- 1Department of Rheumatology, The Second Affiliated Hospital of Zhejiang Chinese Medical University, Hangzhou, Zhejiang, China
- 2The Second Clinical Medical College, Zhejiang Chinese Medical University, Hangzhou, Zhejiang, China
- 3School of Basic Medicine, Zhejiang Chinese Medicine University, Hangzhou, Zhejiang, China
Background: Accumulating evidence suggests that an imbalance of gut microbiota is commonly observed in patients with rheumatoid arthritis (RA). However, it remains unclear whether gut microbiota dysbiosis is a cause or consequence of RA, and the mechanisms by which gut dysbiosis contributes to RA have not been fully understood. This study aimed to investigate the causal relationship between gut microbiota and metabolites with RA.
Methods: A two-sample Mendelian randomization analysis was performed to estimate the causality of gut microbiota and metabolites on RA. A genome-wide association study (GWAS) of 211 gut microbiota and 217 metabolites was used as the exposure, whereas RA was treated as the outcome. Inverse variance weighted (IVW) was regarded as the primary approach for calculating causal estimates. MR Egger method, Weighted median method, Simple mode method, and weighted mode method were used for sensitive analysis. Metabolic pathway analysis was performed via the web-based Metaconflict 5.0. Additionally, an animal study was undertaken to evaluate the results inferred by Mendelian randomization.
Result: This study indicated that six gut microbiota taxa (RuminococcaceaeUCG013, Erysipelotrichia, Erysipelotrichaceae, Erysipelotrichales, Clostridia, and Veillonellaceae) were estimated to exert a positive impact on RA. Conversely, seven gut microbiota taxa (Oxalobacter, Cyanobacteria, RuminococcaceaeUCG002, LachnospiraceaeUCG010, Christensenellaceae, Oxalobacteraceae, Anaerostipes) were estimated to exert a negative impact on RA. Three metabolites, namely indole-3-propionate (IPA), glycine and sphingomyelin (SM 16:1), were found to be linked to lower RA risk, while five metabolites (argininosuccinate, CE 20_4, TAG 58_8, PC 40_6, and LPC 20_4) were linked to higher RA risk. Additionally, four metabolic pathways were identified by metabolic pathway analysis. The collagen-induced arthritis (CIA) rats exhibited a higher relative abundance of Class_Clostridia and a lower abundance of Genus_Lachnospiraceae (p < 0.05) than the healthy controls.
Conclusion: This study identified causal associations between specific gut microbiota, metabolites, and RA. These findings support the significant role of gut microbiota and metabolites in RA pathogenesis.
1 Introduction
Rheumatoid arthritis (RA) is an autoimmune disease characterized by a symmetrical affliction of the peripheral joints, which leads to swelling, morning stiffness, and persistent joint pain, eventually resulting in disability and posing a global health threat. The global incidence of RA is still high, with an average rate of 1%. Females are more likely to develop RA, especially in their middle years. The pathogenesis of RA remains elusive but may ensue due to intricate interactions between multiple genetic, environmental (including the gut microbiota), and immunological factors (Deane et al., 2017). Therefore, RA remains a challenging condition to treat (Li et al., 2023).
The gastrointestinal (GI) tract contains many beneficial gut microorganisms that are important for regulating immune function and overall health. Imbalances in gut microbiota, known as dysbiosis, have been linked to autoimmune disorders like RA. Studies have shown that RA patients and animal models have dysbiotic gut microbiota compositions, characterized by an imbalance in beneficial and harmful bacteria. This highlights the significance of gut microbiota in the development and progression of RA. Patients with RA showed a higher presence of fecal Prevotella spp. during the early stages of the disease, with Prevotella copri being particularly prominent and associated with disease progression compared to the control group (Kishikawa et al., 2020). Another case-control study showed that RA patients had a lower diversity index of gut microbiota, a higher abundance of phylum Verrucomicrobiae and genus Akkermansia, and a lower abundance of Firmicutes. Moreover, the alterations in microbiota levels directly correlated with clinical and pathological characteristics like ACPA antibodies, levels of TNF-α and IL-17A, along with immune cell numbers (Chiang et al., 2019; Li Y. et al., 2021). Several animal studies have also demonstrated the critical role of gut microbiota in arthritis development. SKG mice did not develop arthritis under sterile conditions. However, arthritis was induced in SKG mice when they were treated with Prevotella copri. In addition, RA models treated with probiotics such as Lactobacillus rhamnosus GG showed measurable improvements, including reduced joint swelling (Raaschou et al., 2018).
The relationship between gut dysbiosis and RA remains unclear; it has not been determined whether gut dysbiosis acts as the primary cause or a secondary effect in RA. Moreover, the processes by which gut dysbiosis contributes to RA have yet to be fully understood. Recent studies have indicated that an imbalance in the gut microbiota might lead to RA through the production of functional metabolites, damage to the intestinal mucosal barrier, and molecular mimicry of autoantigens by gut microbes (Yu et al., 2021; He et al., 2022; Zhao et al., 2022). The production of microbial metabolites is recognized as an intermediary mechanism linking gut microbiota and immunity that regulates immune responses and chronic immune disorders such as RA (Yang and Cong, 2021). For example, short-chain fatty acids (SCFAs) synthesized by gut microbiota are noted to engage with germinal center B cells, affect the differentiation of plasmablastes, and play a role in the formation of RA (Rosser et al., 2020).
Moreover, the current knowledge of the microbiome and microbiota-derived metabolites in RA in humans mainly depends on observational studies, which might be susceptible to the effects of confounding factors on the outcomes. In contrast, the Mendelian randomization (MR) approach represents an instrumental variable method that utilizes single-nucleotide polymorphisms (SNPs) as instrumental variables (IVs) to establish causal associations between various traits. MR possesses the distinct advantage of minimizing bias that might arise from confounding variables and reverse causality (Davies et al., 2018). In this study, we employed the two-sample MR method to investigate the possible causal relationship between microbiota, metabolites, and RA using summary statistics from the largest genome-wide association studies (GWAS) thus far.
2 Materials and methods
2.1 GWAS summary statistics
2.1.1 Gut microbiota
Summary statistics for a gut microbiome were extracted from the MiBioGen Consortium (http://www.mibiogen.org). This GWAS study recruited 18,340 participants from various ethnicities (such as European, American Hispanic/Latin, East Asian, etc.) and ages from 24 cohorts with 211 taxa (i.e., 131 genera, 35 families, 20 orders, 16 classes, and nine phyla). The microbiome GWAS analysis between autosomal human genetic variants and gut microbiome was performed in three ways. Firstly, Spearman's correlation analysis was used to examine the association between SNP dosages and three alpha diversity metrics. Secondly, the loci influencing the covariate-adjusted abundance of bacterial taxa were identified. Thirdly, the loci associated with the probability of the presence vs. absence of the bacterial taxon were identified (Kurilshikov et al., 2021).
2.2 Gut metabolites
Summary statistics for gut metabolites were obtained from the Framingham Heart Study Offspring Cohort. This GWAS study recruited 2,076 participants and measured 217 metabolites (113 polar analytes and 104 lipid analytes) in plasma (Rhee et al., 2013).
2.3 Rheumatoid arthritis
GWAS summary data for RA were obtained from the IEU GWAS database (https://gwas.mrcieu.ac.uk/datasets/). This GWAS study recruited 19,234 RA patients and 61,565 controls from European and Asian ancestries (Okada et al., 2014).
2.4 Instrument variable selection
Firstly, quality control measures were applied to select suitable instrumental variables (IVs). For the gut microbiome data, we aimed to ensure data reliability and an adequate number of SNPs for exposure analysis. After reviewing MR research literature, we set the genome-wide threshold for exposure-associated SNPs at 1.0 × 10−5, as widely used in previous MR studies (Sanna et al., 2019; Hu et al., 2024). Regarding SNPs associated with plasma gut metabolites, we applied the standard GWAS thresholds (p < 5.0 × 10−8). Next, clumping was performed to retain only independent SNPs. Based on previous literature on gut microbiome data analysis (Cai et al., 2022a,b; Guo et al., 2023), the threshold for gut microbiome clumping was set to r2 < 0.1 and a 500 kb window. For gut metabolite, the threshold was set at r2 < 0.001 and a window size of 10,000 kb (Hu et al., 2024).
