soil ecosystem (Morriën et al., 2017). The depth of soil further influences microbial communities, revealing gradients in soil resource availability and pH that correlate with variations in the microbiome (Tang et al., 2018).
Bacteria, archaea, and fungi form taxonomically and functionally diverse communities within the soil and rhizosphere that facilitate plant nutrient uptake through direct access to limiting nutrients (Ling et al., 2022). These communities are interconnected through intricate networks, which comprise interactions within and across species and domains (Wassermann et al., 2019). Furthermore, microorganisms also influence ecosystem dynamics by mediating the exchange of compounds between plant vegetation and soils. The interactions between trees and microorganisms within forest ecosystems are vital to shaping soil microbial communities as these relationships influence ecosystem dynamics and nutrient cycling (Ferlian et al., 2021).
Forests, especially in the northern hemisphere, emerge as critical sinks with tree-microorganism interactions (Onufrak et al., 2020): trees harbor specific microorganisms that perform essential functions such as nutrient supply and stress protection, thereby shaping plant health and tree species distribution (Bakker et al., 2020; Pantigoso et al., 2022; Sánchez-Cañizares et al., 2017). The forest environment significantly influences soil microbiomes through aboveground (e.g., leaf litter) and belowground (e.g., rhizodeposition) contributions (Reich et al., 2005; Calvaruso et al., 2010). Symbiotic relationships between plants and microorganisms, including those between bacteria and fungi, play a pivotal role in nutrient and water access (Ramirez et al., 2019; Averill et al., 2019). These differences underscore the necessity of understanding microbial composition at varying depths and over time to appreciate the complex interactions within forest soils fully. Forest soils are predominantly inhabited by fungal communities, with associations varying based on tree mycorrhizal type (Lauber et al., 2009; Averill et al., 2019). Each tree species exhibits distinct rhizodeposition and leaf litter patterns that selectively enhance or suppress soil microbial members (Baldrian, 2017).
Black walnut (Juglans nigra L.) and Northern red oak (Quercus rubra L.) are notable timber species that contribute distinct characteristics to soil conditions (Williams, 1990; Dyderski et al., 2020). Although both tree species influence soil microbial communities but differ in their effects on soil functions and microbial associations (Washington, 2003; Mortier et al., 2020; Gardner et al., 2023). For instance, J. nigra enhances microbial biomass and soil functions, whereas Q. rubra may have opposing effects (Sun et al., 2013; Gardner et al., 2023). Their associations with different tree mycorrhiza types (AM for J. nigra and EM for Q. rubra) also contribute to distinct soil microbial dynamics (Washington, 2003; Mortier et al., 2020; Gardner et al., 2023).
In our study, we sought to elucidate the specific effects of J. nigra and Q. rubra on soil structure. Specifically, our interests involve investigating the composition of bacterial, archaeal, and fungal communities within these plantations a decade post-establishment. We hypothesized that (i) tree species strongly shaped microbial communities in the soils of each forest plantation as a consequence of a change of soil pH; (ii) soil depth will enhance more Proteobacteria and Bacteroidota due to litter addition in the upper layer while Acidobacteroidota is more abundant in deeper layers as a consequence of less nutrient availability, and (iii) seasonal changes on soil temperature and humidity will affect more bacterial communities than fungal communities. To test our hypothesis, we investigated the structure of soil bacterial/archaeal (16S rRNA) and fungal (ITS) communities, using Illumina Miseq sequencing, at two different time points: autumn (October) and spring (March), and two soil depths. Additionally, we analyzed microbial structural and belowground C and N transfer, shaped by trees and through microbial processes. To unravel the intricate relationships within soil microbial communities, we employed co-occurrence networks and evaluations of soil chemical parameters to assess their responses to environmental factors such as seasonality and soil depth variations. Ultimately, our findings contribute to the broader understanding of the complex interplay between tree species and soil microbiomes.
Our investigation was conducted within a J. nigra and Q. rubra forest plantation located near Calcedonia, in midwestern Michigan (42.8412°N, 85.5828°W). The plantation, established in 2008, maintained a 2 m spacing between each tree. Site selection was carefully planned to cover the growth areas of both tree species, ensuring a thorough examination of soil microbial communities. We selected plots for analysis that encompassed each planting. Four composited samples were obtained from adjacent blocks, each confined to a 20 × 20 m area. Because of the near canopy and surface litter, there was no extra vegetation in the Q. rubra’s planted plot. On the other hand, the J. nigra’s planted plot had scant vegetation made up of local grasses. Additionally, a site devoid of plants (DB) adjacent to these blocks served as a reference for detecting changes and was centrally situated between the two sites. The soil in the area is predominantly Blount loam, with a slope averaging 1–3% but ranging from 0 to 6 percent. The texture of the soils was found to be silty clay loam or clay loam till. The average annual temperature at the study site is approximately 9.5°C, with an annual precipitation of 932 mm.
Soil samples were collected in October 2019 and March 2020 using a 2.5 cm diameter soil corer. Cores were taken 1 m away from the trunk in each plot. Surface litter was removed to ensure consistent sample collection as plot surfaces were covered in fallen leaves during the autumn sampling, a condition absent during the spring. In each case, plots were spaced at least 50 m apart, with a total of 4 plots per tree species, each adhering to the standard 20 × 20 m dimensions. Our composite soil sample was created from 25 randomly selected soil cores across each plot. Samples were collected from two soil depths, 0–10 and 10–20 cm, before being promptly transported to the laboratory on freezer packs to preserve microbial integrity. Upon arrival, all soil samples were sieved through a 2-mm mesh, with small roots discarded. After this, samples were split into two, one part was stored at −20°C for DNA extraction, and the other half was air dried for soil properties characterization.
We conducted a series of laboratory analyses, to thoroughly evaluate the soil properties across all samples which included assessments of gravimetric soil moisture, total carbon (TC), total organic carbon (TOC), total nitrogen (TN), and pH (H₂O). Gravimetric soil moisture was first determined by extracting 10 g subsamples, which were subsequently dried in an oven at 105°C for 24 h. These dried samples were then utilized for soil dry weight correction. TOC, TC, and TN were quantified using automated dry combustion, performed with a LECO Tru-Spec CN analyzer at the Instrumentation and Environmental Laboratory Services (IELS) at North Carolina State University (NCSU), located in North Carolina, USA. To assess soil pH, we employed a compound electrode from (Accumet, MA, USA) following the method described by Smith and Mullins (1991) that utilized a soil-water ratio of 1:2.5.
Soil DNA extraction was conducted in triplicate using 0.3 g of soil (total fresh weight) with the FastDNA™ SPIN Kit for Soil (MP Biomedicals, Solon, OH, USA), following the manufacturer’s protocol to accurately analyze microbial communities. Subsequently, we targeted the fungal ITS1 hypervariable region for amplification via PCR, utilizing the primers ITS1F (5′-CTTGGTCATTTAGAGGA AGTAA-3′) and ITS2R (5′-GCTGCGTTCTTCATCGATGC-3′) for fungal community analysis (Yan et al., 2021). Bacterial and archaeal communities were characterized with barcoded fusion primers 341F (CCTACGGGNGGCWGCAG) and 805R (GACTACHVGGGTAT CTAATCC) to amplify and sequence the V3–V4 region of the 16S rDNA gene (Herlemann et al., 2011). The PCR protocol began with a 3 min denaturation step at 95°C, followed by 27 cycles of 30 s denaturation at 95°C, a 30 s annealing at 55°C, and a 45 s elongation at 72°C before concluding with a 10 min extension at 72°C. Reactions were performed in triplicate. Each 20 μL reaction volume contained 2 μL of 2.5 mM dNTPs, 0.8 μL each of the forward and reverse primers, 4 μL of 5× FastPfu Buffer, (5 μM), 0.4 μL of FastPfu Polymerase (TransGen Biotech), and 10 ng of template DNA.
Following agarose gel electrophoresis, amplicons were extracted and purified using the AxyPrep DNA Gel Extraction Kit (Axygen Biosciences, Union City, CA, USA). The purified amplified PCR products were then quantified with a QuantiFluor™-ST Fluorometer (Promega, USA). Next-generation sequencing was conducted at the NC State University Genomic Sciences Laboratory (Raleigh, NC, USA) utilizing Illumina MiSeq technology, employing a paired-end sequencing approach with 2 × 300 bp read lengths.
