- 1Jiangxi Provincial Key Laboratory of Genesis and Remediation of Groundwater Pollution, East China University of Technology, Nanchang, China
- 2Jiangxi Center for Patriotic Health and Health Promotion, Nanchang, China
Airborne microorganisms, an emerging global health threat, have attracted extensive studies. However, few attentions have been paid to the seasonal distribution of airborne pathogens, in particular their associations with antibiotic resistance genes (ARGs). To this end, two-week daily PM2.5 samples were consecutively collected from Nanchang in four seasons, and the human-to-human pathogens were screened based on high-throughput sequencing. The results showed that there were 20 pathogenic taxa in PM2.5 in Nanchang, and the highest relative abundance of pathogens was observed in winter (5.84%), followed by summer (3.51%), autumn (2.66%), and spring (1.80%). Although more than half of pathogenic taxa were shared by the four seasons, the analysis of similarities showed that pathogenic community was shaped by season (r = 0.16, p < 0.01). Co-occurrence network analysis disclosed significant interactions among pathogens in each season. Moreover, some dominant pathogens such as Plesiomonas shigelloides, Bacteroides fragilis, and Escherichia-Shigella were hub pathogens. In addition, PICRUSt2 predicted that there were 35 high-risk ARG subtypes in PM2.5, and the pathogens had strongly positive correlations with these ARGs. Even some pathogens like Plesiomonas shigelloides, Bacteroides fragilis, Aeromonas, Citrobacter, may be multi-drug resistant pathogens, including beta-lactam, aminoglycosides, chloramphenicol and multi-drug resistances, etc. Both air pollutants and meteorological conditions contributed to the seasonal variation of airborne pathogenic bacteria (r = 0.15, p < 0.01), especially CO, O3, PM2.5, temperature and relative humidity. This study furthers our understanding of airborne pathogens and highlights their associations with ARGs.
1 Introduction
Bacteria, the main component of atmospheric particulate matter, are prevalent in the near-surface atmosphere at concentrations of about 104–108 cells/m3 (Bowers et al., 2011; Yan et al., 2022). Airborne bacteria can not only be involved in the formation of clouds and rains as cloud/ice condensation nuclei, affecting the atmospheric physicochemical processes (Wei et al., 2017; Tang et al., 2022), but also pose a threat to human health. Because some human pathogens were detected among airborne microorganisms, accounting for 0.35–26.72% of total airborne bacteria (Du et al., 2018; Fan et al., 2019). Furthermore, unlike drinking water and food, atmospheric particulate matters are generally untreated and directly contact with the human skins or are inhaled by human. Therefore, airborne pathogens attaching on particles may lead to skin irritation or inflammation (Callewaert et al., 2020), respiratory diseases (Xue et al., 2020), cardiovascular disease (Riggs et al., 2018), and so on.
Although numerous studies were conducted about atmospheric bacteria (Files et al., 2020; Ruiz-Gil et al., 2020), systematic studies on airborne pathogenic bacteria were scarce. Moreover, the abundance and composition of pathogenic bacteria varied greatly in the existing reports. For example, Du et al. (2018) have found that pathogenic bacteria in Beijing occupied 0.24–0.75% of the total bacteria, including Streptococcus, Prevotella, Erysipelothrix, Rickettsia, and Enterobacter, while Fan et al. (2019) have reported that the relative abundance of airborne pathogens in Beijing was 1.82–14.50%, containing 22 pathogenic genera such as Bacillus, Pseudomonas, Acinetobacter, and Clostridium. In particular, Lu et al. (2018) have reported that airborne pathogens in Xi’an accounted for higher than 20% of total bacterial community whether in clean or hazy days. This bias may be contributed by the different geophysical locations and sampling time. In additional, most of these potential pathogens were counted at the genus level, where some species involved may not be human pathogens. More accurate statistics are necessary.
Antibiotic resistance genes (ARGs) in atmospheric particulate matter have received widespread attention in recent years (Li et al., 2018; Yan et al., 2022; Ma et al., 2023). Several to hundreds of ARG subtypes have been detected in the atmosphere, with an absolute abundance about 104–105 copies/m3. Some high-risk ARG subtypes, such as sul1, tetM, blaTEM-1, and so on. If these ARGs are carried by human pathogens, once infected by human, they will greatly reduce the effectiveness of antibiotics, increase the cost and duration of treatment, and even lead to death (Zeng et al., 2019). However, the relationship between these ARGs and pathogens in the air remained limited.
Herein, seasonal distribution of human-to-human pathogens in airborne PM2.5 and their potential-carried ARGs were explored. Particle samples in different seasons were collected from Nanchang, the capital of Jiangxi Province, where PM2.5 are still one of the primary pollutants in the air. Moreover, our previous study found that several potential pathogens like Pseudomonas, Clostridium, and Bacillus, were detected in the airborne bacterial community in Nanchang (Pan et al., 2022). Zhu et al. (2021) also found that more than 100 ARG subtypes in the snow samples collected from Nanchang. This study aimed to systematically investigate seasonal variation of pathogenic abundance and community composition, find out the key pathogens and potential ARGs hosts, and finally explore their associations with environmental factors. Overall, this study advances our understanding on seasonal distribution of airborne pathogens and highlights the health risk of ARGs potential-carried by airborne pathogens.
2 Materials and methods
2.1 Sample collection
PM2.5 samples were collected on the roof of the Earth Science Building in East China University of Technology (28.72°N, 115.82°E). There were no obvious emission sources around the sampling site, but it was surrounded by residential areas. A high-flow sampler (KC-1000, Qingdao Electrical Equipment Co., Ltd., China) equipped with a PM2.5 inlet was used at a flow rate of 1 m3/min and 23.5 h for each sample. The daily samples were continuously collected for 2 weeks in each season, and the detailed sampling periods were Apr 30, 2019 – May 13, 2019 (14 samples, spring), Jan 18, 2020 – Jan 30, 2020 (14 samples, winter), Jul 1, 2020 – Jul 15, 2020 (15 samples, summer), and Oct 13, 2020 – Oct 26, 2020 (14 samples, autumn), respectively. To avoid contamination, the sampling filter membrane (8 in × 10 in) was sterilized in a muffle furnace at 450°C for 4 h before use. The inlet was scrubbed using 75% alcohol. A total of 57 samples were collected and stored in a refrigerator at −20°C.
The concentrations of air pollutants (SO2, NO2, O3, CO, PM2.5 and PM10) and meteorological data [temperature (T), relative humidity (RH), wind speed (WS) and precipitation] were collected from the environmental monitoring station nearest to the sampling point, and integrated according to the actual sampling time.
2.2 DNA extraction and sequencing
The DNA extraction method was according to our previous study (Pan et al., 2022, 2024). The filter membrane was firstly ground into powder with agate, and then DNA was extracted with DNeasy PowerSoil Pro Kit (Qiagen, United States) according to the instruction. The extracted DNA was sent to Guangzhou Genedenovo Company for sequencing. The V3–V4 region of 16S rRNA gene was amplified using the specific primers (341F: CCTACGGGNGGCWGCAG; 806R: GGACTACHVGGGTATCT AAT) with barcodes. After purification, the PCR products were quantified and equimolar pooled for sequencing on the Novaseq 6,000 platform. The raw data were uploaded in FASTA format under the SRA database in NCBI [Accession numbers: PRJNA867124 (summer, autumn, winter) and PRJNA867037 (spring)]. The Bioproject ID, Biosample ID and sample name for each season were provided in Supplementary Table S1.
2.3 Sequence analysis
After sequencing, the raw data were filtered with FASTP to obtain clean reads. Clean reads were then assembled into tags and denoised with FLASH (1.2.11) to obtain effective tags (Magoč and Salzberg, 2011). Next, effective tags were clustered according to 97% similarity with UPARSE (9.2.64) to obtain OTUs (Edgar, 2013). Chimeras were removed. The valid sequence with the highest abundance in each cluster was selected as the representative OTU sequence, which was annotated according to the SILVA database with a confidence threshold of 0.8 (Pruesse et al., 2007). All samples were resampled according to the lowest number of tags. Finally, an OTU table with relative abundance could be obtained. Bacterial community composition was then calculated for each season. The rarefaction curve was made and reached a plateau (Supplementary Figure S1), suggesting that the employed sequencing depth have captured the full diversity of bacterial community.