Further, if the outcome datasets lacked SNPs, we selected proxy SNPs following methods from previous studies (Xiang et al., 2021). Using the “extract_outcome_data” function from the TwoSampleMR R package, we identified proxy SNPs with high LD, selecting those with R2 > 0.8 (Hemani et al., 2018; Hou et al., 2023). To prevent weak instrument bias, the F-statistic was utilized to evaluate whether weak IVs were confounded with the selected IVs. IVs with F-statistics < 10 were excluded. The equation 2 × EAF × (1–EAF) × beta2)/(2 × EAF × (1–EAF) × beta2) + (2 × EAF × (1–EAF) × N × SE(beta)2 was employed to calculate the F statistics for each bacterial taxon, beta2/SE2 was applied for each SNP extracted from metabolites. EAF denotes effect allele frequency, beta signifies the genetic impact on physical activity, N is the GWAS sample size for the SNP-physical activity link, and SE(beta) is the standard error of the genetic effect (Papadimitriou et al., 2020).
2.5 Statistical analysis
2.5.1 MR analyses
We performed two-sample MR analysis methods to estimate the causal associations between two instrument exposures (gut microbiota and metabolites) and instrument outcome-RA. Five high-efficiency methods were applied, including the inverse variance weighted (IVW) method, MR Egger method, weighted median method, Simple mode method, and weighted mode method; IVW was regarded as the primary approach. The Wald ratio method was used for the MR analysis for bacterial taxa and metabolites containing only one SNP. Cochrane's Q-test and leave-one-out sensitivity analysis were performed to assess the heterogeneity among SNPs. In the presence of heterogeneity (p < 0.05), heterogeneity was estimated using the inconsistency index (I2) value (I2 values < 50% indicate low to moderate heterogeneity, whereas I2 ≥ 50% indicate moderate to high heterogeneity) and a random-effects IVW test was utilized. MR-Egger intercept, Mendelian Randomization Pleiotropy RESidual Sum, and MR-PRESSO global test were used to detect the presence of pleiotropy and to eliminate the effects of pleiotropy by removing outliers (Verbanck et al., 2018). The following three conditions were used to determine whether there was a causal effect of human gut microbiome composition or gut metabolite on RA risk: (1) A significant difference in the IVW method (p < 0.05), (2) Consistency in the estimation directions of the IVW, weighted median, and MR-Egger methods, and (3) Non-significance in both the MR-Egger intercept test and the MR-PRESSO global test (p > 0.05) (Yuan et al., 2022). All analyses were conducted in R Studio (version 4.2.0) using the TwoSampleMR and MRPRESSO packages.
2.5.2 Metabolic pathway analyses
Metabolic pathways were analyzed using the online platform MetaboAnalyst 5.0 (https://www.metaboanalyst.ca/). The Small Molecule Pathway Database (SMPDB) and the Kyoto Encyclopedia of Genes and Genomes (KEGG) database were used in this study, with the significance level set to default.
2.6 Animal experiments
2.6.1 Animal model construction
The animal experiments were reviewed and approved by the Ethics Committee of Zhejiang Chinese Medical University (Ref No. IACUU-20190218-01). Twelve specific pathogen-free (SPF) female Wistar rats (6–7 weeks old) were obtained from Shanghai SLAC Laboratory Animal Co., Ltd. After an acclimatization period of 7 days, 12 rats were randomly divided into two groups: (1) control group (CT): injected with 200 μL of 0.9% NaCl solution at the base of the tail on days 0 and 7; (2) collagen-induced arthritis (CIA) group: immunized using bovine Collagen Type II (CII): 200 μg (USA, Chondrex) emulsified with 200 μL incomplete Freund's adjuvant (USA, Chondrex) at the base of the tail on day 0, rats were boosted with 100 μg CII in 100 μL incomplete Freund's adjuvant on day 7 (Rosloniec et al., 2010). On day 21, the rats were sacrificed, and tissues along with fecal samples were collected.
2.6.2 Assessment of arthritis severity
The swelling in the hind paws was measured using a paw volume meter (YSC-7C, China). The ankle joints obtained from rats were immobilized in a solution containing 4% formalin. After fixation, the joints were dehydrated and subsequently embedded in paraffin. The tissue sections were stained with hematoxylin-eosin (H&E), and Safranin O-Fast Green (Saf-O/FG).
2.6.3 Fecal sample storage, DNA extraction, and 16S rRNA sequencing
Following the guidelines provided by the manufacturer, fecal DNA extraction was performed with a kit from Tiangen Biotech Co., Ltd., Beijing, China. We evaluated the DNA's concentration and purity using a NanoDrop 2000 spectrophotometer (Thermo Fisher Scientific, USA). The V3-V4 region of the 16S rRNA gene was amplified using broad-range bacterial primers as previously described (Yu et al., 2018). Following the manufacturer's instructions, we purified, quantified, combined, and sequenced the amplified samples using standard methods and the MiSeq Reagents Kit v3 (600 cycles, Illumina). The genetic sequencing was carried out by Hangzhou Legenomics Bio-Pharm Technology Co., Ltd., based in Zhejiang, China.
Sequences were analyzed using Quantitative Insights into Microbial Ecology (QIIME) (Caporaso et al., 2010). The measurement of Operational Taxonomic Units (OTUs), indicative of the variety of bacterial types, was performed with a threshold of 97% identity for each sample. The abundance of OTUs was established at the genus level (Edgar, 2010), and bacterial taxonomy was determined via the use of the SILVA (Quast et al., 2013) and the NCBI database (Sayers et al., 2019). The OTU table was imported into R software, and alpha and beta diversity metrics were calculated using the “vegan” package. Principal coordinate analysis (PCoA) was employed to examine beta diversity via weighted or unweighted UniFrac analysis utilizing R software.
2.6.4 Statistical analysis
Differences in the relative abundance of gut microorganisms between the CIA and CT groups were analyzed using Student's t-tests for data with normal distributions and non-parametric tests for data with non-normal distributions.
3 Results
3.1 Mendelian randomization
3.1.1 Associations between gut microbiota and RA
The Mendelian randomization analysis, illustrated in Figure 1, initially involved 2,281 significant (p < 1 × 10−5) SNPs or their proxies related to gut microbiota traits as genetic instrumental variables. These variables encompassed 211 gut microbiota traits across multiple taxonomic levels (9 phyla, 16 classes, 20 orders, 36 families, 119 genera). After removing the SNPs with LD effects and independence from RA, 2061 SNPs were selected. The F- statistics of each bacterial taxon were all >20, indicating that weak IV bias could be effectively avoided (Supplementary Table 1). The primary data for these SNPs, including their β values, SE, and p-values, were systematically compiled for subsequent analyses.
The results of IVW analyses demonstrated that RuminococcaceaeUCG013 [odds ratio (OR) = 1.32, 95% confidence interval (CI), 1.09–1.61, p = 0.005], Erysipelotrichia, Erysipelotrichaceae, Erysipelotrichales (OR = 1.26, 95% CI, 1.02–1.56, p = 0.035), Clostridia (OR = 1.23, 95% CI, 1.00–1.51, p = 0.049), and Veillonellaceae (OR = 1.17, 95% CI, 1.02–1.33, p = 0.021) exert a positive impact on RA. Oxalobacter (OR =0.85, 95% CI, 0.76–0.94, p = 0.002), Cyanobacteria (OR = 0.85, 95% CI, 0.72–0.99, p = 0.035), RuminococcaceaeUCG002 (OR = 0.84, 95% CI, 0.73–0.97, p = 0.015), LachnospiraceaeUCG010 (OR = 0.84, 95% CI, 0.71–0.99, p = 0.038), Christensenellaceae (OR = 0.83, 95% CI, 0.70–0.98, p = 0.027), Oxalobacteraceae (OR = 0.82, 95% CI, 0.74–0.91, p = 0.000), and Anaerostipes (OR = 0.78, 95% CI, 0.63–0.95, p = 0.016) exerted a negative impact on RA (Figure 2). The estimates of MR Egger, Weighted median, Simple mode, and Weighted mode were consistent with IVW listed in Supplementary Table 1. MR-Egger intercept and Mendelian randomization pleiotropy residual sum and outlier (MR-PRESSO) were utilized to investigate the directional pleiotropy, and all p-values exceeded 0.05, suggesting no significant pleiotropy. The Cochran Q-statistic of the IVW test and the MR Egger regression were used to assess heterogeneity. No discernible heterogeneity was observed across all studies (Supplementary Table 1).
