- 1School of Geography and Tourism, Anhui Normal University, Wuhu, China
- 2Key Laboratory of Vegetation Restoration and Management of Degraded Ecosystems, Chinese Academy of Sciences, Guangzhou, China
- 3Lushan Botanical Garden, Chinese Academy of Sciences, Jiujiang, China
- 4School of Geomatics and Municipal Engineering, Zhejiang University of Water Resources and Electric Power, Hangzhou, China
Bacteria play a crucial role in pollutant degradation, biogeochemical cycling, and energy flow within river ecosystems. However, the underlying mechanisms governing bacterial community assembly and their response to environmental factors at seasonal scales in subtropical rivers remain poorly understood. In this study, we conducted 16S rRNA gene amplicon sequencing on water samples from the Liuxi River to investigate the composition, assembly processes, and co-occurrence relationships of bacterial communities during the wet season and dry season. The results demonstrated that seasonal differences in hydrochemistry significantly influenced the composition of bacterial communities. A more heterogeneous community structure and increased alpha diversity were observed during the dry season. Water temperature emerged as the primary driver for seasonal changes in bacterial communities. Dispersal limitation predominantly governed community assembly, however, during the dry season, its contribution increased due to decreased immigration rates. Co-occurrence network analysis reveals that mutualism played a prevailing role in shaping bacterial community structure. Compared to the wet season, the network of bacterial communities exhibited higher modularity, competition, and keystone species during the dry season, resulting in a more stable community structure. Although keystone species displayed distinct seasonal variations, Proteobacteria and Actinobacteria were consistently abundant keystone species maintaining network structure in both seasons. Our findings provide insights into how bacterial communities respond to seasonal environmental changes, uncovering underlying mechanisms governing community assembly in subtropical rivers, which are crucial for the effective management and conservation of riverine ecosystems.
1 Introduction
The river ecosystems, which belong to lotic ecosystems, serve as a crucial link between terrestrial and coastal ecosystems, playing a vital role in the material cycle of the biosphere (Ouyang et al., 2020). Bacterial communities, being fundamental and integral components of riverine ecosystems, are widely recognized as primary drivers of biogeochemical cycling, pollutant degradation, and energy exchange (Liu et al., 2021; Yang et al., 2023), thereby assuming key responsibilities in maintaining ecological health and equilibrium of rivers (Kallmeyer et al., 2012; Kuypers et al., 2018). It is well known that inputs of matter and energy from the surrounding environment to aquatic ecosystems exhibit temporal variations that pronouncedly influence bacterial communities (Gao et al., 2017; Xiao et al., 2021). The diversity, structure, and function of bacterial communities are greatly influenced by environmental changes (Sun C. et al., 2021; Sun H. et al., 2021; Wang et al., 2019; Zhang et al., 2021). Seasonal variation has been identified as a representative environmental change factor exerting a substantial impact on bacterial communities in time-series studies (Chen et al., 2023; Liu et al., 2022; Ren et al., 2019), and the response mechanisms of bacterial communities to environmental conditions may exhibit seasonal variability. Consequently, comprehending the seasonal distribution patterns of bacterial communities along a river holds immense significance for deciphering their response mechanisms to environmental changes and enhancing water quality and aquatic ecosystem function.
The processes governing the distribution, diversity, function, and succession of microbial communities are commonly referred to as the community assembly mechanism (Chen et al., 2019; Jiao et al., 2020). The niche-based theory proposes that deterministic processes, including abiotic factors (e.g., environmental filtration) and biotic factors (e.g., interspecific interactions), shape microbial communities (Dini-Andreote et al., 2015; Wang J. et al., 2020). In contrast, the neutral theory assumes that stochastic processes (e.g., birth, death, and dispersion events) govern microbial community assembly (Zhang et al., 2022). Currently, it is universally accepted that both stochastic and deterministic processes contribute to microbial community assembly (Shuwang et al., 2023). Nevertheless, ongoing debates persist regarding the relative significance of these processes in structuring microbial communities (Yi et al., 2021; Zeng et al., 2019). For example, Chen et al. (2019) and Zou et al. (2023) have emphasized stochasticity as the primary driving force behind community assembly, while Wang Y. et al. (2024) have underscored determinism as the main cause. Additionally, it has been reported that the variation of bacterial communities was driven by deterministic processes in winter but dominated by stochastic processes in summer within the same area (Fang et al., 2023). In recent years, a variety of models and algorithms, such as the Sloan neutral community model and null model (Sloan et al., 2010; Stegen et al., 2015; Stegen et al., 2012), have been developed to investigate these assembly processes. These tools provide an opportunity to achieve a comprehensive understanding of the mechanisms driving microbial communities at a process level. Additionally, the noteworthy point is that the majority of microorganisms do not live as separate individuals but form intricate co-occurrence networks through direct or indirect interactions (Ji et al., 2021; Yuan et al., 2022). These interactions have significant impacts on ecological assembly processes (Liu et al., 2023; Wu et al., 2024). Co-occurrence network analysis has proven to be a powerful tool for elucidating species interactions and can offer supplementary insights into microbial community assembly that cannot be obtained through other analytical methods (Fuhrman et al., 2006; Li et al., 2023). In the past few years, there has been considerable focus on investigating the microbial community assembly mechanism and conducting ecological network analysis in diverse lentic ecosystems such as oceans (Yu X. et al., 2023), soils/sediments (Li et al., 2023; Zhao Z. et al., 2022), wetlands (Wang B. et al., 2020), and lakes (Jiao et al., 2020). Compared to these lentic ecosystems, subtropical riverine ecosystems exhibit more pronounced seasonal variations in hydro-chemical conditions and bacterial communities (Sun H. et al., 2021). However, our understanding of how seasonality influences the process governing microbial community assembly in subtropical river ecosystems remains limited. Therefore, studying the seasonal dynamics of co-occurrence networks and ecological processes within bacterial communities in subtropical rivers is a crucial step toward comprehensively understanding riverine microbial ecology and its profound impacts on ecosystem functioning.
The Liuxi River, a major tributary of the Pearl River in the subtropical monsoon region, flows through Guangzhou, one of the fastest-growing regions in the Guangdong-Hongkong-Macao Greater Bay Area (GBA) of China. It holds significant social and ecological importance as it provides water for domestic, agricultural, industrial, and landscape use in Guangzhou. However, rapid urbanization processes witnessed in the past three decades have resulted in severe deterioration in water quality (Xie et al., 2020; Zhu et al., 2020), thereby exacerbating the riverine ecosystem vulnerability and diminishing its ecosystem services value (Ji et al., 2015; Zhou et al., 2018). Moreover, the river exhibits highly dynamic environmental conditions that vary between seasons (Yang et al., 2020). Nevertheless, to the best of our knowledge, the ecological processes shaping bacterial communities and how environmental changes affect microbial community assembly within the Liuxi River have not been understood yet. Environmental variations can influence bacterial communities and their assembly mechanisms by regulating deterministic and stochastic processes (Fang et al., 2023; Obieze et al., 2022). We hypothesize that seasonal variations in environmental conditions (e.g., temperature, pH, dissolved oxygen, organic matter, and nutrients) may exert substantial influence on bacterial communities of the Liuxi River as well as their assembly processes and interspecific interactions. Therefore, the bacterial communities of water samples collected from the Liuxi River were evaluated by 16S rRNA high-throughput sequencing. The objectives of this study are to: (1) investigate seasonal patterns of microbial community structure and diversity, (2) characterize the species interactions and topological properties of bacterial co-occurrence networks, and (3) identify the dominant ecological processes governing microbial community assembly. This study will be essential for understanding the response of bacterial communities to seasonal variations and provide a crucial theoretical foundation for enhancing functionality and promoting the sustainable development of river ecosystems.
2 Materials and methods
2.1 Site description and sample collection
The Liuxi River is located in Guangzhou city of GBA (Figure 1), with a length of 171 km and a basin area of 2,300 km2 (Zhu et al., 2020). The river basin is located in the subtropical monsoon climate zone, and its average annual temperature and precipitation are 20.3°C and 2,143.8 mm, respectively (Zhu et al., 2020). Due to the effect of the East Asian monsoonal circulation, approximately 80% of the annual precipitation falls during the wet season from April to September (Yang et al., 2020). Since the 1960s, 5 reservoirs and 8 diversion projects have been conducted within this basin, which affects the water levels and hydrological connectivity of the Liuxi River (Yang et al., 2020; Zhu et al., 2020).
2.2 Sample collection and analysis
2.2.1 Water sample collection
Thirty surface water samples (R1–R15, Figure 1 and Supplementary Table S1) were collected from the Liuxi River during June 2020 (wet season, WS) and January 2021 (dry season, DS), respectively. Dissolved oxygen (DO), electrical conductivity (EC), oxidation-reduction potential (ORP) and temperature (T) were measured in situ using Hach portable meters (HQ40d). The sampling campaigns took place within 2 days for both seasons, with no occurrence of rain events. After collection, the water samples, each with a volume of 1 L, were filtered through 0.22 mm-pore-size polycarbonate filter membranes (Millipore, Billerica, United States). The membranes were then placed into petri dishes and immediately stored at −40°C for DNA extractions. The filtered samples were stored in a refrigerator at 4°C upon arrival at the laboratory. All experimental materials utilized for microbial analysis were sterilized in an autoclave prior to the collection of water samples.
2.2.2 Analysis of environmental factors
The concentrations of ammonium-nitrogen (NH4+) were determined in situ using spectrophotometry (HI96733, HANNA, Italy). Major anions (Cl−, SO42−, and NO3−) and the dissolved organic carbon (DOC) were analyzed by ion chromatography (ICS-900, Dionex, United States) and the TOC analyzer (Vario TOC; Elementar, Germany), respectively.
2.2.3 DNA extraction and high-throughput sequencing
The MoBio PowerSoil DNA Isolation Kit (MoBio Laboratories, Carlsbad, CA, United States) was utilized for the extraction of total DNA from water samples following the manufacturer’s guidelines. The concentration of each DNA sample was quantified using a Qubit® 2.0 fluorometer (Invitrogen, United States) and Nanodrop 2000 spectrophotometer (Thermo, Waltham, MA, United States), and quality assessment was conducted through 1% agarose gel electrophoresis (Zhu et al., 2020). Amplification of the V3–V4 region of bacterial 16S rRNA was performed utilizing the universal primer set 515F (5′-GTGCCAGCMGCCGCGGTAA-3′) and 806R (5′-GGACTACHVGGGTWTCTAAT-3′). The PCR mixture (25 μL) contained 10 ng template DNA, 12.5 μL of Phusion High-Fidelity PCR Master Mix with GC buffer (New England Biolabs, Beverly, MA, United States), and 0.5 μL of forward and reverse primers. The PCR reaction conditions were as follows: 94°C for 5 min, followed by 30 cycles of 94°C for 60 s, 57°C for 45 s, 72°C for 60 s, and a final extension at 72°C for 5 min (Jiang et al., 2018). Each sample was amplified in triplicate. Subsequently, PCR amplicons were sequenced on the Illumina MiSeq platform according to standard protocols at Beijing Biomarker Technologies Co., Ltd. (Beijing, China). The raw sequence data were deposited into the National Center for Biotechnology Information (NCBI) database with the accession number PRJNA1131166.