2.4 Screening of human-to-human pathogens and ARGs prediction
Pathogens in each season were screened according to Human-to-Human Pathogens Database published by1 National Health Commission of the People’s Republic of China (2023), where clearly indicated the pathogenic genera or species. ARGs were screened according to the method of Jiang et al. (2022). Firstly, the bacterial function profiles were predicted with PICRUSt2, and we obtained a table of functional genes including gene description and gene counts known as KOs. Secondly, we downloaded the list of ARGs from the KEGG database2. Thirdly, we intersected the KO table and ARGs list to obtain the potential ARGs composition. We focused on the high risk ARGs, including Rank I and Rank II ARGs as reported by Zhang A. et al. (2021). The average nearest sequenced taxon index (NSTI) used to evaluate the accuracy of PICRUSt2 results was 0.08 (lower than 0.2), suggesting that the PICRUSt2 results were reliable.
2.5 Statistic analysis
Venn diagram was used to show the shared pathogens among four seasons. Student t-test was applied to evaluate the differences in the total relative abundance of pathogenic bacteria between two seasons, and analysis of similarity (ANOSIM) based on Bray–Curtis distance was performed to assess the similarity of pathogen composition between seasons using R software. Co-occurrence network based on Spearman’s correlation (p < 0.05) was performed using Gephi software (0.9.2) to explore the interaction of pathogen and pathogen and then find out the key pathogens (the number of edges occupying more than 20% of the total edges). Likewise, the potential ARG hosts were also mined based on the significantly positive correlations between the relative abundance of pathogens and gene counts of ARGs. Spearman correlation test was performed using R software. Additionally, mantel test, redundancy analysis (RDA) and partial RDA (pRDA) were used to assess the contribution of environmental factors (air pollutants and meteorological conditions) to variation of airborne pathogens using R software. Figures were plotted using OringinLab 2018 and Omicshare platform.
3 Results
3.1 Diversity of airborne human-to-human pathogens
There are 20 human-to-human pathogenic taxa (genera and species) in PM2.5 in Nanchang. The number of pathogenic taxa in each season followed the pattern, summer (19), autumn (17), winter (16), spring (15) (Figure 1A). However, most of these pathogens (13) were shared by four seasons, including species from Prevotella, Escherichia-Shigella, Streptococcus, and so on. Notably, Rickettsia was only observed in spring, and Chromobacterium was a summer-specific pathogenic genus.
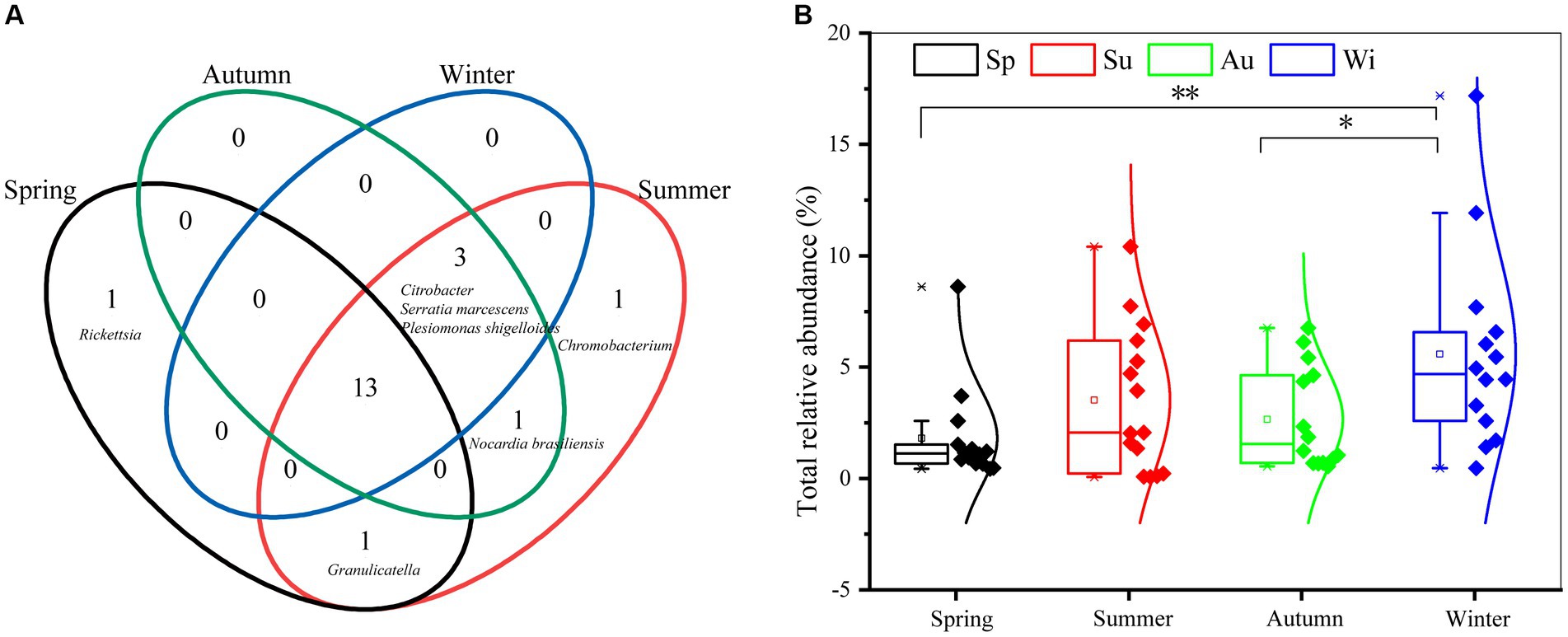
Figure 1. Comparison of pathogenic diversity in different seasons. (A) Venn diagram to show the number of shared pathogens among four seasons. (B) Statistical comparisons of the total relative abundance of pathogens between seasons. The upper line of the box plot is the maximum value, and the center line is the median, and the lower line is the minimum. The square dot is the mean relative abundance. The diamond dot represents samples. * and ** indicate the significant differences between two seasons with p < 0.05 and p < 0.01, respectively.
The total relative abundance of pathogens ranged from 0.07 to 12.21% in all samples, where the shared pathogens by four seasons accounted for 88.25–99.61% in each season. The average of total abundance was highest in winter (5.84% ± 4.44%), followed by summer (3.51% ± 3.25%), autumn (2.66% ± 2.29%), and spring (1.80% ± 2.15%) (Figure 1B). T-test showed the total relative abundance of pathogens in winter was obviously higher than that in spring and autumn.
3.2 Community composition of airborne human-to-human pathogens
As Figure 2 shown, Prevotella (1.59%), Escherichia-Shigella (0.77%), Plesiomonas shigelloides (0.23%), Streptococcus (0.22%), Bacteroides fragilis (0.18%), and Mycobacterium (0.11%), were the dominant pathogens (relative abundance >0.10%) in Nanchang. Among these pathogens, Prevotella and Escherichia-Shigella were found to be dominant in all seasons, and Mycobacterium and Plesiomonas shigelloides were shared by three seasons. However, Bacteroides fragilis occupied higher in winter. Additionally, Vibrio and Citrobacter were enriched in spring and winter, respectively.