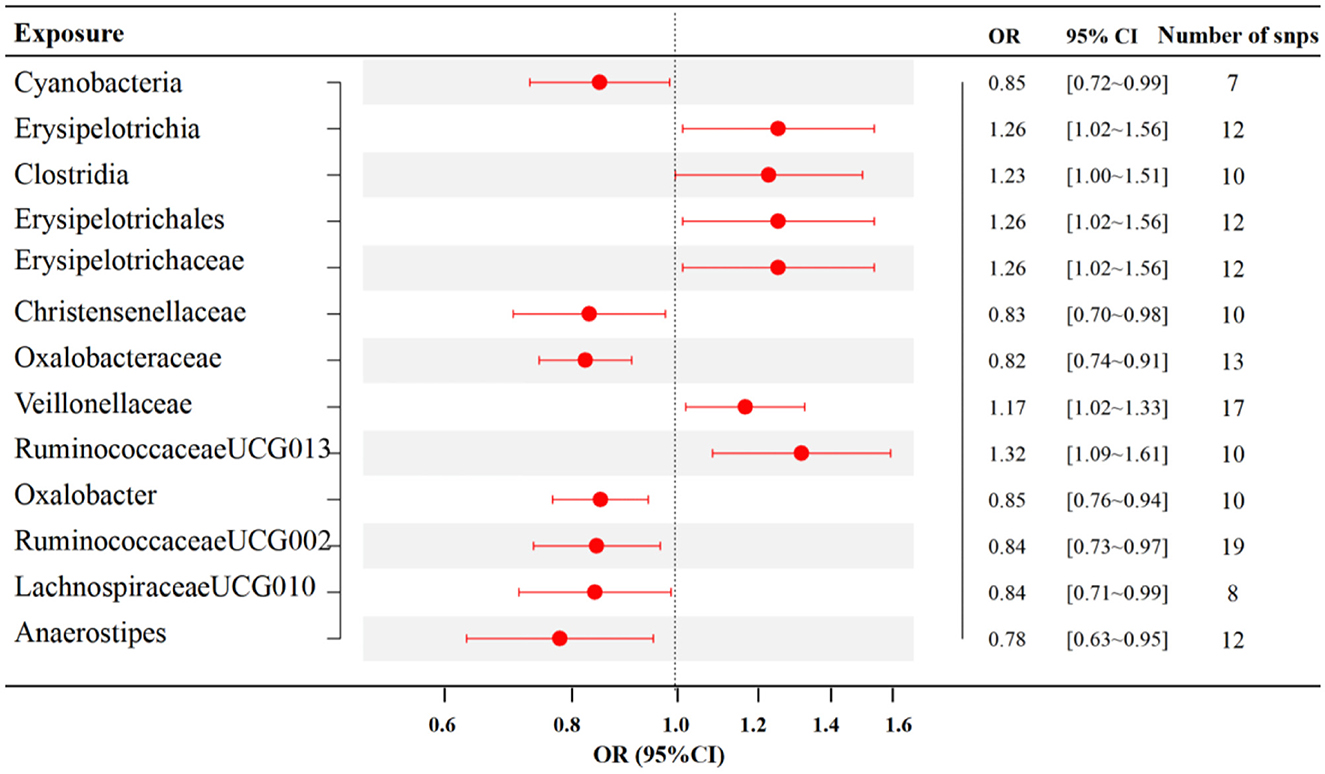
Figure 2. Associations between specific gut microbiota and RA risk (p < 1 × 10−5). SNP, single nucleotide polymorphism; OR, odds ratio; CI, confidence interval.
The findings from the forest plot and scatter plot consistently indicated that Genus_RuminococcaceaeUCG013 linked to a higher RA risk. Furthermore, the leave-one-out sensitivity test was conducted to assess the individual contribution of a single SNP on the overall analysis, and the results demonstrated that no specific SNP exerted a predominant effect (Figures 3A–C). The scatter plot, forest plot, and leave-one-out plot of the remaining 12 bacterial taxa were presented in Supplementary Figures 1–12.
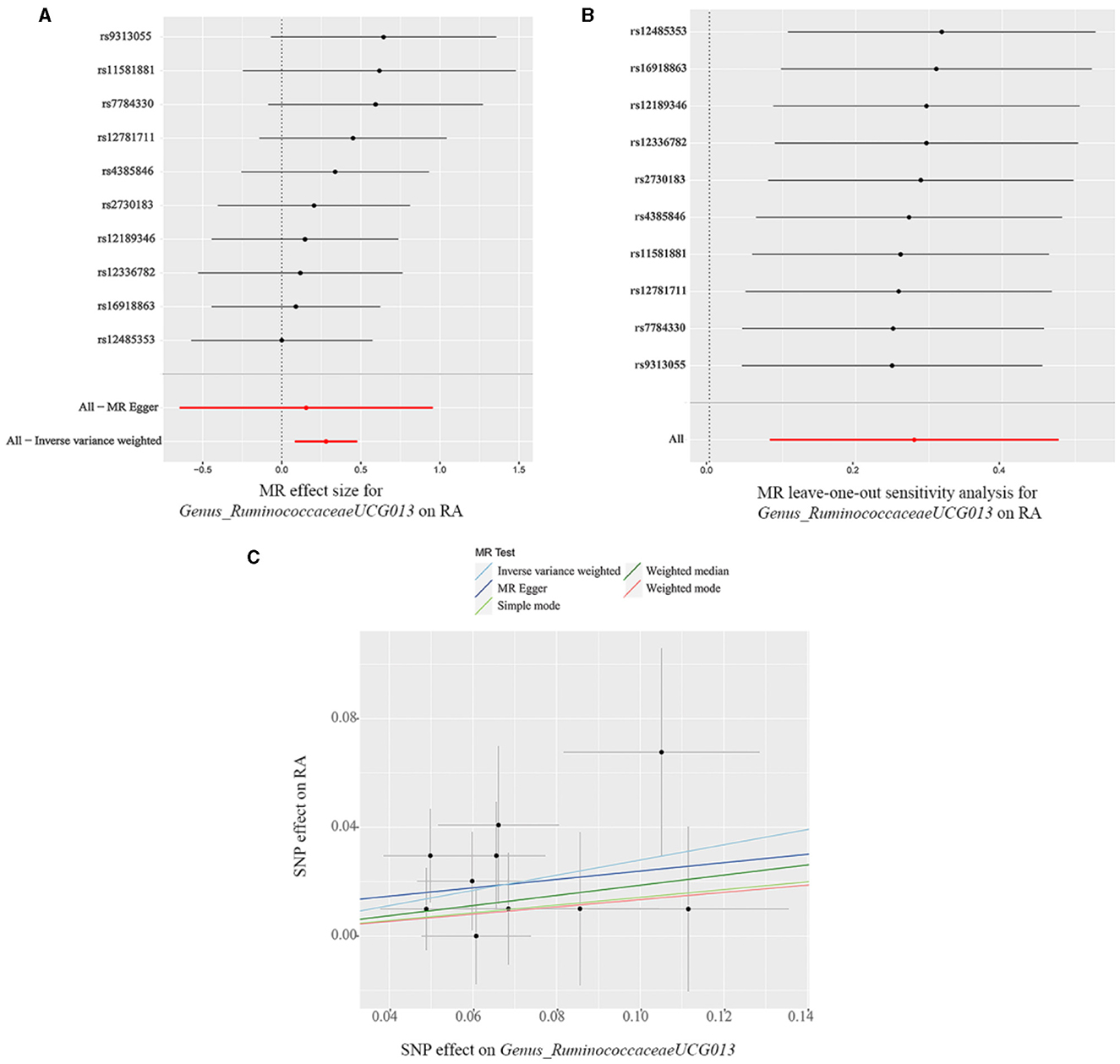
Figure 3. (A) Forest plot, (B) leave-one-out sensitivity analysis, and (C) scatter plot of the causal effect of Genus_RuminococcaceaeUCG013 on RA risk.
3.1.2 Associations between gut metabolites and RA
We initially included 1,924 significant (p < 1 × 10−5) SNPs or their proxies that were associated with gut metabolite features as genetic instrumental variables, including 217 metabolites (113 polar analytes and 104 lipid analytes). After removing the SNPs with LD effects and independence from RA, 1,864 SNPs were selected for further analysis. The F- statistics of each included SNPs were all >10, indicating that weak IV bias could be effectively avoided. The primary information of SNPs, including β, standard error (SE), and p-value, were compiled in Supplementary Table 2.