2.2.4 Bioinformatics analysis
The bioinformatics analysis was carried out on the online platform of Biomarker Cloud Platform.1 Briefly, the paired-end reads obtained from Miseq sequencing were initially spliced according to their overlap relationships. To obtain high-quality clean reads, the Trimmomatic and UCHIME software were utilized with default settings for raw read analysis to extract effective tags. Subsequently, the UPARSE pipeline was used for taxonomic assignment at the 97% similarity level via the Ribosomal Database Project Naïve Bayesian Classifier v.2.2 trained on the SILVA database (Edgar, 2013; Zhu et al., 2020). Alpha diversity indices (Chao 1 and Shannon) were generated using the QIIME2.2
2.2.5 Microbial community assembly analysis
The Sloan neutral community model (NCM) was utilized to predict the occurrence frequency and relative abundance of operational taxonomic units (OTUs), aiming to investigate the potential impact of stochastic processes on bacterial community assembly (Sloan et al., 2010; Zou et al., 2023). Model fitting, including the estimation of the goodness of fit (R2) and migration rate (m), was conducted using R packages “Hmisc,” “minpack.lm,” and “stats4” (Nyirabuhoro et al., 2020; Yu C. et al., 2023). Additionally, a community-level null model analysis was applied to quantify the relative contributions of stochasticity and determinism in microbial community assembly processes (Chen et al., 2022; Stegen et al., 2013). The null model analysis employed two indices: the beta nearest taxon index (βNTI) and the Bray-Curtis-based Raup-Click (RCbray). These indices were calculated using “picante” and “ecodist” packages in R software (Stegen et al., 2012). A value of |βNTI| >2 indicates that deterministic processes dominate the community assembly process, encompassing homogeneous selection (βNTI <−2) and heterogeneous selection (βNTI >2). Otherwise, stochastic processes are considered as controlling factors for community assembly. Additionally, when RCbray >0.95, it indicates dispersal limitation, RCbray <−0.95 represents homogenizing dispersal; while −0.95 < RCbray < 0.95 suggests undominated stochastic processes (Liu et al., 2021). To assess potential drivers influencing compositional turnover in microbial communities, we examined correlations between pairwise βNTI values and changes in all measured environmental variables. The statistical significance of these relationships was further tested using a permutation-based partial Mantel test with 1,000 permutations (Gao et al., 2021).
2.2.6 Ecological network analysis
The Molecular Ecological Network Analysis (MENA; http://ieg4.rccc.ou.edu/mena/) based on the random matrix theory (RMT) was performed to evaluate the primary connections among bacterial communities (Deng et al., 2012; Liu et al., 2022; Sha et al., 2023). Co-occurrence networks were constructed using Spearman correlation analysis with a significance threshold of <0.05. To ensure comparability across different seasons, a consistent similarity threshold (St = 0.78) was applied during the construction of networks. The MENA pipeline was utilized to calculate the topological properties of these networks, while the Gephi 0.9.2 software was employed for their visualization. The nodes within networks are categorized into four groups based on their values of intra-module connectivity (Zi) and inter-module connectivity (Pi): peripherals (Zi ≤ 2.5, Pi ≤ 0.62), connectors (Zi ≤ 2.5, Pi > 0.62), module hubs (Zi > 2.5, Pi ≤ 0.62) and network hubs (Zi > 2.5, Pi > 0.62). Except for peripherals, the other three types of nodes are commonly regarded as keystone species (Deng et al., 2012; Shuwang et al., 2023).
2.2.7 Statistical analysis
The alpha diversity indexes (Chao1 and Shannon) and the beta diversity index (Bray–Curtis) were calculated using the “vegan” package in R software. The differentiation of these indices between seasons was tested using the Kruskal–Wallis method (Yang et al., 2023). Analysis of variance (ANOVA) was employed to assess differences in environmental factors across seasons. Principal coordinate analysis (PCoA) based on the Bray–Curtis distance was performed to investigate the dissimilarities in bacterial community structures between the two seasons (Fang et al., 2023). Similarity analysis (ANOSIM) was conducted to explore the statistical significance of variations in bacterial communities between groups. Bacteria with significantly different abundances in different seasons were identified by STAMP, utilizing a Wilcox rank-sum test with an adjusted false discovery rate (FDR) set at 0.05 (Lv et al., 2022; Sha et al., 2023). The Redundancy analysis (RDA) and Mantel test were applied to examine relationships between environmental factors and bacterial community structure. To eliminate multicollinearity among environmental factors, the variance inflation factor (VIF) was computed and a threshold of 10 was used for VIF to identify redundant variables (Yu C. et al., 2023). These statistical analyses were conducted using R software with packages including “stats,” “vegan,” “rdacca.hp,” and “LinkET” (Lai et al., 2022).
3 Results
3.1 Physicochemical properties
The physicochemical factors are presented in Supplementary Figure S1. Except for SO42−, all other environmental factors exhibited statistically significant differences between the WS and DS (p < 0.05). Overall, pH, T, NH4+, and DOC displayed significantly higher mean values in the WS compared to the DS, while EC, DO, NO3−, Cl−, HCO3−, and ORP demonstrated opposite trends. Notably, ammonia nitrogen pollution was severe in WS, as NH4+ concentrations exceeded the class III national surface water quality standard of China (GB 3838-2002) in 100 and 53.5% of the samples during the WS and DS, respectively.
3.2 The distribution of diversity, composition and function profiles of bacterial communities
The global rarefaction curves of water samples (Supplementary Figure S2) collected from the Liuxi River in the WS and DS approached saturation, indicating that the sequencing depth was sufficient for subsequent analyses. After quality control, a total of 1,994,476 high-quality reads were obtained and clustered into 14,060 operational taxonomic units (OTUs) at a similarity level of 97% in 30 water samples. The Chao1 index in the DS (600.6 ± 75.8) was significantly higher than that in the WS (443.2 ± 62.4) (p < 0.05, Figure 2A), while the Shannon index also exhibited a higher value in the DS although the difference did not reach statistical significance (Figure 2B). Overall, there was greater alpha-diversity observed within the bacterial community in DS compared to WS.
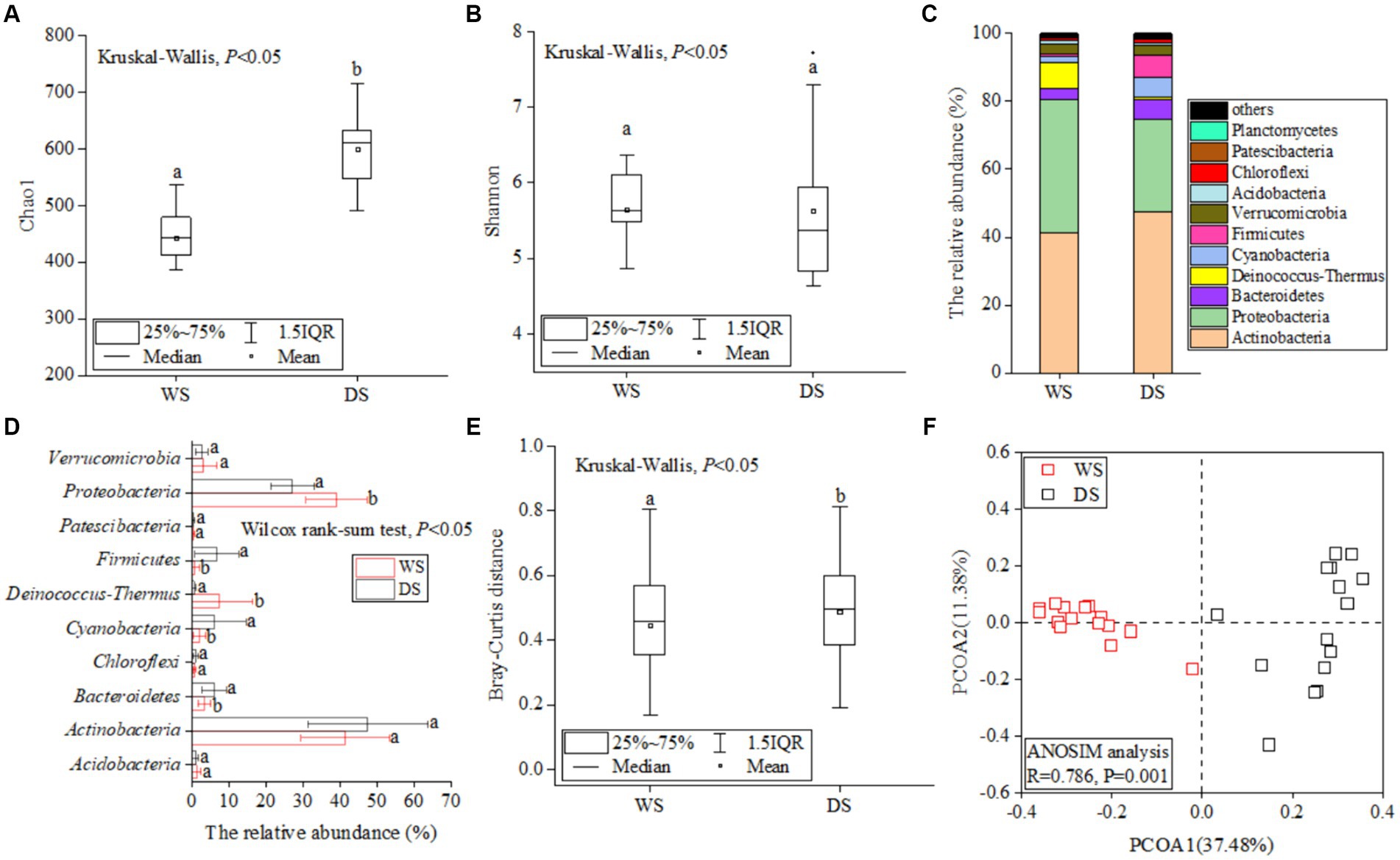
Figure 2. The seasonal distribution patterns of bacterial communities. The differences of Chao1 (A) and Shannon (B) index between the WS and DS. The relative abundance of bacterial communities at the phylum level (C). Comparative analysis of the dominant bacterial taxa (D) across different seasons, significant differences (p < 0.05) between the WS and DS are indicated by letters above the bars. The difference in Bray–Curtis distance between the WS and DS (E). Bray Curtis-based PCoA of bacterial community structures between the WS and DS (F).