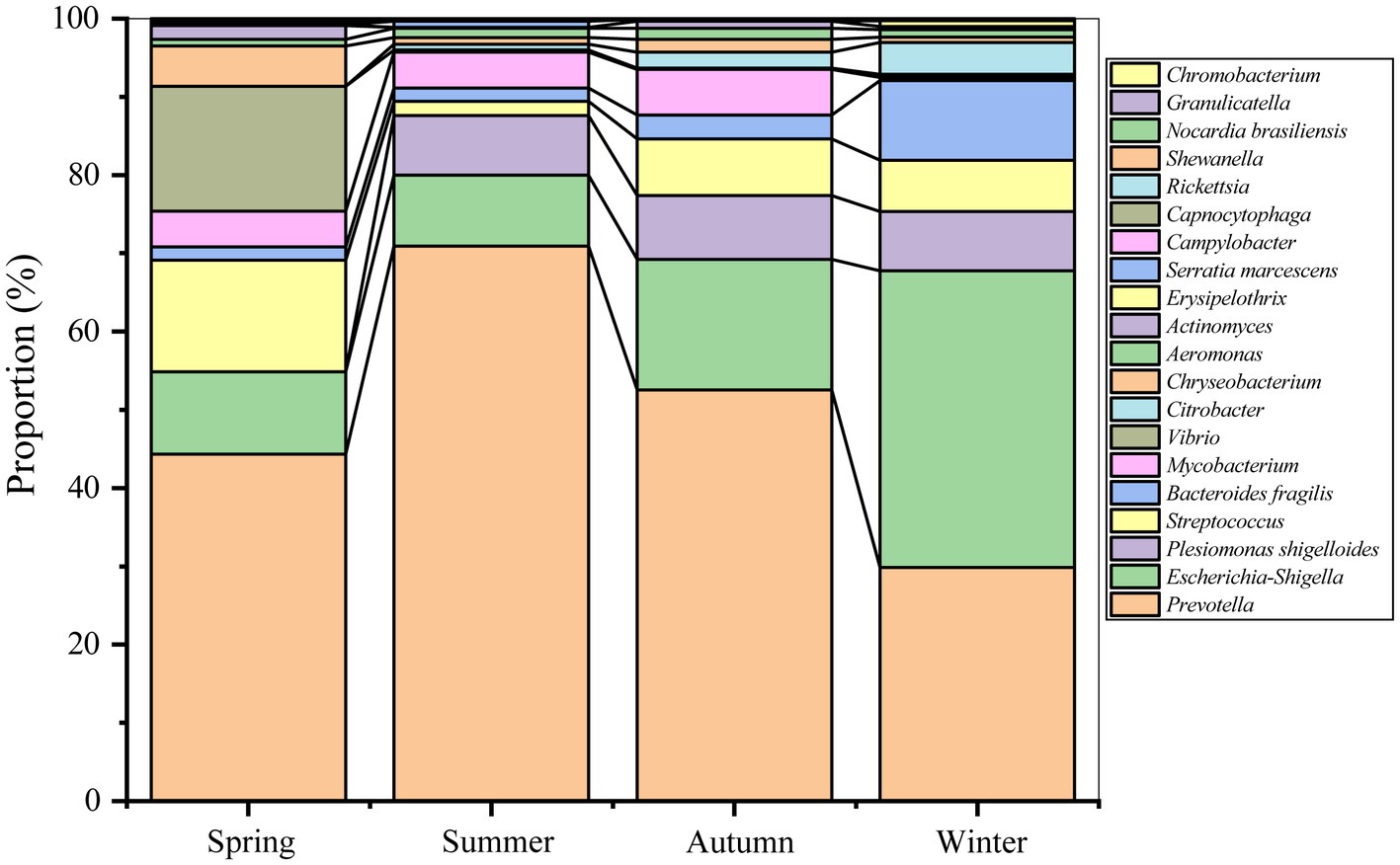
Figure 2. Diagram of pathogen composition based on the proportion of each pathogen in all pathogens in each season.
ANOSIM test showed that distinct differences were observed for pathogenic community composition among four seasons (r = 0.16, p = 0.00). Except between summer and autumn/winter, significant differences in pathogenic communities were observed between the other seasons (Table 1). In details, Actinomyces, Campylobacter, Chryseobacterium, Vibrio, and Rickettsia, were enriched in spring, while the relative abundances of Plesiomonas shigelloides and Prevotella were lower than that in other seasons (Figure 2 and Supplementary Figure S2). Similarly, Granulicatella, Capnocytophaga, Prevotella, Serratia marcescens, Nocardia brasiliensis, Chromobacterium were abundant in summer, while Actinomyces and Streptococcus were less abundant than other seasons. Shewanella, Escherichia-Shigella, Bacteroides fragilis, Citrobacter, and Erysipelothrix were enriched in winter, while a lower relative abundance was for Mycobacterium compared with other seasons. There was no enrichment of pathogens in autumn, whereas Campylobacter was noticeably less abundant than other seasons.
3.3 Interactions between airborne pathogens in different seasons
The interactions between human-to-human pathogens were evaluated for each season. Except spring, most of pathogens (12–17) in the other seasons (in particular summer) were closely related (Figure 3, Table 2, and Supplementary Table S2). The total number of edges in summer, autumn, winter, was 46, 26, and 22, respectively. Moreover, Plesiomonas shigelloides and Bacteroides fragilis played the core role in the interaction network in summer. Aeromonas, Plesiomonas shigelloides, Mycobacterium, Escherichia-Shigella, and Prevotella were the hub pathogens in autumn, and Erysipelothrix and Plesiomonas shigelloides was also the key pathogen in winter. Among these hub pathogens, the relative abundances of some bacteria taxa like Bacteroides fragilis, Aeromonas, and Erysipelothrix was less than 0.10%.
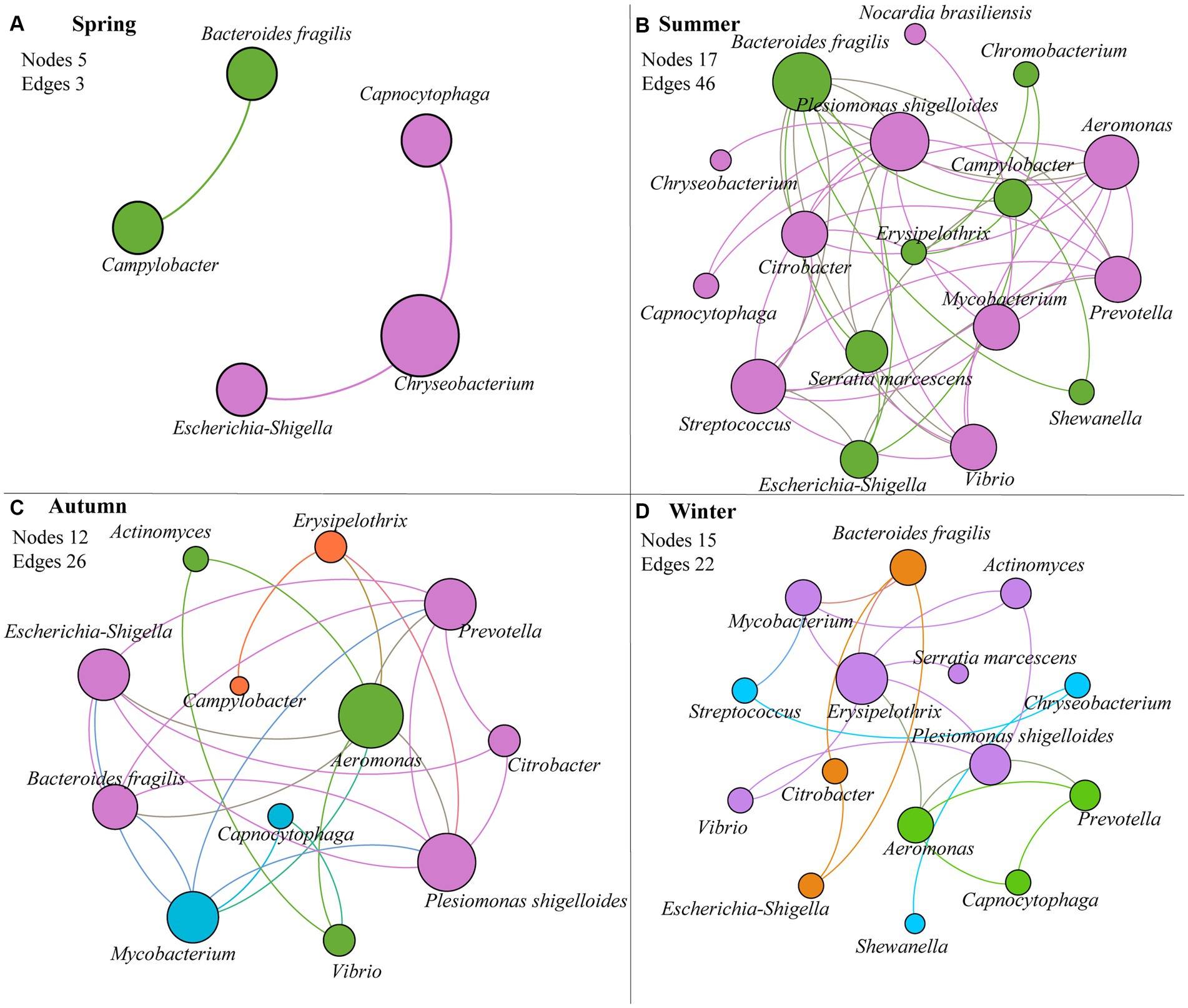
Figure 3. Co-occurrence interaction network between pathogens based on Spearman’s correlation (p < 0.05) of their relative abundances in each season. (A) spring; (B) summer; (C) autumn; (D) winter. Node size is proportional to node degree; edges represent interactions between nodes. The nodes (pathogens) with the same color are from the same modularity class.