Twelve gut metabolites were filtered out using the IVW method with p < 0.05, in which TAG 60_12 and deoxycholate arginine were excluded because the estimation directions of IVW, weighted median, and MR-Egger methods were inconsistent. The LPC 20_3 gene was removed with significant variability (I2 ≥ 50%). Furthermore, the results were judged to be reliable, with no evidence of heterogeneity, pleiotropic effects, or outliers, as shown in Supplementary Table 2.
MR analyses indicated eight gut metabolites were found to have causal associations with RA, including three amino acids and five lipid analytes (Figure 4). In tryptophan and glycine metabolism, our study revealed that indole-3-propionate (IPA) (OR = 0.94, 95% CI, 0.90–0.99, p = 0.021) and glycine (OR = 0.94, 95% CI, 0.89–0.99, p = 0.023) were estimated to exert a negative impact on RA. However, argininosuccinate (OR = 1.05, 95% CI, 1.00–1.09, p = 0.039) exerted a positive impact on RA. For the lipid analytes, SM 16_1 (OR = 0.89, 95% CI, 0.83–0.96, p = 0.003) was estimated to exert a negative impact on RA, CE 20_4 (OR = 1.06, 95% CI, 1.01–1.10, p = 0.009), TAG 58_8 (OR = 1.07, 95% CI, 1.02–1.13, p = 0.012), PC 40_6(OR = 1.07, 95% CI, 1.01–1.14, p = 0.027), and LPC 20_4 (OR = 1.05, 95% CI, 1.00–1.11, p = 0.039) were exert a positive impact on RA.
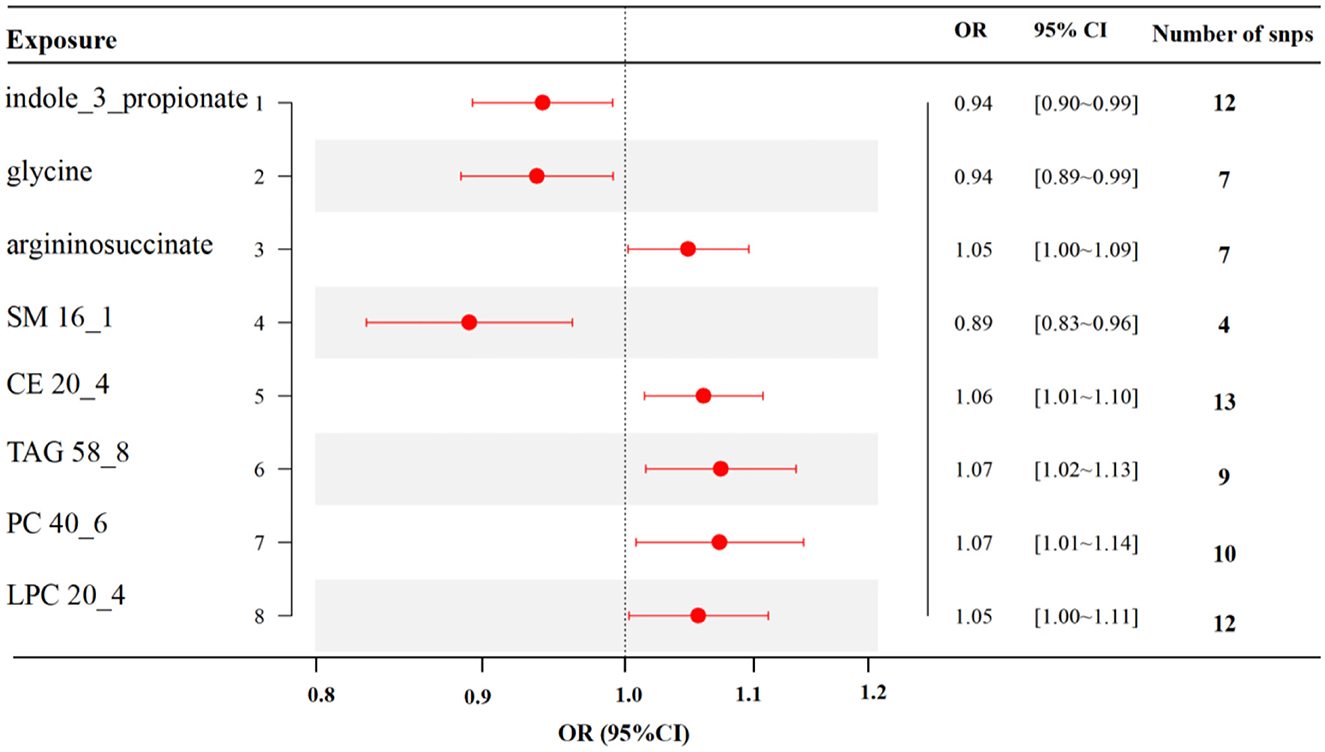
Figure 4. MR results of causal links between gut metabolites and RA risk (p < 1 × 10−5). SM, sphingomyelin; CE, cholesteryl ester; TAG, triglycerides; PC, phosphatidylcholine; LPC, lysoPC.
The scatter plot and forest plot also revealed IPA linked to lower RA risk. Additionally, the leave-one-out sensitivity test showed that no single SNP had a dominant effect on the overall assessment (Figures 5A–C). The scatter plot, forest plot, and leave-one-out plot of the remaining seven gut metabolites were shown in Supplementary Figures 13–19.
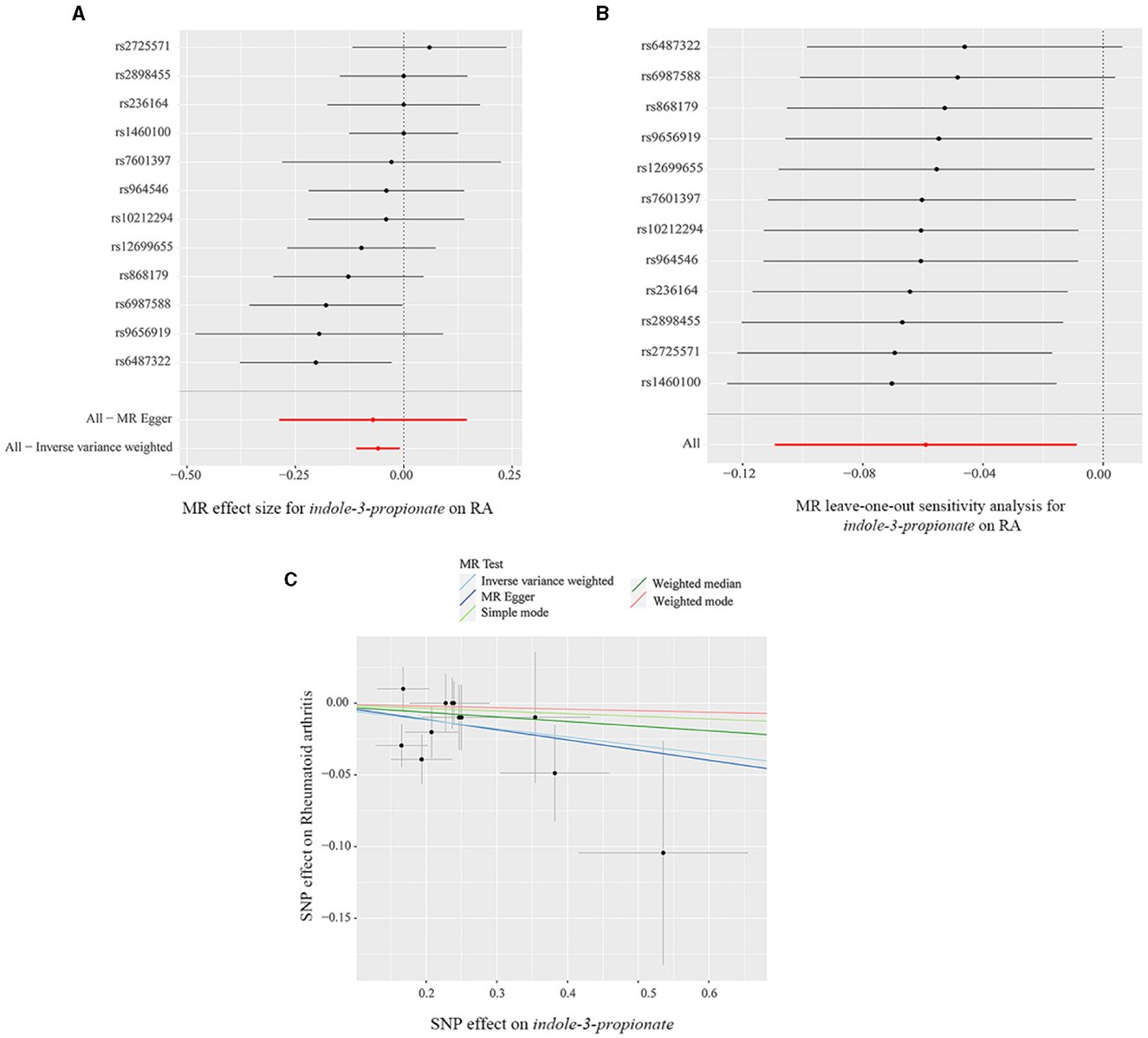
Figure 5. (A) Forest plot, (B) leave-one-out sensitivity analysis, and (C) scatter plot of the causal effect of indole-3-propionate on RA risk.