The OTUs were assigned to 24 phyla, 61 classes, 143 orders, 250 families, and 461 genera. At the phylum level, Actinobacteria (44.5 ± 14.3%) was dominant, followed by Proteobacteria (33.1 ± 9.3%) and Bacteroidetes (4.6 ± 2.9%); these top three phyla accounted for a total of 82.3% of all OTUs (Figure 2C; Supplementary Figure S3). Comparative analysis using STAMP revealed significantly higher relative abundances of Proteobacteria and Deinococcus-Thermus in the WS compared to the DS (Figure 2D, p < 0.05), while Bacteroidetes and Firmicutes exhibited significantly higher relative abundance in the DS (Figure 2D, p < 0.05). Bray-Curtis distances reflecting community similarity were lower in the DS than in the WS (Figure 2E), suggesting a more similar community structure in the WS. Bray Curtis-based PCoA was conducted to display the beta diversity patterns of bacterial communities across different seasons (Figure 2F). The two principal coordinate axes explained >40% of the total variations in bacterial community composition. Samples from the WS and DS were partitioned into two clusters, and the ANOSIM analysis further confirmed significant dissimilarity in the bacterial community structures between the WS and DS (R = 0.786, p = 0.001).
3.3 Relationships between environmental factors and bacterial community structure
The impact of environmental factors on bacterial communities was assessed using the RDA and Mantel test (Supplementary Figures S4A–C). To eliminate collinearity among factors, we calculated the VIF for each environmental factor before analysis. Ultimately, seven factors including pH, T, DO, NO3−, NH4+, DOC, and ORP were selected for these analyses (Supplementary Table S2). The RDA reveals significant associations between variations in bacterial community composition (BCC) and pH, T, DO, NO3−, NH4+, and DOC. These environmental factors accounted for 53.9% of the variation in BCC, as shown in Supplementary Figure S4A. Importantly, T emerged as the most crucial variable in explaining seasonal variations of BCC, which contributed to the largest variation at 16.6% (Supplementary Figure S4B). The Mantel test demonstrate significant correlations between BCC with pH and T (p < 0.01, Supplementary Figure S4C). Furthermore, the Chao1 index exhibited significant negative relationships with pH and T (p < 0.001, Supplementary Figure S4C), while showing significant positive correlations with DO (p < 0.01, Supplementary Figure S4C), NO3− (p < 0.05, Supplementary Figure S4C), and ORP (p < 0.01, Supplementary Figure S4C).
3.4 Ecological assembly processes controlling bacterial communities
The NCM successfully estimated a large fraction of associations between the occurrence frequency of OTUs and their relative abundances, explaining 63.5 and 71.8% of community variance in the WS and DS, respectively (Figures 3A,B). These findings suggest that stochastic processes played a crucial role in shaping bacterial community assembly, particularly in the DS. However, since the neutral model failed to explain all variations in the community, deterministic processes also contribute to community assembly. Additionally, there was a noticeably lower migration rate observed in the DS compared to the WS (m = 0.026 vs. m = 0.105).
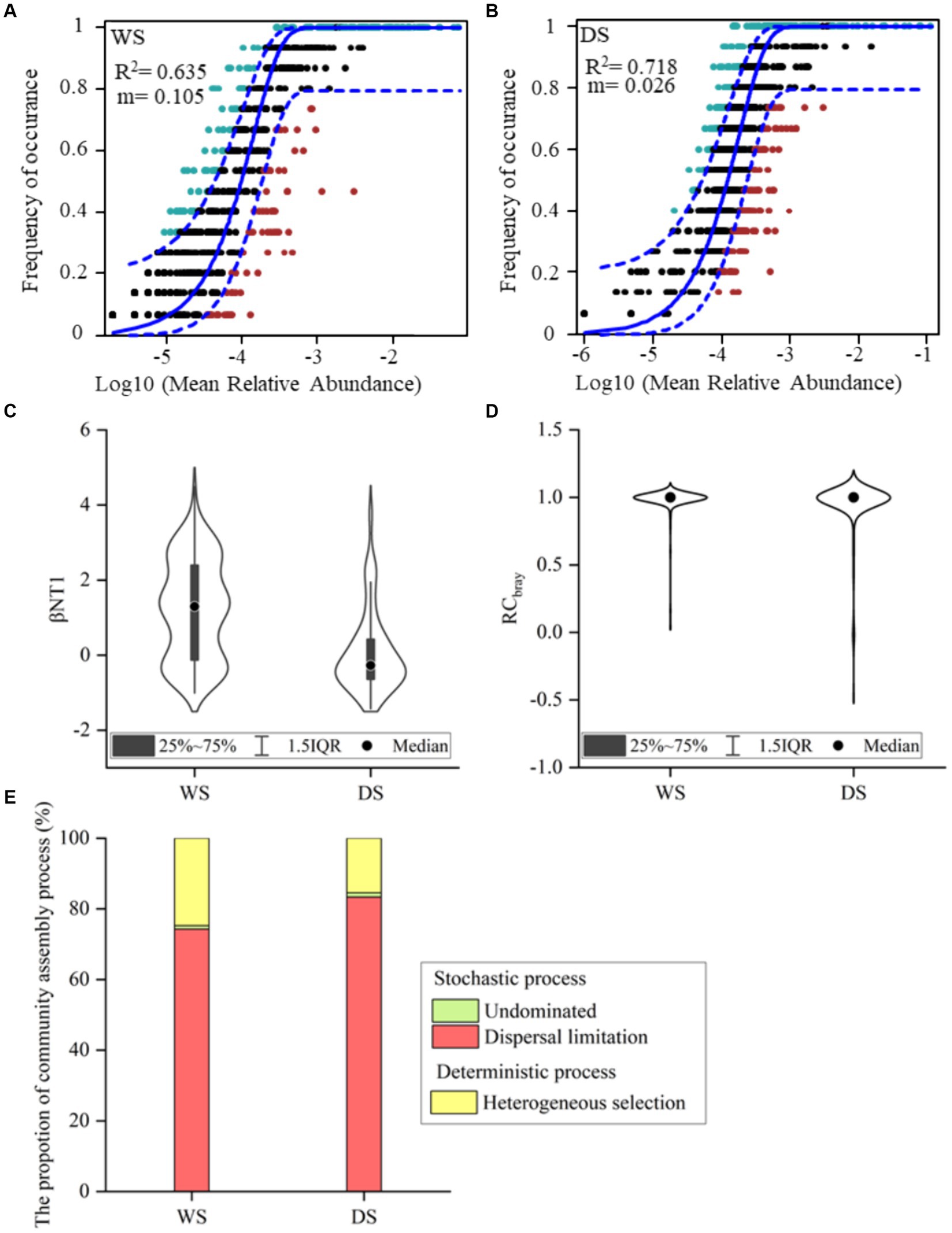
Figure 3. The bacterial community assembly processes. The contribution of stochastic processes to the assembly of bacterial community explained by NCM (A,B). R2 represents the fit to the neutral model and m indicates the immigration rate of metacommunity. The points falling above and below the 95% confidence interval are colored green and red, respectively, and those within the interval are colored black. The quantification of bacterial community assembly driven by different ecological processes (C–E). βNTI (C) and RC bray (D) of bacterial communities between different seasons. The percentage contribution of individual processes to community assembly (E).
The null model analysis was conducted to quantify the relative contributions of stochastic and deterministic processes to community assembly (Figures 3C–E). As illustrated in Figure 3C, the βNTI values of bacterial communities were primarily concentrated within the range of −2 to 2, further confirming that stochastic processes predominantly governed the assembly of bacterial communities in both seasons. However, the relative contributions of stochastic and deterministic processes differed between seasons (Figure 3E). During the WS, stochastic processes accounted for 75.2% of the community variance, with dispersal limitation contributing to 74.3% and undominated processes accounting for only 0.95%. Deterministic processes solely included heterogeneous selection which explained 24.8% of the community variance. During the DS, stochastic processes explained 84.5% of the community variance, with dispersal limitation and undominated processes accounting for 83.3 and 1.21%, respectively, while deterministic processes (solely heterogeneity selection) explained only 15.5% of the community variance.
To gain a more comprehensive understanding of bacterial community assembly, we conducted a linear regression analysis to explore the relationships between changes in environmental factors and βNTI values among samples (Supplementary Figure S5). The findings revealed significant negative correlations between differences in NO3− (R = −0.377, p = 0.000) and DOC (R = −0.233, p = 0.001) with βNTI. The impact of deterministic processes decreased as levels of NO3− and DOC increased. Specifically, the contribution of deterministic processes became negligible when ΔNO3− exceeded 1.94 mg/L and ΔDOC surpassed 10.1 mg/L. Notably, ΔNO3− had the most pronounced influence on the community assembly.
3.5 Co-occurrence network of bacterial communities
The bacterial co-occurrence networks were constructed for the WS and DS (Figures 4A,B), and their topological parameters are presented in Table 1. It was observed that both networks followed a power-law distribution pattern, with R2 values of 0.795 in the WS and 0.882 in the DS, respectively (Table 1). This indicates that the networks had scale-free and non-random structures. Moreover, both networks demonstrated modular properties as evidenced by modularity values of 0.426 in the WS and 0.690 in the DS (>0.4). Additionally, the networks exhibited predominantly positive correlations among nodes, accounting for approximately 92.9% in the WS network and 64.9% in the DS network.