Raw reads were processed using Mothur v.1.48.1 software (Schloss, 2020) for comprehensive bioinformatics and computational analysis. Trimming operations adhered to specific criteria, requiring a minimum length of 425 and 350 bp for bacterial and fungal amplicons, a minimum quality score of 25, allowance for one primer mismatch, and no barcode mismatches. Additionally, homopolymers greater than 10 were restricted. Reads with indistinct bases and singletons were eliminated, and chimeras were detected and eliminated using Uchime (Version 4.2) within the Mothur platform (Edgar, 2013). Subsequently, alignment and classification of 16S rDNA and ITS sequences were performed against the SILVA bacterial SSU reference database v138.2 (Yilmaz et al., 2014) and UNITE v10 database (Abarenkov et al., 2023), respectively. The resultant cleaned and refined sequences were grouped into distinct operational taxonomic units (OTUs) through the Average-Neighbor clustering algorithm – UPGMA implementation in Mothur, with a 97% minimum sequence similarity criterion. The average sequence count per sample was 160,813 for 16S rDNA and 135,690 for ITS sequences. Rarefaction was conducted using the Mothur program to standardize sample sizes to the smallest number of sequences (66,066 for 16S rDNA and 68,492 for ITS sequences) using the ‘sub.sample’ command (Supplementary Figure 1). Diversity Shannon index between samples was fair and accounted for differences in sequencing depth.
Fungal operational taxonomic units (OTUs) were then assigned to fungal functional guilds using the FungalTraits database (Pölme et al., 2020). OTUs assigned for more than one functional guild were analyzed by dividing the sequence counts of each OTU by the number of functional guilds assigned to that OTU. This approach allowed for visualization of differences in predicted fungal function between tree species. Stacked bar plots depicting the relative abundance of each fungal functional guild were created for each tree species, according to season, and depth.
Data interpretation and graphical representation were obtained using the R software environment (version: 4.1.2, R Core Team, 2017), and all the figures were designed using ggplot2 package (v3.5.0) in R (Wickham, 2016). Differences in soil nutrient content and microbial community diversity (Shannon alpha-diversity index; α-diversity) were assessed through analysis of variance (ANOVA) with Tukey’s post hoc test (stats, v4.3.0, and agricolae, v1.3–7, packages). Nonmetric dimensional scaling (NMDS) ordinations based on Bray–Curtis dissimilarity matrices aided in visualizing beta-diversity (β-diversity) patterns (ordinate function, phyloseq package, v1.46.0). Treatment effects on β-diversity were assessed utilizing permutation-based analysis of variance (PERMANOVA), with subsequent multiple comparisons and Bonferroni adjustment to determine differences in community composition (adonis function, vegan package, v2.4–6). The Shannon diversity index was calculated using normalized OTU data (phyloseq package). Indicator species analyses were conducted to identify bacterial/archaeal and fungal OTUs indicative of tree species, soil depth, and seasonal variations (De Cáceres, 2013). Validation and identification of taxa responsible for observed variations were performed through Generalized Linear Model (GLM) fitting using the negative binomial distribution via the DESeq function of the DESeq2 package (v1.42.0) in R (Love et al., 2014). Visual representations of taxa abundance differences between treatments were generated using the phyloseq package (McMurdie and Holmes, 2013). The validity of the differential abundance analysis was confirmed by employing Analysis of Compositions of Microbiomes with Bias Correction (ANCOM-BC, v2.4.0), ensuring a robust assessment of microbial absolute abundances (Lin and Peddada, 2020). Microbial co-occurrence patterns across tree species and the reference sample (DB) were investigated using the SpiecEasi package (v1.0.0) in R (Kurtz et al., 2015), and the sparCC function (SpiecEasi package) was used to calculate the correlation between each genus. The genus with a frequency lower than 75% and sparCC correlation values less or equal to 0.6 were removed from the network to reduce artificial correlation. Primary keystone microbes were identified based on their betweenness centrality and degree values within the bacterial and fungal networks (Vick-Majors et al., 2014; Tipton et al., 2018). Keystone taxa in the bacterial network had degrees surpassing 100 and betweenness centrality scores exceeding 800, while in the fungal network, keystone taxa had degrees above 3 and betweenness centrality scores above 150 (Kourtellis et al., 2013). Network complexity was assessed based on average connectivity, clustering coefficient, and edge number using the vegan and igraph packages (v1.6.0) in R (Shi et al., 2016; Banerjee et al., 2019). Mantel test and Spearman correlation were used to correlate the bacterial/archaeal and fungal communities with chemical, physical, and biochemical parameters.
All sequencing data (raw) for bacterial and fungal reads have been archived in the Sequence Read Archive (SRA) of the National Center for Biotechnology Information (NCBI) under BioProject number PRJNA1035935. Additionally, the count tables, taxonomic tables, and metadata tables generated during this study are accessible at https://zenodo.org/record/10070239. Furthermore, the R scripts utilized for data analysis and processing are openly available at https://github.com/JuanFrene/Black-Walnut. These resources are provided to facilitate transparency, reproducibility, and further investigation by the scientific community.
A summary of the environmental properties assessed in this research is found in Table 1. Significant variations were observed in TOC (p = 0.0018), TC (p < 0.0001), C to N ratio (C/N) (p = 0.0482), and TN (p = 0.0002), primarily influenced by tree species. In particular, J. nigra exhibited higher levels of these parameters compared to Q. rubra (Table 1). Seasonal analysis further revealed that TOC (p = 0.0424), TC (p = 0.0424), and soil humidity (p = 0.0088) were significantly higher during autumn and in the upper soil layer (0–10 cm). TN levels, on the other hand, were consistently greater in the upper layer regardless of season (p = 0.0424) (Table 1). Furthermore, dramatic differences in pH levels were observed, with Q. rubra exhibiting a lower pH than J. nigra (p < 0.0001) and the control site DB (Table 1).
We conducted NMDS ordination based on Bray-Curtis distances and subsequently performed PERMANOVA analysis to highlight seasonal and species-specific variation in microbial community structure to elucidate the factors influencing microbial community composition. Tree species emerged as the strongest predictor of bacterial/archaeal community composition (p = 0.001), followed by depth (p = 0.002) and season (p = 0.015, Figure 1a). Specifically, tree species accounted for 17.8% of bacterial/archaeal community variation, while depth and season contributed 4.2 and 6.2%, respectively (Figure 1a). Notably, both J. nigra and Q. rubra shared approximately 20% of unique bacterial/archaeal OTUs, with 26 and 20% unique OTUs, respectively. In contrast, DB harbored 15% unique bacterial/archaeal OTUs (Supplementary Figure 2a). Genus-level relative abundance data illustrated that Acidobacteriota and Proteobacteria were the most abundant taxa (Figure 2a). Specifically, Subgroup_2_ge, Candidatus_Solibacter (both Acidobacteriota) and Candidatus_Udaeobacter (Verrucomicrobiota) exhibited greater relative abundance in Q. rubra, whereas Flavobacterium (Bacteroidota), Vicinamibacteraceae_ge, and Comamonadaceae_unclassified (Proteobacteria) were more prevalent in DB and J. nigra (Figure 2a). The most pronounced differences were observed between Q. rubra and the other treatments. J. nigra and DB samples were primarily distinguished by season while depth exerted a greater influence on Q. rubra samples. Moreover, bacterial/archaeal α-diversity, as indicated by the Shannon index, was significantly higher under J. nigra for both seasons and depths compared to Q. rubra and DB (Figures 3a,b). Spring values of α -diversity generally exceeded those in autumn though no significant differences were detected across depth or season and no significant interactions among these factors were noted (p > 0.05) (Figures 3a,b).
Figure 1. Non-metric multidimensional analysis (NMDS) plot illustrating the influence of tree species on (a) bacterial/archaeal and (b) fungal community composition. Each character corresponds to a sample collected in each season, while each color represents a category (J. nigra, Q. rubra, or DB).
Figure 2. Heatmap showing the abundances of the 50 most abundant (a) bacterial/archaeal genera and (b) fungal genera in the soils for all three categories by depth, and season. Data were centered and scaled to the mean of each taxon’s log-transformed relative abundance. Samples are clustered along the top x-axis dendrogram based on community composition, while taxa are clustered on the side y-axis dendrogram according to their relative abundance distributed across samples. DB, non-plant soil; BW, J. nigra; RO, Q. rubra.
Figure 3. Relative abundances of (a) bacterial/archaeal and (b) fungal taxa, estimated from the proportional abundances of classifiable sequences, excluding unclassified sequences below the domain level. DB, non-plant soil; BW, J. nigra; RO, Q. rubra.