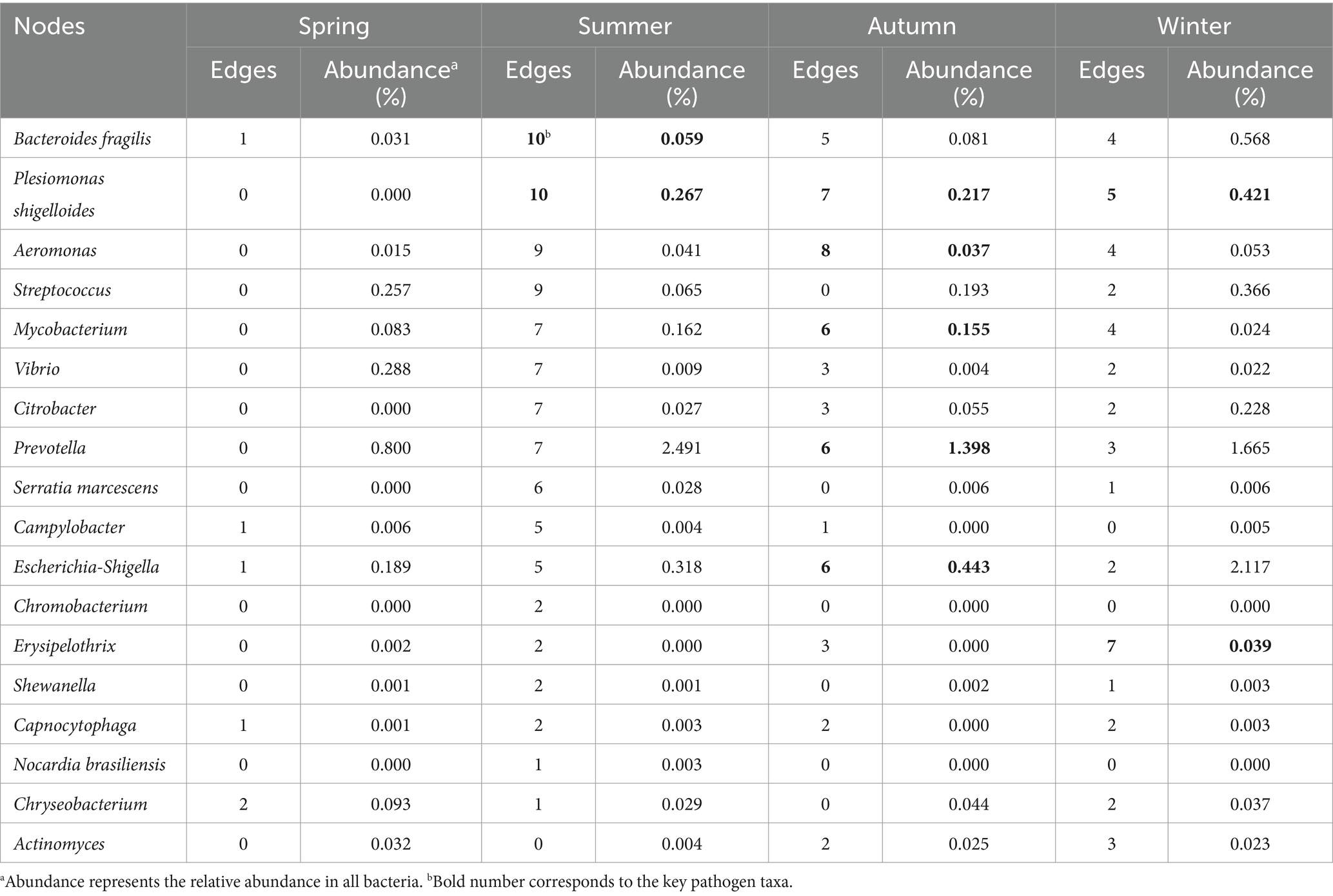
Table 2. Edge number and relative abundance of each node in pathogen-pathogen co-occurrence network.
3.4 Potential ARGs carried by airborne pathogens
There were 35 high-risk ARG subtypes (Rank I and Rank II) predicted in the atmosphere in Nanchang. The predominant ARGs (gene counts >5,000) included bacA (β-lactam), catA (chloramphenicol), mdtA (multidrug), tetM (tetracycline), mepA (multidrug), and vanY (vancomycin). T-test showed that there were significant differences on the total abundance of ARGs between seasons, except between winter and summer, and the total abundance of ARGs followed the pattern, summer > winter > autumn > spring. Furthermore, human-to-human pathogens were significantly associated with these ARGs, and each pathogen carried an average of 7.54 ARG subtypes (Figure 4). The above core pathogens like Plesiomonas shigelloides, Escherichia-Shigella, and Bacteroides fragilis were positively related with more than 10 ARG subtypes, respectively. Moreover, these ARGs were affiliated with more than 3 antibiotic types.
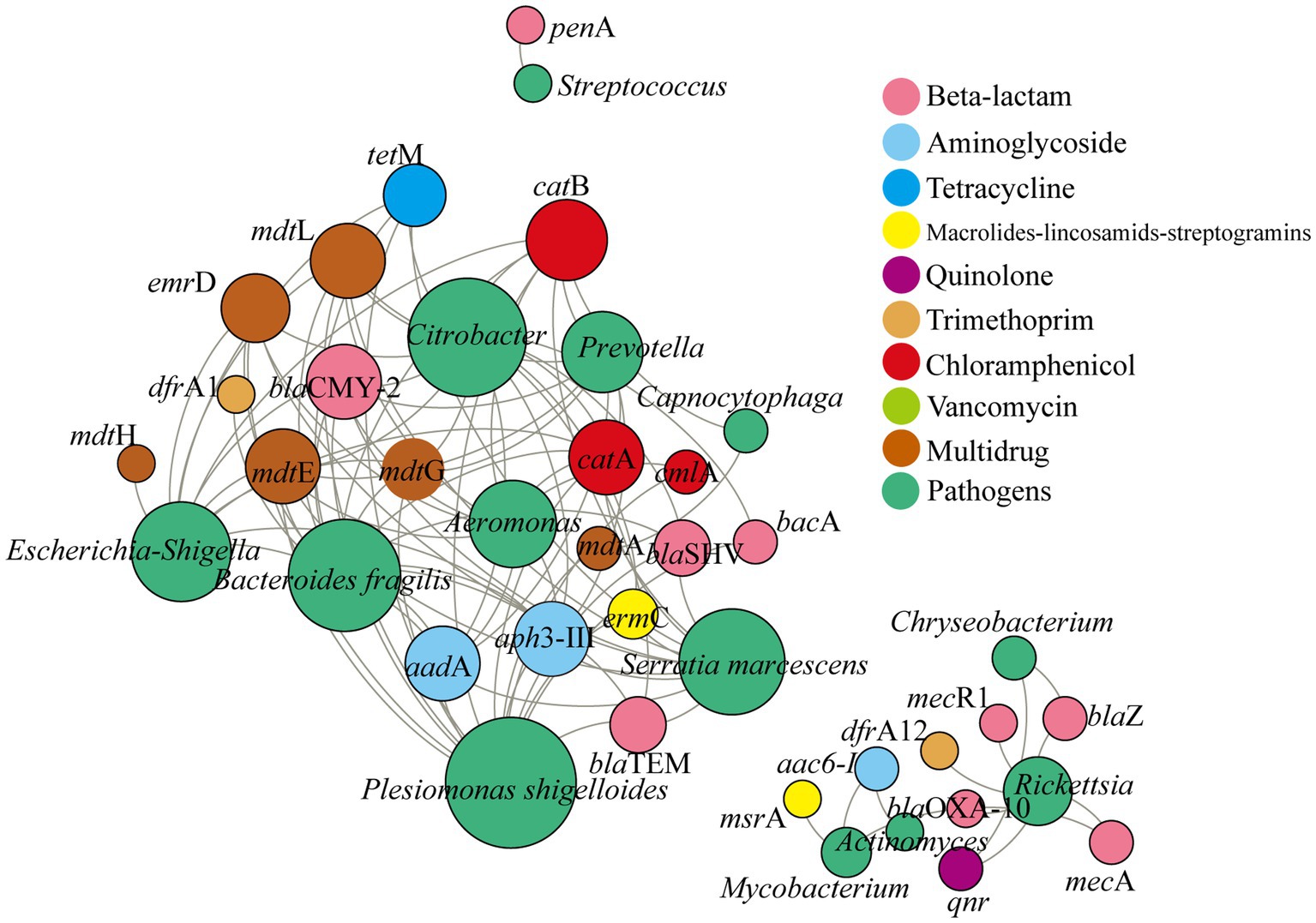
Figure 4. Co-occurrence network analysis between pathogens and potential ARG subtypes based on Spearman correlation between the relative abundance of pathogens and gene counts of ARGs subtypes (predicted by PICRUSt). Only the positive and significant correlations between pathogens and ARGs were displayed. The size of each node is proportional the degree, and the color represents the antibiotic types and pathogens.