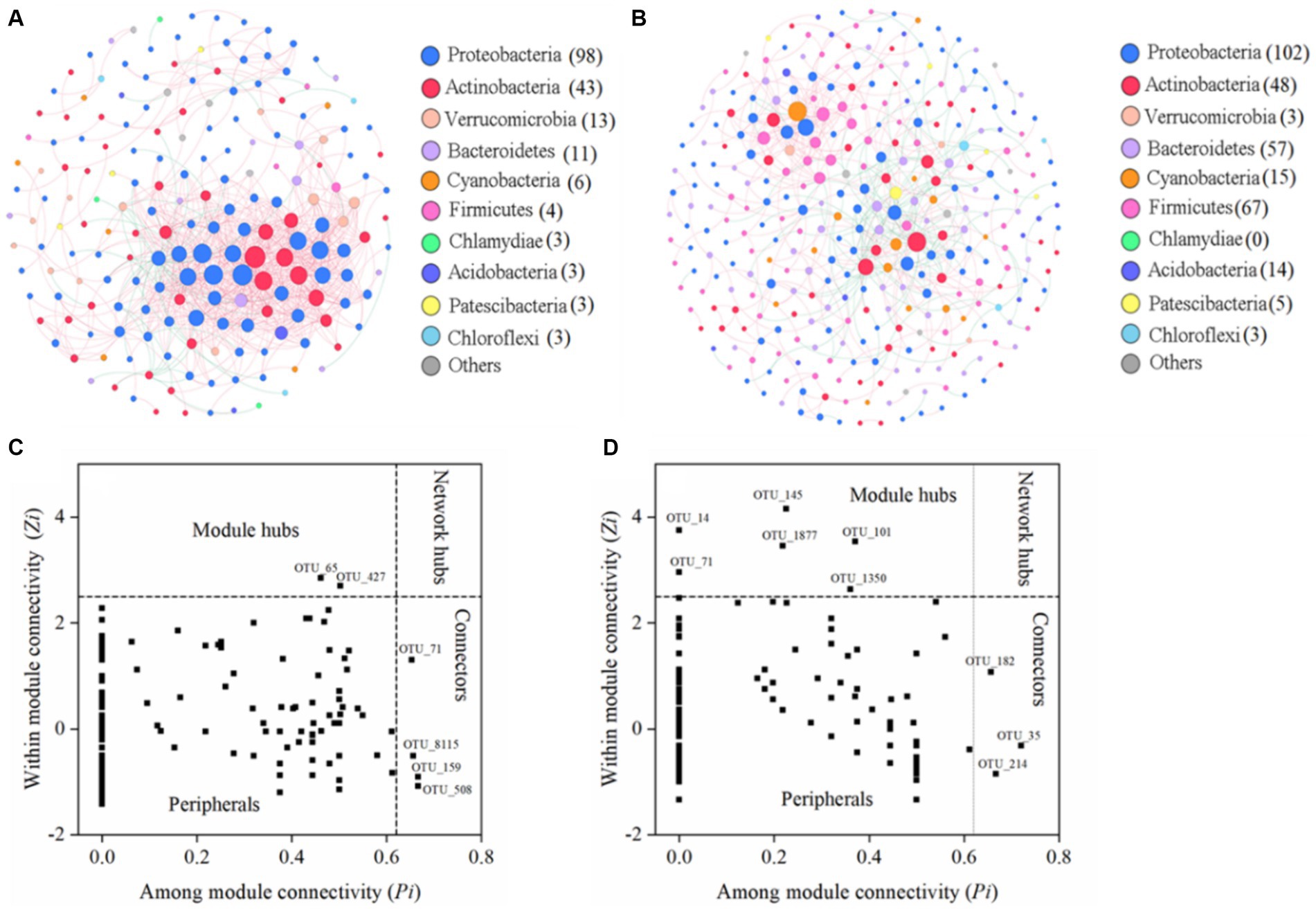
Figure 4. The seasonal co-occurrence patterns of bacterial communities. Network analysis showing the bacterial community interspecies interactions in the WS (A) and DS (B). Each node presents one OTU, and the size of node presents the number of connections. Bacteria at the phylum level are colored by different color nodes. The different colors of the edge represent the positive (pink) and negative (green) correlations of species. Identification of keystone taxa in the WS (C) and DS (D) by their topological characteristics in networks.
Furthermore, multiple network topological metrics revealed that the co-occurrence patterns differed profoundly between these two seasons (Table 1). In comparison to the DS network, the WS network exhibited a lower GD value (3.75 vs. 5.42), higher average degree (avgK, 8.47 vs. 3.41), and average clustering coefficient (avgCC, 0.303 vs. 0.117), suggesting stronger connections within bacterial communities during the WS. The higher avgCC in the WS further suggests that there were more links within clusters than between clusters. Apart from variations in the network topological indices, distinct differences were observed in terms of network compositions across seasons. The bacterial community network during the WS consisted of 192 nodes and 813 edges, while during the DS, it was comprised of 321 nodes and 548 edges. The nodes in the WS network were primarily composed of Proteobacteria, Actinobacteria, and Verrucomicrobia, while in the DS network, they mainly belonged to Proteobacteria, Firmicutes, Actinobacteria, Bacteroidetes, Cyanobacteria, and Acidobacteria. Additionally, seasonal variations were also observed regarding positive and negative relationships among microorganisms. For instance, Actinobacteria was positively correlated with Proteobacteria and Bacteroidetes in the WS, but negatively correlated with Proteobacteria and Bacteroidetes in the DS (Figures 4A,B).
The identification of keystone species in the networks was based on the Zi and Pi values (Figures 4C,D). While the majority of nodes (>90%) were classified as peripherals, there were also connectors and module hubs present in both networks that met the criteria for being considered as keystone species. As shown in Supplementary Table S3, the number and composition of keystone taxa exhibited distinct seasonal variations. During the WS, two module hubs and four connectors belonging to Proteobacteria and Actinobacteria were identified as keystone species, whereas six module hubs and three connectors belonging to Actinobacteria, Cyanobacteria, Proteobacteria, and Bacteroidetes were identified during the DS.
4 Discussion
4.1 Seasonal variations in taxonomic and functional community profile of bacteria
In this study, Proteobacteria, Actinobacteria, and Bacteroidetes were identified as the dominant phyla in the Liuxi River (Figure 2C), which aligns with previous reports of their widespread presence in various riverine habitats (Liu et al., 2021; Zhang et al., 2020; Zou et al., 2023). However, significant differences in relative abundance were observed for Proteobacteria, Deinococcus-Thermus, Bacteriodetes, and Firmicutes between seasons (Figure 2). This finding can be attributed to variations in bacterial adaptability to different environment factors such as pH, T, EC, and nutrient concentrations (Xu et al., 2020; Yan et al., 2023). For instance, many members of Proteobacteria have been reported as potential nitrifiers and tend to thrive in high ammonia environments (Fan et al., 2020; Wang J. et al., 2024). Deinococcus-Thermus belongs to aerobic or facultative anaerobic bacteria primarily found in neutral to alkaline pH with high temperature water bodies (Qing et al., 2023; Sharma et al., 2020). Bacteriodetes and Firmicutes are commonly enriched in high EC and chloride environments due to their high salt tolerance levels (Miao et al., 2015; Yang et al., 2021; Zhao et al., 2020). The Bray Curtis-based PCoA manifested that bacterial communities from the same season clustered together (Figure 2D), indicating distinct community compositions between the WS and DS. Several reasons may account for this phenomenon. Firstly, the results of RDA and Mantel test indicate that T, pH, DO, NO3−, NH4+, and DOC exerted significant effects on bacterial community structures (Supplementary Figure S4). These environmental variables showed substantial variations between the two seasons as depicted in Supplementary Figure S1. Therefore, the pronounced seasonal fluctuations in environmental conditions could be responsible for the differentiation of community structures across seasons. Secondly, in the Liuxi River, rainfall predominantly occurs during the WS, resulting in elevated water levels and increased river flows during this period (Chen et al., 2019; Zhu et al., 2020). The intensified precipitation and river flow during the WS can enhance bacterial diffusion and dilution processes, thereby altering the community composition (Huang et al., 2023b). Thirdly, the seasonal succession of bacterial communities may be driven by species interactions (Chen et al., 2019; Huang et al., 2023b; Yang et al., 2023), as stochastic processes were found to dominate the bacterial community assembly within the river. Moreover, Bray-Curtis distances indicated significantly lower dissimilarity among microbial communities during the WS compared to the DS periods (Figure 2E). This observation can plausibly be explained by the increased hydrological connectivity and dispersal rates facilitated by higher river flow during the WS (Isabwe et al., 2018; Wang et al., 2015); high dispersal rates may contribute to greater homogenization within the microbial community (Pan et al., 2022; Zhang et al., 2022).
Apart from the evident variations in bacterial communities between the two seasons, we also observed the seasonal change in alpha diversity, with a higher level of alpha diversity in the DS, which aligns consistently with previous research findings (Lu L. et al., 2022; Ren et al., 2019; Zhou et al., 2021). The more stable hydraulic characteristics in the DS, compared to the fluctuating aquatic conditions caused by frequent rainfall in the WS, potentially contribute to a favorable environment for microbial reproduction and growth (Lu L. et al., 2022; Zhao et al., 2021). Furthermore, several studies have reported that high concentrations of ammonia nitrogen can inhibit bacteria survival and reduce bacterial diversity (Do et al., 2021; Ning et al., 2023; Wang J. et al., 2024). Therefore, the excessive presence of ammonia nitrogen in the WS may lead to a decrease in microbial diversity. Additionally, during the WS, the mean temperature of river water exceeded 30°C (Supplementary Figure S1), which could exclude species vulnerable to temperature and subsequently decrease microbial diversity (Chen et al., 2022). A previous study has indicated that a neutral pH plays a crucial role in promoting microbial proliferation in aquatic environments (Ji et al., 2022). Therefore, the increase in microbial diversity can also be attributed to the observed neutral pH values during the DS. This notion aligns with our results that the Chao1 index exhibited significantly negative correlations with pH and T (Figure 3C), noting that both pH and T were significantly higher during the WS. However, our findings contradict those of Zeng et al. (2019), who reported higher alpha diversity among bacterial communities during the WS compared to the DS. This discrepancy may arise due to different environmental conditions and hydrological characteristics across different regions (Fang et al., 2023; Wang et al., 2023; Zeng et al., 2019).
4.2 Stochastic processes governing the bacterial community assembly
The disentanglement of mechanisms shaping bacterial community assembly is a fundamental prerequisite for elucidating the response of bacterial communities to seasonal environmental changes and has always been a central focus of microbial ecology (Fang et al., 2023; Jiao et al., 2020; Yang et al., 2023). In the study, we employed the NCM and null model to evaluate and quantify the ecological processes. Consistent results obtained from the NCM and null model indicate that stochastic processes predominantly governed bacterial community assembly in both seasons (Figures 3A–E). Our findings were in line with previous studies conducted in river ecosystems. For example, Sun C. et al. (2021) and Sun H. et al. (2021) revealed that distinct stochastic processes dominated the bacterial community structure in the Han River, China. Additionally, Chen et al. (2019) reported that stochastic processes accounted for approximately 85% of variations in microbial communities within the Tingjiang River (China). The null model further revealed that dispersal limitation was the primary ecological control process among stochastic processes in both seasons (Figure 3E). Dispersal limitation refers to the restricted movement and colonization behaviors of individuals in new locations (Qin et al., 2022). It’s well known that microbial communities are dispersed actively or passively by river flow, unable to counteract its unidirectional movement (Chen et al., 2019). The construction of numerous reservoirs and water diversion projects along the Liuxi River since the 1960s has resulted in hydrologically isolated flow paths, which may contribute significantly to the observed substantial dispersal limitation (Dini-Andreote et al., 2015; He et al., 2021). The dominant role of dispersal limitation in controlling microbial assembly has also been documented by Yang et al. (2023). Furthermore, the null model indicated that dispersal limitation played a more influential role in shaping bacterial communities during the DS (Figure 4E). The value of m calculated by the NCM is interpreted as a measure of dispersal limitation, indicating higher levels when bacterial communities are less affected by dispersal limitation. The significantly lower m value in the DS further confirms that the impact of dispersal limitation on bacterial communities was more pronounced during this period. This phenomenon can be attributed to variable rates at which microbial communities disperse due to hydrological changes (Milke et al., 2022; Palmer and Ruhi, 2019). During the WS, abundant precipitation and river discharge enhanced the hydrological connectivity, facilitating easier dispersion and long-distance movement of microbes (Lu L. et al., 2022; Sun H. et al., 2021; Yang et al., 2023). Conversely, reduced hydrological connectivity and runoff in the DS hindered the dispersion of bacterial communities (Huang et al., 2023a; Huber et al., 2020).