Tree species also significantly influenced fungal communities (p = 0.001), followed by soil depth (p = 0.001) (Figure 1b). A weak but significant interaction was observed relating to tree species and season (p = 0.049). Tree species accounted for 12.6% of fungal community variation, while depth and season explained 3% (Figure 1a). Moreover, J. nigra and Q. rubra shared 7% of unique fungal OTUs, with J. nigra and Q. rubra accounting for 34.75 and 22.25%, respectively. In contrast, DB contained 21.32% unique fungal OTUs (Supplementary Figure 2b). Genus-level relative abundance data exhibited variability across tree species, season, and soil depth (Figure 2b). Q. rubra exhibited a higher abundance of Cortinarius, Tuber, Russula, Lactarius, and Ramularia while J. nigra exhibited a higher abundance of Linnemannia, Podila, Trichoderma, and Fusarium (Figure 2b). Fungal α-diversity, as measured by the Shannon index, showed significant differences related to tree species (p = 0.0004), depth, and season (Figures 3c,d). Specifically, J. nigra exhibited the highest α-diversity, followed by Q. rubra and DB (Figure 3c). No significant differences were observed across depth or season, nor were there significant interactions among these factors (p > 0.05).
The analysis of fungal communities allowed for an exploration of functional guilds’ distribution and an assessment of their response to different environmental factors. Among fungal sequences, 1,046 of 7,893 OTUs were ascribed to a particular fungal functional guild. Ectomycorrhizas (EcM) were the most commonly identified guild, followed by saprotroph communities (Supplementary Figure 3). No significant differences in fungal guild relative abundances were observed between treatments, depth, or season (p > 0.05). However, significant differences in the richness of fungal guilds between tree species were noted. Saprotroph richness was notably higher in J. nigra (p = 0.0315), while Q. rubra exhibited greater EcM richness (p < 0.001). Additionally, saprotroph diversity showed significant seasonal variation (p = 0.0178), with greater diversity observed during autumn (Supplementary Table 1).
In the J. nigra, Q. rubra, and DB samples, Proteobacteria emerged as the dominant phylum among the recovered bacteria/archaea sequences, comprising 23.2% of the total, followed by Acidobacteriota (21.2%), Bacteroidota (13.4%), Verrucomicrobiota (9.9%), Planctomycetota (4.5%), and Actinobacteriota (3.8%) (Figure 4a). These top 6 phyla collectively accounted for over 90% of the recovered sequences. A total of 54 phyla were identified in the soil samples. At the class level, Gammaproteobacteria and Alphaproteobacteria, both belonging to the Proteobacteria phylum, represented 15.29 and 7.97% of the recovered 16S sequences, respectively. Proteobacteria exhibited significant variations associated with soil depths, while Acidobacteriota, Bacteroidota, and Myxococcota displayed variations linked to species and soil depth (Figure 4a). Other phyla such as Actinobacteriota and Planctomycetota were significantly influenced across depth and season. Notably, Armatimonadota were significantly influenced by tree species, while Chloroflexi showed a significant correlation with soil depth (Figure 4a).
Figure 4. Influence of tree species, season, and depth on α-diversity for (a,b) bacteria/archaeal communities and (c,d) fungal communities at 0–10 cm (a,c) and 10-20 cm (b,d) soil depths. Values with different letters are significantly different among seasons (p-value < 0.05; ANOVA, followed by Tukey post-hoc test). DB, non-plant soil; BW, J. nigra; RO, Q. rubra.
Among the recovered fungal sequences from J. nigra, Q. rubra, and DB, Basidiomycota emerged as the predominant phylum, accounting for 59.3% of the total, followed by Ascomycota (15.3%), and Mortierellomycota (16.2%) (Figure 4b). Together, these phyla represented over 90% of the recovered sequences. Basidiomycota, Aphelidiomycota, and Chytridiomycota were significantly influenced by tree species, whereas Kickxellomycota was predominantly influenced by seasonal variations (Figure 4b). Mortierellomycota exhibited variations primarily associated with tree species that were evident only in autumn (Figure 4b).
A total of 1,680 indicator operational taxonomic units (OTUs) were identified across the three designations: J. nigra (498 OTUs), Q. rubra (629 OTUs), and DB (256 OTUs) for bacterial/archaeal communities. Notably, J. nigra exhibited a higher percentage of Bacteroidota and Planctomycetota, while Q. rubra displayed elevated levels of Acidobacteriota (primarily Acidibacter, Bryobacter, and Subgroup_2_ge), Armatimonadota, and Planctomycetota compared to DB (Supplementary Table 2). Within Proteobacteria, J. nigra increased α- and β-Proteobacteria, whereas Q. rubra specifically elevated α-Proteobacteria compared to DB. Conversely, the presence of J. nigra led to a reduction in Actinobacteriota and Chloroflexi percentages, while Q. rubra limited the presence of Firmicutes (Supplementary Table 2). DESeq analysis corroborated these findings, highlighting significant taxonomic distinctions observed in J. nigra and Q. rubra (Figure 5a). This analysis revealed a total of 110 different OTUs belonging to 12 different phyla. The most abundant taxa within Q. rubra included Subgroup_2_ge, Granulicella, Bryobacter, Occallatibacter, Candidatus_Solibacter, and the Pedosphaeraceae_unclassified. Conversely, prevalent responders in J. nigra encompassed Sphingobium, Gemmatimonas, Tolumonas, Bacillales_Unclassified, and Perlucidibaca (Figure 5a). Comparing Q. rubra to DB unveiled pronounced differences primarily in Acidobacteriota and β-Proteobacteria taxa (Supplementary Figure 4a). A comparison between J. nigra and DB indicated distinct taxa, including GOUTA6 and Gematimonas in J. nigra and Granulicella and Subgroup_2_ge (Supplementary Figure 4b). Additionally, DESeq analysis was validated using ANCOM (Supplementary Figure 5a).
Figure 5. Differential representation of significant abundance OTUs, based on DESeq, between J. nigra and Q. rubra at the genus level for (a) bacterial/archaeal and (b) fungal taxa. Dots represent taxa, with colors indicating phylum and labels representing genus. Multiple points per genus correspond to different species of the genus. Positive values indicate a positive response to Q. rubra. Negative values indicate a positive response to J. nigra. Only OTUs with differential abundance at p < 0.05 are shown.
A total of 275 fungal community indicator OTUs were detected across our three categories: J. nigra (133 OTUs), Q. rubra (65 OTUs), and DB (65 OTUs) (Supplementary Table 3). The presence of J. nigra correlated with the Hymenogastraceae and Ceratobasidiaceae families, and the Coniochaeta genus, whereas Q. rubra showed elevated Basidiomycota, predominantly comprising ectomycorrhizal (EcM) fungi. Conversely, J. nigra led to a decrease in the percentage of Ascomycota, while Q. rubra exhibited reductions in Glomeromycota and Chytridiomycota. DESeq analysis demonstrated significant differences in fungal taxa within the J. nigra-Q. rubra comparison (Figure 5b), which was further validated using ANCOM (Supplementary Figure 5b). Specifically, Q. rubra enriched EcM fungal taxa such as Tuber, Inocybe, Tomentella, Amanita, and Russula, while J. nigra favored Hymenogaster, Lobulomyces, Thanatephorus, Otospora, and Entrophospora. These variations extended to comparisons with DB (Supplementary Figures 3c,d).
The soil bacterial/archaeal community network exhibited distinct co-occurrence patterns for each tree species and the DB category (Figure 6; Table 2). BD presented a higher number of nodes and edges than both tree species. Additionally, BD presented values for density, clustering coefficient, and avg. number of neighbors (Table 2). Q. rubra presented the highest mean degree and average path length values (Table 2). Among the identified keystones for bacterial networks under different plant species, 42 taxa belonging to five phyla were identified, including Sphingomonas, Arenimonas, Bryobacter, Bradyrhizobium, Ellin6067, and Subgroup_2_ge (Supplementary Table 4).
Figure 6. Co-occurrence patterns in bacterial/archaeal (a–c) and fungal (d–f) microbiomes in (a,d) J. nigra, (b,d) Q. rubra, and (c,e) DB. Connections represent strong (Spearman’s ρ > 0.6) and significant (FDR-corrected p < 0.01) correlations. Node size corresponds to the OTUs’ degree and edge thickness corresponds to Spearman’s correlation coefficient value. Node size of individual nodes corresponds to the OTUs’ degree, and the thickness of connections between noted (edges) corresponds to Spearman’s correlation coefficient value. DB, non-plant soil; BW, J. nigra; RO, Q. rubra.
Table 2. Topological features of the co-occurrence networks constructed based on bacteria and fungi.