3.5 Environmental factors shaped airborne pathogens
Mantel test showed that environmental factors shaped the pathogenic community in PM2.5 (r = 0.15, p = 0.01), in particular CO, O3, PM2.5, T, and RH. Both air pollutants and meteorological conditions significantly influenced the composition of pathogens (rpollutants = 0.142, ppollutants = 0.01; rmeteorology = 0.109, pmeteorology = 0.04). Moreover, the RDA results suggested that environmental variables totally explained 26.38% variation of pathogenic community (Figure 5). Air pollutants and meteorological conditions explained 19.91 and 15.12%, respectively. Spearman correlation analysis also found that different pathogens were influenced by environmental factors in different patterns (Figure 6). For example, meteorological conditions (relative humidity and precipitation) contributed to the diffusion of Plesiomonas shigelloides, Aeromonas, and Bacteroides fragilis in the air, whereas air pollutants enhanced the dissemination of Mycobacterium, Streptococcus, and Actinomyces.
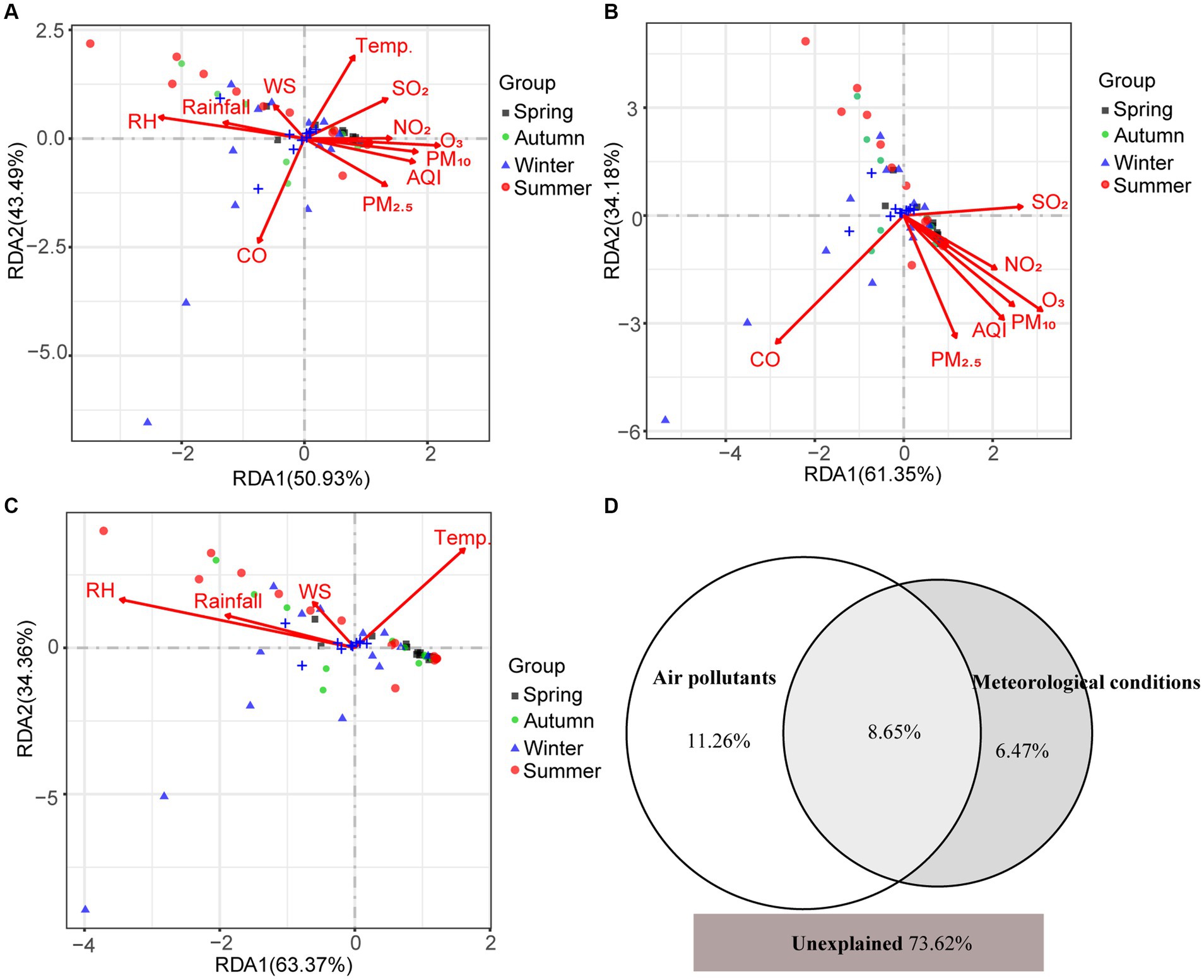
Figure 5. The contribution of environmental factors to variation of airborne pathogens. (A) RDA analysis between environmental factors and pathogen community. (B) RDA analysis between air pollutants and pathogen community. (C) RDA analysis between meteorological conditions and pathogen community. (D) Venn diagram for the contribution of air pollutants and meteorological conditions to variation of airborne pathogens by partial RDA. The RDA1 and RDA2 in (A–C) refer to the proportion of the constrained portion.