3.1.3 Metabolic pathway analysis
The metabolic pathway analysis of eight metabolites identified four significant metabolic pathways, including Arginine and Proline Metabolism (p = 0.00263), Alanine Metabolism (p = 0.0329), Glutathione Metabolism (p = 0.0406), and Carnitine Synthesis (p = 0.0425), as shown in Figure 6 and Supplementary Table 3.
3.2 Animal experiments
3.2.1 Assessment of arthritis severity
The CIA group exhibited significant joint swelling (p < 0.05). Pathologically, there was notable destruction of the joint structure, narrowing of the joint space, and extensive infiltration of inflammatory cells in the CIA group (Supplementary Figures 20A, B). These results suggest that a CIA rat model was effectively established.
3.3 β-diversity
We performed a principal component analysis (PCoA) based on the weighted UniFrac distance and NMDS analysis to assess β-diversity. The PCoA analysis indicated that the two groups achieved statistical significance (p = 0.004) (Figure 7A) and the NMDS analysis (Stress = 0.126, p = 0.004). The results were consistent with the previous findings (Figure 7B).
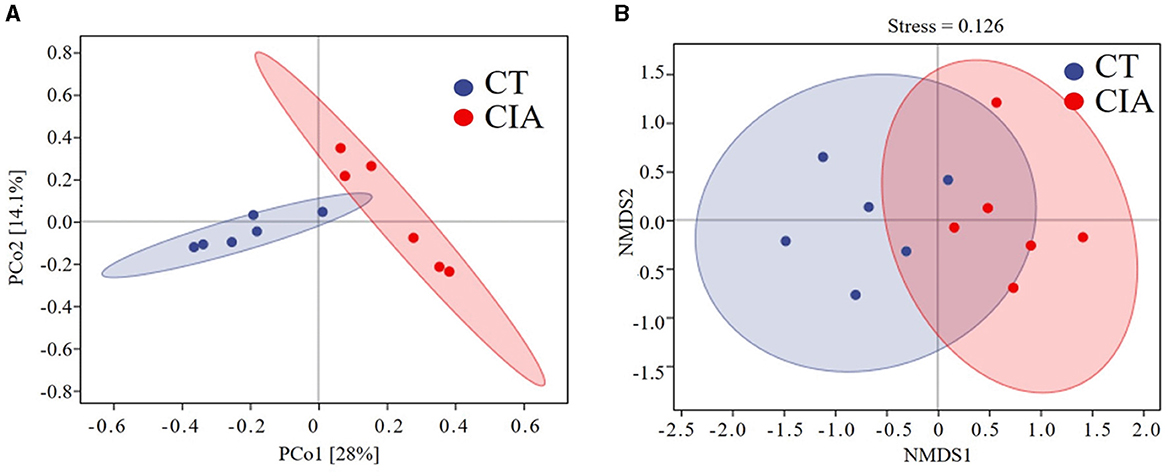
Figure 7. (A) β-diversity was described by PCoA based on weighted UniFrac distance metric; (B) and non-metric multidimensional scaling (NMDS) analysis. CIA, collagen-induced arthritis group; CT, healthy control group.
3.3.1 Validation of MR results
To evaluate the MR findings, animal experiments were performed. We extracted the relative abundance of Class_Clostridia, Order_Erysipelotrichales, Family_Christensenellaceae, Family_Erysipelotrichaceae, and Genus_Lachnospiraceae and compared them using bar plots (Figures 8A–E).
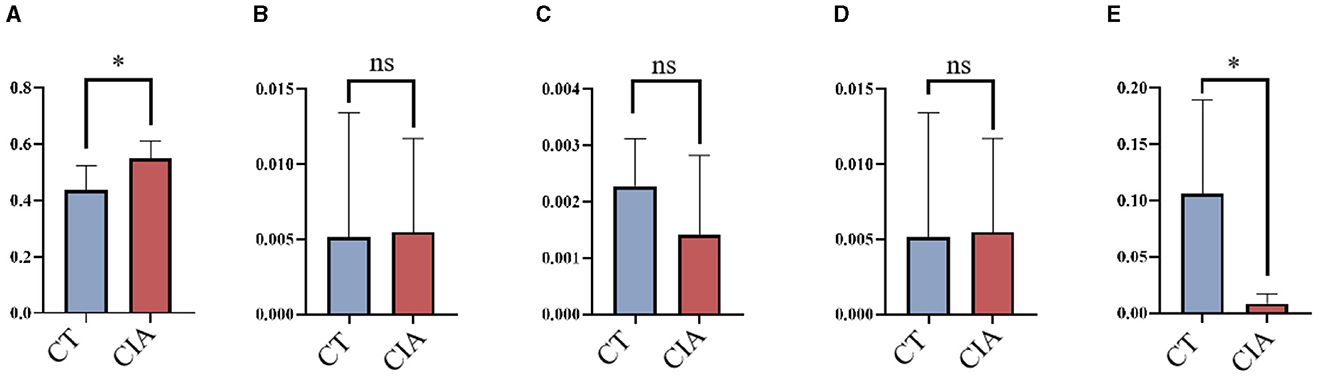
Figure 8. The relative abundance of bacterial taxa by bar plots (A–E) the relative abundance of Class_Clostridia, Order_Erysipelotrichales, Family_Christensenellaceae, Family_Erysipelotrichaceae, and Genus_Lachnospiraceae in the control group and CIA group. *p < 0.05. CIA, collagen-induced arthritis group; CT, healthy control group.
4 Discussion
Our study showed that increased abundance of RuminococcaceaeUCG013, Erysipelotrichia, Erysipelotrichaceae, Erysipelotrichales, Clostridia, and Veillonellaceae was linked to higher RA risk. Conversely, Cyanobacteria, RuminococcaceaeUCG002, Oxalobacter, LachnospiraceaeUCG010, Christensenellaceae, Oxalobacteraceae, and Anaerostipes were estimated to exert a negative impact on RA. Furthermore, we identified three amino acids and five lipid analytes, which are linked to the gut microbiota, as having a causal relationship with RA risk, highlighting their importance in the gut microbiota-metabolite axis.