Similarly, both tree species experienced reduced nodes and edges in the soil fungal community network, resulting in a smaller mean degree and clustering coefficient (Figure 6d; Table 2). Notably, the Q. rubra network exhibited the highest clustering coefficient and modularity (Figure 6d; Table 2). The Nectriaceae and Ceratobasidiaceae families were the only ones identified as a keystone taxon in the DB network. Additionally, Saitozyma presented high values of mean degree and betweenness centrality in both Q. rubra and DB specifically. Finally, Tuber was presented in both tree plant species (Supplementary Table 4). Furthermore, other keystone taxa identified in Q. rubra were Inocybe, Talaromyces, Laccaria, and Podila while in J. nigra appeared Solicoccozyma, Staphylotrichum, Cladosporium, Phlegmacium, and Cortinarius (Supplementary Table 4).
Results from a Mantel test revealed the significant influence of soil variables on microbial community structures. Specifically, soil pH (r = 0.3, p = 0.001) significantly affected bacterial/archaeal community structure. Additionally, pH (r = 0.3, p = 0.001), TN (r = 0.18, p = 0.003), and TC (r = 0.077, p = 0.044) collectively shaped fungal community structure. Humidity was significantly correlated with Ascomycota (r = 0.38, p = 0.006) and Kickxellomycota (r = −0.36, p = 0.011) among fungal phyla. In contrast, Glomeromycota and Kickxellomycota exhibited negative correlations with pH, TOC, TC, and TN (Supplementary Table 5). The C/N ratio also demonstrated a significant correlation with Murocomycota (r = −0.33, p = 0.021).
Chemical properties represented by TOC, TC, and TN positively correlated with Proteobacteria, Bacteroidota, and TM7 to a significant degree. These same chemical properties were negatively correlated with Acidobacteriota, Firmicutes (only TOC), Planctomycetota, and Chloroflexi (Supplementary Table 5). A significant correlation was observed between pH and several phyla, including Proteobacteria, Acidobacteriota, Actinobacteriota, Firmicutes, and Armatimonadota. The C/N ratio impacted Bacteroidota, Actinobacteriota, and Chloroflexi while soil humidity showed correlations with Bacteroidota and Chloroflexi (Supplementary Table 5).
Tree species composition can drastically reshape soil microbial communities and consequently induce suppression or acceleration of soil functions (Chen et al., 2019; Ramirez et al., 2019). Therefore, understanding how different tree species influence soil microbial communities is imperative for elucidating these dynamics. In our work, we revealed significant distinctions in soil microbial communities associated with J. nigra, Q. rubra, and the DB. Specifically, building upon previous research by Gardner et al. (2023), our results underscore how the introduction of trees, particularly J. nigra and Q. rubra, significantly modified and enriched microbial diversity within the DB soil structure. Notably, an impressive 47 and 56% of bacterial/archaeal and fungal OTUs appeared only in the plots with trees. These results support our principal hypothesis that tree species were the strongest influencers of microbial communities. Furthermore, the distribution of bacterial/archaeal and fungal OTUs revealed that J. nigra harbored a higher proportion of these taxa compared to other species. Tedersoo et al. (2013) showed that J. nigra in agroforestry systems enhanced soil bacterial/archaeal diversity, particularly with increasing tree biomass and agroforestry age in a previous study. Also, litter from J. nigra can improve vital soil fertility indicators, such as C and N content, promoting abundance and diversity principally within bacterial/archaeal communities (Hong-ye et al., 2016). In contrast, fungal communities associated with both J. nigra and Q. rubra displayed a higher species diversity compared with soil without trees. The presence of J. nigra led to the highest increase in diversity within bacterial/archaeal communities.
Our findings revealed distinct responses of bacterial and fungal communities to different tree species, consistent with prior studies (Lu-Irving et al., 2019; Onufrak et al., 2020). This observation highlights that tree species exert varying degrees of influence on bacterial and fungal communities, shedding light on the complex interactions between plants and soil microbes. However, these results contrast previous studies, where soil depth was the main driver of bacterial community (Prada-Salcedo et al., 2022). In our study, depth and season had a significant but limited impact on bacterial and fungal communities. The influence of soil depth on microbial communities is a result of the nutrient gradient effect. Specifically, soil depth influenced Acidobacteriota, with higher levels detected in the deeper soil profiles (Eilers et al., 2012) where nutrient availability is often less abundant (DeAngelis et al., 2009). The seasonal effects mainly affect two important variables for soil microbial communities: humidity and temperature. Our samplings were carried out in autumn and spring when the climate is not extreme like in summer or winter, thus the effect on the microbial communities might be less influential or need more days to show significant changes detectable by technique (Gardner et al., 2023).
Bacterial community analysis showed Proteobacteria and Acidobacteriota, common in forest soil, were the most abundant phyla recovered in this study, a finding that aligns with observations by other researchers (Uroz et al., 2016). Additionally, this analysis detected a notable abundance of Bacteroidota, Verrucomicrobiota, and Actinobacteriota. J. nigra significantly increased the abundance of Bacteroidota and Proteobacteria in comparison to Q. rubra. In contrast, Onufrak et al. (2020) showed that the Spirosomaceae family (Bacteroidota) appeared as an indicator of J. nigra. Both phyla are well-known for their copiotrophic life strategy, different from oligotrophs in that they thrive in highly organic, nutrient-rich soils (Fierer et al., 2007; Prada-Salcedo et al., 2022). Conversely, Q. rubra increased the representation of Acidobacteriota members, a phylum characterized by their survival ability in low C availability or oligotrophic environments (Fierer et al., 2007). The shift is supported by the positive correlation between C and N stocks with Proteobacteria and the negative correlation with Acidobacteriota, supporting the theory of life strategy attributes. The decline in pH in Q. rubra soils allows for Acidobacteriota populations to rise.
Bacterial taxa in forests and plantations have been documented extensively (Uroz et al., 2016; Landesman et al., 2014). The substantially higher proportion of Subgroup_2_ge, Granulicella, Bryobacter, Occallatibacter, and Candidatus_Solibacter can be explained by the positive relationship between Acidobacteriota and the decreased soil pH found in Q. rubra (Fierer et al., 2007). Within the Q. rubra plots, we also observed a significant abundance of genera such as Burkholderia, Sphingomonas, and Rhizomicrobium, which can be associated with the robust growth of these trees (Landesman et al., 2014; Morrison et al., 2014). These genera play crucial roles in N cycling as nitrogen-fixing bacteria (Anderson and Habiger, 2012) and in phosphorous solubilization (Halder et al., 1990). Additionally, Kuramae et al. (2019) indicated a significant positive correlation between Acidobacteriota and nitrogen-fixing bacteria (Rhizobiales and Burkholderiales) and decomposers (Elsterales and Xanthomonadales). The enhancement of two Bacteroidota genera, Flavobacterium and Chryseobacterium, known for their occurrence in root associations and deadwood, was linked to J. nigra. This is consistent with the association of Bacteroidota with carbon-rich soils like those found around J. nigra (Fierer et al., 2012) and their diverse physiological capabilities, including ligninolytic activity (Dilly et al., 2001; Kolton et al., 2013).
Soil-associated fungal communities vary with tree species (Baldrian, 2017). In this study, the fungal community primarily featured phyla such as Basidiomycota, Ascomycota, and Mortierellomycota. Our findings align with prior studies indicating that Ascomycota and Basidiomycota dominate forest and plantation soils (Urbanova et al., 2015). Specifically, J. nigra increased the abundance of Glomeromycota, while Q. rubra favored Basidiomycota and Ascomycota. Several studies suggest that the uptick in Basidiomycota is linked to their capacity for decomposing more recalcitrant litter (Nguyen et al., 2016). In contrast, Glomeromycota represents AM fungi which can be associated with J. nigra (Štursová et al., 2016).
The identity of plant species remains a principal factor shaping AM fungal communities (Mummey and Rillig, 2006; Baldrian, 2017). Our findings suggest host preference in symbiotic EcM fungal species, with Q. rubra hosting the following genera: Inocybe, Amanita, Tomentella, Lactarius, and Russula, whereas J. nigra was more commonly associated with Hebeloma, Trichoderma, Coniochaeta, Coralloidiomyces, and Enterocarpus, as reported in previous research (Zhang and Wang, 2017). Previous research has emphasized the impact of N supplies and soil pH on EcM community diversification (Suz et al., 2014). Additionally, some studies have shown the crucial role of EcM fungi in accessing organic nutrients, particularly under acidic conditions (Averill et al., 2019), which is congruent with our results here. Moreover, the increased abundance of EcM fungi in Q. rubra is consistent with the greater EcM richness observed in these plots compared to J. nigra and DB. Furthermore, when comparing J. nigra with DB, J. nigra exhibited a greater abundance of various EcM fungi, including Hebeloma, Laccaria, Hymenogasters, and Protoglossum. In contrast to saprophytic fungal communities, tree host specialization is a significant force influencing EcM fungal populations (Ferlian et al., 2018; Chen et al., 2019).