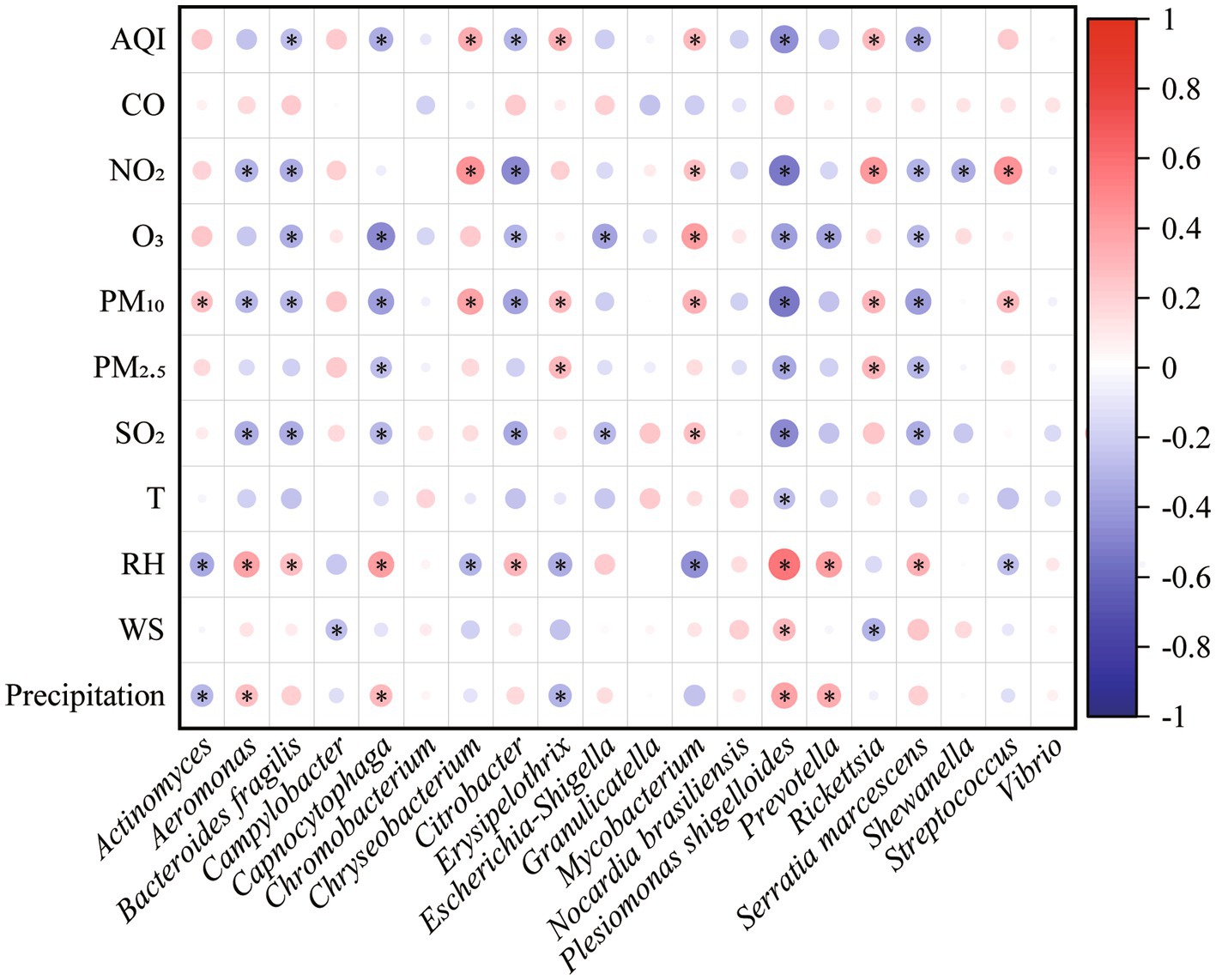
Figure 6. Spearman correlations of specific pathogen with environmental factors. The circle size is proportional to the spearman correlation coefficient. Red color represents positive correlation while blue color represents negative correlation. * indicates p < 0.05.
4 Discussion
The seasonal characteristics of airborne microbial community have been extensively studied, and most of studies showed that air quality and meteorological conditions shaped the microbial community (Li et al., 2019; Files et al., 2020; Ruiz-Gil et al., 2020). Several human pathogens had been identified in the airborne particles (Du et al., 2018; Lu et al., 2018; Fan et al., 2019). Nevertheless, the seasonal characteristics of airborne pathogens and their potential health risk remained unclear. In this study, we found that human-to-human pathogens in winter had the highest proportion than that in other seasons (Figure 1), consistent with the result of Du et al. (2018) and Fan et al. (2019). It suggested that airborne pathogens posed greater threat to human in winter than the other seasons. Although the low temperature in winter was not conducive for microbial reproduction, high relative humidity (80.62%) favored the survival of microorganisms, which was confirmed by the high correlation between relative humidity and relative abundance of pathogens (r = 0.44, p < 0.01).
Some human pathogens such as Prevotella, Escherichia-Shigella, and Streptococcus, were frequently detected in the atmosphere (Lu et al., 2018; Fan et al., 2019; Jiang et al., 2022), indicating that they were resident airborne pathogens in the urban. Prevotella have been implicated in multiple diseases including opportunistic infections, bacterial vaginosis, oral biofilms, etc. (Tett et al., 2021). Escherichia-Shigella are generally regarded as proinflammatory bacteria and associated with gut dysbiosis (Li et al., 2022). Streptococcus are usually associated with bacteremia, acute respiratory distress syndrome, neonatal sepsis and meningitis (Hughes et al., 2009; Yacoub et al., 2014). However, part of pathogens showed seasonal preference (Figure 2 and Supplementary Figure S2), and then lead to the seasonal heterogeneity (Table 1). For instance, the dominant pathogen Plesiomonas shigelloides was most abundant in winter (0.44%), which also can cause intestinal diseases, sepsis and meningitis (Ingo, 2004). Chryseobacterium was enriched in spring (0.09%), Prevotella in summer (2.49%), and Actinomyces in autumn (0.02%), respectively. Even, Rickettsia was spring-specific pathogen and not detected in other seasons, which has been reported to cause many human diseases, including spotted fever, epidemic typhus, and Brill-Zinsser disease (Kelly et al., 2002; Perlman et al., 2006). Similar with the pathogen abundance, the pathogen composition also showed seasonal distribution.
Airborne microorganisms usually showed strong interactions (Fan et al., 2019; Jiang et al., 2022). In this study, we found that human pathogens in PM2.5 also were associated with each other (Figure 3 and Table 2), especially pathogens in summer (Supplementary Table S2). In general, significantly related pathogens may be from the same source (Pan et al., 2022). For instance, Plesiomonas shigelloides – Aeromonas – Prevotella had strongly positive correlation with each other in all seasons except spring, and they were widely distributed in aquatic environments (Fernández-Bravo and Figueras, 2020; Jang et al., 2023). Notably, the predominant pathogen Plesiomonas shigelloides was also the hub pathogen in three seasons, thus it could be used as a biomarker to monitor air pollution by pathogens.
In addition, more than half of airborne pathogens were promising hosts of high-risk ARGs (Figure 4). Moreover, some pathogens may carry multiple type of ARGs and be multi-resistant pathogens. Plesiomonas shigelloides had significantly correlation with ARGs belonging to beta-lactam, chloramphenicol, multidrug resistance, etc., the same as Bacteroides fragilis, Escherichia-Shigella, Citrobacter, and Aeromonas. These were consistent with previous studies (Ekundayo and Okoh, 2020; Norouzi Bazgir et al., 2020; Zhu et al., 2020; Li et al., 2021; Hassoubah, 2023). For instance, Ekundayo and Okoh (2020) have reported that Plesiomonas shigelloides in freshwater showed resistance to antibiotics and part of them had multi-resistance. Similarly, Bacteroides fragilis was previously found to be multi resistant to chloramphenicol, tetracyclines, macrolides-lincosamides-streptogramins, etc. (Niestępski et al., 2019). Li et al. (2021) found that both Escherichia and Bacteroides were the main ARG carriers in the hospital air dust. Notably, these multi-resistant pathogens tended to be more abundant in winter (Figure 2), suggesting that particulate matter in winter posed higher health risks to human.
Both air pollutants and meteorological conditions affected the seasonal distribution of pathogenic bacteria in the atmosphere (Figures 5, 6). The positive correlations between pathogens (e.g., Mycobacterium and Streptococcus) and anthropogenic activities were supported by a previous study (Fan et al., 2019). Bacteroides fragilis, Prevotella, and Plesiomonas shigelloides were usually found in water. This characteristic may explain their positive correlations with precipitation and relative humidity, which enhance the aerosolization of bacteria from water (Zhen et al., 2017). Therefore, the low temperature (6.92°C) and high relative humidity (80.62%) in winter promoted the enrichment of Plesiomonas shigelloides (Figure 2). Similarly, high PM10 concentration (77.36 μg/m3) and low relative humidity (70.95%) made Chryseobacterium relatively abundant in spring. Nevertheless, different from the effect of environmental factors on bacterial community (Zhen et al., 2017; Fan et al., 2019), air pollutants (19.91%) played more important role on variations of airborne pathogens than meteorological conditions (15.12%). It highlighted the effects of air quality on airborne pathogens. Overall, there were still a large proportion of variations could not be explained, indicating that some other factors might shape airborne pathogenic community. For example, some biotoxin substances absorbed on atmospheric particulate matter, such as heavy metals and volatile organic compounds, have been reported to have impacts on airborne microbial community (Li et al., 2023; Ma et al., 2023). Our findings can advance people’s knowledge of the health risks of airborne particles and manage daily production more pertinently to reduce the health risks.