The GI tract is abundantly colonized with trillions of symbiotic gut microbiomes that participate in the modulation and maintenance of the host immune system (Zhao et al., 2022). Altering specific bacterial lineages may result in dysbiosis within the gut microbiota, ultimately leading to the onset of autoimmune diseases (Wang et al., 2022). Multiple studies have shown that the microbiota composition, including pathogenic bacteria and probiotics, is significantly altered in RA patients. Probiotics, such as Bifidobacterium, are live microorganisms that can benefit health by competing with pathogenic bacteria for nutrition and colonization sites (Chen et al., 2021). Probiotics have been shown to reduce RA symptoms by producing antibiotics and strengthening the intestinal barrier, which enhances immune function modulation (Ferro et al., 2021). However, the causal relationship between RA risk and gut microbiota dysbiosis is ambiguous. This study is the first MR analysis that explores this topic from phylum to genus levels of 211 taxa. Seven bacterial taxa were estimated to exert a negative impact on RA. Among these, Lachnospiraceae and Ruminococcaceae are recognized as probiotic strains that colonize the human gut. Takahashi reported the abundance of Lachnospiraceae was downregulated in RA patients compared to healthy controls. Further animal experiments have identified Lachnospiraceae as one of the major butyrate producers that can limit the autoimmune response by promoting the differentiation of follicular regulatory T cells (Takahashi et al., 2020). Data from two cohorts identified that RA patients had less Firmicutes of the Ruminococcaceae family and more Proteobacteria of the Enterobacteriaceae family than HC, indicating the protective effect of Ruminococcaceae on RA (Breban et al., 2017). Interestingly, our analysis showed that the genera RuminococcaceaeUCG002 and RuminococcaceaeUCG013 have varying impacts on RA risk, which reminds us that inconsistencies in previous clinical studies may be due to insufficiently digging deeper into the classification at the genera level of gut microbiota. A few studies have reported the role of the family Oxalobacteraceae and genus Oxalobacter in RA. A case-control study reported that the relative abundance of Oxalobacter was negatively related to TNF-α, IL-6, and IL-17 in RA patients. These cytokines are closely linked to bone erosion via enhanced differentiation and activation of osteoclasts (Li Y. et al., 2021). Cyanobacteria, Christensenellaceae, and Anaerostipes have not been reported to be protective factors for RA, while alterations in other autoimmune diseases have been reported. For example, Ma et al. (2022) reported that Anaerostipes was enriched in healthy controls compared to Crohn's patients. We also identified six bacterial taxa that were estimated to exert a positive impact on RA. The family Erysipelotrichaceae and the class Clostridia are proinflammatory bacteria overrepresented in RA animal models (Nguyen et al., 2022; Wang et al., 2023). The results of Veillonellaceae in this study were also consistent with the previous literature (Manoil et al., 2021). Regarding class Erysipelotrichia and order Erysipelotrichales, there are few reports about their role in RA. Overall, these results suggest that dysregulation of gut microbiota might contribute to the development of RA, though the underlying mechanisms are still to be elucidated.
Eight gut metabolites were identified to have causal associations from this MR analysis: IPA, glycine, argininosuccinate, SM 16_1, CE 20_4, TAG 58_8, PC 40_6, and LPC 20_4. Among these, the metabolic pathway analysis highlighted four pathways causally associated to RA, some of which are well-documented in experimental studies for their role in RA pathogenesis. For instance, recent integrated analyses of plasma metabolomics, intestinal bacteria, and fungi have shown that arginine and proline metabolic pathways are involved in RA pathogenesis (Zhu et al., 2022).
Among these eight metabolites, IPA is a tryptophan-derived intestinal bacterial metabolite known for inducing the expression of tight junction proteins such as ZO-1 and Occludin, promoting intestinal epithelium homeostasis, inhibiting NF-κB signaling, and reducing levels of proinflammatory cytokines like TNF-α, IL-1β, and IL-6 (Zhao et al., 2019). Additionally, IPA enhances the protective mucus barrier by increasing the production of mucins, specifically MUC2 and MUC4, and secretion products from goblet cells, including TFF3 and RELMβ (Li J. et al., 2021). Its production is closely linked to the presence of the genus Lachnospiraceae, known for its protective effects against RA. Thus, we speculate that probiotic bacteria may metabolize IPA to bolster intestinal barrier functions, thereby mitigating the inflammatory responses associated with RA injury, as illustrated in Figure 9. Similar mechanisms have been observed in other diseases (Zhao et al., 2019; Serger et al., 2022). Supporting our findings, other metabolites like LPC 20_4 and argininosuccinate are implicated in RA pathogenesis, while glycine appears to exert a protective effect by reducing oxidative stress on intestinal epithelial cells, decreasing pro-inflammatory cytokine release, and alleviating epithelial barrier damage (Marchesini et al., 2003; Tang et al., 2021). However, research on the roles of remaining metabolites (SM 16_1, CE 20_4, TAG 58_8, PC 40_6) in RA remains limited.
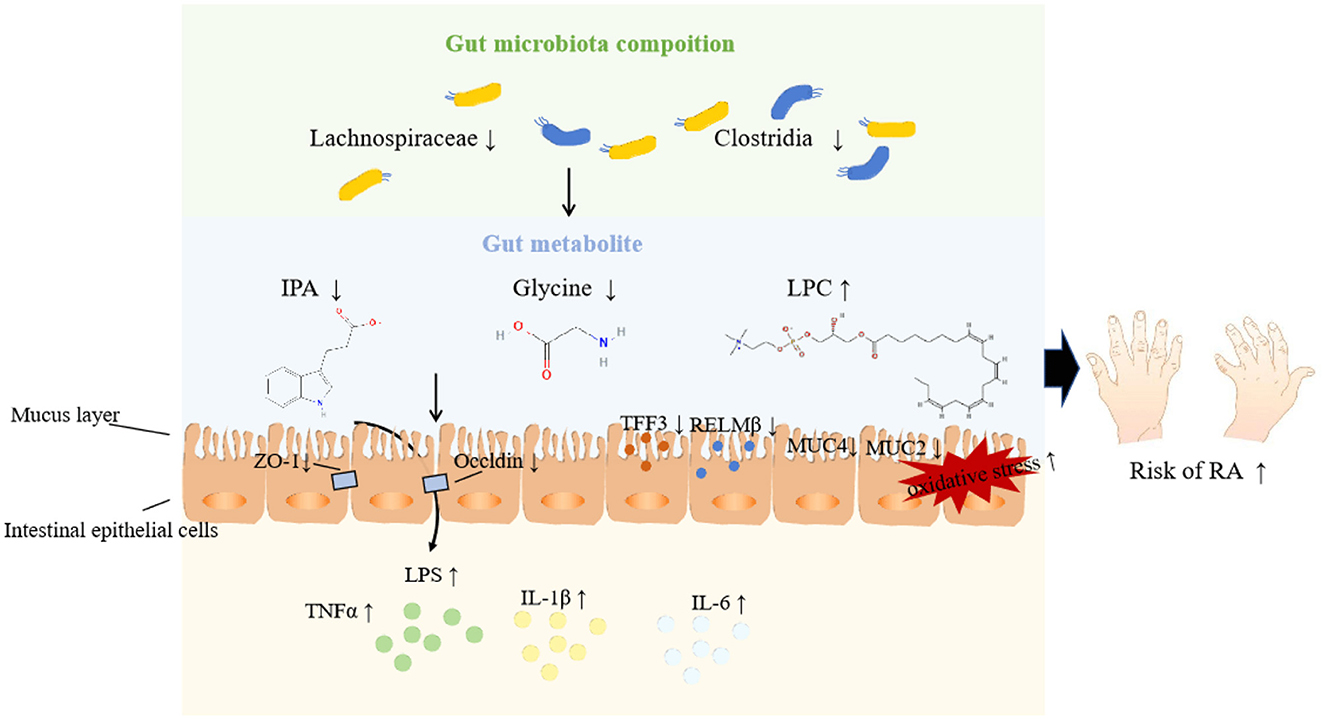
Figure 9. Potential pathways underpinning the relationship among gut microbiota, metabolites, and RA.
The primary strength of this study lies in the application of the MR approach, which minimizes both confounding and reverse causality, providing a more robust assessment of causality than observational studies. To our knowledge, this is the first MR analysis examining the causal relationships between gut microbiome, metabolites, and RA. Various analyses were conducted to assess the robustness of the results. Moreover, animal experiments were employed to evaluate the outcomes of the MR analysis, reinforcing the conviction of the causal associations between gut microbiota and RA. However, our study has some limitations. Firstly, due to the multi-stage statistical approach, the adjustment for FDR was not conducted to identify potential strains and metabolites that may be causally associated with rheumatoid arthritis to the greatest extent possible. Secondly, our animal experiments do not definitively establish a causal association between gut microbiota and RA; further gut microbiota transplantation studies are needed to clarify this association. Thirdly, gut microbiota composition is influenced by various factors such as diet, lifestyle, gender, sex hormones, disease severity, and medication status. However, further subgroup analyses were challenging to perform due to the unavailability of detailed information.
5 Conclusion
Using MR, our study identified causal associations between specific gut microbiota, metabolites, and RA. These findings support the significant role of gut microbiota and metabolites in RA pathogenesis, particularly highlighting the Genus_Lachnospiraceae and IPA.
Data availability statement
The original contributions presented in the study are included in the article/Supplementary material, further inquiries can be directed to the corresponding authors.
Ethics statement
Ethical approval was not required for the study involving humans in accordance with the local legislation and institutional requirements. Written informed consent to participate in this study was not required from the participants or the participants' legal guardians/next of kin in accordance with the national legislation and the institutional requirements. The animal studies were approved by Zhejiang Chinese Medical University Experimental Animal Management and Ethics Committee. The studies were conducted in accordance with the local legislation and institutional requirements. Written informed consent was obtained from the owners for the participation of their animals in this study.