Microorganisms thrive in complex associations rather than in isolation (Banerjee et al., 2019). To explore this, we created distinct co-occurrence networks for bacterial/archaeal and fungal communities and analyzed their features to understand how plant species affect microbial co-occurrence patterns. Our findings revealed that the DB zone presented the highest number of connections (edges) between the different nodes for bacterial/archaeal and fungal co-occurrence networks. Additionally, DB networks presented the highest values for clustering coefficient and density parameters. On the contrary, the Q. rubra presented higher values for the network average path length and mean degree parameters. This observation suggests that greater microbial diversity does not necessarily lead to more complex co-occurrence networks. This phenomenon can be explained by the ecological theory of the species-energy relationship (Evans et al., 2005; Gaston, 2000; Wright, 1983). J. nigra’s richer C and N environment provides a diverse range of energy sources for bacterial/archaeal communities, enhancing their diversity. Consequently, bacterial/archaeal species are more dependent on environmental energy and nutrients than on complex interactions with other bacterial species (Tu et al., 2020).
Keystone taxa are highly linked microorganisms that play essential roles in the structure and function of the microbiota and operate as environmental indicators (Banerjee et al., 2019). In our analysis, we identified keystone taxa belonging to Proteobacteria associated with tree species’ plantations, Arenimonas, Sphingomonas, KF-JG30-C25_ge, and Oxalobacteraceae_unclassified for J. nigra. These findings highlight the specific bacterial taxa that may be crucial in maintaining microbial community stability under varying soil conditions. This response pattern is consistent with the soil pH decrease produced by these trees. Additional keystone taxa associated with trees were Actinobacteriota which have a significant impact on the structure and function of forest ecosystems (Lupatini et al., 2014) due to their role in the degradation of cellulitic materials and the solubilization of P (Mitra et al., 2022; Zhou et al., 2020). Furthermore, Sphingomonas is capable of degrading very complex carbon substrates including both substituted and unsubstituted mono- and poly-aromatic hydrocarbons up to five rings (Jones et al., 2011), giving this genus a distinctive advantage in decomposing recalcitrant carbon sources such as lignin. When we analyzed fungal keystone taxa, Tuber, belonging to the Pezizales order, was a common keystone taxon in both plant species and DB. This fungus is integral to the forest food web, forming EcM associations that are most widely distributed in forests of the northern hemisphere (Obase et al., 2011; Bahram et al., 2013). Conversely, Mortierella emerged as a keystone taxon associated with J. nigra, a genus known for its saprotrophic capabilities (Yadav et al., 2015). These findings emphasize the divergent ecological roles that fungal communities may play depending on the host tree species.
Our findings show that tree species significantly influence C and N stocks and pH levels (Gardner et al., 2023). Variations in these soil properties can be attributed to differences in tree physiology and their subsequent impact on soil chemistry. Existing research strongly supports the notion that forest trees contribute to soil acidification over decadal intervals (Binkley, 1995; Mitchell et al., 2010). In addition, our study unveiled significant correlations between soil properties and microbial community structures, echoing findings from previous research (Lauber et al., 2009; Banerjee et al., 2019). These correlations underscore the intricate interplay between environmental factors and microbial community dynamics in soil ecosystems. Specifically, the correlation between total C and N with the fungal community illustrates the essential role of fungi in the C and N cycle. Fungi are the primary agents involved in the decomposition of soil organic matter in temperate forests (Baldrian, 2008). However, it is important to note that bacterial and fungal communities are not governed by the same environmental parameters (Uroz et al., 2016). Notably, fungal communities are strongly influenced by tree species, while bacterial communities are primarily influenced by root exudates (Urbanova et al., 2015).
One of our work’s limitations is that J. nigra and Q. rubra associate with different types of mycorrhizas, which introduces variability in fungal community analysis. The fungal primers used here are not suitable for sequencing AM fungi. As a result, our findings may include a greater number of mycorrhizal fungi that are more closely related to Q. rubra than J. nigra. Future studies should incorporate primers that effectively capture the entire spectrum of soil mycorrhizal communities associated with these tree species to achieve a more comprehensive analysis. Although observational study designs limit causal inference, they are beneficial for exploring an understudied system and generating suggestions to guide future microbiome research on hardwood trees. Another potential limitation of our work is the use of OTUs instead of amplicon sequence variants (ASVs). Previous studies suggest that the diversity index based on ASV may outperform those based on OTUs (Joos et al., 2020). Although rarefaction and clustering level-identity OTUs appear to bridge the gap between OTU- and ASV-based techniques (Chiarello et al., 2022), we rarified the samples in this study to be consistent. Future research should consider the adoption of ASV techniques to improve the accuracy of microbial diversity assessments.
In summary, this study provides compelling evidence of the strong impact of tree species on soil properties and soil bacterial/archaeal and fungal communities. Our findings demonstrate that tree species differ significantly in shaping belowground communities. Specifically, tree species, characterized by distinct litter quality and mycorrhiza fungal associations, impact not only the taxonomy of bacterial/archaeal and fungal communities but also their diversity and interactions through interspecific connections. Moreover, tree species directly or indirectly alter soil properties (Sommer et al., 2017). These changes in soil properties can either enhance or diminish a forest’s resilience to climate-related stressors such as drought, diseases, and pests (Buma and Wessman, 2013; Williams and Ginzel, 2020). Consequently, those tree species able to adapt to the modified soil conditions may thrive, while others may struggle, thereby causing significant impacts on the overall resilience and diversity of the forest ecosystem. Looking ahead, research is necessary to associate the soil microbial communities with plant health and development. Such studies are crucial to determine which microbial species can enhance early tree plantation growth thereby ensuring greater plant growth and yields.
The datasets presented in this study can be found in online repositories. The names of the repository/repositories and accession number(s) can be found in the article/Supplementary material.
JF: Conceptualization, Data curation, Investigation, Methodology, Writing – original draft, Writing – review & editing. SL: Conceptualization, Funding acquisition, Investigation, Methodology, Project administration, Resources, Visualization, Writing – original draft, Writing – review & editing. NL: Data curation, Writing – review & editing. RC: Resources, Validation, Writing – review & editing. TG: Conceptualization, Data curation, Funding acquisition, Investigation, Methodology, Project administration, Resources, Supervision, Visualization, Writing – original draft, Writing – review & editing.
The author(s) declare that financial support was received for the research, authorship, and/or publication of this article. Financial support provided by Hardwood Tree Improvement & Regeneration Center (HTIRC) grant F.90023500.06.002 and US Forest Service grant 20-CR-11242314108.
We extend our sincere gratitude to the USDA Forest Service Northern Research Station for their invaluable assistance during fieldwork. Our deepest appreciation goes to the laboratory staff for their expert advice and technical support. We are especially grateful to Zachary Senwo at Alabama A&M University and Veronica Acosta-Martinez of the USDA Agricultural Research Service for their insightful guidance on the experimental design. Finally, we acknowledge the financial support provided by Hardwood Tree Improvement & Regeneration Center (HTIRC).
The authors declare that the research was conducted in the absence of any commercial or financial relationships that could be construed as a potential conflict of interest.
All claims expressed in this article are solely those of the authors and do not necessarily represent those of their affiliated organizations, or those of the publisher, the editors and the reviewers. Any product that may be evaluated in this article, or claim that may be made by its manufacturer, is not guaranteed or endorsed by the publisher.
The Supplementary material for this article can be found online at: https://www.frontiersin.org/articles/10.3389/fmicb.2024.1442026/full#supplementary-material
SUPPLEMENTARY FIGURE 1 | Rarefaction curves of observed species plotted against sampling depth for (a) bacteria/archaea and (b) fungi.
SUPPLEMENTARY FIGURE 2 | Venn diagrams illustrating the shared OTUs of the (a) fungal and (b) bacterial/archaeal microbiomes associated with J. nigra, Q. rubra, and DB. The diagrams show the absolute number of OTUs shared between microbiomes for both tree species and DB across seasons and depths. DB, non-plant soil; BW, J. nigra; RO, Q. rubra.
SUPPLEMENTARY FIGURE 3 | Relative abundance of fungal functional guilds in soils associated with both tree species and the DB environment. DB, non-plant soil; BW, J. nigra; RO, Northern red oak.