This study also has some limitations. Although the reliability of PICRUSt has been validated by numerous studies using metagenomic shotgun sequencing (Zaura et al., 2015; Šamanic et al., 2021; Zhang L. et al., 2021), we applied PICRUSt to predict functional genes based on 16S rRNA gene sequencing in this study. It cannot cover the full diversity of microorganisms, and some ARGs in PM2.5 were extracellular. In addition, because only V3–V4 region of 16S rRNA gene was sequenced, not all pathogens can be detected at the species level. Also, a longer sampling period would be expected in the future study. Thus, the relative abundance of pathogenic bacteria may be underestimated. In the future, using metagenomic shotgun sequencing to confirm the results is necessary.
5 Conclusion
Airborne human-to-human pathogens in PM2.5 showed seasonal characteristics. The highest relative abundance of pathogens was observed in winter. Although more than half of pathogens were shared by four seasons, the pathogenic community structure shifted by season. Several pathogens tended to enrich in specific season. However, most of pathogens were obviously correlated with each other. Pathogens such as Bacteroides fragilis, Aeromonas, Plesiomonas shigelloides were the hub pathogens and not related with their abundances. Furthermore, these hub pathogens may be multidrug-resistant bacteria, which would pose a heavy threat on human health. Given that Plesiomonas shigelloides was the core pathogen in three seasons and it may be multi-resistant pathogen, it might be used as a biomarker to monitor air pollution by pathogens. Additionally, both air pollutants and meteorological conditions could partially but obviously explain the seasonal variation of pathogens.
Data availability statement
The datasets presented in this study can be found in online repositories. The names of the repository/repositories and accession number(s) can be found in the article/Supplementary material.
Author contributions
ZZ: Data curation, Formal analysis, Writing – original draft. YP: Funding acquisition, Investigation, Supervision, Writing – review & editing. XH: Data curation, Investigation, Writing – review & editing. TY: Writing – review & editing, Funding acquisition. CL: Writing – review & editing. XC: Writing – review & editing. ZX: Writing – review & editing, Formal analysis, Methodology, Supervision.
Funding
The author(s) declare that financial support was received for the research, authorship, and/or publication of this article. This work was supported by the National Natural Science Foundation of China (No. 31900106), and Experimental Technology Research and Development Project of East China University of Technology (1310610091).
Conflict of interest
The authors declare that the research was conducted in the absence of any commercial or financial relationships that could be construed as a potential conflict of interest.
Publisher’s note
All claims expressed in this article are solely those of the authors and do not necessarily represent those of their affiliated organizations, or those of the publisher, the editors and the reviewers. Any product that may be evaluated in this article, or claim that may be made by its manufacturer, is not guaranteed or endorsed by the publisher.
Supplementary material
The Supplementary material for this article can be found online at: https://www.frontiersin.org/articles/10.3389/fmicb.2024.1422637/full#supplementary-material
Footnotes
References
Bowers, R. M., McLetchie, S., Knight, R., and Fierer, N. (2011). Spatial variability in airborne bacterial communities across land-use types and their relationship to the bacterial communities of potential source environments. ISME J. 5, 601–612. doi: 10.1038/ismej.2010.167
Callewaert, C., Helffer, K. R., and Lebaron, P. (2020). Skin microbiome and its interplay with the environment. Am. J. Clin. Dermatol. 21, 4–11. doi: 10.1007/s40257-020-00551-x
Du, P., Du, R., Ren, W., Lu, Z., and Fu, P. (2018). Seasonal variation characteristic of inhalable microbial communities in PM2.5 in Beijing city. China. Sci. Total Environ. 610-611, 308–315. doi: 10.1016/j.scitotenv.2017.07.097
Edgar, R. C. (2013). UPARSE: highly accurate OTU sequences from microbial amplicon reads. Nat. Methods 10, 996–998. doi: 10.1038/nmeth.2604
Ekundayo, T. C., and Okoh, A. I. (2020). Antimicrobial resistance in freshwater Plesiomonas shigelloides isolates: implications for environmental pollution and risk assessment. Environ. Pollut. 257:113493. doi: 10.1016/j.envpol.2019.113493
Fan, X., Gao, J., Pan, K., Li, D., Dai, H., and Li, X. (2019). More obvious air pollution impacts on variations in bacteria than fungi and their co-occurrences with ammonia-oxidizing microorganisms in PM2.5. Environ. Pollut. 251, 668–680. doi: 10.1016/j.envpol.2019.05.004
Fernández-Bravo, A., and Figueras, M. J. (2020). An update on the genus Aeromonas: taxonomy, epidemiology, and pathogenicity. Microorganisms. 8:129. doi: 10.3390/microorganisms8010129
Files, E. J., Clarke, L. J., Brook, B. W., and Jones, P. (2020). Urbanisation reduces the abundance and diversity of airborne microbes - but what does that mean for our health? A systematic review. Sci. Total Environ. 738:140337. doi: 10.1016/j.scitotenv.2020.140337
Hassoubah, S. A. (2023). Genetic analysis of multidrug-resistant and AmpC-producing Citrobacter freundii. Eur. Rev. Med. Pharmacol. Sci. 27, 1147–1154. doi: 10.26355/eurrev_202302_31221
Hughes, J. M., Wilson, M. E., Wertheim, H. F. L., Nghia, H. D. T., Taylor, W., and Schultsz, C. (2009). Streptococcus suis: an emerging human pathogen. Clin. Infect. Dis. 48, 617–625. doi: 10.1086/596763
Ingo, S. (2004). Plesiomonas shigelloides an emerging pathogen with unusual properties. Rev. Med. Microbiol. 15, 129–139. doi: 10.1097/00013542-200410000-00002
Jang, J., Abbas, A., Kim, H., Rhee, C., Shin, S. G., Chun, J. A., et al. (2023). Prediction and interpretation of pathogenic bacteria occurrence at a recreational beach using data-driven algorithms. Ecol. Inform. 78:102370. doi: 10.1016/j.ecoinf.2023.102370
Jiang, X., Wang, C., Guo, J., Hou, J., Guo, X., and Zhang, H. (2022). Global meta-analysis of airborne bacterial communities and associations with anthropogenic activities. Environ. Sci. Technol. 56, 9891–9902. doi: 10.1021/acs.est.1c07923
Kelly, D. J., Richards, A. L., Temenak, J., Strickman, D., and Dasch, G. A. (2002). The past and present threat of Rickettsial diseases to military medicine and international public health. Clin. Infect. Dis. 34, S145–S169. doi: 10.1086/339908
Li, J., Cao, J., Zhu, Y., Chen, Q., Shen, F., Wu, Y., et al. (2018). Global survey of antibiotic resistance genes in air. Environ. Sci. Technol. 52, 10975–10984. doi: 10.1021/acs.est.8b02204
Li, S., Guo, J., Liu, R., Zhang, F., Wen, S., Liu, Y., et al. (2022). Predominance of Escherichia-Shigella in gut microbiome and its potential correlation with elevated level of plasma tumor necrosis factor alpha in patients with tuberculous meningitis. Microbiol. Spectrum. 10, 1–14. doi: 10.1128/spectrum.01926-22
Li, H., Hong, Y., Gao, M., An, X., Yang, X., Zhu, Y., et al. (2023). Distinct responses of airborne abundant and rare microbial communities to atmospheric changes associated with Chinese new year. Imeta 2:e140. doi: 10.1002/imt2.140
Li, X., Wu, Z., Dang, C., Zhang, M., Zhao, B., Cheng, Z., et al. (2021). A metagenomic-based method to study hospital air dust resistome. Chem. Eng. J. 406:126854. doi: 10.1016/j.cej.2020.126854
Li, H., Zhou, X., Yang, X., Zhu, Y., Hong, Y., and Su, J. (2019). Spatial and seasonal variation of the airborne microbiome in a rapidly developing city of China. Sci. Total Environ. 665, 61–68. doi: 10.1016/j.scitotenv.2019.01.367
Lu, R., Li, Y., Li, W., Xie, Z., Fan, C., Liu, P., et al. (2018). Bacterial community structure in atmospheric particulate matters of different sizes during the haze days in Xi'an. China. Sci. Total Environ. 637-638, 244–252. doi: 10.1016/j.scitotenv.2018.05.006
Ma, L., Yabo, S. D., Lu, L., Jiang, J., Meng, F., and Qi, H. (2023). Seasonal variation characteristics of inhalable bacteria in bioaerosols and antibiotic resistance genes in Harbin. J. Hazard. Mater. 446:130597. doi: 10.1016/j.jhazmat.2022.130597
Magoč, T., and Salzberg, S. L. (2011). FLASH: fast length adjustment of short reads to improve genome assemblies. Bioinformatics 27, 2957–2963. doi: 10.1093/bioinformatics/btr507
National Health Commission of the People’s Republic of China . List of human pathogenic microorganisms, (2023), 8, 18. Available at: http://www.nhc.gov.cn/qjjys/s7948/202308/b6b51d792d394fbea175e4c8094dc87e.shtml
Niestępski, S., Harnisz, M., Korzeniewska, E., Aguilera-Arreola, M. G., Contreras-Rodríguez, A., Filipkowska, Z., et al. (2019). The emergence of antimicrobial resistance in environmental strains of the Bacteroides fragilis group. Environ. Int. 124, 408–419. doi: 10.1016/j.envint.2018.12.056
Norouzi Bazgir, Z., Mirzaei, B., Haghshenas, M.R., Goli, H.R., and Shafaie, E.. (2020). Multi-drug resistant Citrobacter freundii isolates in a burn hospital in Northeast of Iran: a single-center cross-sectional study. Research in Molecular Medicine. 8, 63–70.