Author contributions
DZ: Validation, Writing – original draft, Writing – review & editing, Data curation, Funding acquisition, Methodology, Project administration. WJ: Validation, Visualization, Investigation, Resources, Writing – review & editing. WS: Project administration, Data curation, Investigation, Software, Writing – original draft. QW: Conceptualization, Resources, Supervision, Writing – original draft. MC: Conceptualization, Funding acquisition, Project administration, Resources, Supervision, Writing – review & editing.
Funding
The author(s) declare financial support was received for the research, authorship, and/or publication of this article. This research was supported by the Foundation from Joint Science and Technology Program Project by the National Administration of Traditional Chinese Medicine and the Zhejiang Administration of Traditional Chinese Medicine (GZY-ZJ-KJ-23016), and the Foundation from Zhejiang Provincial Administration of Traditional Chinese Medicine (2023ZL057, 2021ZQ028).
Acknowledgments
We thank all the researchers of the studies included in the current analyses.
Conflict of interest
The authors declare that the research was conducted in the absence of any commercial or financial relationships that could be construed as a potential conflict of interest.
Publisher's note
All claims expressed in this article are solely those of the authors and do not necessarily represent those of their affiliated organizations, or those of the publisher, the editors and the reviewers. Any product that may be evaluated in this article, or claim that may be made by its manufacturer, is not guaranteed or endorsed by the publisher.
Supplementary material
The Supplementary Material for this article can be found online at: https://www.frontiersin.org/articles/10.3389/fmicb.2024.1431367/full#supplementary-material
References
Breban, M., Tap, J., Leboime, A., Said-Nahal, R., Langella, P., Chiocchia, G., et al. (2017). Faecal microbiota study reveals specific dysbiosis in spondyloarthritis. Ann. Rheum. Dis. 76, 1614–1622. doi: 10.1136/annrheumdis-2016-211064
Cai, J., He, L., Wang, H., Rong, X., Chen, M., Shen, Q., et al. (2022a). Genetic liability for prescription opioid use and risk of cardiovascular diseases: a multivariable Mendelian randomization study. Addiction 117, 1382–1391. doi: 10.1111/add.15767
Cai, J., Li, X., Wu, S., Tian, Y., Zhang, Y., Wei, Z., et al. (2022b). Assessing the causal association between human blood metabolites and the risk of epilepsy. J. Transl. Med. 20:437. doi: 10.1186/s12967-022-03648-5
Caporaso, J. G., Kuczynski, J., Stombaugh, J., Bittinger, K., Bushman, F. D., Costello, E. K., et al. (2010). QIIME allows analysis of high-throughput community sequencing data. Nat. Methods 7, 335–336. doi: 10.1038/nmeth.f.303
Chen, Y., Ma, C., Liu, L., He, J., Zhu, C., Zheng, F., et al. (2021). Analysis of gut microbiota and metabolites in patients with rheumatoid arthritis and identification of potential biomarkers. Aging 13, 23689–23701. doi: 10.18632/aging.203641
Chiang, H. I., Li, J. R., Liu, C. C., Liu, P. Y., Chen, H. H., Chen, Y. M., et al. (2019). An association of gut microbiota with different phenotypes in Chinese patients with rheumatoid arthritis. J. Clin. Med. 8:1770. doi: 10.3390/jcm8111770
Davies, N. M., Holmes, M. V., and Davey Smith, G. (2018). Reading Mendelian randomisation studies: a guide, glossary, and checklist for clinicians. BMJ 362:k601. doi: 10.1136/bmj.k601
Deane, K. D., Demoruelle, M. K., Kelmenson, L. B., Kuhn, K. A., Norris, J. M., and Holers, V. M. (2017). Genetic and environmental risk factors for rheumatoid arthritis. Best Pract. Res. Clin. Rheumatol. 31, 3–18. doi: 10.1016/j.berh.2017.08.003
Edgar, R. C. (2010). Search and clustering orders of magnitude faster than BLAST. Bioinformatics 26, 2460–2461. doi: 10.1093/bioinformatics/btq461
Ferro, M., Charneca, S., Dourado, E., Guerreiro, C. S., and Fonseca, J. E. (2021). Probiotic supplementation for rheumatoid arthritis: a promising adjuvant therapy in the gut microbiome era. Front. Pharmacol. 12:711788. doi: 10.3389/fphar.2021.711788
Guo, Z., Zhang, T., Yun, Z., Jin, Q., Cao, X., Kong, D., et al. (2023). Assessing the causal relationships between human blood metabolites and the risk of NAFLD: a comprehensive mendelian randomization study. Front. Genet. 14:1108086. doi: 10.3389/fgene.2023.1108086
He, J., Chu, Y., Li, J., Meng, Q., Liu, Y., Jin, J., et al. (2022). Intestinal butyrate-metabolizing species contribute to autoantibody production and bone erosion in rheumatoid arthritis. Sci. Adv. 8:eabm1511. doi: 10.1126/sciadv.abm1511
Hemani, G., Zheng, J., Elsworth, B., Wade, K. H., Haberland, V., Baird, D., et al. (2018). The MR-Base platform supports systematic causal inference across the human phenome. ELife 7:12. doi: 10.7554/eLife.34408.012
Hou, T., Dai, H., Wang, Q., Hou, Y., Zhang, X., Lin, H., et al. (2023). Dissecting the causal effect between gut microbiota, DHA, and urate metabolism: a large-scale bidirectional Mendelian randomization. Front. Immunol. 14:1148591. doi: 10.3389/fimmu.2023.1148591
Hu, X., Binxu, Q., Shao, G.-Z., Huang, Y., and Qiu, W. (2024). Gut microbiota, circulating metabolites, and gallstone disease: a Mendelian randomization study. Front. Microbiol. 15:1336673. doi: 10.3389/fmicb.2024.1336673
Kishikawa, T., Maeda, Y., Nii, T., Motooka, D., Matsumoto, Y., Matsushita, M., et al. (2020). Metagenome-wide association study of gut microbiome revealed novel aetiology of rheumatoid arthritis in the Japanese population. Ann. Rheum. Dis. 79, 103–111. doi: 10.1136/annrheumdis-2019-215743
Kurilshikov, A., Medina-Gomez, C., Bacigalupe, R., Radjabzadeh, D., Wang, J., Demirkan, A., et al. (2021). Large-scale association analyses identify host factors influencing human gut microbiome composition. Nat. Genet. 53, 156–165. doi: 10.1038/s41588-020-00763-1
Li, C., Han, Y., Luo, X., Qian, C., Li, Y., Su, H., et al. (2023). Immunomodulatory nano-preparations for rheumatoid arthritis. Drug Deliv. 30, 9–19. doi: 10.1080/10717544.2022.2152136
Li, J., Zhang, L., Wu, T., Li, Y., Zhou, X., and Ruan, Z. (2021). Indole-3-propionic acid improved the intestinal barrier by enhancing epithelial barrier and mucus barrier. J. Agric. Food Chem. 69, 1487–1495. doi: 10.1021/acs.jafc.0c05205
Li, Y., Zhang, S. X., Yin, X. F., Zhang, M. X., Qiao, J., Xin, X. H., et al. (2021). The gut microbiota and its relevance to peripheral lymphocyte subpopulations and cytokines in patients with rheumatoid arthritis. J. Immunol. Res. 2021:6665563. doi: 10.1155/2021/6665563
Ma, X., Lu, X., Zhang, W., Yang, L., Wang, D., Xu, J., et al. (2022). Gut microbiota in the early stage of Crohn's disease has unique characteristics. Gut Pathog. 14:46. doi: 10.1186/s13099-022-00521-0
Manoil, D., Bostanci, N., Mumcu, G., Inanc, N., Can, M., Direskeneli, H., et al. (2021). Novel and known periodontal pathogens residing in gingival crevicular fluid are associated with rheumatoid arthritis. J. Periodontol. 92, 359–370. doi: 10.1002/JPER.20-0295