SUPPLEMENTARY FIGURE 4 | Differential representation of significant abundance OTUs between J. nigra and Q. rubra at the genus level for (a,b) bacterial/archaeal and (c,d) fungal taxa. Figures (a) and (c) compare J. nigra vs. DB, while (b) and (d) compare Q. rubra vs. DB. Dots represent taxa, colors indicate phylum, and labels represent genus. Multiple points per genus represent distinct species of a given genus. Positive values indicate a positive response to J. nigra or Q. rubra, while negative values indicate a positive response to DB. Only OTUs with differential abundance at p < 0.05 are represented. DB, non-plant soil; BW, J. nigra; RO, Northern red oak.
SUPPLEMENTARY FIGURE 5 | Differential representation of significant abundance OTUs, based on ANCOM, between J. nigra and Q. rubra at the genus level for (a) bacterial/archaeal and (b) fungal taxa. Positive values indicate a positive response to Q. rubra. Negative values indicate a positive response to J. nigra. Only the probabilities with a differential abundance at p < 0.05 are represented.
Abarenkov, K., Nilsson, R. H., Larsson, K.-H., Taylor, A. F. S., May, T. W., Frøslev, T. G., et al. (2023). The UNITE database for molecular identification and taxonomic communication of fungi and other eukaryotes: sequences, taxa and classifications reconsidered. Nucleic Acids Res. 52, D791–D797. doi: 10.1093/nar/gkad1039
Anderson, M., and Habiger, J. (2012). Characterization and identification of productivity-associated Rhizobacteria in wheat. Appl. Environ. Microbiol. 78, 4434–4446. doi: 10.1128/AEM.07466-11
Averill, C., Bhatnagar, J. M., Dietze, M. C., Pearse, W. D., and Kivlin, S. N. (2019). Global imprint of mycorrhizal fungi on whole-plant nutrient economics. Proc. Natl. Acad. Sci. 116, 23163–23168. doi: 10.1073/pnas.1906655116
Bahram, M., Kõljalg, U., Kohout, P., Mirshahvaladi, S., and Tedersoo, L. (2013). Ectomycorrhizal fungi of exotic pine plantations in relation to native; host trees in Iran: evidence of host range expansion by local symbionts; to distantly related host taxa. Mycorrhiza 23, 11–19. doi: 10.1007/s00572-012-0445-z
Bakker, P. A. H. M., Berendsen, R. L., Van Pelt, J. A., Vismans, G., Yu, K., Li, E., et al. (2020). The soil-borne identity and microbiome-assisted agriculture: looking back to the future. Mol. Plant 13, 1394–1401. doi: 10.1016/j.molp.2020.09.017
Baldrian, P. (2017). Forest microbiome: diversity, complexity and dynamics. FEMS Microbiol. Rev. 41, 109–130. doi: 10.1093/femsre/fuw040
Baldrian, P. (2008). “Enzymes of saprotrophic basidiomycetes” in Ecology of saprotrophic basidiomycetes. eds. L. Boddy, J. C. Frankland, and P. van West (New York: Academic Press), 19–41.
Banerjee, S., Walder, F., Büchi, L., Meyer, M., Held, A. Y., Gattinger, A., et al. (2019). Agricultural intensification reduces microbial network complexity and the abundance of keystone taxa in roots. ISME J. 13, 1722–1736. doi: 10.1038/s41396-019-0383-2
Binkley, D. (1995). The influence of tree species on Forest soils: processes and patterns. Canterbury, New Zealand: Special Publication - Agronomy Society of New Zealand, 1–34.
Buma, B., and Wessman, C. A. (2013). Forest resilience, climate change, and opportunities for adaptation: a specific case of a general problem. Forest Ecol. Manag. 306, 216–225. doi: 10.1016/j.foreco.2013.06.044
Calvaruso, C., Turpault, M. P., Leclerc, E., Ranger, J., Garbaye, J., Uroz, S., et al. (2010). Influence of forest trees on the distribution of mineral weathering-associated bacterial communities of the scleroderma citrinum mycorrhizosphere. Appl. Environ. Microbiol. 76, 4780–4787. doi: 10.1128/AEM.03040-09
Chen, L., Xiang, W., Wu, H., Ouyang, S., Zhou, B., Zeng, Y., et al. (2019). Tree species identity surpasses richness in affecting soil microbial richness and community composition in subtropical forests. Soil Biol. Biochem. 130, 113–121. doi: 10.1016/j.soilbio.2018.12.008
Chiarello, M., McCauley, M., Villéger, S., and Jackson, C. R. (2022). Ranking the biases: the choice of OTUs vs. ASVs in 16S rRNA amplicon data analysis has stronger effects on diversity measures than rarefaction and OTU identity threshold. PLoS One 17:e0264443. doi: 10.1371/journal.pone.0264443
DeAngelis, K. M., Brodie, E. L., DeSantis, T. Z., Andersen, G. L., Lindow, S. E., and Firestone, M. K. (2009). Selective progressive response of soil microbial community to wild oat roots. ISME J. 3, 168–178. doi: 10.1038/ismej.2008.103
Dilly, O., Bartsch, S., Rosenbrock, P., Buscot, F., and Munch, J. C. (2001). Shifts in physiological capabilities of the microbiota during the decomposition of leaf litter in a black alder (Alnus glutinosa (Gaertn.) L.) forest. Soil Biol. Biochem. 33, 921–930. doi: 10.1016/S0038-0717(00)00239-X
Dyderski, M. K., Chmura, D., Dylewski, Ł., Horodecki, P., Jagodzinski, A. M., Pietras, M., et al. (2020). Biological flora of the British Isles, Quercus rubra. J. Ecol. 108, 1199–1225. doi: 10.1111/1365-2745.13375
Edgar, R. C. (2013). UPARSE: highly accurate OTU sequences from microbial amplicon reads. Nat. Methods 10, 996–998. doi: 10.1038/nmeth.2604
Eilers, K. G., Debenport, S., Anderson, S., and Fierer, N. (2012). Digging deeper to find unique microbial communities: the strong effect of depth on the structure of bacterial and archaeal communities in soil. Soil Biol. Biochem. 50, 58–65. doi: 10.1016/j.soilbio.2012.03.011
Evans, K. L., Greenwood, J. J., and Gaston, K. J. (2005). Relative contribution of abundant and rare species to species–energy relationships. Biol. Lett. 1, 87–90. doi: 10.1098/rsbl.2004.0251
Ferlian, O., Biere, A., Bonfante, P., Buscot, F., Eisenhauer, N., Fernandez, I., et al. (2018). Growing research networks on mycorrhizae for mutual benefits. Trends Plant Sci. 23, 975–984. doi: 10.1016/j.tplants.2018.08.008
Ferlian, O., Goldmann, K., Eisenhauer, N., Tarkka, M. T., Buscot, F., and Heintz-Buschart, A. (2021). Distinct effects of host and neighbour tree identity on arbuscular and ectomycorrhizal fungi along a tree diversity gradient. ISME Commun. 1:40. doi: 10.1038/s43705-021-00042-y
Fierer, N. (2017). Embracing the unknown: disentangling the complexities of the soil microbiome. Nat. Rev. Microbiol. 15, 579–590. doi: 10.1038/nrmicro.2017.87
Fierer, N., Bradford, M. A., and Jackson, R. B. (2007). Toward an ecological classification of soil bacteria. Ecology 88, 1354–1364. doi: 10.1890/05-1839
Fierer, N., Leff, J. W., Adams, B. J., Nielsen, U. N., Bates, S. T., Lauber, C. L., et al. (2012). Cross-biome metagenomic analyses of soil microbial communities and their functional attributes. PNAS 109, 21390–21395. doi: 10.1073/pnas.1215210110
Gardner, T. G., Frene, J. P., Lawson, S. S., Lue Sue, N. D., Handy, J., and Crawford, R. H. (2023). The impact of tree species on microbial community structure and soil function on forest plantations in the central hardwoods region (CHR). Forests 14:859. doi: 10.3390/f14050859
Halder, A. K., Mishra, A. K., Bhattacharyya, P., and Chakrabartty, P. K. (1990). Solubilization of rock phosphate by rhizobium and bradyrhizobium. J. Gen. Appl. Microbiol. 36, 81–92. doi: 10.2323/jgam.36.81