Furthermore, deterministic processes also partially explained turnover in community compositions, with a higher contribution observed during the WS compared to the DS. It has been well established that environmental heterogeneity greatly influences the relative contribution of deterministic and stochastic processes (Dini-Andreote et al., 2015; Liu et al., 2023; Shuwang et al., 2023; Wu et al., 2024). In our study, the linear regression analysis on changes in environmental factors and βNTI values demonstrates that NO3− played a prominent role in driving microbial community assembly, with community determinism decreasing as NO3− concentrations increased (Supplementary Figure S5). As an important nitrogen source for microbial growth, NO3− directly or indirectly affects metabolisms and growth of microbes (Han et al., 2020; Kutvonen et al., 2015), making it a major factor mediating the balance between stochastic and deterministic assembly processes. Therefore, the observed lower contribution of deterministic processes could be ascribed to the notably higher level of NO3− in the DS. Collectively, these findings highlight the linkages of hydrological conditions and environmental variables with community assembly processes that may further influence the diversity, composition, and function stability of bacteria.
4.3 Divergent patterns in species interactions between seasons
Species interactions have significant impacts on the structure and diversity of microbial communities (Ji et al., 2021; Lu M. et al., 2022; Zhang et al., 2021). Co-occurrence network analysis facilitates a comprehensive understanding of potential ecological relationships among microorganisms (Du et al., 2023; Huang et al., 2023b). In microbial co-occurrence networks, positive and negative correlations signify cooperative and competitive relationships among microbial species (Li et al., 2023; Yu C. et al., 2023). The network analysis conducted in this study revealed that mutualism or commensalism played a pivotal role in shaping the bacterial community structure, as the proportion of positive correlations exceeded 60% in both networks. From the perspective of the stress gradient hypothesis, cooperative relationships between microbes can act as buffers against environmental stress, thereby enhancing their resilience in harsh environments (Li et al., 2022; Wu et al., 2024; Zelezniak et al., 2015). The Liuxi River was contaminated with ammonia nitrogen, this “harsh” environment likely led to the dominance of cooperative correlations between microbes during both seasons. Synergistic or symbiotic relationships among different microorganisms may have contributed to mitigating the toxicity associated with ammonia nitrogen pollution. It is noteworthy that compared to the DS network, the WS network exhibited a higher proportion of positive correlations due to more severe ammonia pollution, elevated temperature, and unstable hydrological conditions, which made it a more challenging environment for microbes in the WS. Previous studies have suggested that greater microbial diversity promotes species interactions and enhances the connectivity within co-occurrence networks (Fang et al., 2023; Shuwang et al., 2023; Sun H. et al., 2021). However, our findings indicate that although high bacterial community diversity was observed in the DS, species interactions were not as strong as those observed in the WS (Figure 4 and Table 1). One possible explanation is that significantly lower DOC concentrations restricted microbial utilization of carbon resources leading to reduced interactions among microorganisms when faced with limited available carbon resources. The results were consistent with previous studies, which suggested that the availability of environmental resources could have a significant impact on network connectivity (Dai et al., 2022; Huang et al., 2023b; Lu M. et al., 2022). Furthermore, compared to the DS network, the WS network exhibited a lower level of modularity. In microbial ecology, modules can be interpreted as niches, and a higher modularity value indicates a stronger niche differentiation (She et al., 2022; Yan et al., 2023). Therefore, the decreased modularity in the WS suggests that microorganisms share similar niches and interact more frequently. This reduced modularity could be attributed to the lower dissimilarity in the WS, which induces increased microbial co-occurrence patterns and diminished modularity (Widder et al., 2014; Yang et al., 2023). Additionally, the higher modularity observed in the DS implies a more stable and ordered structure of bacterial communities (Ji et al., 2021; Yan et al., 2023). Previous studies have demonstrated that negative associations (competition) within communities can stabilize community co-oscillation and enhance network stability (Coyte et al., 2015; Li et al., 2023; Wagg et al., 2019). Our findings demonstrate that the DS network exhibited a higher proportion of negative correlations than the WS network, providing further evidence for its greater stability. The disparities in bacterial community stability are likely to be associated with the elevated dispersal rates during the WS, as these rates can result in frequent immigration and emigration of microorganisms, disrupting established interaction relationships and consequently reducing ecological network stability (Lu M. et al., 2022; Widder et al., 2014; Yu X. et al., 2023). Moreover, it has been reported that an increased number of keystone species contributes to enhanced stability and orderliness in microbial networks (Jiao et al., 2020; She et al., 2021). Therefore, our identification of more keystone taxa in the DS may also contribute to constructing a more robust network architecture.
Keystone species play essential roles in driving the structure and function of microbial communities (Banerjee et al., 2018; Ji et al., 2021), as their absence can lead to the disintegration of modules or even networks (Wu et al., 2024; Zhao et al., 2016). In our study, we observed that all keystone species in these two ecological networks exhibited remarkably low abundance levels (Supplementary Table S3). This finding aligns with previous studies highlighting the crucial roles of rare taxa in maintaining the stability of microbial interaction networks (Sun C. et al., 2021; Wu et al., 2024; Yan et al., 2023). Notably, there were seasonal shifts in the compositions of keystone taxa in the river. In the WS, Proteobacteria and Actinobacteria were identified as keystone species, while the DS included Proteobacteria, Actinobacteria, Cyanobacteria, and Bacteroidetes as keystone taxa (Supplementary Table S3). Proteobacteria and Actinobacteria were found to be the most abundant keystone species in both networks. Previous research has emphasized their significant contributions to organic matter degradation and nitrogen cycling processes (Chen et al., 2022; Gao et al., 2021; Ruprecht et al., 2021; Spain et al., 2009). Moreover, these phyla are widely distributed in environments impacted by anthropogenic activities due to their high metabolic diversities and ability to withstand environmental stress effectively (Ohore et al., 2023; Wu et al., 2022; Zhong et al., 2023). Interestingly, Cyanobacteria and Bacteroidetes were exclusively present as keystone taxa in the DS possibly due to the presence of water hyacinth during this period. It is well-known that Cyanobacteria are associated with cyanobacteria blooms in rivers and lakes (Ohore et al., 2023), while Bacteroidetes can effectively acquire organics from algae, and their distribution directly correlates with the presence of algal within aquatic environments (Fang et al., 2023; Shen et al., 2020). Additionally, Cyanobacteria and Bacteroidetes actively participate in biogeochemical carbon and nitrogen cycling processes (Xue et al., 2022; Zhong et al., 2023). Overall, these keystone taxa perform an indispensable role in carbon and nitrogen cycles and hold ecological significance for regulating community structure and maintaining the ecosystem stability of the Liuxi River. Furthermore, Bacteroidetes are normally found in human/animal intestines, suggesting that their appearance may be related to domestic wastewater discharge (Wang et al., 2018). Therefore, the exclusive presence of Bacteroidetes as keystone taxa in the DS suggests that the river was more susceptible to anthropogenic impacts during this period. Previous studies have reported that water bodies contaminated by anthropogenic activities such as domestic sewage discharge exhibit elevated levels of EC and Cl− (Chen et al., 2014; Zhu et al., 2019). Consistent with this, significantly higher concentrations of EC and Cl− were observed during the DS compared to the WS, further indicating the pronounced impact of human activities on the river. At the genus level, more than half of the identified genera, including uncultured_bacterium_f_Burkholderiaceae, Enterobacter, Acinetobacter, Actinomadura, and uncultured_bacterium_f_Methylophilaceae are known pathogens associated with human disease (Gao et al., 2017; Zhou et al., 2020). These findings suggest that Liuxi River may provide niches for pathogen growth and dissemination that pose a threat to public health. Therefore, it’s crucial to conduct further investigations into bacterial composition within Liuxi River.
5 Conclusion
The composition, co-occurrence patterns, and assembly processes of bacterial communities in the Liuxi River were investigated during the WS and DS. Noticeable differences were observed in the seasonal variation of bacterial community. The relative abundance of Proteobacteria and Deinococcus-Thermus was significantly higher in the WS, while Bacteriodetes and Firmicutes exhibited significantly higher relative abundance in the DS. Bacterial communities in the DS showed higher alpha diversity and heterogeneity compared to the WS. The Mantel test and RDA identified pH, T, DO, NO3−, NH4+, and DOC as major factors affecting seasonal variation in bacterial communities. Ecological network analysis revealed that bacterial communities in the WS exhibited more interactions and a higher proportion of positive correlations than those in the DS; whereas they exhibited higher modularity and competition in the DS. Furthermore, the number and compositions of keystone taxa also exhibited distinct seasonal variations. Based on Zi and Pi values, six keystone species belonging to Proteobacteria and Actinobacteria were identified during the WS, while nine keystone species belonging to Actinobacteria, Cyanobacteria, Proteobacteria, and Bacteroidetes were identified during the DS. The results from both the null model and the neutral community model consistently indicate that stochasticity especially dispersal limitation dominated the assembly process for bacterial communities in both seasons; however, during the DS, dispersal limitation exerts a greater influence due to lower immigration rates of bacterial communities. This study extends our understanding of the microbial community ecology in subtropical rivers. However, considering potential future environmental disturbances resulting from climate change and/or human activities on this river ecosystem, periodic investigations on a larger temporal scale are recommended to gain a better understanding of these dynamics.
Data availability statement
The original contributions presented in the study are included in the article/Supplementary material, further inquiries can be directed to the corresponding authors.
Author contributions
AZ: Conceptualization, Data curation, Formal analysis, Funding acquisition, Investigation, Methodology, Project administration, Writing – original draft, Writing – review & editing. ZL: Investigation, Writing – review & editing. LG: Funding acquisition, Investigation, Writing – review & editing. ZX: Investigation, Writing – review & editing.
Funding
The author(s) declare that financial support was received for the research, authorship, and/or publication of this article. The study was supported by the National Natural Science Foundation of China (41907179), the Research Fund Program of Key Laboratory of Vegetation Restoration and Management of Degraded Ecosystems, Chinese Academy of Sciences (VRMDE2202) and the Youth Innovation Promotion Association CAS (2022352) and the Science and Technology Project of Zhejiang Water Resources Department, China (RC2226).
Conflict of interest
The authors declare that the research was conducted in the absence of any commercial or financial relationships that could be construed as a potential conflict of interest.
Publisher’s note
All claims expressed in this article are solely those of the authors and do not necessarily represent those of their affiliated organizations, or those of the publisher, the editors and the reviewers. Any product that may be evaluated in this article, or claim that may be made by its manufacturer, is not guaranteed or endorsed by the publisher.