Marchesini, G., Bianchi, G., Merli, M., Amodio, P., Panella, C., Loguercio, C., et al. (2003). Nutritional supplementation with branched-chain amino acids in advanced cirrhosis: a double-blind, randomized trial. Gastroenterology 124, 1792–1801. doi: 10.1016/S0016-5085(03)00323-8
Nguyen, N. T., Sun, W. H., Chen, T. H., Tsai, P. C., Chen, C. C., and Huang, S. L. (2022). Gut mucosal microbiome is perturbed in rheumatoid arthritis mice and partly restored after TDAG8 deficiency or suppression by salicylanilide derivative. Int. J. Mol. Sci. 23:73527. doi: 10.3390/ijms23073527
Okada, Y., Wu, D., Trynka, G., Raj, T., Terao, C., Ikari, K., et al. (2014). Genetics of rheumatoid arthritis contributes to biology and drug discovery. Nature 506, 376–381. doi: 10.1038/nature12873
Papadimitriou, N., Dimou, N., Tsilidis, K. K., Banbury, B., Martin, R. M., Lewis, S. J., et al. (2020). Physical activity and risks of breast and colorectal cancer: a Mendelian randomisation analysis. Nat. Commun. 11:597. doi: 10.1038/s41467-020-14389-8
Quast, C., Pruesse, E., Yilmaz, P., Gerken, J., Schweer, T., Yarza, P., et al. (2013). The SILVA ribosomal RNA gene database project: improved data processing and web-based tools. Nucl. Acids Res. 41, D590–D596. doi: 10.1093/nar/gks1219
Raaschou, P., Soderling, J., Turesson, C., Askling, J., and Group, A. S. (2018). Tumor necrosis factor inhibitors and cancer recurrence in Swedish patients with rheumatoid arthritis: a nationwide population-based cohort study. Ann. Intern. Med. 169, 291–299. doi: 10.7326/M17-2812
Rhee, E. P., Ho, J. E., Chen, M. H., Shen, D., Cheng, S., Larson, M. G., et al. (2013). A genome-wide association study of the human metabolome in a community-based cohort. Cell Metab. 18, 130–143. doi: 10.1016/j.cmet.2013.06.013
Rosloniec, E. F., Cremer, M., Kang, A. H., Myers, L. K., and Brand, D. D. (2010). Collagen-induced arthritis. Curr. Protoc. Immunol. Chapter 15:15.5.1–15.5.25. doi: 10.1002/0471142735.im1505s89
Rosser, E. C., Piper, C. J. M., Matei, D. E., Blair, P. A., Rendeiro, A. F., Orford, M., et al. (2020). Microbiota-derived metabolites suppress arthritis by amplifying aryl-hydrocarbon receptor activation in regulatory B cells. Cell Metab. 31, 837–851 e810. doi: 10.1016/j.cmet.2020.03.003
Sanna, S., van Zuydam, N. R., Mahajan, A., Kurilshikov, A., Vich Vila, A., Võsa, U., et al. (2019). Causal relationships among the gut microbiome, short-chain fatty acids and metabolic diseases. Nat. Genet. 51, 600–605. doi: 10.1038/s41588-019-0350-x
Sayers, E. W., Agarwala, R., Bolton, E. E., Brister, J. R., Canese, K., Clark, K., et al. (2019). Database resources of the National Center for Biotechnology Information. Nucleic Acids Res. 47, D23–D28. doi: 10.1093/nar/gky1069
Serger, E., Luengo-Gutierrez, L., Chadwick, J. S., Kong, G., Zhou, L., Crawford, G., et al. (2022). The gut metabolite indole-3 propionate promotes nerve regeneration and repair. Nature 607, 585–592. doi: 10.1038/s41586-022-04884-x
Takahashi, D., Hoshina, N., Kabumoto, Y., Maeda, Y., Suzuki, A., Tanabe, H., et al. (2020). Microbiota-derived butyrate limits the autoimmune response by promoting the differentiation of follicular regulatory T cells. EBioMedicine 58:102913. doi: 10.1016/j.ebiom.2020.102913
Tang, X., Wang, W., Hong, G., Duan, C., Zhu, S., Tian, Y., et al. (2021). Gut microbiota-mediated lysophosphatidylcholine generation promotes colitis in intestinal epithelium-specific Fut2 deficiency. J. Biomed. Sci. 28:20. doi: 10.1186/s12929-021-00711-z
Verbanck, M., Chen, C. Y., Neale, B., and Do, R. (2018). Detection of widespread horizontal pleiotropy in causal relationships inferred from Mendelian randomization between complex traits and diseases. Nat. Genet. 50, 693–698. doi: 10.1038/s41588-018-0099-7
Wang, Q., Zhang, S. X., Chang, M. J., Qiao, J., Wang, C. H., Li, X. F., et al. (2022). Characteristics of the gut microbiome and its relationship with peripheral CD4(+) T cell subpopulations and cytokines in rheumatoid arthritis. Front. Microbiol. 13:799602. doi: 10.3389/fmicb.2022.799602
Wang, X., Liu, D., Li, D., Yan, J., Yang, J., Zhong, X., et al. (2023). Combined treatment with glucosamine and chondroitin sulfate improves rheumatoid arthritis in rats by regulating the gut microbiota. Nutr. Metab. 20:22. doi: 10.1186/s12986-023-00735-2
Xiang, K., Wang, P., Xu, Z., Hu, Y.-Q., He, Y.-S., Chen, Y., et al. (2021). Causal effects of gut microbiome on systemic lupus erythematosus: a two-sample mendelian randomization study. Front. Immunol. 12:667097. doi: 10.3389/fimmu.2021.667097
Yang, W., and Cong, Y. (2021). Gut microbiota-derived metabolites in the regulation of host immune responses and immune-related inflammatory diseases. Cell. Mol. Immunol. 18, 866–877. doi: 10.1038/s41423-021-00661-4
Yu, D., Du, J., Pu, X., Zheng, L., Chen, S., Wang, N., et al. (2021). The gut microbiome and metabolites are altered and interrelated in patients with rheumatoid arthritis. Front. Cell. Infect. Microbiol. 11:763507. doi: 10.3389/fcimb.2021.763507
Yu, Y., Liu, Q., Li, H., Wen, C., and He, Z. (2018). Alterations of the gut microbiome associated with the treatment of hyperuricaemia in male rats. Front. Microbiol. 9:2233. doi: 10.3389/fmicb.2018.02233
Yuan, J., Peng, L., Luan, F., Li, J., Zhang, J., Jiang, W., et al. (2022). Causal effects of genetically predicted cystatin C on osteoporosis: a two-sample mendelian randomization study. Front. Genet. 13:849206. doi: 10.3389/fgene.2022.849206
Zhao, T., Wei, Y., Zhu, Y., Xie, Z., Hai, Q., Li, Z., et al. (2022). Gut microbiota and rheumatoid arthritis: From pathogenesis to novel therapeutic opportunities. Front. Immunol. 13:1007165. doi: 10.3389/fimmu.2022.1007165
Zhao, Z. H., Xin, F. Z., Xue, Y., Hu, Z., Han, Y., Ma, F., et al. (2019). Indole-3-propionic acid inhibits gut dysbiosis and endotoxin leakage to attenuate steatohepatitis in rats. Exp. Mol. Med. 51, 1–14. doi: 10.1038/s12276-019-0304-5
Keywords: Mendelian randomization, gut microbiota, metabolites, autoimmune disease, rheumatoid arthritis
Citation: Zhou D, Jiao W, Shi W, Wang Q and Chen M (2024) Mendelian randomization identifies causal associations between GWAS-associated bacteria and their metabolites and rheumatoid arthritis. Front. Microbiol. 15:1431367. doi: 10.3389/fmicb.2024.1431367
Received: 11 May 2024; Accepted: 15 August 2024;
Published: 02 September 2024.
Edited by:
Jennifer K. Spinler, Baylor College of Medicine, United StatesReviewed by:
Jonathan Monk, University of California, San Diego, United StatesTeleky Bernadette-Emoke, University of Agricultural Sciences and Veterinary Medicine of Cluj-Napoca, Romania
Joseph Atia Ayariga, Alabama State University, United States
Copyright © 2024 Zhou, Jiao, Shi, Wang and Chen. This is an open-access article distributed under the terms of the Creative Commons Attribution License (CC BY). The use, distribution or reproduction in other forums is permitted, provided the original author(s) and the copyright owner(s) are credited and that the original publication in this journal is cited, in accordance with accepted academic practice. No use, distribution or reproduction is permitted which does not comply with these terms.
*Correspondence: Qiao Wang, wangqiao0309@163.com; Muzhi Chen, cmzzjsxhyy@163.com
†These authors have contributed equally to this work