Herlemann, D. P. R., Labrenz, M., Jürgens, K., Bertilsson, S., Waniek, J. J., and Andersson, A. F. (2011, 2011). Transitions in bacterial communities along the 2000 km salinity gradient of the Baltic Sea. ISME J. 5, 1571–1579. doi: 10.1038/ismej.2011.41
Hong-ye, M., Xue-Jun, P., Wen-e, Z., and Mao-qiao, L. (2016). Soil chemical and biological property associated with walnut (Juglans sigillata Dode) leaf decomposition. J. Northeast. Agric. Univ. 23, 26–39. doi: 10.1016/S1006-8104(17)30004-1
Jones, M. D., Crandell, D. W., Singleton, D. R., and Aitken, M. D. (2011). Stable-isotope probing of the polycyclic aromatic hydrocarbon-degrading bacterial guild in a contaminated soil. Environ. Microbiol. 13, 2623–2632. doi: 10.1111/j.1462-2920.2011.02501.x
Joos, L., Beirinckx, S., Haegeman, A., Debode, J., Vandecasteele, B., Baeyen, S., et al. (2020). Daring to be differential: metabarcoding analysis of soil and plant-related microbial communities using amplicon sequence variants and operational taxonomical units. BMC genomics 21, 1–17. doi: 10.1186/s12864-020-07126-4
Kolton, M., Sela, N., Elad, Y., and Cytryn, E. (2013). Comparative genomic analysis indicates that niche adaptation of terrestrial Flavobacteria is strongly linked to plant glycan metabolism. PLoS One 8:e76704. doi: 10.1371/journal.pone.0076704
Kourtellis, N., Alahakoon, T., Simha, R., Iamnitchi, A., and Tripathi, R. (2013). Identifying high betweenness centrality nodes in large social networks. Soc. Netw. Anal. Min. 3, 899–914. doi: 10.1007/s13278-012-0076-6
Kuramae, E. E., Leite, M. F. A., Suleiman, A. K. A., Gough, C. M., Castillo, B. T., Faller, L., et al. (2019). Wood decay characteristics and interspecific interactions control bacterial community succession in Populus grandidentata (Bigtooth Aspen). Front. Microbiol. 10:979. doi: 10.3389/fmicb.2019.00979
Kurtz, Z. D., Muller, C. L., Miraldi, E. R., Littman, D. R., Blaser, M. J., and Bonneau, R. A. (2015). Sparse and compositionally robust inference of microbial ecological networks. PLoS Comput. Biol. 11:e1004226. doi: 10.1371/journal.pcbi.1004226
Landesman, W. J., Nelson, D. M., and Fitzpatrick, M. C. (2014). Soil properties and tree species drive the ß-diversity of soil bacterial communities. Soil Biol. Biochem. 76, 201–209. doi: 10.1016/j.soilbio.2014.05.025
Lauber, C. L., Hamady, M., Knight, R., and Fierer, N. (2009). Pyrosequencing-based assessment of soil pH as a predictor of soil bacterial community structure at the continental scale. Appl. Environ. Microbiol. 75, 5111–5120. doi: 10.1128/AEM.00335-09
Lin, H., and Peddada, S. D. (2020). Analysis of compositions of microbiomes with bias correction. Nat. Commun. 11, 1–11. doi: 10.1038/s41467-020-17041-7
Ling, N., Wang, T., and Kuzyakov, Y. (2022). Rhizosphere bacteriome structure and functions. Nat. Commun. 13:836. doi: 10.1038/s41467-022-28448-9
Love, M. I., Huber, W., and Anders, S. (2014). Moderated estimation of fold change and dispersion for RNA-seq data with DESeq2. Genome Biol. 15, 1–21. doi: 10.1186/s13059-014-0550-8
Lu-Irving, P., Harenčár, J. G., Sounart, H., Welles, S. R., Swope, S. M., Baltrus, D. A., et al. (2019). Native and invading yellow Starthistle (Centaurea solstitialis) microbiomes differ in composition and diversity of bacteria. Sphere 4, e00088–e00019. doi: 10.1128/mSphere.00088-19
Lupatini, M., Suleiman, A. K. A., Jacques, R. J. S., Antoniolli, Z. I., Ferrreira, A. S., Kuramae, E., et al. (2014). Network topology reveals high connectance levels and few key microbial genera within soils. Front. Environ. Sci. 2, 1–11. doi: 10.3389/fenvs.2014.00010
McMurdie, P. J., and Holmes, S. (2013). phyloseq: an R package for reproducible interactive analysis and graphics of microbiome census data. PloS one 8:e61217. doi: 10.1371/journal.pone.0061217
Mitchell, R. J., Campbell, C. D., Chapman, S. J., and Cameron, C. M. (2010). The ecological engineering impact of a single tree species on the soil microbial community. J. Ecol. 98, 50–61. doi: 10.1111/j.1365-2745.2009.01601.x
Mitra, D., Mondal, R., Khoshru, B., Senapati, A., Radha, T. K., Mahakur, B., et al. (2022). Actinobacteria-enhanced plant growth, nutrient acquisition, and crop protection: advances in soil, plant, and microbial multifactorial interactions. Pedosphere 32, 149–170. doi: 10.1016/S1002-0160(21)60042-5
Morriën, E., Hannula, S., Snoek, L., Helmsing, N. R., Zweers, H., de Hollander, M., et al. (2017). Soil networks become more connected and take up more carbon as nature restoration progresses. Nat. Commun. 8:14349. doi: 10.1038/ncomms14349
Morrison, D. J., Cruickshank, M. G., and Lalumière, A. (2014). Control of laminated and Armillaria root diseases by stump removal and tree species mixtures: amount and cause of mortality and impact on yield after 40 years. For. Ecol. Manag. 319, 75–98. doi: 10.1016/j.foreco.2014.02.007
Mortier, E., Lamotte, O., Martin-Laurent, F., and Recorbet, G. (2020). Forty years of study on interactions between walnut trees and arbuscular mycorrhizal fungi. A review. Agron. Sustain. Dev. 40:43. doi: 10.1007/s13593-020-00647-y
Mummey, D. L., and Rillig, M. C. (2006). The invasive plant species Centaurea maculosa alters arbuscular mycorrhizal fungal communities in the field. Plant Soil 288, 81–90. doi: 10.1007/s11104-006-9091-6
Nguyen, N. H., Williams, L. J., Vincent, J. B., Stefanski, A., Cavender-Bares, J., Messier, C., et al. (2016). Ectomycorrhizal fungal diversity and saprotrophic fungal diversity are linked to different tree community attributes in a field-based tree experiment. Mol. Ecol. 25, 4032–4046. doi: 10.1111/mec.13719
Obase, K., Lee, J. K., and Chun, K. W. (2011). Diversity and community structure of ectomycorrhizal fungi in Pinus hunbergia coastal forests in the eastern region of Korea. Mycoscience 52, 383–391. doi: 10.1007/S10267-011-0123-6
Onufrak, A. J., Williams, G. M., Klingeman, W. E. III, Cregger, M. A., Klingeman, D. M., DeBruyn, J. M., et al. (2020). Regional differences in the structure of Juglans nigra phytobiome reflect geographical differences in thousand cankers disease severity. Phytobiomes J. 4, 388–404. doi: 10.1094/PBIOMES-05-20-0044-R
Pantigoso, H. A., Newberger, D., and Vivanco, J. M. (2022). The rhizosphere microbiome: plant–microbial interactions for resource acquisition. J. Appl. Microbiol. 133, 2864–2876. doi: 10.1111/jam.15686
Prada-Salcedo, L. D., Prada-Salcedo, J. P., Heintz-Buschart, A., Buscot, F., and Goldmann, K. (2022). Effects of tree composition and soil depth on structure and functionality of belowground microbial communities in temperate European forests. Front. Microbiol. 13:920618. doi: 10.3389/fmicb.2022.920618
Pölme, S., Abarenkov, K., Henrik Nilsson, R., Lindahl, B. D., Clemmensen, K. E., Kauserud, H., et al. (2020). FungalTraits: a user-friendly traits database of fungi and fungus-like stramenopiles. Fungal Divers. 105, 1–16. doi: 10.1007/s13225-020-00466-2
Ramirez, K. S., Snoek, L. B., Koorem, K., Stefan Geisen, S., Janneke Bloem, L., ten Hooven, F., et al. (2019). Range-expansion effects on the belowground plant microbiome. Nat. Ecol. Evol. 3, 604–611. doi: 10.1038/s41559-019-0828-z
R Core Team (2017). R: A language and environment for statistical computing. Vienna, Austria: R Foundation for Statistical Computing. Available at: https://www.R-project.org/.