Supplementary material
The Supplementary material for this article can be found online at: https://www.frontiersin.org/articles/10.3389/fmicb.2024.1430073/full#supplementary-material
Footnotes
References
Banerjee, S., Schlaeppi, K., and van der Heijden, M. G. A. (2018). Keystone taxa as drivers of microbiome structure and functioning. Nat. Rev. Microbiol. 16, 567–576. doi: 10.1038/s41579-018-0024-1
Chen, H., Chen, Z., Chu, X., Deng, Y., Qing, S., Sun, C., et al. (2022). Temperature mediated the balance between stochastic and deterministic processes and reoccurrence of microbial community during treating aniline wastewater. Water Res. 221:118741. doi: 10.1016/j.watres.2022.118741
Chen, C., Li, P., Yin, M., Wang, J., Sun, Y., Ju, W., et al. (2023). Deciphering characterization of seasonal variations in microbial communities of marine ranching: diversity, co-occurrence network patterns, and assembly processes. Mar. Pollut. Bull. 197:115739. doi: 10.1016/j.marpolbul.2023.115739
Chen, W., Ren, K., Isabwe, A., Chen, H., Liu, M., and Yang, J. (2019). Stochastic processes shape microeukaryotic community assembly in a subtropical river across wet and dry seasons. Microbiome 7:138. doi: 10.1186/s40168-019-0749-8
Chen, J., Zhu, A., Tang, C., Luo, Y., and Zhang, Y. (2014). Nitrogen aspects of hydrological processes: a case study in Likeng landfill, Guangzhou, China. Environ. Sci.: Processes Impacts 16, 2604–2616. doi: 10.1039/C4EM00194J
Coyte, K. Z., Schluter, J., and Foster, K. R. (2015). The ecology of the microbiome: networks, competition, and stability. Science 350, 663–666. doi: 10.1126/science.aad2602
Dai, T., Wen, D., Bates, C. T., Wu, L., Guo, X., Liu, S., et al. (2022). Nutrient supply controls the linkage between species abundance and ecological interactions in marine bacterial communities. Nat. Commun. 13:175. doi: 10.1038/s41467-021-27857-6
Deng, Y., Jiang, Y. H., Yang, Y., He, Z., Luo, F., and Zhou, J. (2012). Molecular ecological network analyses. BMC Bioinformatics 13:113. doi: 10.1186/1471-2105-13-113
Dini-Andreote, F., Stegen, J. C., van Elsas, J. D., and Salles, J. F. (2015). Disentangling mechanisms that mediate the balance between stochastic and deterministic processes in microbial succession. Proc. Natl. Acad. Sci. U.S.A. 112, E1326–E1332. doi: 10.1073/pnas.1414261112
Do, M. H., Ngo, H. H., Guo, W., Chang, S. W., Nguyen, D. D., Sharma, P., et al. (2021). Performance of a dual-chamber microbial fuel cell as biosensor for on-line measuring ammonium nitrogen in synthetic municipal wastewater. Sci. Total Environ. 795:148755. doi: 10.1016/j.scitotenv.2021.148755
Du, L., Zhong, S., Luo, K., Yang, S., Xia, J., and Chen, Q. (2023). Effect of metal pollution on the distribution and co-occurrence pattern of bacterial, archaeal and fungal communities throughout the soil profiles. Chemosphere 315:137692. doi: 10.1016/j.chemosphere.2022.137692
Edgar, R. C. (2013). UPARSE: highly accurate OTU sequences from microbial amplicon reads. Nat. Methods 10, 996–998. doi: 10.1038/nmeth.2604
Fan, N. S., Bai, Y. H., Wu, J., Zhang, Q., Fu, J. J., Zhou, W. L., et al. (2020). A two-stage anammox process for the advanced treatment of high-strength ammonium wastewater: microbial community and nitrogen transformation. J. Clean. Prod. 261:121148. doi: 10.1016/j.jclepro.2020.121148
Fang, W., Fan, T., Xu, L., Wang, S., Wang, X., Lu, A., et al. (2023). Seasonal succession of microbial community co-occurrence patterns and community assembly mechanism in coal mining subsidence lakes. Front. Microbiol. 14:1098236. doi: 10.3389/fmicb.2023.1098236
Fuhrman, J. A., Hewson, I., Schwalbach, M. S., Steele, J. A., Brown, M. V., and Naeem, S. (2006). Annually reoccurring bacterial communities are predictable from ocean conditions. Proc. Natl. Acad. Sci. U.S.A. 103, 13104–13109. doi: 10.1073/pnas.0602399103
Gao, Y., Wang, C., Zhang, W., Di, P., Yi, N., and Chen, C. (2017). Vertical and horizontal assemblage patterns of bacterial communities in a eutrophic river receiving domestic wastewater in southeast China. Environ. Pollut. 230, 469–478. doi: 10.1016/j.envpol.2017.06.081
Gao, Y., Zhang, W., Li, Y., Wu, H., Yang, N., and Hui, C. (2021). Dams shift microbial community assembly and imprint nitrogen transformation along the Yangtze River. Water Res. 189:116579. doi: 10.1016/j.watres.2020.116579
Han, F., Zhang, M., Shang, H., Liu, Z., and Zhou, W. (2020). Microbial community succession, species interactions and metabolic pathways of sulfur-based autotrophic denitrification system in organic-limited nitrate wastewater. Bioresour. Technol. 315:123826. doi: 10.1016/j.biortech.2020.123826
He, Q., Wang, S., Hou, W., Feng, K., Li, F., Hai, W., et al. (2021). Temperature and microbial interactions drive the deterministic assembly processes in sediments of hot springs. Sci. Total Environ. 772:145465. doi: 10.1016/j.scitotenv.2021.145465
Huang, Z., Pan, B., Soininen, J., Liu, X., Hou, Y., and Liu, X. (2023a). Seasonal variation of phytoplankton community assembly processes in Tibetan Plateau floodplain. Front. Microbiol. 14:1122838. doi: 10.3389/fmicb.2023.1122838
Huang, Z., Pan, B., Zhao, X., Liu, X., Liu, X., and Zhao, G. (2023b). Hydrological disturbances enhance stochastic assembly processes and decrease network stability of algae communities in a highland floodplain system. Sci. Total Environ. 903:166207. doi: 10.1016/j.scitotenv.2023.166207
Huber, P., Metz, S., Unrein, F., Mayora, G., Sarmento, H., and Devercelli, M. (2020). Environmental heterogeneity determines the ecological processes that govern bacterial metacommunity assembly in a floodplain river system. ISME J. 14, 2951–2966. doi: 10.1038/s41396-020-0723-2
Isabwe, A., Yang, J. R., Wang, Y., Liu, L., Chen, H., and Yang, J. (2018). Community assembly processes underlying phytoplankton and bacterioplankton across a hydrologic change in a human-impacted river. Sci. Total Environ. 630, 658–667. doi: 10.1016/j.scitotenv.2018.02.210
Ji, D., Wen, Y., Wei, J., Wu, Z., and Liu, Q. (2015). Relationships between landscape spatial characteristics and surface water quality in the Liuxi River watershed. Acta Ecol. Sin. 35, 246–253. doi: 10.5846/stxb201303230496 (in Chinese).
Ji, L., Yang, Y., and Yang, L. (2021). Seasonal variations in soil fungal communities and co-occurrence networks along an altitudinal gradient in the cold temperate zone of China: a case study on Oakley Mountain. Catena 204:105448. doi: 10.1016/j.catena.2021.105448
Ji, L., Zhang, L., Wang, Z., Zhu, X., and Ning, K. (2022). High biodiversity and distinct assembly patterns of microbial communities in groundwater compared with surface water. Sci. Total Environ. 834:155345. doi: 10.1016/j.scitotenv.2022.155345
Jiang, L., Luo, C., Zhang, D., Song, M., Sun, Y., and Zhang, G. (2018). Biphenyl-metabolizing microbial community and a functional operon revealed in E-waste-contaminated soil. Environ. Sci. Technol. 52, 8558–8567. doi: 10.1021/acs.est.7b06647
Jiao, C., Zhao, D., Zeng, J., Guo, L., and Yu, Z. (2020). Disentangling the seasonal co-occurrence patterns and ecological stochasticity of planktonic and benthic bacterial communities within multiple lakes. Sci. Total Environ. 740:140010. doi: 10.1016/j.scitotenv.2020.140010
Kallmeyer, J., Pockalny, R., Adhikari, R. R., Smith, D. C., and D’Hondt, S. (2012). Global distribution of microbial abundance and biomass in subseafloor sediment. Proc. Natl. Acad. Sci. U.S.A. 109, 16213–16216. doi: 10.1073/pnas.1203849109
Kutvonen, H., Rajala, P., Carpén, L., and Bomberg, M. (2015). Nitrate and ammonia as nitrogen sources for deep subsurface microorganisms. Front. Microbiol. 6:1079. doi: 10.3389/fmicb.2015.01079
Kuypers, M. M. M., Marchant, H. K., and Kartal, B. (2018). The microbial nitrogen-cycling network. Nat. Rev. Microbiol. 16, 263–276. doi: 10.1038/nrmicro.2018.9
Lai, J., Zou, Y., Zhang, J., and Peres-Neto, P. R. (2022). Generalizing hierarchical and variation partitioning in multiple regression and canonical analyses using the rdacca.hp R package. Methods Ecol. Evol. 13, 782–788. doi: 10.1111/2041-210X.13800
Li, H., Liu, P. Q., Luo, Q. P., Ma, J. J., Yang, X. R., Yan, Y., et al. (2022). Spatiotemporal variations of microbial assembly, interaction, and potential risk in urban dust. Environ. Int. 170:107577. doi: 10.1016/j.envint.2022.107577
Li, M., Yao, J., Sunahara, G., Duran, R., Liu, B., Cao, Y., et al. (2023). Assembly processes of bacterial and fungal communities in metal(loid)s smelter soil. J. Hazard. Mater. 451:131153. doi: 10.1016/j.jhazmat.2023.131153
Liu, S., Wang, P., Wang, C., Chen, J., Wang, X., Hu, B., et al. (2021). Ecological insights into the disturbances in bacterioplankton communities due to emerging organic pollutants from different anthropogenic activities along an urban river. Sci. Total Environ. 796:148973. doi: 10.1016/j.scitotenv.2021.148973
Liu, Q., Wu, H., Huang, C., Lin, H., Li, W., Zhao, X., et al. (2022). Microbial compositions, ecological networks, and metabolomics in sediments of black-odour water in Dongguan, China. Environ. Res. 210:112918. doi: 10.1016/j.envres.2022.112918
Liu, H., Yao, J., Liu, B., Li, M., Liu, J., Jiang, S., et al. (2023). Active tailings disturb the surrounding vegetation soil fungal community: diversity, assembly process and co-occurrence patterns. Sci. Total Environ. 865:161133. doi: 10.1016/j.scitotenv.2022.161133
Lu, L., Tang, Q., Li, H., and Li, Z. (2022). Damming river shapes distinct patterns and processes of planktonic bacterial and microeukaryotic communities. Environ. Microbiol. 24, 1760–1774. doi: 10.1111/1462-2920.15872