Pan, Y., Xiao, H., Xiao, H., and Zhu, R. (2022). Distribution characteristics of airborne bacterial community in different anthropogenic activity regions across Nanchang. China. Atmos. Pollut. Res. 13:101610. doi: 10.1016/j.apr.2022.101610
Pan, Y., Zhang, W., Xu, Z., Zuo, Z., and Yuan, T. (2024). Fungal community shows more variations by season and particle size than bacteria. Sci. Total Environ. 925:171584. doi: 10.1016/j.scitotenv.2024.171584
Perlman, S. J., Hunter, M. S., and Zchori-Fein, E. (2006). The emerging diversity of Rickettsia. Proc. Biol. Sci. 273, 2097–2106.
Pruesse, E., Quast, C., Knittel, K., Fuchs, B. M., Ludwig, W., Peplies, J., et al. (2007). SILVA: a comprehensive online resource for quality checked and aligned ribosomal RNA sequence data compatible with ARB. Nucleic Acids Res. 35, 7188–7196. doi: 10.1093/nar/gkm864
Riggs, D. W., Yeager, R. A., and Bhatnagar, A. (2018). Defining the human envirome: an omics approach for assessing the environmental risk of cardiovascular disease. Circ. Res. 122, 1259–1275. doi: 10.1161/CIRCRESAHA.117.311230
Ruiz-Gil, T., Acuña, J. J., Fujiyoshi, S., Tanaka, D., Noda, J., Maruyama, F., et al. (2020). Airborne bacterial communities of outdoor environments and their associated influencing factors. Environ. Int. 145:106156. doi: 10.1016/j.envint.2020.106156
Šamanic, I., Kalinić, H., Fredotović, Ž., Dzelalija, M., Bungur, A. M., and Maravić, A. (2021). Bacteria tolerant to colistin in coastal marine environment: detection, microbiome diversity and antibiotic resistance gene’s repertoire. Chemosphere 281:130945. doi: 10.1016/j.chemosphere.2021.130945
Tang, K., Sánchez-Parra, B., Yordanova, P., Wehking, J., Backes, A. T., Pickersgill, D. A., et al. (2022). Bioaerosols and atmospheric ice nuclei in a Mediterranean dryland: community changes related to rainfall. Biogeosciences 19, 71–91. doi: 10.5194/bg-19-71-2022
Tett, A., Pasolli, E., Masetti, G., Ercolini, D., and Segata, N. (2021). Prevotella diversity, niches and interactions with the human host. Nat. Rev. Microbial. 19, 585–599. doi: 10.1038/s41579-021-00559-y
Wei, M., Xu, C., Chen, J., Zhu, C., Li, J., and Lv, G. (2017). Characteristics of bacterial community in cloud water at Mt tai: similarity and disparity under polluted and non-polluted cloud episodes. Atmos. Chem. Phys. 17, 5253–5270. doi: 10.5194/acp-17-5253-2017
Xue, Y., Chu, J., Li, Y., and Kong, X. (2020). The influence of air pollution on respiratory microbiome: a link to respiratory disease. Toxicol. Lett. 334, 14–20. doi: 10.1016/j.toxlet.2020.09.007
Yacoub, A. T., Mojica, L., Jones, L., Knab, A., Alrabaa, S., and Greene, J. (2014). The role of corticosteroids in adult respiratory distress syndrome caused by viridans group streptococci bacteremia in neutropenic patients. Mediterr. J. Hematol. Infect. Dis. 6:e2014055. doi: 10.4084/mjhid.2014.055
Yan, X., Ma, J., Chen, X., Lei, M., Li, T., and Han, Y. (2022). Characteristics of airborne bacterial communities and antibiotic resistance genes under different air quality levels. Environ. Int. 161:107127. doi: 10.1016/j.envint.2022.107127
Zaura, E., Brandt, B. W., Teixeira de Mattos, M. J., Buijs, M. J., Caspers, M. P., Rashid, M. U., et al. (2015). Same exposure but two radically different responses to antibiotics: resilience of the salivary microbiome versus long-term microbial shifts in feces. MBio 6:e01693. doi: 10.1128/mBio.01693-15
Zeng, S., Xu, Z., Wang, X., Liu, W., Qian, L., Chen, X., et al. (2019). Time series analysis of antibacterial usage and bacterial resistance in China: observations from a tertiary hospital from 2014 to 2018. Infect. Drug Resist. 12, 2683–2691. doi: 10.2147/IDR.S220183
Zhang, A., Gaston, J. M., Dai, C. L., Zhao, S., Poyet, M., Groussin, M., et al. (2021). An omics-based framework for assessing the health risk of antimicrobial resistance genes. Nat. Commun. 12:4765. doi: 10.1038/s41467-021-25096-3
Zhang, L., Shen, L., Ju, Z., Fu, Y., Qin, S., and Cui, J. (2021). The key environmental influencing factors for the change of sediment bacterial community and antibiotics resistance genes in a long-term polluted lake, China. Ecotoxicology 30, 1538–1549. doi: 10.1007/s10646-020-02309-x
Zhen, Q., Deng, Y., Wang, Y., Wang, X., Zhang, H., Sun, X., et al. (2017). Meteorological factors had more impact on airborne bacterial communities than air pollutants. Sci. Total Environ. 601-602, 703–712. doi: 10.1016/j.scitotenv.2017.05.049
Zhu, G., Wang, X., Yang, T., Su, J., Qin, Y., Wang, S., et al. (2021). Air pollution could drive global dissemination of antibiotic resistance genes. ISME J. 15, 270–281. doi: 10.1038/s41396-020-00780-2
Keywords: PM2.5, human-to-human pathogens, seasonal variation, hub pathogens, antibiotic resistance genes (ARGs)
Citation: Zuo Z, Pan Y, Huang X, Yuan T, Liu C, Cai X and Xu Z (2024) Seasonal distribution of human-to-human pathogens in airborne PM2.5 and their potential high-risk ARGs. Front. Microbiol. 15:1422637. doi: 10.3389/fmicb.2024.1422637
Edited by:
Hazem Ramadan, Mansoura University, EgyptReviewed by:
Mohamed Samir, The Pirbright Institute, United KingdomDong Yang, Tianjin Institute of Environmental and Operational Medicine, China
Copyright © 2024 Zuo, Pan, Huang, Yuan, Liu, Cai and Xu. This is an open-access article distributed under the terms of the Creative Commons Attribution License (CC BY). The use, distribution or reproduction in other forums is permitted, provided the original author(s) and the copyright owner(s) are credited and that the original publication in this journal is cited, in accordance with accepted academic practice. No use, distribution or reproduction is permitted which does not comply with these terms.
*Correspondence: Yuanyuan Pan, cGFueXl1YW5AZWN1dC5lZHUuY24=; Zhongji Xu, eHV6aG9uZ2ppMjAxOEAxNjMuY29t