Reich, P. B., Oleksyn, J., Modrzynski, J., Mrozinski, P., Hobbie, S. E., Eissenstat, D. M., et al. (2005). Linking litter calcium, earthworms and soil properties: a common garden test with 14 tree species. Ecol. Lett. 8, 811–818. doi: 10.1111/j.1461-0248.2005.00779.x
Sánchez-Cañizares, C., Jorrín, B., Poole, P. S., and Tkacz, A. (2017). Understanding the holobiont: the interdependence of plants and their microbiome. Curr. Opin. Microbol. 38, 188–196. doi: 10.1016/j.mib.2017.07.001
Schloss, P. D. (2020). Reintroducing mothur: 10 years later. Appl. Environ. Microbiol. 86, e02343–e02319. doi: 10.1128/AEM.02343-19
Shi, S., Nuccio, E. E., Shi, Z. J., He, Z., Zhou, J., and Firestone, M. K. (2016). The interconnected rhizosphere: high network complexity dominates rhizosphere assemblages. Ecol. Lett. 19, 926–936. doi: 10.1111/ele.12630
Smith, K., and Mullins, C. E. (1991). Soil analysis: physical methods. New York: Marcel Dekker, Inc.
Sommer, J., Dippold, M. A., Zieger, S. L., Handke, A., Scheu, S., and Kuzyakov, Y. (2017). The tree species matters: belowground carbon input and utilization in the myco-rhizosphere. Eur. J. Soil Biol. 81, 100–107. doi: 10.1016/j.ejsobi.2017.07.001
Štursová, M., Bárta, J., Šantrůčková, H., and Baldrian, P. (2016). Small-scale spatial heterogeneity of ecosystem properties, microbial community composition and microbial activities in a temperate mountain forest soil. FEMS Microbiol. Ecol. 92:fiw185. doi: 10.1093/femsec/fiw185
Sun, K., Kang, M., Zhang, Z., Jin, J., Wang, Z., Pan, Z., et al. (2013). Impact of deashing treatment on biochar structural properties and potential sorption mechanisms of phenanthrene. Environ, Science & Techn. 47, 11473–11481. doi: 10.1016/j.ecoleng.2013.10.027
Suz, L. M., Barsoum, N., Benham, S., Dietrich, H.-P., Fetzer, K. D., Fischer, R., et al. (2014). Environmental drivers of ectomycorrhizal communities in Europe's temperate oak forests. Mol. Ecol. 23, 5628–5644. doi: 10.1111/mec.12947
Tang, Y., Yu, G., Zhang, X., Wang, Q., Ge, J., and Liu, S. (2018). Changes in nitrogen-cycling microbial communities with depth in temperate and subtropical forest soils. Appl. Soil Ecol. 124, 218–228. doi: 10.1016/j.apsoil.2017.10.029
Tecon, R., and Or, D. (2017). Biophysical processes supporting the diversity of microbial life in soil. FEMS Microbiol. Rev. 41, 599–623. doi: 10.1093/femsre/fux039
Tedersoo, L., Bahram, M., Dickie, I. A., and Dickie, I. A. (2013). Does host plant richness explain diversity of ectomycorrhizal fungi? Re-evaluation of Gao et al. (2013) data sets reveals sampling effects. Mol. Ecol. 23, 992–995. doi: 10.1111/mec.12660
Tipton, L., Müller, C. L., Kurtz, Z. D., Huang, L., Kleerup, E., Morris, A., et al. (2018). Fungi stabilize connectivity in the lung and skin microbial ecosystems. Microbiome 6:12. doi: 10.1186/s40168-017-0393-0
Tu, Q., Yan, Q., Deng, Y., Michaletz, S. T., Buzzard, V., Weiser, M. D., et al. (2020). Biogeographic patterns of microbial co-occurrence ecological networks in six American forests. Soil Biol. Biochem. 148:107897. doi: 10.1016/j.soilbio.2020.107897
Urbanova, M., Šnajdr, J., and Baldrian, P. (2015). Composition of fungal and bacterial communities in forest litter and soil is largely determined by dominant trees. Soil Biol. Biochem. 84, 53–64. doi: 10.1016/j.soilbio.2015.02.011
Uroz, S., Oger, P., Tisserand, E., Cebron, A., Turpault, M. P., Buee, M., et al. (2016). Specific impacts of beech and Norway spruce on the structure and diversity of the rhizosphere and soil microbial communities. Sci. Rep. 6:e27756. doi: 10.1038/srep27756
Vick-Majors, T. J., Priscu, J. C., and Amaral-Zettler, L. A. (2014). Modular community structure suggests metabolic plasticity during the transition to polar night in ice-covered Antarctic lakes. ISME J. 8, 778–789. doi: 10.1038/ismej.2013.190
Wall, L. G., Gabbarini, L. A., Ferrari, A. E., Frene, J. P., Covelli, J. M., Reyna, D. L., et al. (2019). Changes of paradigms in agriculture soil microbiology and new challenges in microbial ecology. Acta Oecol. 95, 68–73. doi: 10.1016/j.actao.2019.02.001
Washington, D. M. (2003). Fungi associated with northern red oak (Quercus rubra L.) acorns. (Graduate theses, dissertations, and problem reports), 1405.
Wassermann, B., Cernava, T., Müller, H., Berg, C., and Berg, G. (2019). Seeds of native alpine plants host unique microbial communities embedded in cross-kingdom networks. Microbiome 7:108. doi: 10.1186/s40168-019-0723-5
Williams, R. D. (1990). Juglans nigra L. black walnut. eds. R. M. Burns and B. H. Honkala (Silvics of North America: Hardwoods), 391–399.
Williams, G. M., and Ginzel, M. D. (2020). Spatial and climatic factors influence ambrosia beetle (Coleoptera: Curculionidae) abundance in intensively managed plantations of eastern black walnut. Environ. Entomol. 49, 49–58. doi: 10.1094/PBIOMES-02-21-0014-R
Wright, D. H. (1983). Species-energy theory: an extension of species-area theory. Oikos 41:496. doi: 10.2307/3544109
Yadav, D. R., Kim, S. W., Adhikari, M., Hyun Um, Y., Seung Kim, H., Kim, C., et al. (2015). Three new records of Mortierella species isolated from crop field soil in Korea. Mycobiology 43, 203–209. doi: 10.5941/MYCO.2015.43.3.203
Yan, K., Abbas, M., Meng, L., Cai, H., Peng, Z., Li, Q., et al. (2021). Analysis of the fungal diversity and community structure in Sichuan dark tea during pile-fermentation. Front. Microbiol. 12:706714. doi: 10.3389/fmicb.2021.706714
Yilmaz, P., Parfrey, L. W., Yarza, P., Gerken, J., Pruesse, E., Quast, C., et al. (2014). The SILVA and “all-species living tree project (LTP)” taxonomic frameworks. Opens external link in new windowNucl. Acids Res. 42, D643–D648. doi: 10.1093/nar/gkt1209
Zhang, L., and Wang, S. (2017). Bacterial community diversity on in-shell walnut surfaces from six representative provinces in China. Sci. Rep. 7:10054. doi: 10.1038/s41598-017-10138-y
Keywords: forestry, microbiome, plants, soil, soil ecology
Citation: Frene JP, Lawson SS, Lue Sue ND, Crawford RH and Gardner TG (2024) Effects of tree species identity on soil microbial communities in Juglans nigra and Quercus rubra plantations. Front. Microbiol. 15:1442026. doi: 10.3389/fmicb.2024.1442026
Received: 01 June 2024; Accepted: 09 October 2024;
Published: 29 October 2024.
Edited by:
Antonio Castellano-Hinojosa, Universidad de Granada, SpainReviewed by:
Dipti Rai, North Carolina Agricultural and Technical State University, United StatesCopyright © 2024 Frene, Lawson, Lue Sue, Crawford and Gardner. This is an open-access article distributed under the terms of the Creative Commons Attribution License (CC BY). The use, distribution or reproduction in other forums is permitted, provided the original author(s) and the copyright owner(s) are credited and that the original publication in this journal is cited, in accordance with accepted academic practice. No use, distribution or reproduction is permitted which does not comply with these terms.
*Correspondence: Terrence G. Gardner, dGVycmVuY2UuZ2FyZG5lckBtb3JlaG91c2UuZWR1
†Present addresses: Juan P. Frene, Agricultural Science Center, New Mexico State University, Clovis, NM, United States
Terrence G. Gardner, Department of Biology, Morehouse College, Atlanta, GA, United States
Disclaimer: All claims expressed in this article are solely those of the authors and do not necessarily represent those of their affiliated organizations, or those of the publisher, the editors and the reviewers. Any product that may be evaluated in this article or claim that may be made by its manufacturer is not guaranteed or endorsed by the publisher.
Research integrity at Frontiers
Learn more about the work of our research integrity team to safeguard the quality of each article we publish.