Lu, M., Wang, X., Li, H., Jiao, J. J., Luo, X., Luo, M., et al. (2022). Microbial community assembly and co-occurrence relationship in sediments of the river-dominated estuary and the adjacent shelf in the wet season. Environ. Pollut. 308:119572. doi: 10.1016/j.envpol.2022.119572
Lv, B., Shi, J., Li, T., Ren, L., Tian, W., Lu, X., et al. (2022). Deciphering the characterization, ecological function and assembly processes of bacterial communities in ship ballast water and sediments. Sci. Total Environ. 816:152721. doi: 10.1016/j.scitotenv.2021.152721
Miao, Y., Liao, R., Zhang, X.-X., Liu, B., Li, Y., Wu, B., et al. (2015). Metagenomic insights into salinity effect on diversity and abundance of denitrifying bacteria and genes in an expanded granular sludge bed reactor treating high-nitrate wastewater. Chem. Eng. J. 277, 116–123. doi: 10.1016/j.cej.2015.04.125
Milke, F., Wagner-Doebler, I., Wienhausen, G., and Simon, M. (2022). Selection, drift and community interactions shape microbial biogeographic patterns in the Pacific Ocean. ISME J. 16, 2653–2665. doi: 10.1038/s41396-022-01318-4
Ning, S., Hu, J., Yu, J., Huang, L., Lai, M., Li, X., et al. (2023). Hydrogen injection reduces ammonia nitrogen and changes microbial community composition in aquaculture water. Front. Mar. Sci. 10:1111067. doi: 10.3389/fmars.2023.1111067
Nyirabuhoro, P., Liu, M., Xiao, P., Liu, L., Yu, Z., Wang, L., et al. (2020). Seasonal variability of conditionally rare taxa in the water column bacterioplankton community of subtropical reservoirs in China. Microb. Ecol. 80, 14–26. doi: 10.1007/s00248-019-01458-9
Obieze, C. C., Wani, G. A., Shah, M. A., Reshi, Z. A., Comeau, A. M., and Khasa, D. P. (2022). Anthropogenic activities and geographic locations regulate microbial diversity, community assembly and species sorting in Canadian and Indian freshwater lakes. Sci. Total Environ. 826:154292. doi: 10.1016/j.scitotenv.2022.154292
Ohore, O. E., Wang, Y., Wei, Y., Sanganyado, E., Shafiq, M., Jiao, X., et al. (2023). Ecological mechanisms of sedimental microbial biodiversity shift and the role of antimicrobial resistance genes in modulating microbial turnover. J. Environ. Manag. 325:116547. doi: 10.1016/j.jenvman.2022.116547
Ouyang, L., Chen, H., Liu, X., Wong, M. H., Xu, F., Yang, X., et al. (2020). Characteristics of spatial and seasonal bacterial community structures in a river under anthropogenic disturbances. Environ. Pollut. 264:114818. doi: 10.1016/j.envpol.2020.114818
Palmer, M., and Ruhi, A. (2019). Linkages between flow regime, biota, and ecosystem processes: implications for river restoration. Science 365:eaaw2087. doi: 10.1126/science.aaw2087
Pan, B., Liu, X., Chen, Q., Sun, H., Zhao, X., and Huang, Z. (2022). Hydrological connectivity promotes coalescence of bacterial communities in a floodplain. Front. Microbiol. 13:971437. doi: 10.3389/fmicb.2022.971437
Qin, Z., Zhao, Z., Xia, L., and Wang, S. (2022). Pollution pressure and soil depth drive prokaryotic microbial assemblage and co-occurrence patterns in an organic polluted site. J. Hazard. Mater. 438:129570. doi: 10.1016/j.jhazmat.2022.129570
Qing, C., Nicol, A., Li, P., Planer-Friedrich, B., Yuan, C., and Kou, Z. (2023). Different sulfide to arsenic ratios driving arsenic speciation and microbial community interactions in two alkaline hot springs. Environ. Res. 218:115033. doi: 10.1016/j.envres.2022.115033
Ren, Z., Qu, X., Zhang, M., Yu, Y., and Peng, W. (2019). Distinct bacterial communities in wet and dry seasons during a seasonal water level fluctuation in the largest freshwater lake (Poyang Lake) in China. Front. Microbiol. 10:1167. doi: 10.3389/fmicb.2019.01167
Ruprecht, J. E., Birrer, S. C., Dafforn, K. A., Mitrovic, S. M., Crane, S. L., Johnston, E. L., et al. (2021). Wastewater effluents cause microbial community shifts and change trophic status. Water Res. 200:117206. doi: 10.1016/j.watres.2021.117206
Sha, C., Wu, J., Shen, C., Wu, J., Yan, Z., and Wang, M. (2023). The ecology of bacterial communities in groundwater of industrial areas: diversity, composition, network, and assembly. Environ. Pollut. 322:121207. doi: 10.1016/j.envpol.2023.121207
Sharma, N., Kumar, J., Abedin, M. M., Sahoo, D., Pandey, A., Rai, A. K., et al. (2020). Metagenomics revealing molecular profiling of community structure and metabolic pathways in natural hot springs of the Sikkim Himalaya. BMC Microbiol. 20:246. doi: 10.1186/s12866-020-01923-3
She, J., Liu, J., He, H., Zhang, Q., Lin, Y., Wang, J., et al. (2022). Microbial response and adaption to thallium contamination in soil profiles. J. Hazard. Mater. 423:127080. doi: 10.1016/j.jhazmat.2021.127080
She, Z., Pan, X., Wang, J., Shao, R., Wang, G., Wang, S., et al. (2021). Vertical environmental gradient drives prokaryotic microbial community assembly and species coexistence in a stratified acid mine drainage lake. Water Res. 206:117739. doi: 10.1016/j.watres.2021.117739
Shen, X., Xu, M., Li, M., Zhao, Y., and Shao, X. (2020). Response of sediment bacterial communities to the drainage of wastewater from aquaculture ponds in different seasons. Sci. Total Environ. 717:137180. doi: 10.1016/j.scitotenv.2020.137180
Shuwang, X., Zhang, G., Li, D., Wen, Y., Zhang, G., and Sun, J. (2023). Spatial and temporal changes in the assembly mechanism and co-occurrence network of the chromophytic phytoplankton communities in coastal ecosystems under anthropogenic influences. Sci. Total Environ. 877:162831. doi: 10.1016/j.scitotenv.2023.162831
Sloan, W. T., Lunn, M., Woodcock, S., Head, I. M., Nee, S., and Curtis, T. P. (2010). Quantifying the roles of immigration and chance in shaping prokaryote community structure. Environ. Microbiol. 96, 732–740. doi: 10.1111/j.1462-2920.2005.00956.x
Spain, A. M., Krumholz, L. R., and Elshahed, M. S. (2009). Abundance, composition, diversity and novelty of soil Proteobacteria. ISME J. 3, 992–1000. doi: 10.1038/ismej.2009.43
Stegen, J. C., Lin, X., Fredrickson, J. K., Chen, X., Kennedy, D. W., Murray, C. J., et al. (2013). Quantifying community assembly processes and identifying features that impose them. ISME J. 7, 2069–2079. doi: 10.1038/ismej.2013.93
Stegen, J. C., Lin, X., Fredrickson, J. K., and Konopka, A. E. (2015). Estimating and mapping ecological processes influencing microbial community assembly. Front. Microbiol. 6:370. doi: 10.3389/fmicb.2015.00370
Stegen, J. C., Lin, X., Konopka, A. E., and Fredrickson, J. K. (2012). Stochastic and deterministic assembly processes in subsurface microbial communities. ISME J. 6, 1653–1664. doi: 10.1038/ismej.2012.22
Sun, H., Pan, B., He, H., Zhao, G., Hou, Y., and Zhu, P. (2021). Assembly processes and co-occurrence relationships in the bacterioplankton communities of a large river system. Ecol. Indic. 126:107643. doi: 10.1016/j.ecolind.2021.107643
Sun, C., Zhang, B., Ning, D., Zhang, Y., Dai, T., Wu, L., et al. (2021). Seasonal dynamics of the microbial community in two full-scale wastewater treatment plants: diversity, composition, phylogenetic group based assembly and co-occurrence pattern. Water Res. 200:117295. doi: 10.1016/j.watres.2021.117295
Wagg, C., Schlaeppi, K., Banerjee, S., Kuramae, E. E., and van der Heijden, M. G. A. (2019). Fungal-bacterial diversity and microbiome complexity predict ecosystem functioning. Nat. Commun. 10:4841. doi: 10.1038/s41467-019-12798-y
Wang, J., Legendre, P., Soininen, J., Yeh, C. F., Graham, E., Stegen, J., et al. (2020). Temperature drives local contributions to beta diversity in mountain streams: stochastic and deterministic processes. Glob. Ecol. Biogeogr. 29, 420–432. doi: 10.1111/geb.13035
Wang, Y., Liu, L., Chen, H., and Yang, J. (2015). Spatiotemporal dynamics and determinants of planktonic bacterial and microeukaryotic communities in a Chinese subtropical river. Appl. Microbiol. Biotechnol. 99, 9255–9266. doi: 10.1007/s00253-015-6773-0
Wang, Y., Liu, Y., Wang, J., Luo, T., Zhang, R., Sun, J., et al. (2019). Seasonal dynamics of bacterial communities in the surface seawater around subtropical Xiamen Island, China, as determined by 16S rRNA gene profiling. Mar. Pollut. Bull. 142, 135–144. doi: 10.1016/j.marpolbul.2019.03.035
Wang, H., Liu, X., Wang, Y., Zhang, S., Zhang, G., Han, Y., et al. (2023). Spatial and temporal dynamics of microbial community composition and factors influencing the surface water and sediments of urban rivers. J. Environ. Sci. 124, 187–197. doi: 10.1016/j.jes.2021.10.016
Wang, Y., Wang, Y., Shang, J., Wang, L., Li, Y., Wang, Z., et al. (2024). Redox gradients drive microbial community assembly patterns and molecular ecological networks in the hyporheic zone of effluent-dominated rivers. Water Res. 248:120900. doi: 10.1016/j.watres.2023.120900
Wang, J., Xie, R., He, N., Wang, W., Wang, G., Yang, Y., et al. (2024). Five years nitrogen reduction management shifted soil bacterial community structure and function in high-yielding ‘super’ rice cultivation. Agric. Ecosyst. Environ. 360:108773. doi: 10.1016/j.agee.2023.108773
Wang, L., Zhang, J., Li, H., Yang, H., Peng, C., Peng, Z., et al. (2018). Shift in the microbial community composition of surface water and sediment along an urban river. Sci. Total Environ. 627, 600–612. doi: 10.1016/j.scitotenv.2018.01.203
Wang, B., Zheng, X., Zhang, H., Xiao, F., Gu, H., Zhang, K., et al. (2020). Bacterial community responses to tourism development in the Xixi National Wetland Park, China. Sci. Total Environ. 720:137570. doi: 10.1016/j.scitotenv.2020.137570
Widder, S., Besemer, K., Singer, G. A., Ceola, S., Bertuzzo, E., Quince, C., et al. (2014). Fluvial network organization imprints on microbial co-occurrence networks. Proc. Natl. Acad. Sci. U.S.A. 111, 12799–12804. doi: 10.1073/pnas.1411723111
Wu, L., Ding, X., Lin, Y., Lu, X., Lv, H., Zhao, M., et al. (2022). Nitrogen removal by a novel heterotrophic nitrification and aerobic denitrification bacterium Acinetobacter calcoaceticus TY1 under low temperatures. Bioresour. Technol. 353:127148. doi: 10.1016/j.biortech.2022.127148
Wu, Z., Sun, J., Xu, L., Zhou, H., Cheng, H., Chen, Z., et al. (2024). Depth significantly affects plastisphere microbial evenness, assembly and co-occurrence pattern but not richness and composition. J. Hazard. Mater. 463:132921. doi: 10.1016/j.jhazmat.2023.132921
Xiao, Z., Li, G., Zhao, Y., Xiao, K., Chen, Q., Bao, P., et al. (2021). Bacterioplankton richness and composition in a seasonal urban river. Front. Environ. Sci. 9:731227. doi: 10.3389/fenvs.2021.731227
Xie, Z., Gao, L., Liang, Z., Chen, J., Li, S., Zhu, A., et al. (2020). Characteristics, sources, and risks of polycyclic aromatic hydrocarbons in topsoil and surface water from the Liuxi River basin, south China. Arch. Environ. Contam. Toxicol. 78, 401–415. doi: 10.1007/s00244-020-00711-4
Xu, R., Sun, X., Lin, H., Han, F., Xiao, E., Li, B., et al. (2020). Microbial adaptation in vertical soil profiles contaminated by an antimony smelting plant. FEMS Microbiol. Ecol. 96:fiaa188. doi: 10.1093/femsec/fiaa188
Xue, Y., Liu, M., Chen, H., Jeppesen, E., Zhang, H., Ren, K., et al. (2022). Microbial hierarchical correlations and their contributions to carbon-nitrogen cycling following a reservoir cyanobacterial bloom. Ecol. Indic. 143:109401. doi: 10.1016/j.ecolind.2022.109401
Yan, K., You, Q., Wang, S., Zou, Y., Chen, J., Xu, J., et al. (2023). Depth-dependent patterns of soil microbial community in the E-waste dismantling area. J. Hazard. Mater. 444:130379. doi: 10.1016/j.jhazmat.2022.130379
Yang, N., Hou, X., Li, Y., Zhang, H., Wang, J., Hu, X., et al. (2023). Inter-basin water diversion homogenizes microbial communities mainly through stochastic assembly processes. Environ. Res. 223:115473. doi: 10.1016/j.envres.2023.115473
Yang, C., Li, K., Lv, D., Jiang, S., Sun, J., Lin, H., et al. (2021). Inconsistent response of bacterial phyla diversity and abundance to soil salinity in a Chinese delta. Sci. Rep. 11:12870. doi: 10.1038/s41598-021-92502-7
Yang, Z., Liang, Z., Gao, L., Li, R., Wang, Z., Zhu, A., et al. (2020). Quantifying the impacts of anthropogenic activity on chemical weathering based on source identification of hydrochemistry: the Liuxi River basin, southern China. Environ. Sci.: Processes Impacts 22, 2406–2417. doi: 10.1039/D0EM00332H
Yi, M., Fang, Y., Hu, G., Liu, S., Ni, J., and Liu, T. (2021). Distinct community assembly processes underlie significant spatiotemporal dynamics of abundant and rare bacterioplankton in the Yangtze River. Front. Environ. Sci. Eng. 16:79. doi: 10.1007/s11783-021-1513-4
Yu, X., Gao, X., Shang, L., Wang, X., Jiao, Y., Zhang, X. H., et al. (2023). Spatial and temporal change determined co-occurrence networks stability and community assembly processes of epipelagic seawater microbial community in the Nordic Sea. Sci. Total Environ. 859:160321. doi: 10.1016/j.scitotenv.2022.160321
Yu, C., Zhu, Z., Meng, K., Zhang, H., and Xu, M. (2023). Unveiling the impact and mechanisms of cd-driven ecological assembly and coexistence of bacterial communities in coastal sediments of Yellow Sea. J. Hazard. Mater. 460:132309. doi: 10.1016/j.jhazmat.2023.132309
Yuan, B., Guo, M., Wu, W., Zhou, X., Li, M., and Xie, S. (2022). Spatial and seasonal patterns of sediment bacterial communities in large river cascade reservoirs: drivers, assembly processes, and co-occurrence relationship. Microb. Ecol. 85, 586–603. doi: 10.1007/s00248-022-01999-6
Zelezniak, A., Andrejev, S., Ponomarova, O., Mende, D. R., Bork, P., and Patil, K. R. (2015). Metabolic dependencies drive species co-occurrence in diverse microbial communities. Proc. Natl. Acad. Sci. U.S.A. 112, 6449–6454. doi: 10.1073/pnas.1421834112
Zeng, J., Lin, Y., Zhao, D., Huang, R., Xu, H., and Jiao, C. (2019). Seasonality overwhelms aquacultural activity in determining the composition and assembly of the bacterial community in Lake Taihu, China. Sci. Total Environ. 683, 427–435. doi: 10.1016/j.scitotenv.2019.05.256
Zhang, L., Delgado-Baquerizo, M., Shi, Y., Liu, X., Yang, Y., and Chu, H. (2021). Co-existing water and sediment bacteria are driven by contrasting environmental factors across glacier-fed aquatic systems. Water Res. 198:117139. doi: 10.1016/j.watres.2021.117139
Zhang, T., Xu, S., Yan, R., Wang, R., Gao, Y., Kong, M., et al. (2022). Similar geographic patterns but distinct assembly processes of abundant and rare bacterioplankton communities in river networks of the Taihu Basin. Water Res. 211:118057. doi: 10.1016/j.watres.2022.118057
Zhang, L., Zhong, M., Li, X., Lu, W., and Li, J. (2020). River bacterial community structure and co-occurrence patterns under the influence of different domestic sewage types. J. Environ. Manag. 266:110590. doi: 10.1016/j.jenvman.2020.110590
Zhao, Q., Bai, J., Gao, Y., Zhao, H., Zhang, G., and Cui, B. (2020). Shifts in the soil bacterial community along a salinity gradient in the Yellow River Delta. Land Degrad. Dev. 31, 2255–2267. doi: 10.1002/ldr.3594
Zhao, Z., Li, H., Sun, Y., Zhan, A., Lan, W., Woo, S. P., et al. (2022). Bacteria versus fungi for predicting anthropogenic pollution in subtropical coastal sediments: assembly process and environmental response. Ecol. Indic. 134:108484. doi: 10.1016/j.ecolind.2021.108484
Zhao, J., Peng, W., Ding, M. J., Nie, M. H., and Huang, G. X. (2021). Effect of water chemistry, land use patterns, and geographic distances on the spatial distribution of bacterioplankton communities in an anthropogenically disturbed riverine ecosystem. Front. Microbiol. 12:633993. doi: 10.3389/fmicb.2021.633993
Zhao, D., Shen, F., Zeng, J., Huang, R., Yu, Z., and Wu, Q. L. (2016). Network analysis reveals seasonal variation of co-occurrence correlations between Cyanobacteria and other bacterioplankton. Sci. Total Environ. 573, 817–825. doi: 10.1016/j.scitotenv.2016.08.150
Zhong, X., Chen, Z., Ding, K., Liu, W. S., Baker, A. J. M., Fei, Y. H., et al. (2023). Heavy metal contamination affects the core microbiome and assembly processes in metal mine soils across eastern China. J. Hazard. Mater. 443:130241. doi: 10.1016/j.jhazmat.2022.130241
Zhou, Q., Lin, L., Wang, J., Huang, X., Tang, Q., and Wei, G. (2018). Seasonal and spatial variation of nitrogen and phosphorus concentrations in Liuxi River in Guangzhou. Chin. J. Ecol. 37, 3100–3109. doi: 10.13292/j.1000-4890.201810.013 (in Chinese).
Zhou, L., Liu, L., Chen, W. Y., Sun, J. J., Hou, S. W., Kuang, T. X., et al. (2020). Stochastic determination of the spatial variation of potentially pathogenic bacteria communities in a large subtropical river. Environ. Pollut. 264:114683. doi: 10.1016/j.envpol.2020.114683
Zhou, L., Wang, P., Huang, S., Li, Z., Gong, H., Huang, W., et al. (2021). Environmental filtering dominates bacterioplankton community assembly in a highly urbanized estuarine ecosystem. Environ. Res. 196:110934. doi: 10.1016/j.envres.2021.110934
Zhu, A., Chen, J., Gao, L., Shimizu, Y., Liang, D., Yi, M., et al. (2019). Combined microbial and isotopic signature approach to identify nitrate sources and transformation processes in groundwater. Chemosphere 228, 721–734. doi: 10.1016/j.chemosphere.2019.04.163
Zhu, A., Yang, Z., Liang, Z., Gao, L., Li, R., Hou, L., et al. (2020). Integrating hydrochemical and biological approaches to investigate the surface water and groundwater interactions in the hyporheic zone of the Liuxi River basin, southern China. J. Hydrol. 583:124622. doi: 10.1016/j.jhydrol.2020.124622
Zou, K., Zhu, Y., Jiang, Y., Ma, S., Li, M., Zhao, W., et al. (2023). Distinct stochastic processes drive bacterial community assembly and co-occurrence patterns with common antibiotic resistance genes in two highly urbanised coastal ecosystems of the Pearl River Estuary. J. Hazard. Mater. 459:132161. doi: 10.1016/j.jhazmat.2023.132161
Keywords: bacterial communities, seasonal patterns, ecological network analysis, null model, Liuxi River
Citation: Zhu A, Liang Z, Gao L and Xie Z (2024) Dispersal limitation determines the ecological processes that regulate the seasonal assembly of bacterial communities in a subtropical river. Front. Microbiol. 15:1430073. doi: 10.3389/fmicb.2024.1430073
Edited by:
Hidetoshi Urakawa, Florida Gulf Coast University, United StatesReviewed by:
Jie Wang, China Agricultural University, ChinaAshraf Mohammad Fahmi Al Ashhab, Dead Sea and Arava Science Center, Israel
Xun Wang, Hohai University, China
Copyright © 2024 Zhu, Liang, Gao and Xie. This is an open-access article distributed under the terms of the Creative Commons Attribution License (CC BY). The use, distribution or reproduction in other forums is permitted, provided the original author(s) and the copyright owner(s) are credited and that the original publication in this journal is cited, in accordance with accepted academic practice. No use, distribution or reproduction is permitted which does not comply with these terms.
*Correspondence: Aiping Zhu, zhuaiping329@126.com; Lei Gao, nvtoo@sina.com